⚠️ 以下所有内容总结都来自于 大语言模型的能力,如有错误,仅供参考,谨慎使用
🔴 请注意:千万不要用于严肃的学术场景,只能用于论文阅读前的初筛!
💗 如果您觉得我们的项目对您有帮助 ChatPaperFree ,还请您给我们一些鼓励!⭐️ HuggingFace免费体验
2024-12-11 更新
Towards Long Video Understanding via Fine-detailed Video Story Generation
Authors:Zeng You, Zhiquan Wen, Yaofo Chen, Xin Li, Runhao Zeng, Yaowei Wang, Mingkui Tan
Long video understanding has become a critical task in computer vision, driving advancements across numerous applications from surveillance to content retrieval. Existing video understanding methods suffer from two challenges when dealing with long video understanding: intricate long-context relationship modeling and interference from redundancy. To tackle these challenges, we introduce Fine-Detailed Video Story generation (FDVS), which interprets long videos into detailed textual representations. Specifically, to achieve fine-grained modeling of long-temporal content, we propose a Bottom-up Video Interpretation Mechanism that progressively interprets video content from clips to video. To avoid interference from redundant information in videos, we introduce a Semantic Redundancy Reduction mechanism that removes redundancy at both the visual and textual levels. Our method transforms long videos into hierarchical textual representations that contain multi-granularity information of the video. With these representations, FDVS is applicable to various tasks without any fine-tuning. We evaluate the proposed method across eight datasets spanning three tasks. The performance demonstrates the effectiveness and versatility of our method.
长视频理解已成为计算机视觉中的一项关键任务,推动了从监控到内容检索等多个应用的发展。现有的视频理解方法在处理长视频理解时面临两个挑战:复杂的长上下文关系建模和冗余信息的干扰。为了解决这些挑战,我们引入了精细详细视频故事生成(FDVS)方法,它将长视频解释为详细的文本表示。具体来说,为了实现长时内容的精细建模,我们提出了自下而上的视频解释机制,该机制逐步从片段到视频解释视频内容。为了避免视频中的冗余信息造成的干扰,我们引入了语义冗余减少机制,该机制在视觉和文本层面消除了冗余。我们的方法将长视频转换为层次化的文本表示形式,包含视频的多粒度信息。利用这些表示形式,FDVS可广泛应用于各种任务,无需进行微调。我们在三个任务的八个数据集上评估了所提出的方法。性能结果证明了我们的方法的有效性和通用性。
论文及项目相关链接
Summary
长视频理解已成为计算机视觉中的关键任务,推动了从监控到内容检索等多个应用的发展。针对长视频理解,现有方法面临复杂的长上下文关系建模和冗余信息的干扰两大挑战。为解决这些问题,我们提出了精细详细视频故事生成(FDVS)方法,将长视频转化为详细的文本表示。通过自下而上的视频解释机制和语义冗余减少机制,实现了对长视频内容的精细颗粒度建模和冗余信息的避免。该方法将长视频转化为层次化的文本表示,包含视频的多粒度信息,并适用于各种任务而无需微调。实验结果表明,该方法的有效性和通用性。
Key Takeaways
- 长视频理解在计算机视觉中至关重要,推动了多种应用的发展。
- 现有方法在长视频理解中面临两大挑战:复杂的长上下文关系建模和冗余信息的干扰。
- 提出的Fine-Detailed Video Story generation(FDVS)方法将长视频转化为详细的文本表示。
- 通过自下而上的视频解释机制实现长视频内容的精细颗粒度建模。
- 语义冗余减少机制避免了冗余信息的干扰。
- 该方法将长视频转化为层次化的文本表示,包含视频的多粒度信息。
点此查看论文截图
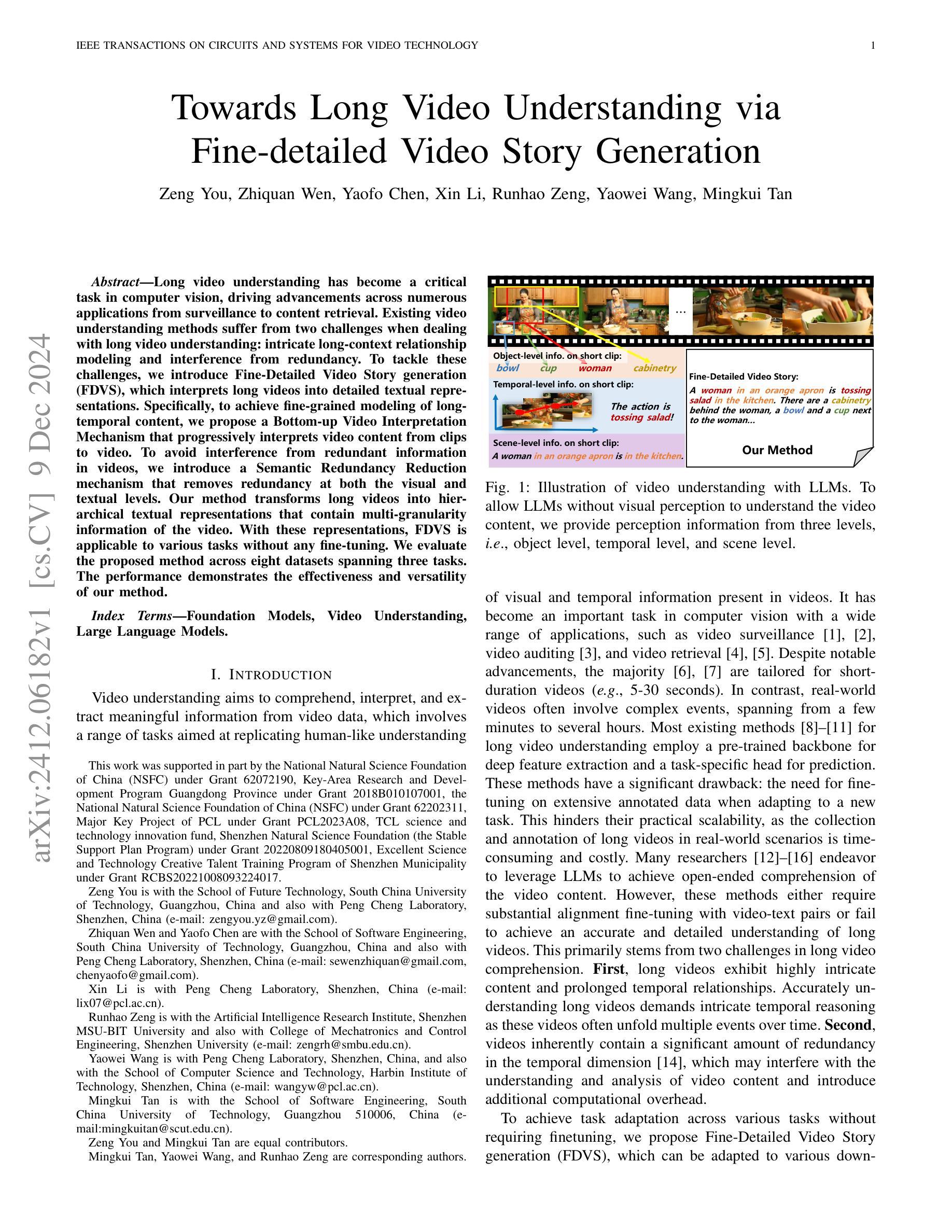
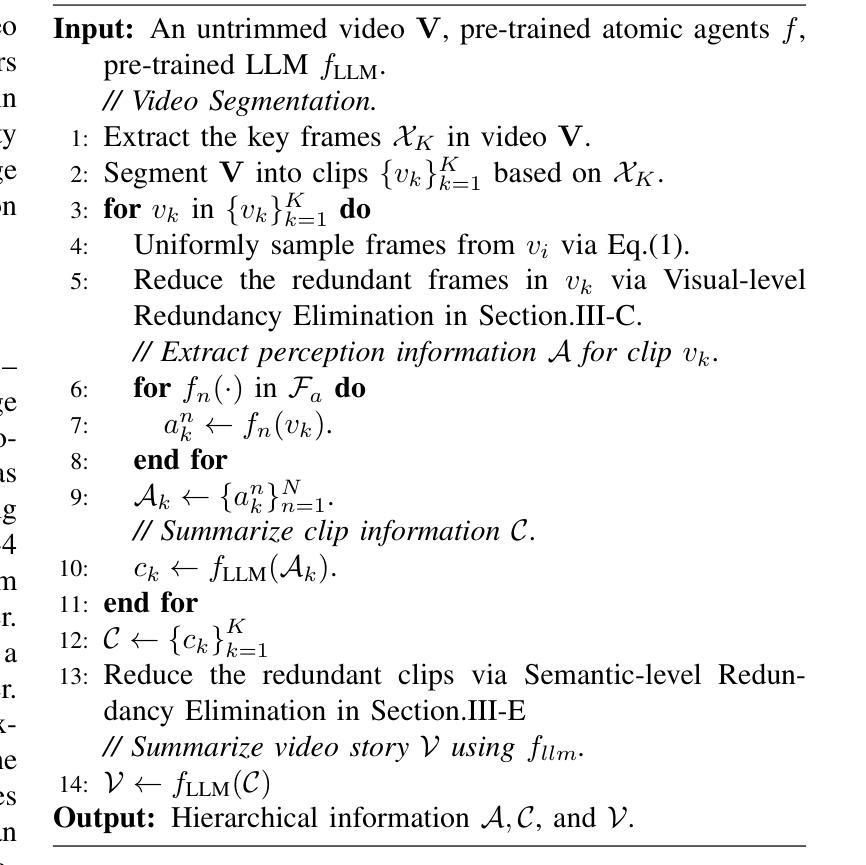
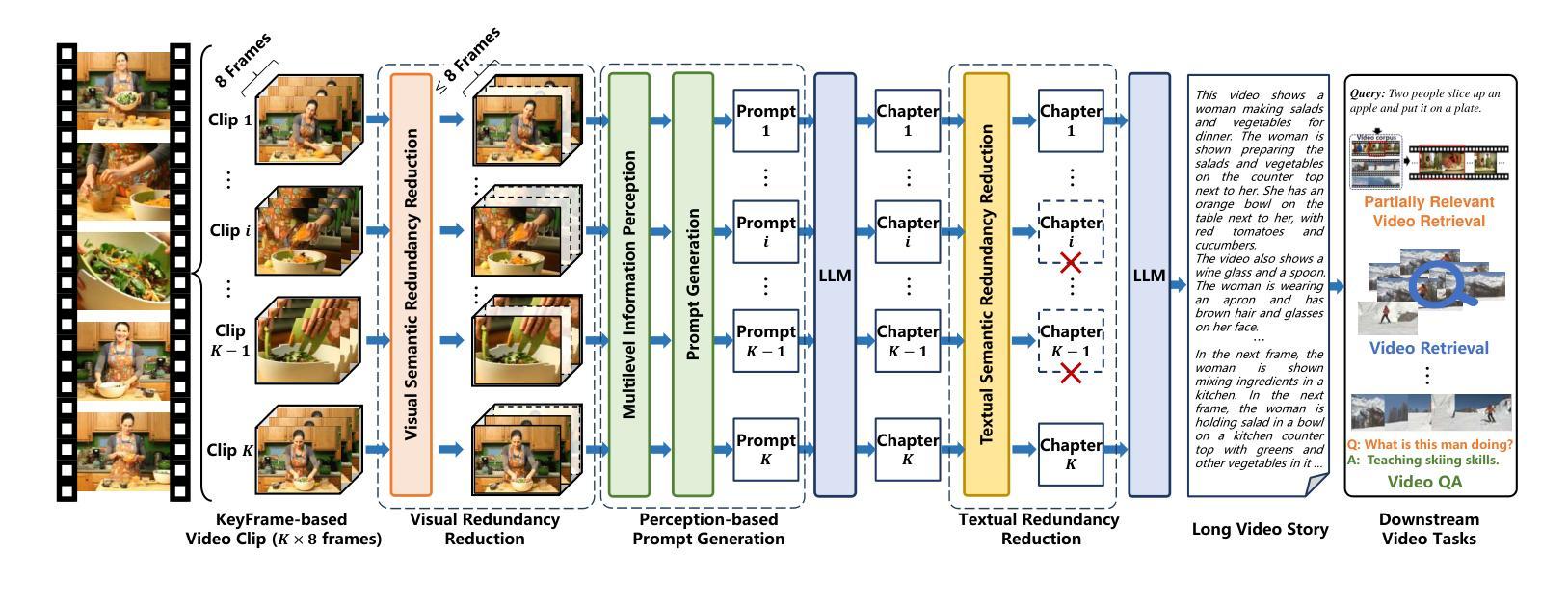
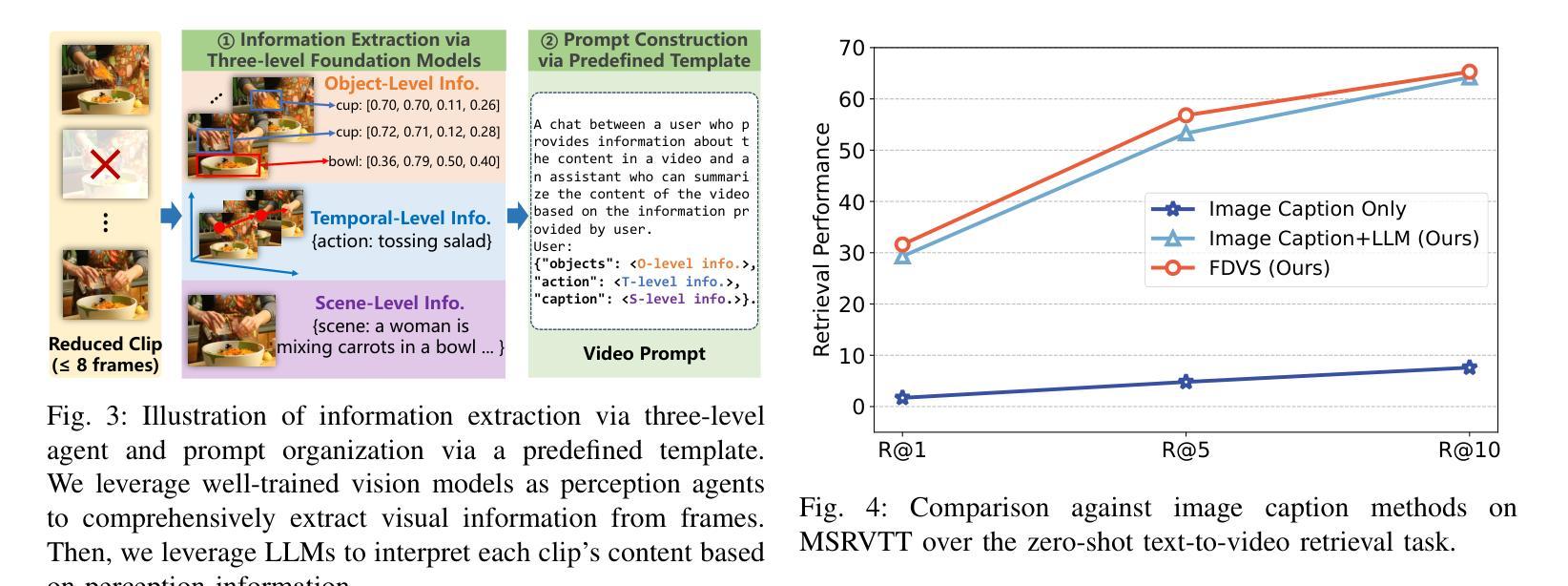
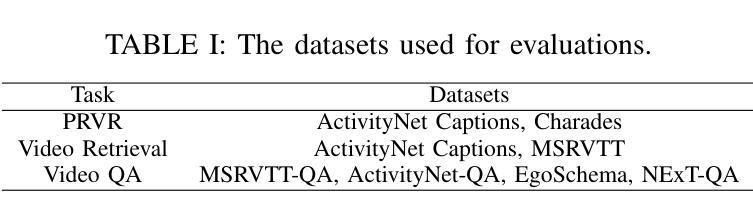
Holmes-VAU: Towards Long-term Video Anomaly Understanding at Any Granularity
Authors:Huaxin Zhang, Xiaohao Xu, Xiang Wang, Jialong Zuo, Xiaonan Huang, Changxin Gao, Shanjun Zhang, Li Yu, Nong Sang
How can we enable models to comprehend video anomalies occurring over varying temporal scales and contexts? Traditional Video Anomaly Understanding (VAU) methods focus on frame-level anomaly prediction, often missing the interpretability of complex and diverse real-world anomalies. Recent multimodal approaches leverage visual and textual data but lack hierarchical annotations that capture both short-term and long-term anomalies. To address this challenge, we introduce HIVAU-70k, a large-scale benchmark for hierarchical video anomaly understanding across any granularity. We develop a semi-automated annotation engine that efficiently scales high-quality annotations by combining manual video segmentation with recursive free-text annotation using large language models (LLMs). This results in over 70,000 multi-granular annotations organized at clip-level, event-level, and video-level segments. For efficient anomaly detection in long videos, we propose the Anomaly-focused Temporal Sampler (ATS). ATS integrates an anomaly scorer with a density-aware sampler to adaptively select frames based on anomaly scores, ensuring that the multimodal LLM concentrates on anomaly-rich regions, which significantly enhances both efficiency and accuracy. Extensive experiments demonstrate that our hierarchical instruction data markedly improves anomaly comprehension. The integrated ATS and visual-language model outperform traditional methods in processing long videos. Our benchmark and model are publicly available at https://github.com/pipixin321/HolmesVAU.
我们如何才能使模型理解发生在不同时间尺度和上下文中的视频异常?传统的视频异常理解(VAU)方法主要关注帧级的异常预测,往往忽略了复杂和多样化的真实世界异常的解读。最近的多模式方法利用视觉和文本数据,但缺乏能够捕捉短期和长期异常的层次化注释。为了解决这一挑战,我们推出了HIVAU-70k,这是一个用于任何粒度层次化视频异常理解的大规模基准测试。我们开发了一个半自动注释引擎,通过结合手动视频分割和基于大型语言模型(LLM)的递归文本注释,有效地扩展了高质量注释的规模。这产生了超过7万多个多粒度注释,按剪辑级别、事件级别和视频级别分段组织。为了在长视频中进行高效的异常检测,我们提出了以异常为重点的临时采样器(ATS)。ATS将异常评分者与密度感知采样器相结合,根据异常评分自适应地选择帧,确保多模式LLM专注于异常丰富的区域,这显著提高了效率和准确性。大量实验表明,我们的层次化指令数据显著提高了异常理解能力。我们集成的ATS和视觉语言模型在处理长视频时,表现优于传统方法。我们的基准测试和模型在https://github.com/pipixin321/HolmesVAU公开可用。
论文及项目相关链接
PDF 21 pages
Summary
为应对模型在不同时间尺度和上下文环境中理解视频异常的问题,研究引入了HIVAU-70k大规模基准测试集和半自动标注引擎。此外,还提出了针对长视频中异常检测问题的Anomaly-focused Temporal Sampler(ATS)。研究成果包括大规模基准测试集、半自动标注引擎和高效的异常检测采样方法,能有效提高模型的异常理解能力。
Key Takeaways
- 引入HIVAU-70k基准测试集:针对视频异常理解(VAU)的挑战,建立了一个大规模的基准测试集,用于评估模型在不同时间尺度和上下文中的异常理解能力。
- 半自动标注引擎:结合手动视频分割和基于大型语言模型(LLM)的递归自由文本标注,实现了高效的标注扩展。
- 多层次标注:提供了超过70,000个多层次标注,包括剪辑级别、事件级别和视频级别分段。
- Anomaly-focused Temporal Sampler(ATS):提出了一种针对长视频中异常检测的有效方法,通过结合异常评分者和密度感知采样器,自适应地选择关键帧进行集中处理。
- 提高了异常理解能力:通过大量实验证明,利用层次结构指令数据可以显著提高模型的异常理解能力。
- 多模态LLM的应用:结合异常评分者和多模态大型语言模型,提高了处理长视频的效率和准确性。
- 公开可用:基准测试和模型已公开在GitHub上供公众使用。
点此查看论文截图
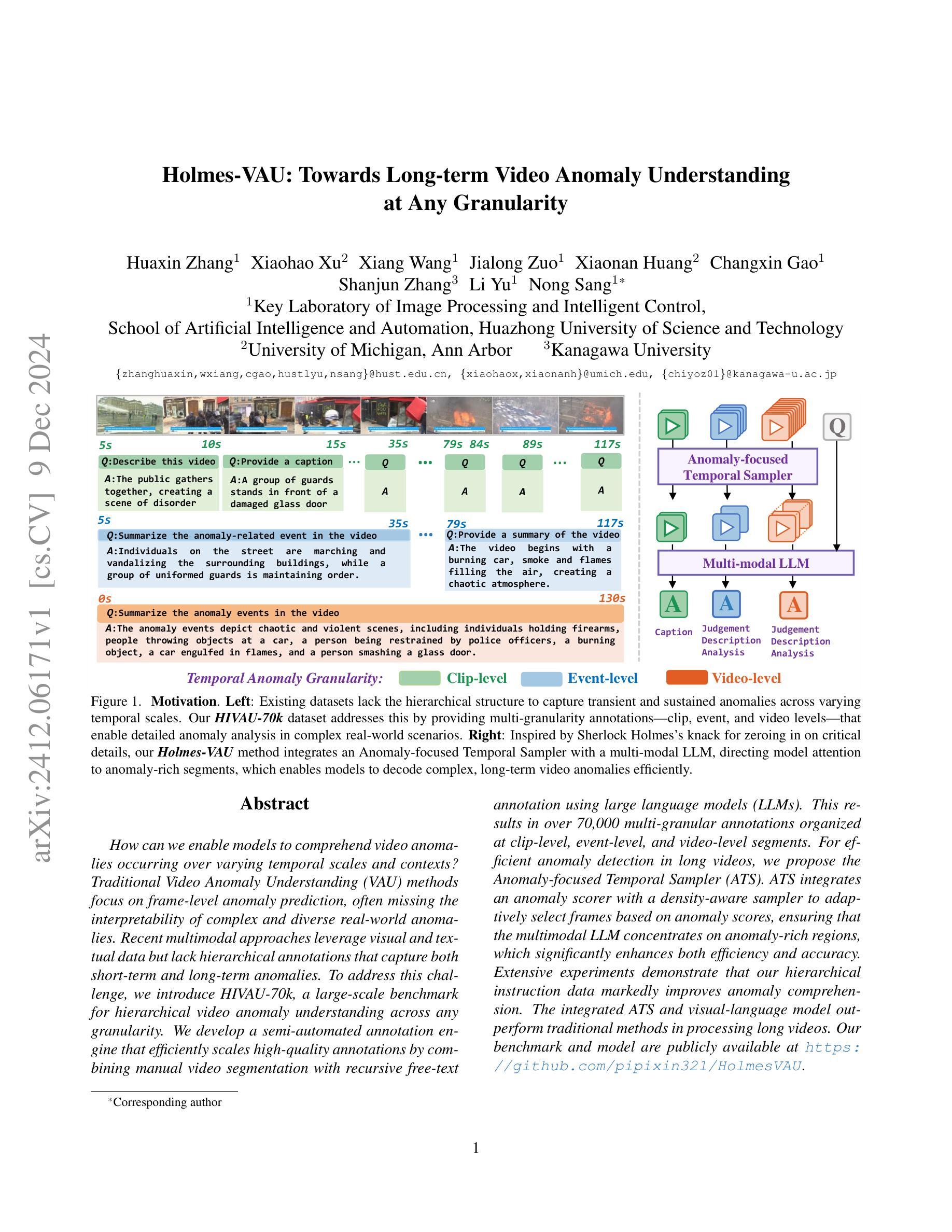
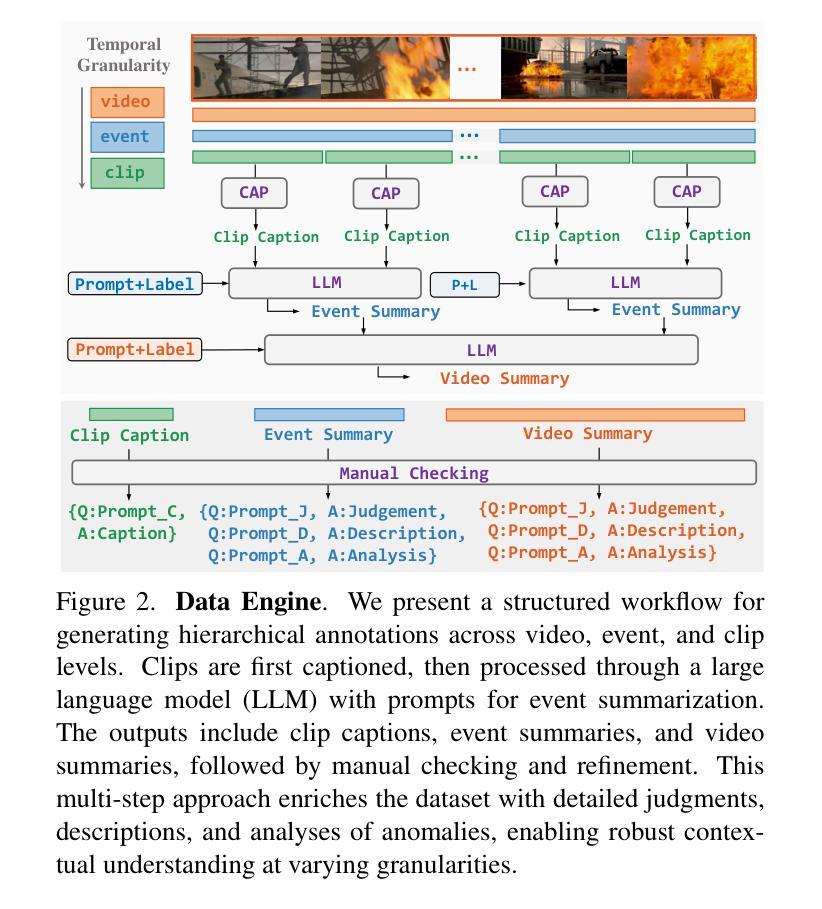
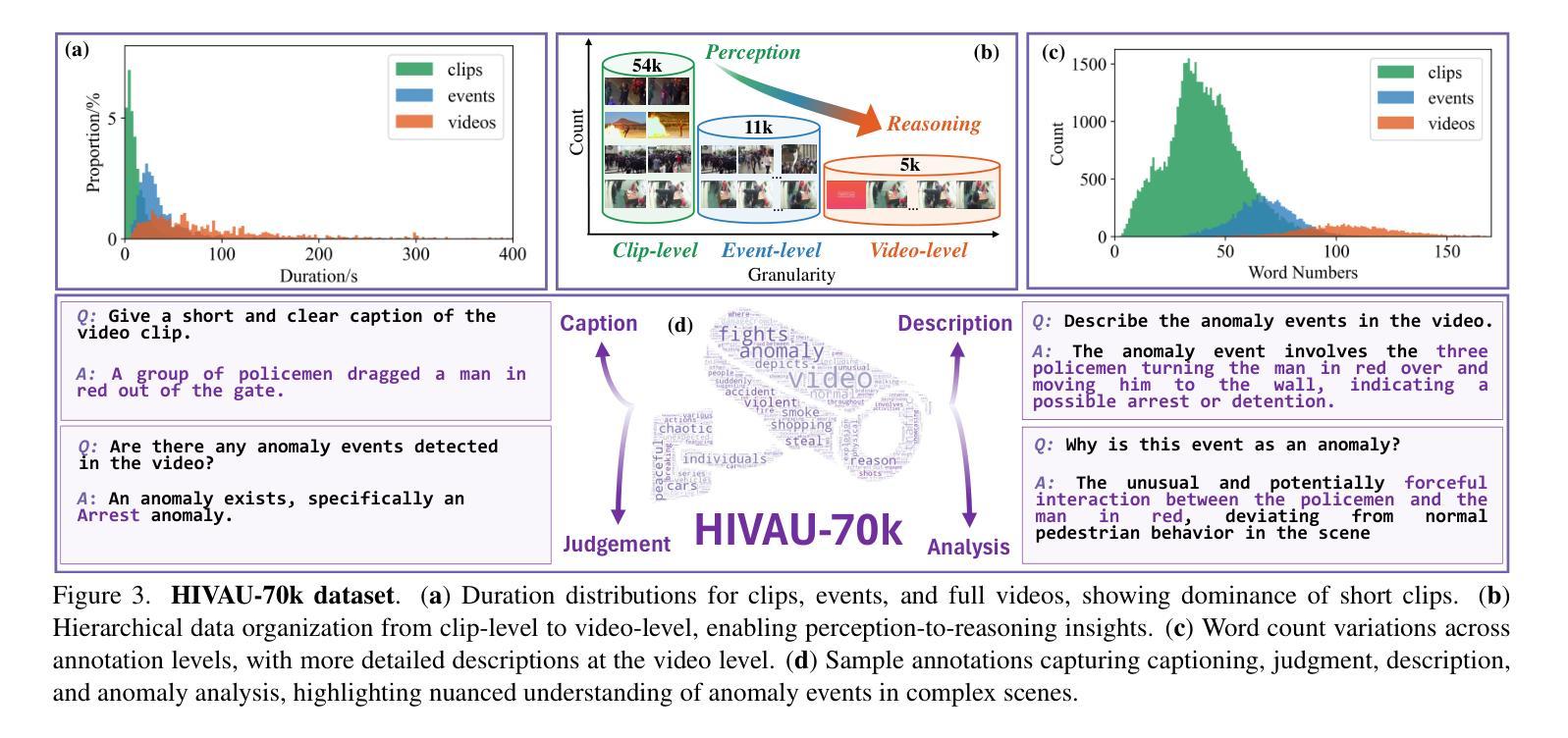
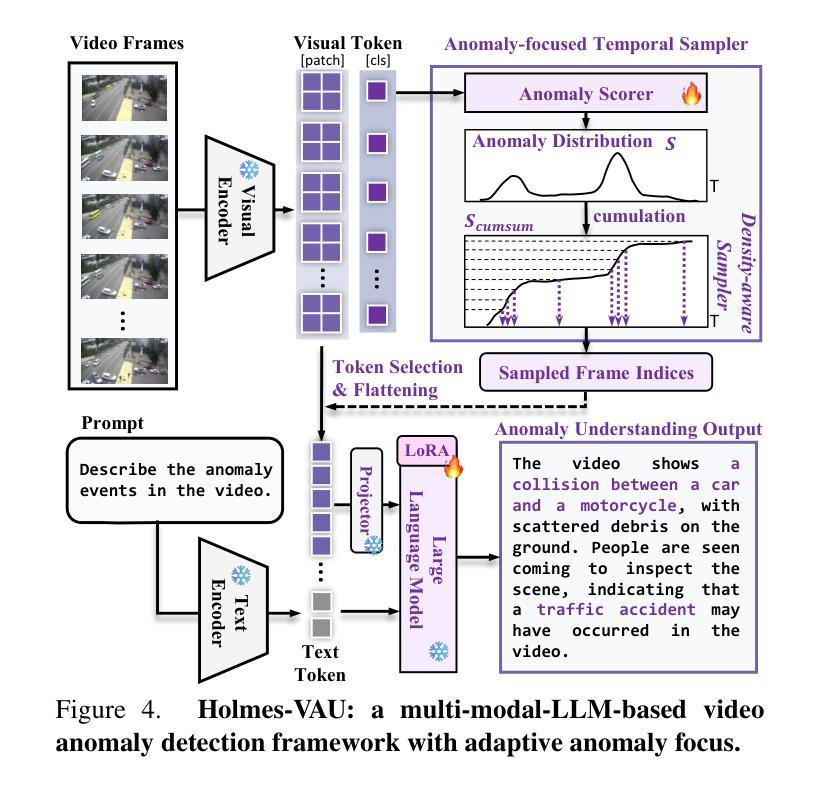
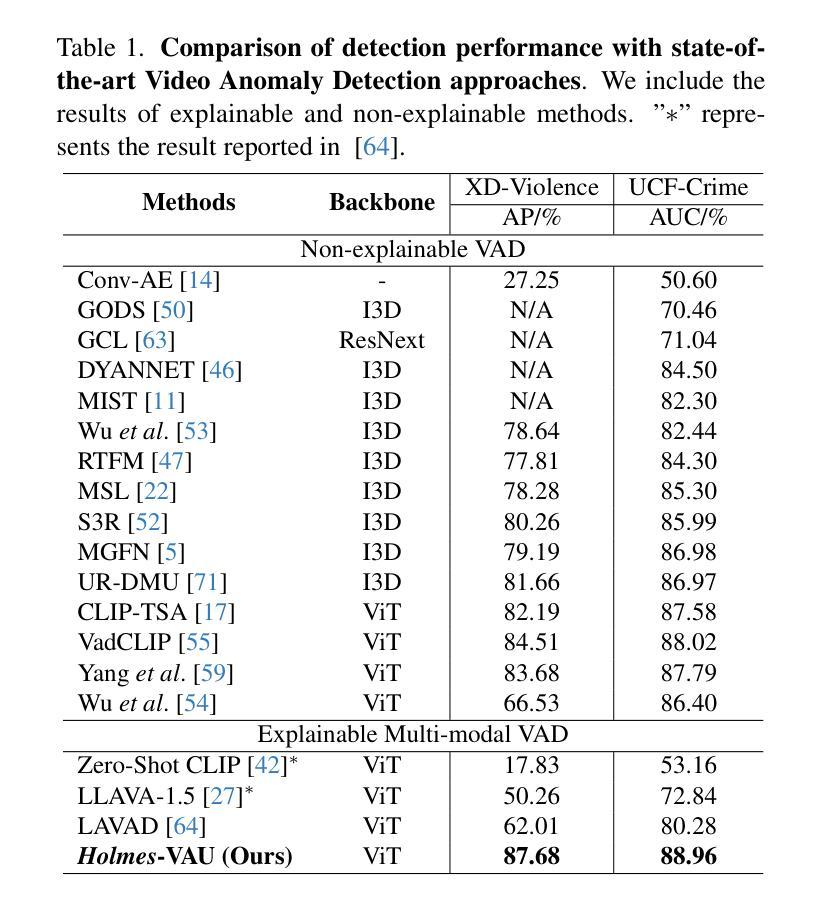
LinVT: Empower Your Image-level Large Language Model to Understand Videos
Authors:Lishuai Gao, Yujie Zhong, Yingsen Zeng, Haoxian Tan, Dengjie Li, Zheng Zhao
Large Language Models (LLMs) have been widely used in various tasks, motivating us to develop an LLM-based assistant for videos. Instead of training from scratch, we propose a module to transform arbitrary well-trained image-based LLMs into video-LLMs (after being trained on video data). To better adapt image-LLMs for processing videos, we introduce two design principles: linear transformation to preserve the original visual-language alignment and representative information condensation from redundant video content. Guided by these principles, we propose a plug-and-play Linear Video Tokenizer(LinVT), which enables existing image-LLMs to understand videos. We benchmark LinVT with six recent visual LLMs: Aquila, Blip-3, InternVL2, Mipha, Molmo and Qwen2-VL, showcasing the high compatibility of LinVT. LinVT-based LLMs achieve state-of-the-art performance across various video benchmarks, illustrating the effectiveness of LinVT in multi-modal video understanding.
大规模语言模型(LLMs)已在各种任务中得到了广泛应用,这促使我们开发基于LLM的视频助理。我们不是从头开始训练,而是提出一个模块,将任意的、基于图像的良好训练的LLMs转化为在视频数据训练后的视频LLMs。为了更好地适应图像LLMs处理视频,我们引入了两个设计原则:线性变换以保留原始视觉语言对齐和从冗余视频内容中凝练代表性信息。在这些原则的指导下,我们提出了即插即用的线性视频令牌化器(LinVT),使现有的图像LLMs能够理解视频。我们使用LinVT对六种最新的视觉LLMs进行了基准测试:Aquila、Blip-3、InternVL2、Mipha、Molmo和Qwen2-VL,展示了LinVT的高兼容性。基于LinVT的LLMs在各项视频基准测试中达到了最先进的性能,证明了LinVT在多模态视频理解中的有效性。
论文及项目相关链接
Summary
基于大型语言模型(LLM)在多种任务中的广泛应用,本文提出了一种基于LLM的视频助理。不同于从头开始训练,本文提出了一种模块,可将任意已训练好的图像LLM转化为视频LLM(经过视频数据训练后)。为了更好地适应视频处理的图像LLM,本文引入了线性变换以保留原始视觉语言对齐和代表性信息冷凝的设计原则。基于此原则,我们提出了即插即用的线性视频令牌器(LinVT),使现有图像LLM能够理解视频。我们对LinVT进行了基准测试,与最近的六种视觉LLM进行比对:Aquila、Blip-3、InternVL2、Mipha、Molmo和Qwen2-VL,证明了LinVT的高度兼容性。基于LinVT的LLM在各种视频基准测试中达到了业界最佳性能,证明了LinVT在多模态视频理解中的有效性。
Key Takeaways
- LLMs广泛用于各种任务,激励我们开发基于LLM的视频助理。
- 提出了一种模块化的方法,将图像LLM转化为视频LLM,通过视频数据训练。
- 引入线性变换和代表性信息冷凝的设计原则,使图像LLM更好地适应视频处理。
- 提出了即插即用的Linear Video Tokenizer(LinVT),使现有图像LLM能理解视频。
- LinVT与六种视觉LLM基准测试表现出高兼容性。
- 基于LinVT的LLM在多种视频基准测试中达到业界最佳性能。
点此查看论文截图
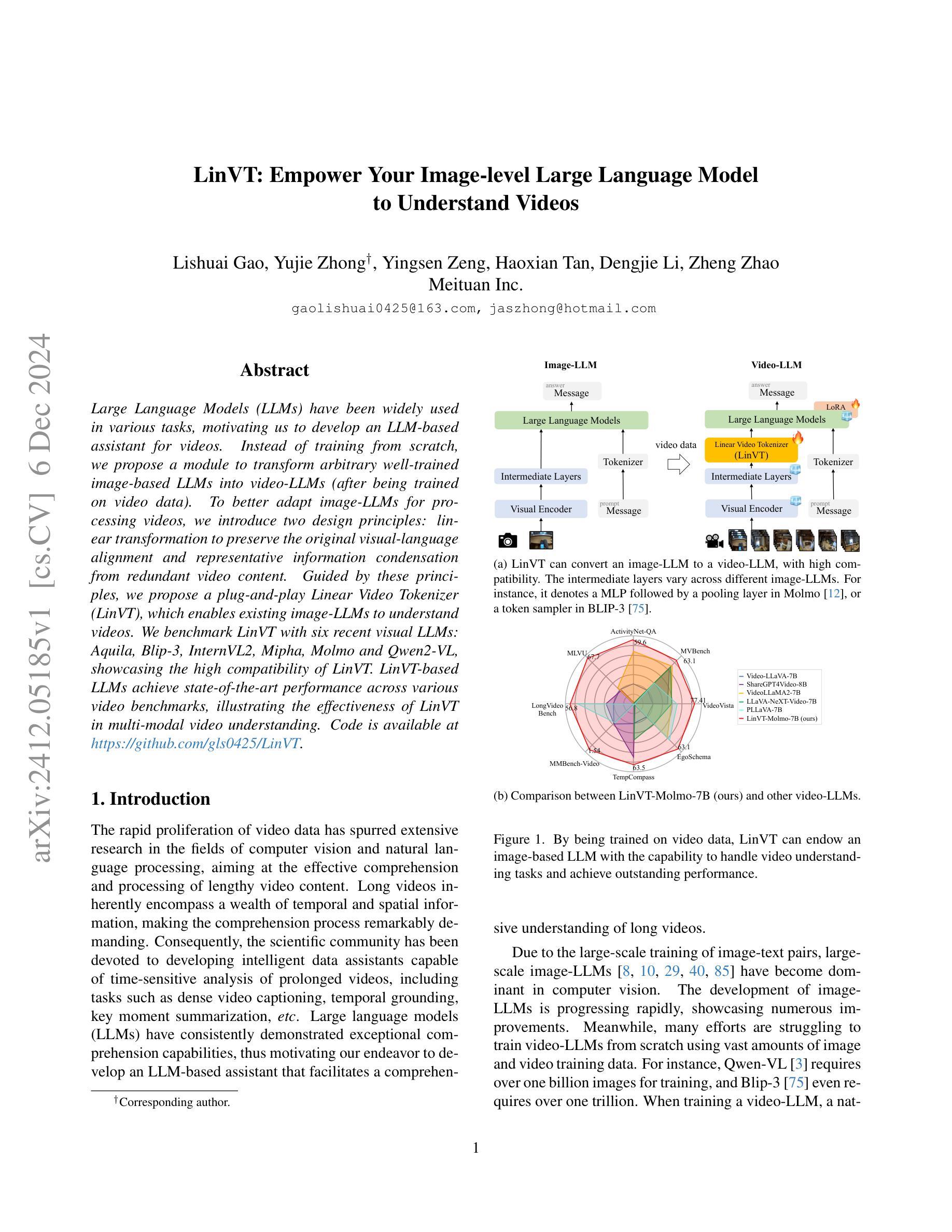
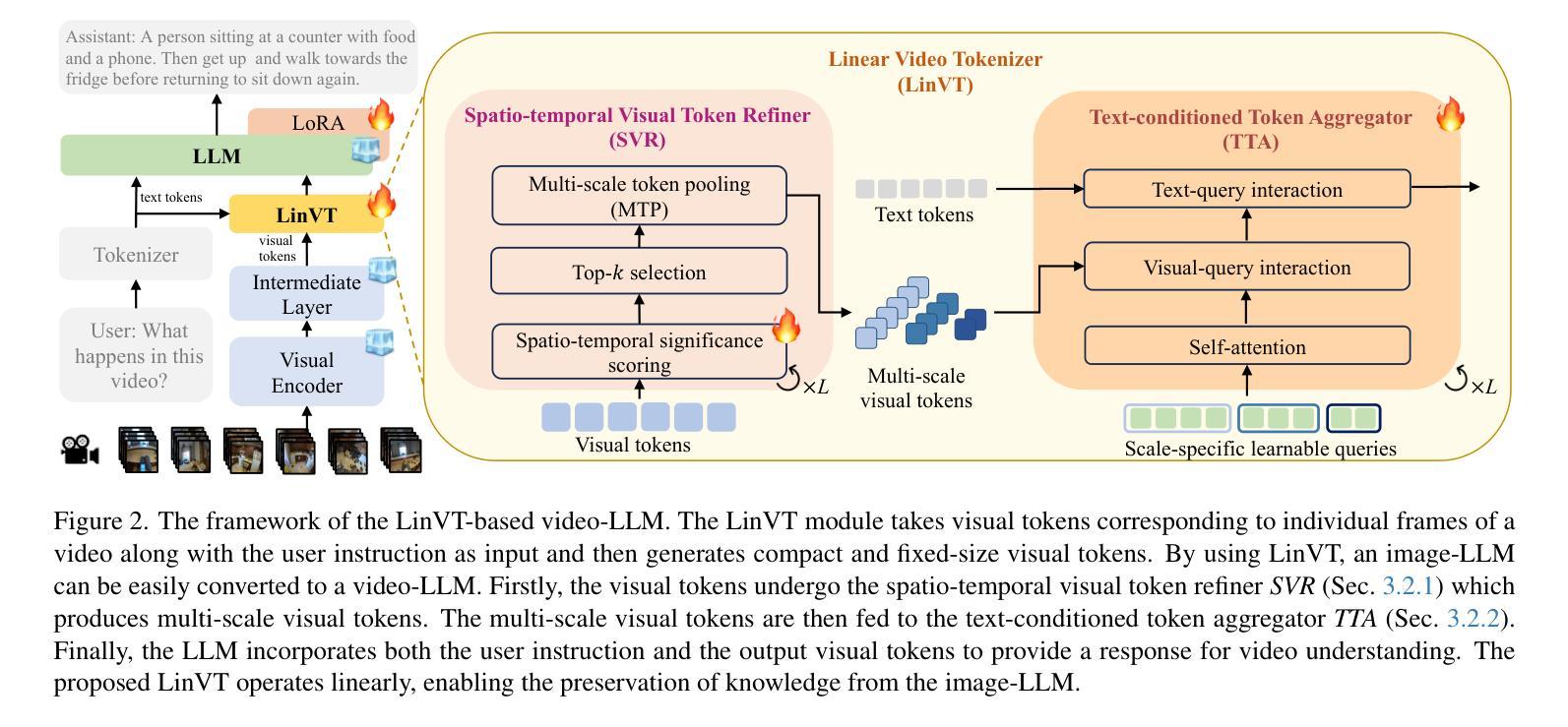
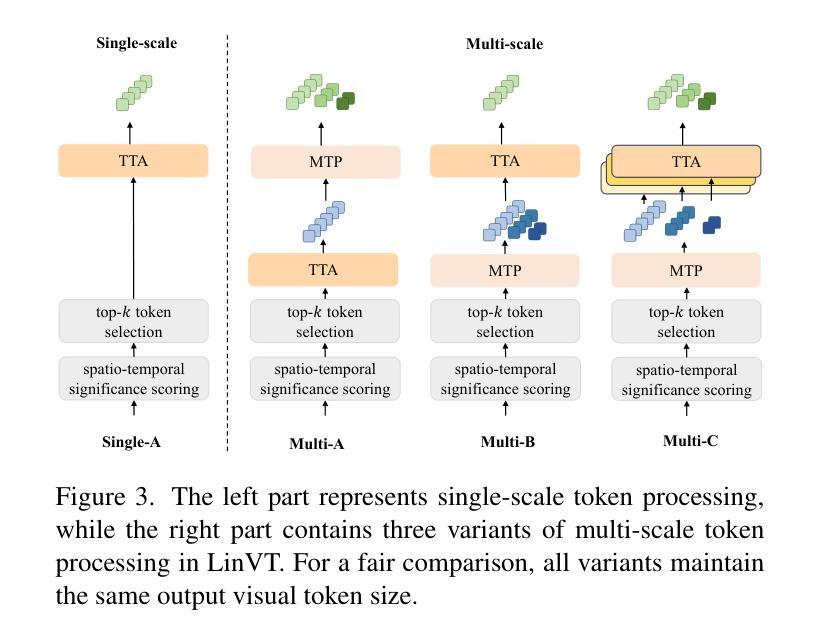
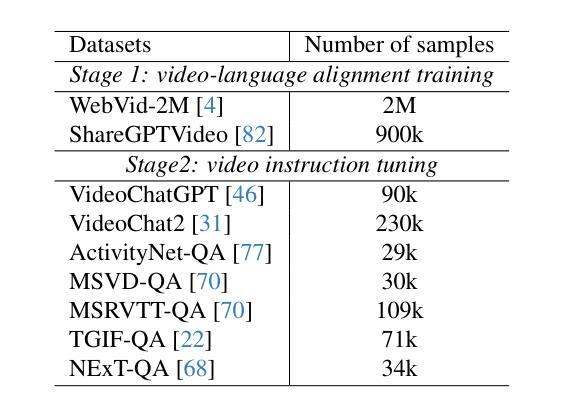
VidHalluc: Evaluating Temporal Hallucinations in Multimodal Large Language Models for Video Understanding
Authors:Chaoyu Li, Eun Woo Im, Pooyan Fazli
Multimodal large language models (MLLMs) have recently shown significant advancements in video understanding, excelling in content reasoning and instruction-following tasks. However, the problem of hallucination, where models generate inaccurate or misleading content, remains underexplored in the video domain. Building on the observation that the visual encoder of MLLMs often struggles to differentiate between video pairs that are visually distinct but semantically similar, we introduce VidHalluc, the largest benchmark designed to examine hallucinations in MLLMs for video understanding tasks. VidHalluc assesses hallucinations across three critical dimensions: (1) action, (2) temporal sequence, and (3) scene transition. VidHalluc consists of 5,002 videos, paired based on semantic similarity and visual differences, focusing on cases where hallucinations are most likely to occur. Through comprehensive testing, our experiments show that most MLLMs are vulnerable to hallucinations across these dimensions. Furthermore, we propose DINO-HEAL, a training-free method that reduces hallucinations by incorporating spatial saliency information from DINOv2 to reweight visual features during inference. Our results demonstrate that DINO-HEAL consistently improves performance on VidHalluc, achieving an average improvement of 3.02% in mitigating hallucinations among all tasks. Both the VidHalluc benchmark and DINO-HEAL code can be accessed via $\href{https://vid-halluc.github.io/}{\text{this link}}$.
多模态大型语言模型(MLLMs)在视频理解方面最近取得了重大进展,尤其是在内容推理和指令遵循任务方面表现出色。然而,模型生成不准确或误导性内容的问题——即幻觉问题,在视频领域仍被探索得不够深入。我们观察到MLLM的视觉编码器在区分视觉上不同但语义上相似的视频对时经常遇到困难,于是我们引入了VidHalluc,这是为视频理解任务中MLLM的幻觉问题设计的最大基准测试。VidHalluc评估幻觉的三个关键维度:(1)动作、(2)时间序列、(3)场景过渡。VidHalluc包含5002个视频,这些视频基于语义相似性和视觉差异进行配对,重点关注幻觉最可能发生的情况。通过全面的测试,我们的实验表明,大多数MLLM在这些维度上都容易受到幻觉的影响。此外,我们提出了DINO-HEAL,这是一种无需训练的方法,通过结合DINOv2的空间显著性信息来在推理过程中重新加权视觉特征,从而减少幻觉。我们的结果表明,DINO-HEAL在VidHalluc上持续提高了性能,在所有任务中减轻幻觉的平均改进率为3.02%。可以通过此链接访问VidHalluc基准测试和DINO-HEAL代码。
论文及项目相关链接
Summary
本文介绍了多模态大型语言模型(MLLMs)在视频理解领域的最新进展,特别是在内容推理和指令遵循任务中的优异表现。然而,模型生成不准确或误导性内容的问题——即幻视现象,在视频领域仍被忽视。针对MLLMs的视觉编码器难以区分视觉上有差异但语义上相似的视频对的问题,本文引入了VidHalluc基准测试,它是专为视频理解任务中检查MLLMs的幻视现象而设计的大型基准测试。VidHalluc从动作、时间顺序和场景过渡三个关键维度评估幻视现象。它包含5002个视频,基于语义相似性和视觉差异进行配对,重点关注最可能发生幻视的情况。实验表明,大多数MLLMs在这些维度上都容易受到幻视的影响。此外,本文提出了DINO-HEAL方法,这是一种无需训练即可减少幻视的方法,它通过结合DINOv2的空间显著性信息在推理时重新加权视觉特征。结果显示,DINO-HEAL在VidHalluc上表现一致,在所有任务中平均提高了3.02%的幻视减轻效果。
Key Takeaways
- 多模态大型语言模型(MLLMs)在视频理解领域有显著的进展,尤其在内容推理和指令遵循任务方面表现优秀。
- 幻视现象在视频领域的MLLMs中仍然是一个被忽视的问题,这指的是模型生成不准确或误导性的内容。
- VidHalluc基准测试是为了检查视频理解任务中MLLMs的幻视现象而设计的大型基准测试。它包含5002个视频,专注于评估动作、时间顺序和场景过渡三个关键维度的幻视情况。
- 大多数MLLMs在视觉上有差异但语义上相似的视频对上的表现存在问题,容易受到幻视的影响。
- DINO-HEAL是一种无需训练的方法,通过结合空间显著性信息来减少幻视现象,这在推理时能够改善视频理解的效果。
- DINO-HEAL在VidHalluc基准测试上的表现一致,并且在所有任务中平均提高了幻视减轻的效果达到3.02%。
点此查看论文截图
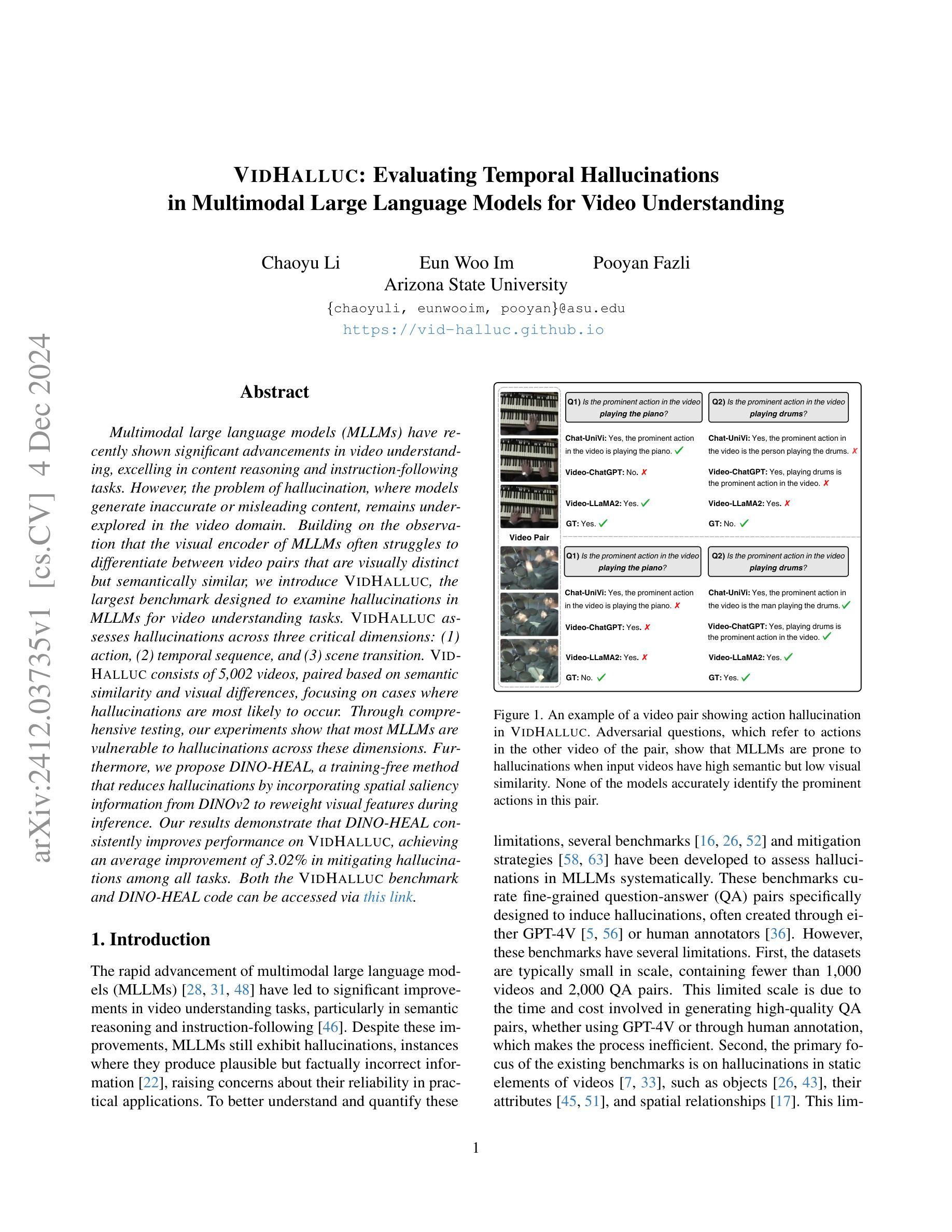
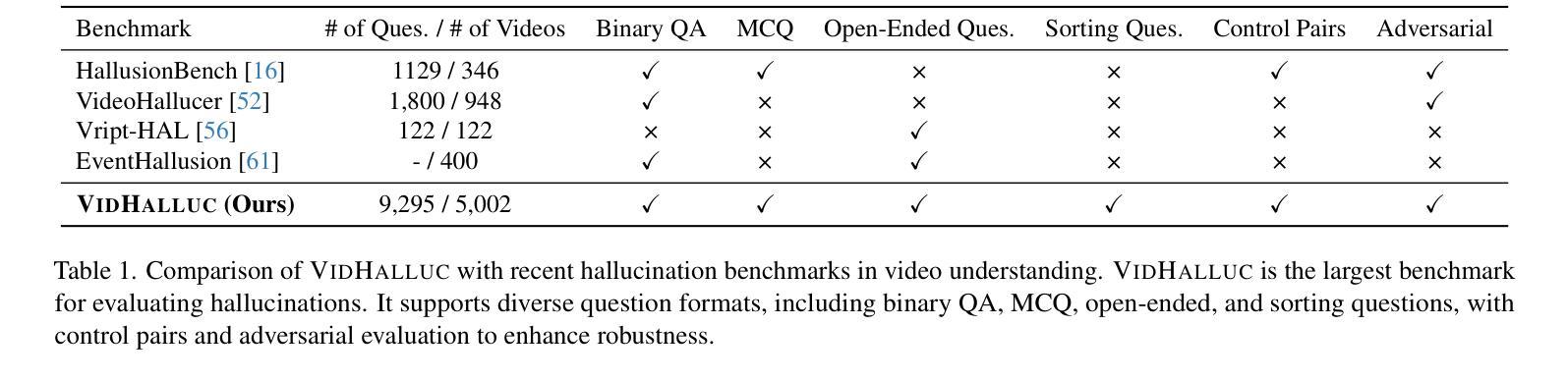
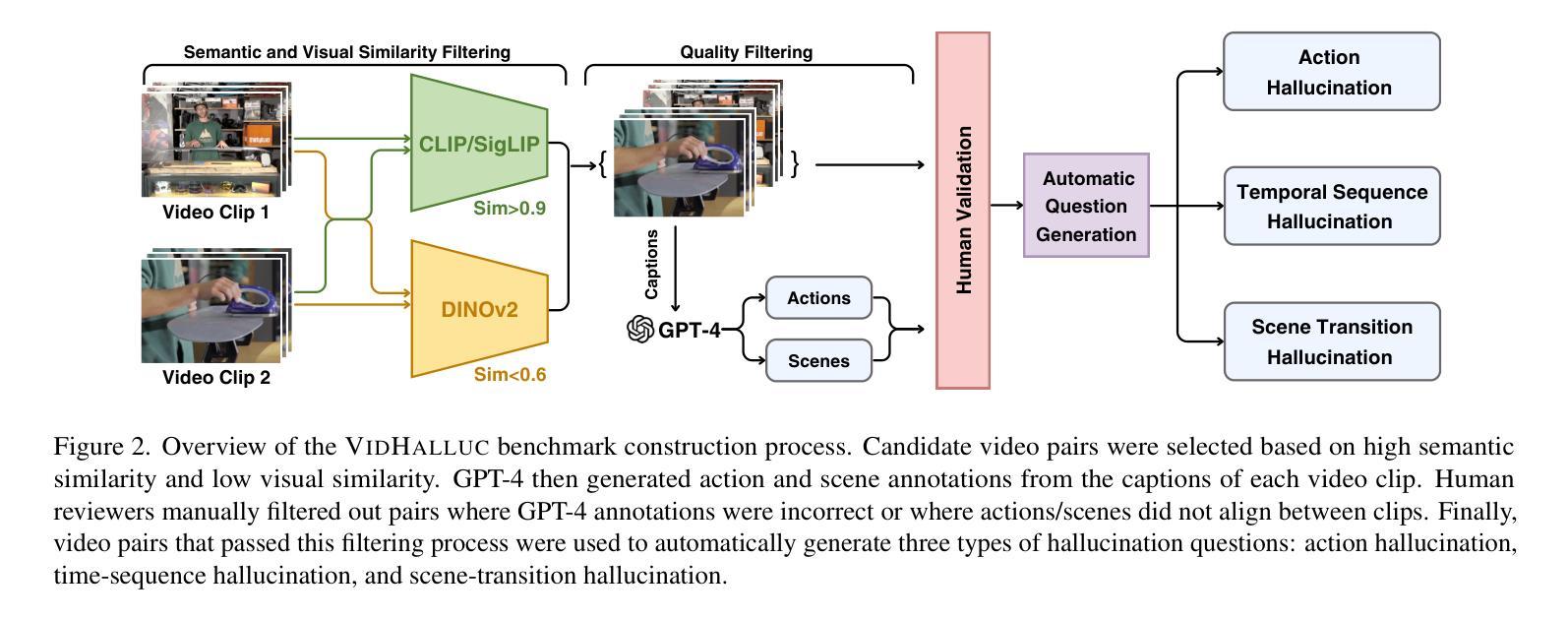
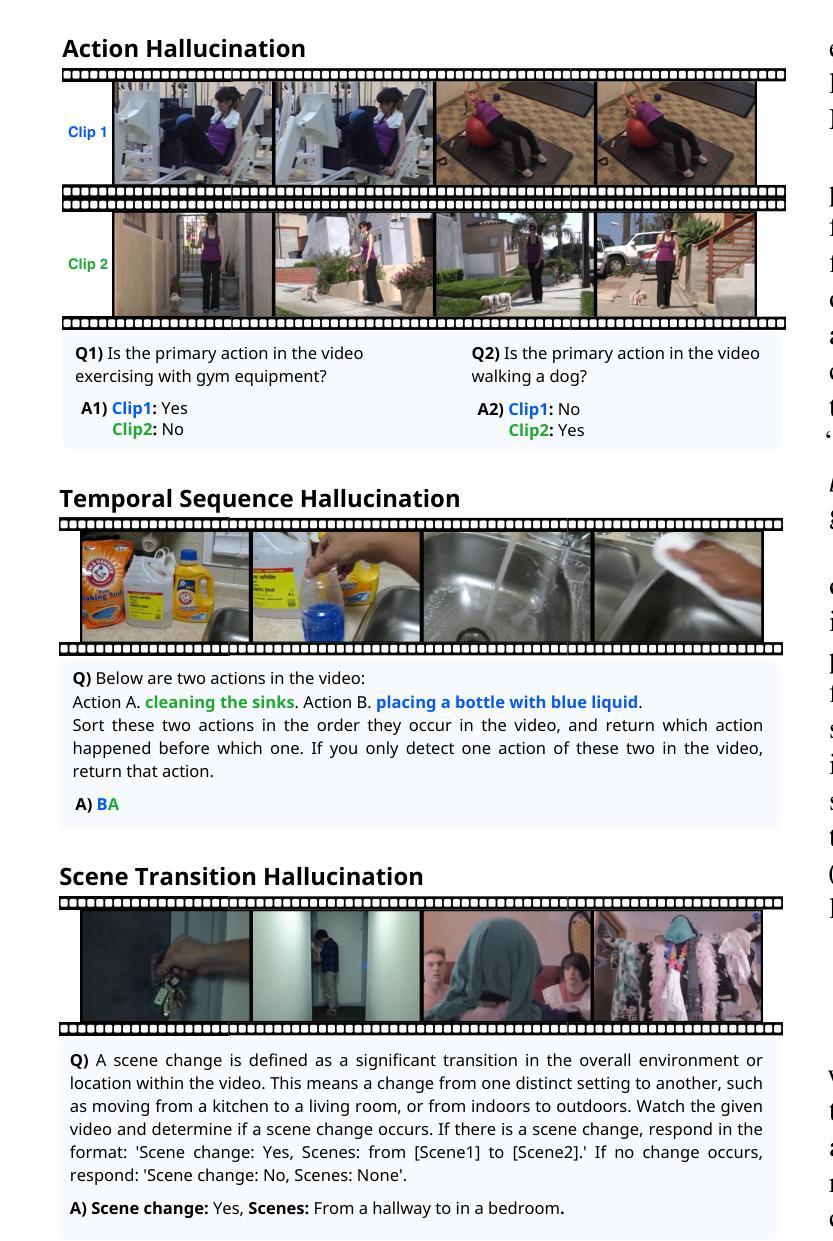
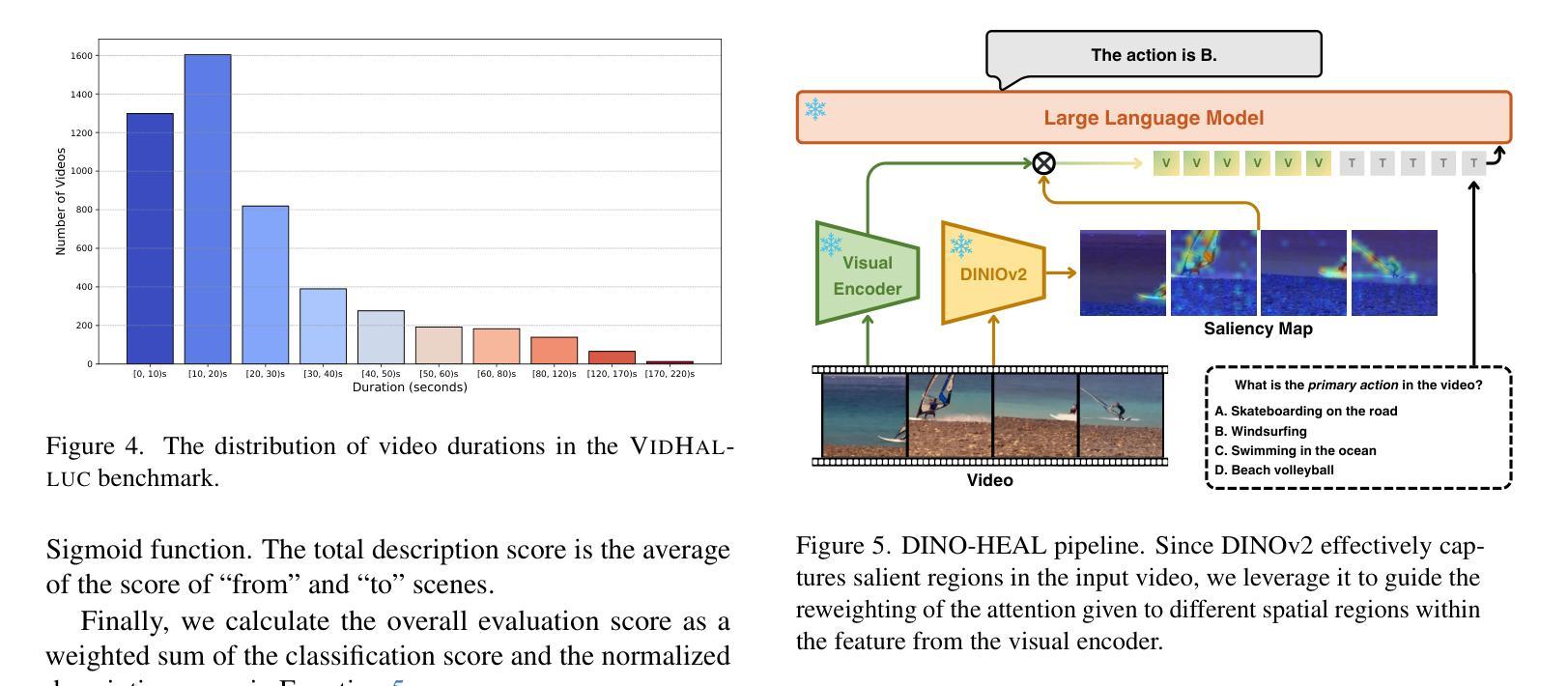
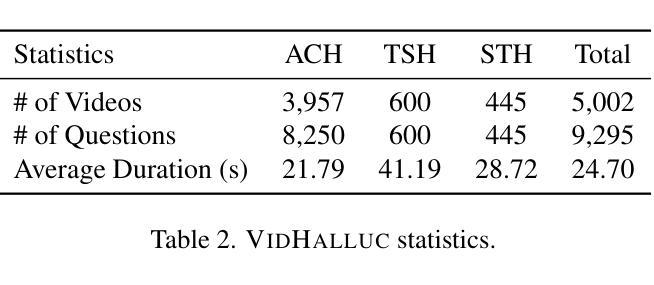
Mimir: Improving Video Diffusion Models for Precise Text Understanding
Authors:Shuai Tan, Biao Gong, Yutong Feng, Kecheng Zheng, Dandan Zheng, Shuwei Shi, Yujun Shen, Jingdong Chen, Ming Yang
Text serves as the key control signal in video generation due to its narrative nature. To render text descriptions into video clips, current video diffusion models borrow features from text encoders yet struggle with limited text comprehension. The recent success of large language models (LLMs) showcases the power of decoder-only transformers, which offers three clear benefits for text-to-video (T2V) generation, namely, precise text understanding resulting from the superior scalability, imagination beyond the input text enabled by next token prediction, and flexibility to prioritize user interests through instruction tuning. Nevertheless, the feature distribution gap emerging from the two different text modeling paradigms hinders the direct use of LLMs in established T2V models. This work addresses this challenge with Mimir, an end-to-end training framework featuring a carefully tailored token fuser to harmonize the outputs from text encoders and LLMs. Such a design allows the T2V model to fully leverage learned video priors while capitalizing on the text-related capability of LLMs. Extensive quantitative and qualitative results demonstrate the effectiveness of Mimir in generating high-quality videos with excellent text comprehension, especially when processing short captions and managing shifting motions. Project page: https://lucaria-academy.github.io/Mimir/
文本在视频生成中扮演着关键控制信号的角色,这是由于它的叙事性质。为了将文本描述转化为视频片段,当前的视频扩散模型借鉴了文本编码器的特征,但在文本理解方面存在局限。最近大型语言模型(LLMs)的成功展示了只含解码器的变压器的力量,它为文本到视频(T2V)生成提供了三大明确优势,分别是:由于出色的可扩展性而实现的精确文本理解、通过下一个令牌预测实现的超越输入文本的想象力,以及通过指令调整来优先考虑用户兴趣的灵活性。然而,两种不同文本建模范式产生的特征分布差距阻碍了LLMs在现有T2V模型中的直接使用。这项工作通过Mimir解决了这一挑战,Mimir是一个端到端的训练框架,具有精心定制的令牌融合器,以协调文本编码器和LLMs的输出。这样的设计允许T2V模型充分利用学习的视频先验知识,同时利用LLMs的文本相关功能。大量的定量和定性结果表明,Mimir在生成高质量视频方面非常有效,具有良好的文本理解能力,尤其是在处理短标题和管理动态场景转换时。项目页面:[https://lucaria-academy.github.io/Mimir/]
论文及项目相关链接
Summary
文本在视频生成中扮演着关键的控制信号角色,得益于其叙事性质。当前视频扩散模型借鉴文本编码器的特性,但在文本理解方面存在局限。大型语言模型(LLMs)的近期成功展示了仅解码器转换器模型的威力,为文本到视频(T2V)生成提供了三大优势:源于卓越可扩展性的精确文本理解、由下一个令牌预测激发的超越输入文本的想象力以及通过指令调整优先用户兴趣的灵活性。然而,两种文本建模范式产生的特征分布差距阻碍了LLMs在现有T2V模型中的直接使用。本研究通过Mimir解决这一挑战,这是一个端到端的训练框架,配备精心定制的分隔器来调和文本编码器和LLMs的输出。这种设计允许T2V模型充分利用学习到的视频先验知识,同时利用LLMs的文本相关功能。大量定量和定性结果表明,Mimir在生成高质量视频方面非常有效,特别是在处理短字幕和管理动态场景方面表现出色。
Key Takeaways
- 文本在视频生成中起关键作用,得益于其叙事性质。
- 当前视频扩散模型在文本理解方面存在局限。
- 大型语言模型(LLMs)为文本到视频(T2V)生成提供了三大优势。
- 文本建模的两种范式之间存在特征分布差距。
- Mimir是一个端到端的训练框架,可以调和文本编码器和LLMs的输出。
- Mimir允许T2V模型利用视频先验知识和LLMs的文本相关功能。
点此查看论文截图
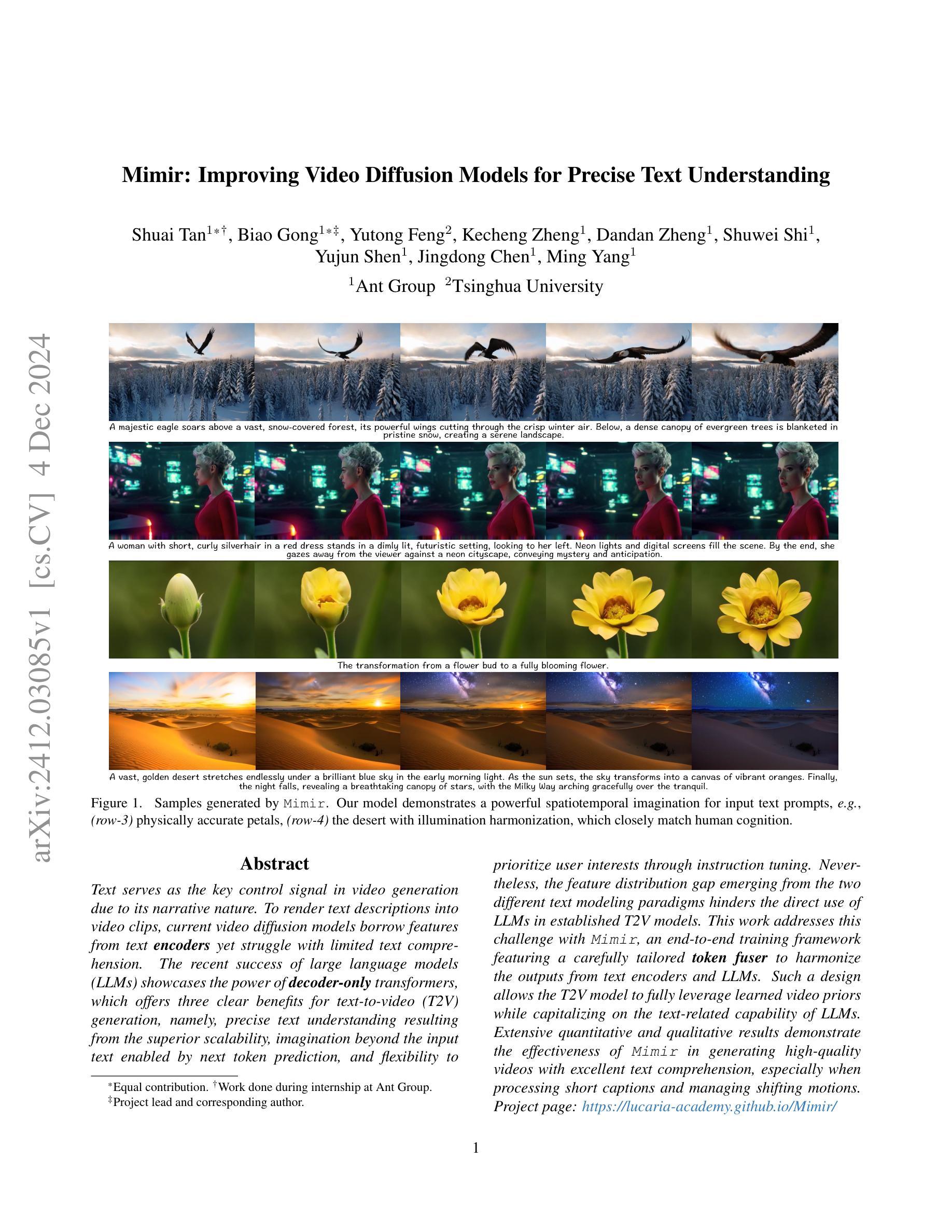
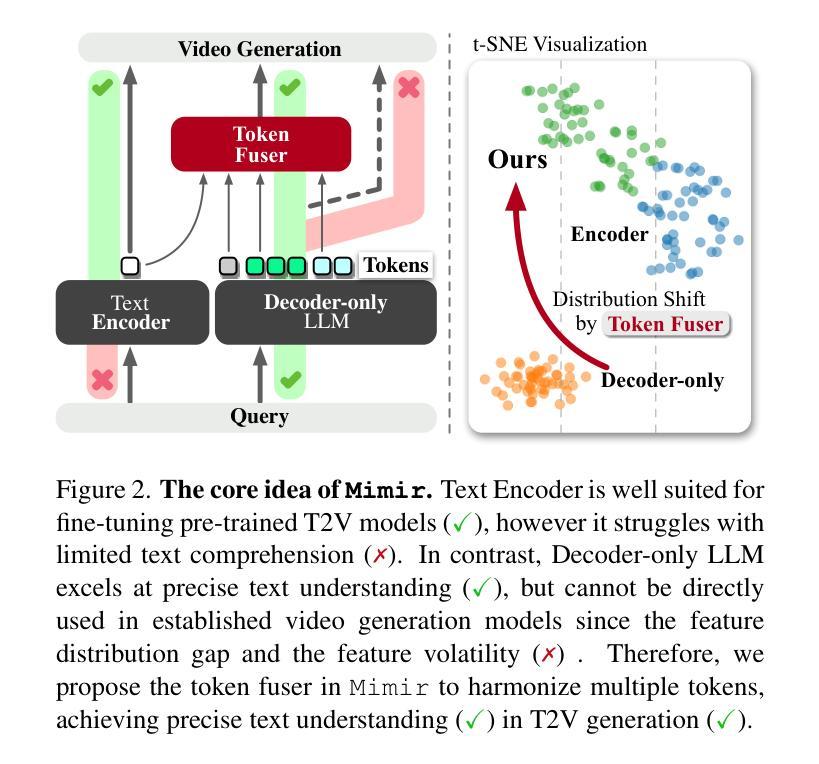
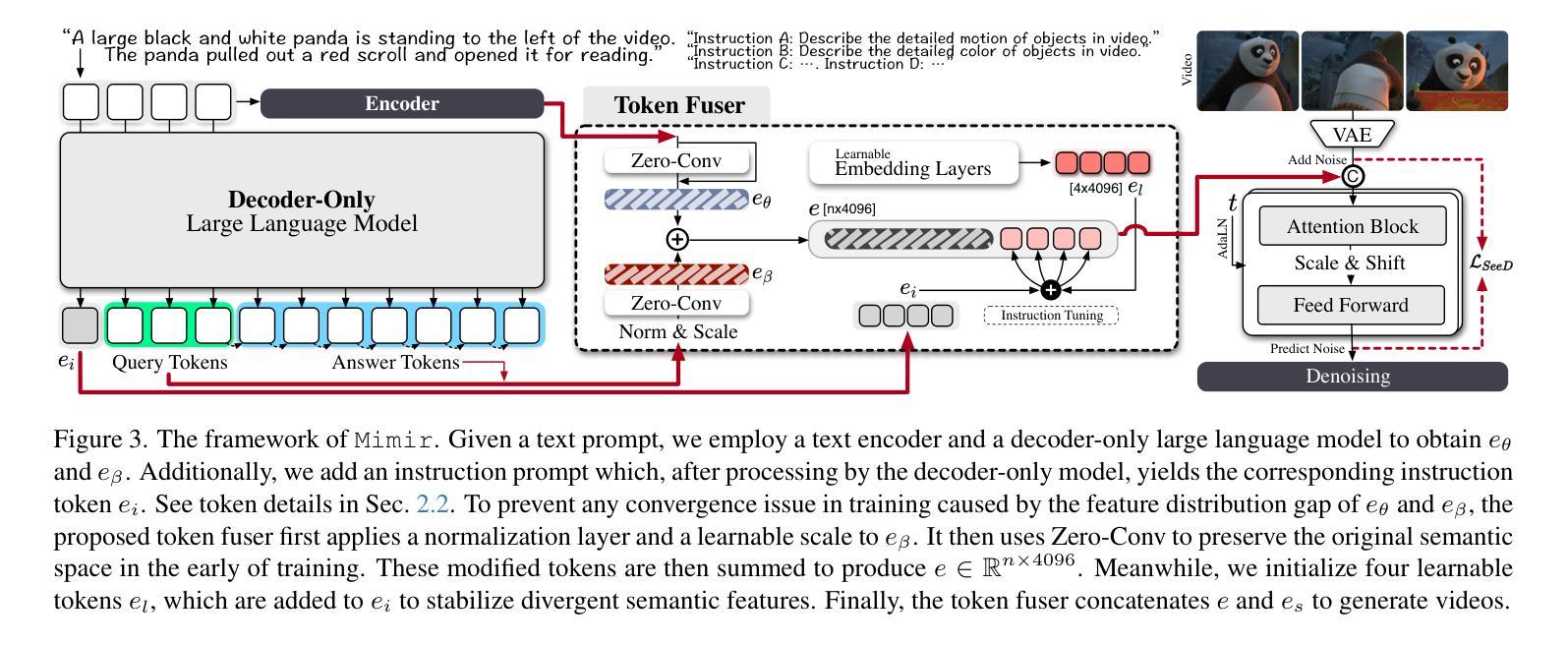
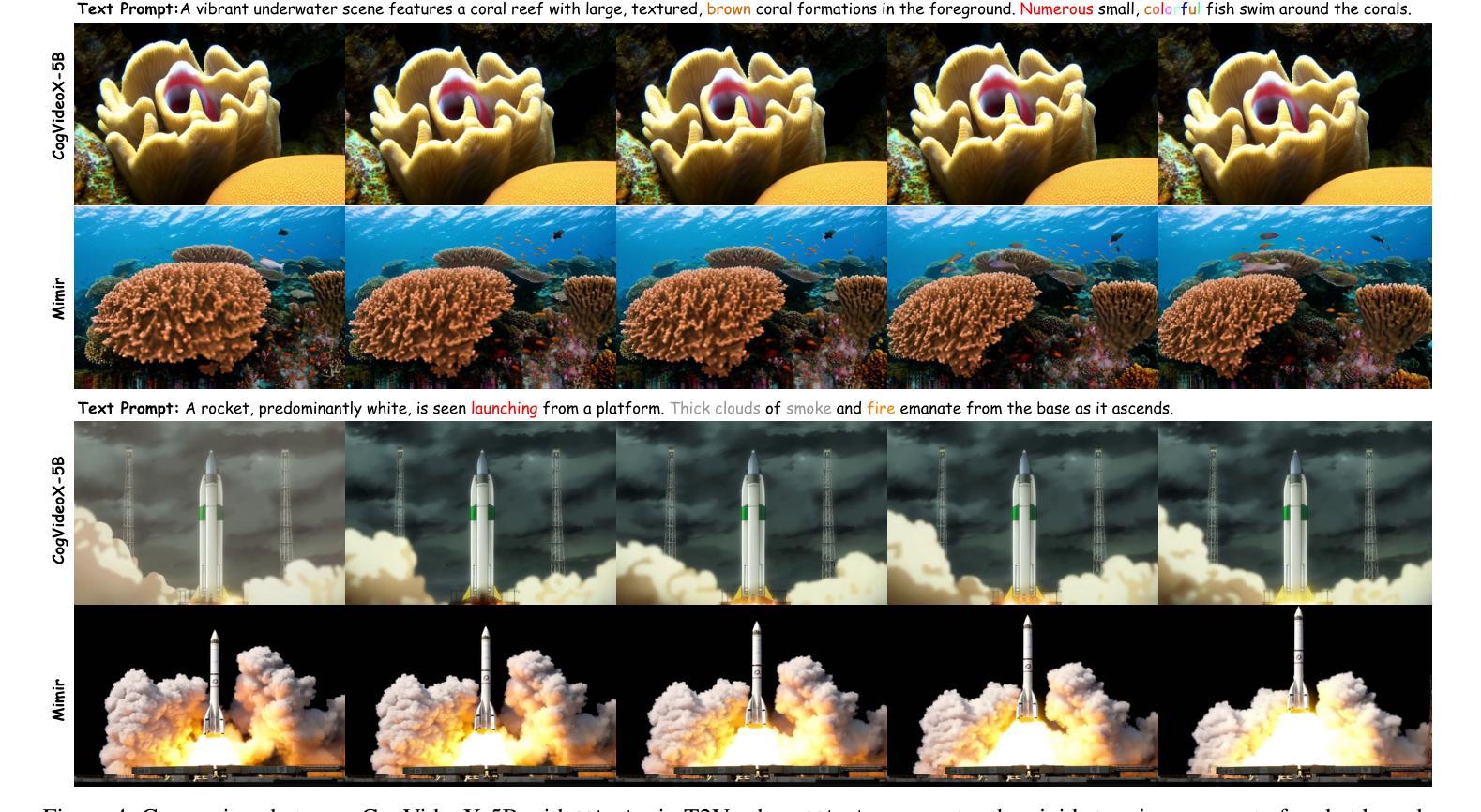
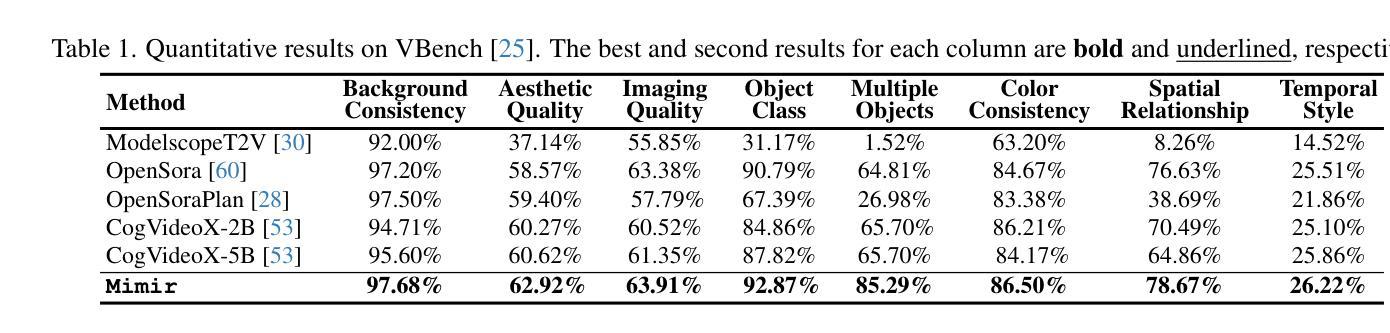
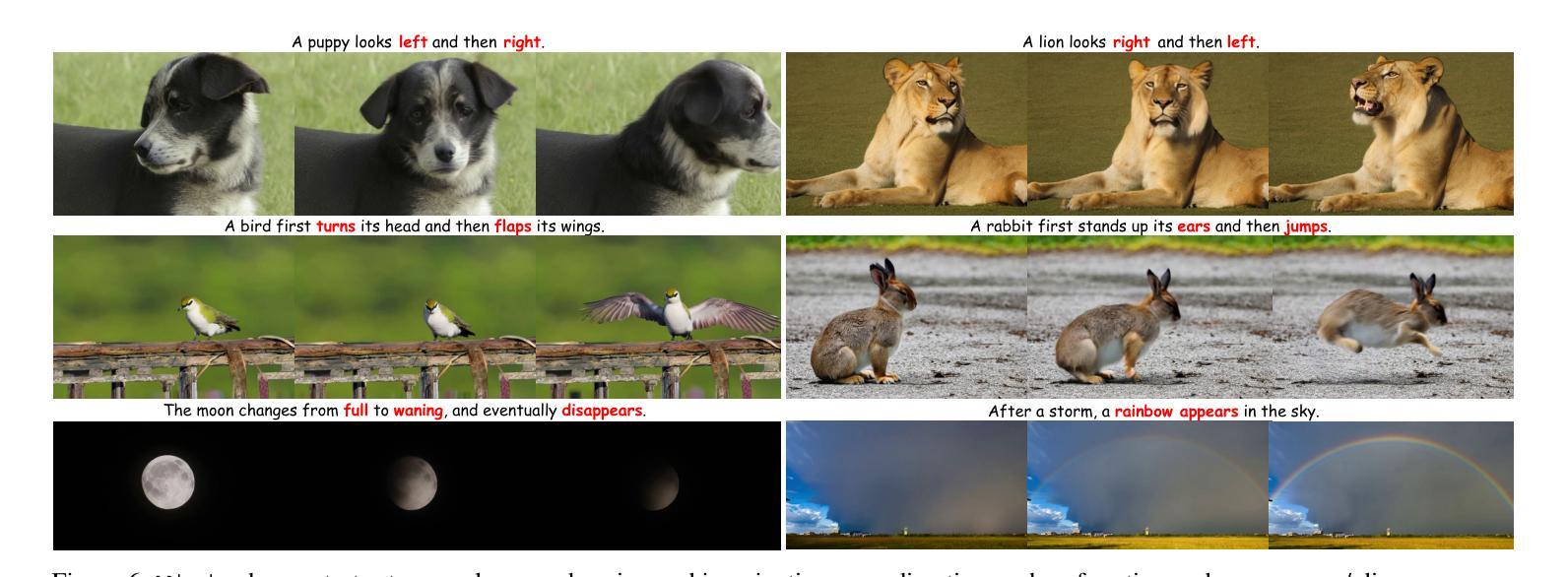
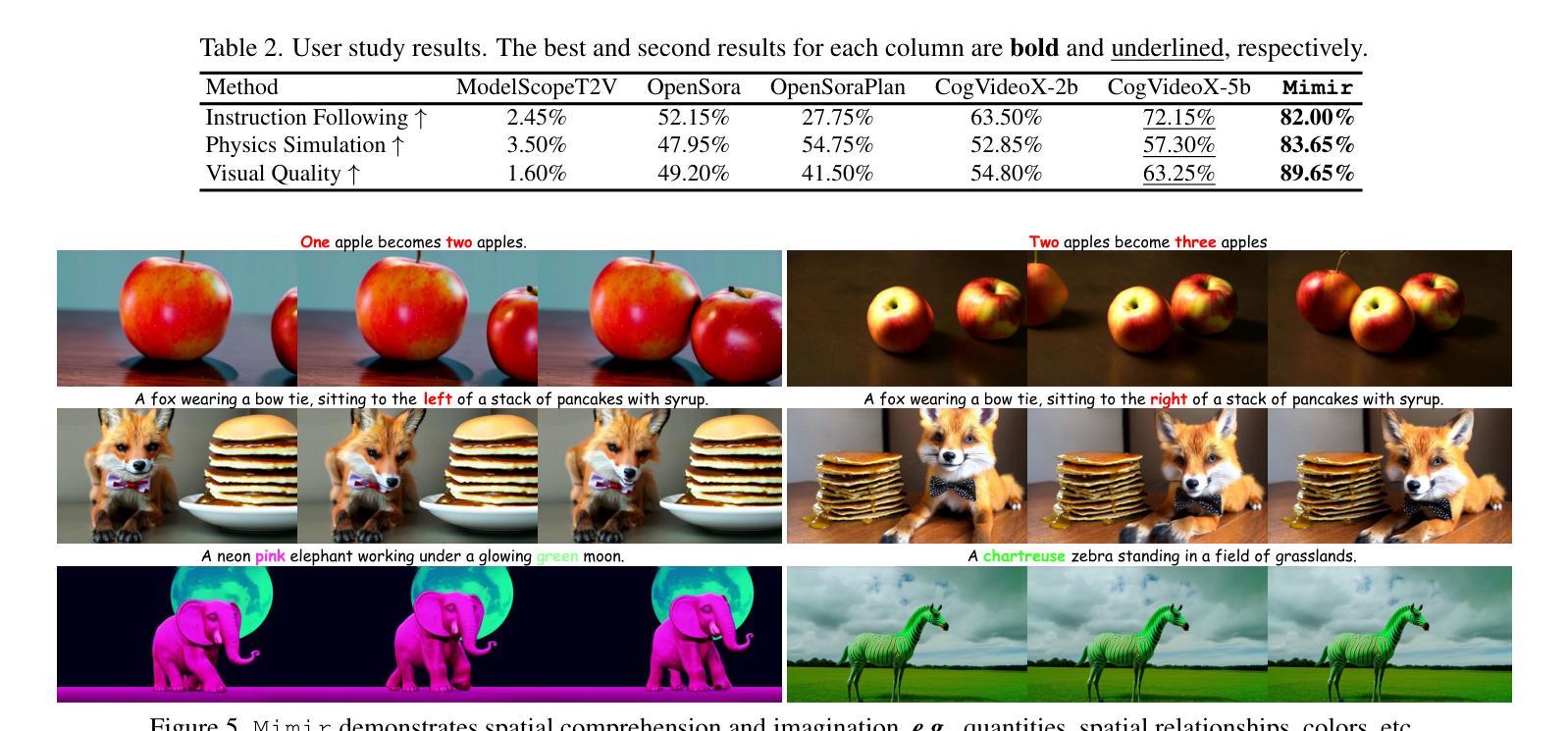
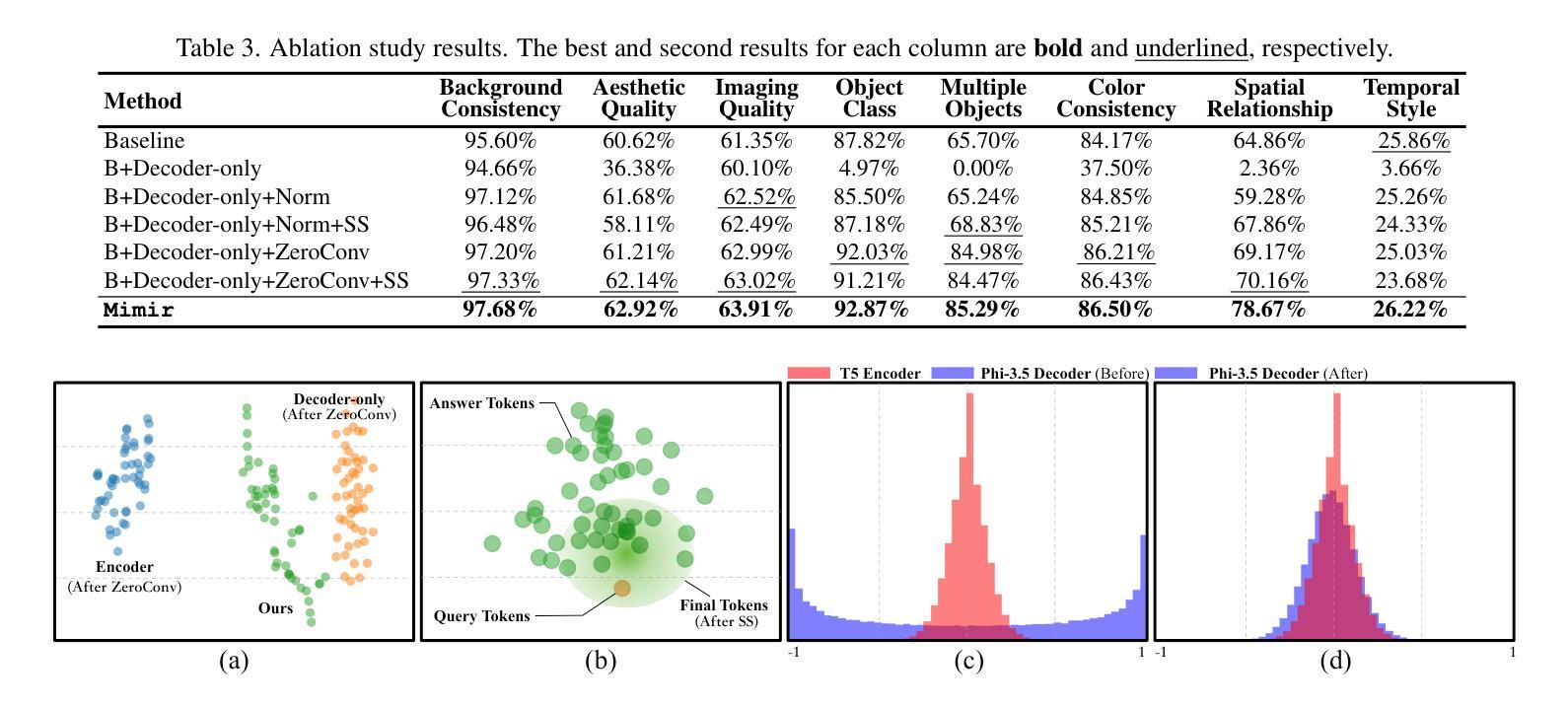
VideoICL: Confidence-based Iterative In-context Learning for Out-of-Distribution Video Understanding
Authors:Kangsan Kim, Geon Park, Youngwan Lee, Woongyeong Yeo, Sung Ju Hwang
Recent advancements in video large multimodal models (LMMs) have significantly improved their video understanding and reasoning capabilities. However, their performance drops on out-of-distribution (OOD) tasks that are underrepresented in training data. Traditional methods like fine-tuning on OOD datasets are impractical due to high computational costs. While In-context learning (ICL) with demonstration examples has shown promising generalization performance in language tasks and image-language tasks without fine-tuning, applying ICL to video-language tasks faces challenges due to the limited context length in Video LMMs, as videos require longer token lengths. To address these issues, we propose VideoICL, a novel video in-context learning framework for OOD tasks that introduces a similarity-based relevant example selection strategy and a confidence-based iterative inference approach. This allows to select the most relevant examples and rank them based on similarity, to be used for inference. If the generated response has low confidence, our framework selects new examples and performs inference again, iteratively refining the results until a high-confidence response is obtained. This approach improves OOD video understanding performance by extending effective context length without incurring high costs. The experimental results on multiple benchmarks demonstrate significant performance gains, especially in domain-specific scenarios, laying the groundwork for broader video comprehension applications. Code will be released at https://github.com/KangsanKim07/VideoICL
近期视频大型多模态模型(LMMs)的进展显著提高了其视频理解和推理能力。然而,它们在训练数据代表性不足的跨分布(OOD)任务上的表现有所下降。传统方法如在跨分布数据集上进行微调并不实用,因为计算成本高昂。尽管无需微调的在语境中学习(ICL)方法通过示例展示在语言和图像语言任务中显示出有前景的泛化性能,但将其应用于视频语言任务时面临着挑战,因为视频LMM中的语境长度有限,而视频需要更长的令牌长度。为了解决这个问题,我们提出了VideoICL,这是一种新型的面向跨分布任务的视频语境学习框架。它引入了一种基于相似度的相关示例选择策略和一种基于信心的迭代推理方法。这允许选择最相关的示例并根据相似性对其进行排名,以供推理使用。如果生成的响应缺乏信心,我们的框架会选择新的示例并再次进行推理,通过迭代优化结果,直至获得高信心的响应。这种方法通过扩展有效的语境长度而无需产生高昂成本,提高了跨分布视频理解性能。在多个基准测试上的实验结果表明,尤其是在特定领域场景中,取得了显著的性能提升,为更广泛的视频理解应用奠定了基础。代码将发布在https://github.com/KangsanKim07/VideoICL。
论文及项目相关链接
Summary
近期视频大型多模态模型(LMMs)的进展显著提高了视频理解和推理能力。然而,它们在训练数据缺乏代表性(OOD)的任务上的性能会下降。传统方法如精细调整OOD数据集因高计算成本而不实用。尽管通过展示范例进行上下文内学习(ICL)已在语言任务和图像语言任务中展现出令人鼓舞的泛化性能,但将其应用于视频语言任务时面临挑战,因为视频需要更长的令牌长度。为解决这些问题,我们提出了VideoICL,这是一种用于OOD任务的新型视频上下文学习框架,它引入了基于相似度的相关示例选择策略和基于信心的迭代推理方法。这允许选择最相关的示例并根据相似性对其进行排名,用于推理。如果生成的响应缺乏信心,我们的框架会选择新的示例并再次进行推理,通过迭代优化结果直至获得高信心的响应。这种方法在不产生高昂成本的情况下扩展了有效的上下文长度,提高了OOD视频理解性能。在多个基准测试上的实验结果表明了显著的性能提升,特别是在特定领域场景中,为更广泛的视频理解应用奠定了基础。
Key Takeaways
- 视频大型多模态模型(LMMs)在视频理解和推理方面取得了显著进展。
- 在训练数据缺乏代表性的任务(OOD任务)上,LMMs性能有所下降。
- 传统方法在OOD任务上因高计算成本而不实用。
- 上下文内学习(ICL)在视频语言任务中应用时面临挑战,尤其是视频需要更长的令牌长度。
- VideoICL是一种新型视频上下文学习框架,用于处理OOD任务。
- VideoICL通过引入基于相似度的相关示例选择策略和基于信心的迭代推理方法,提高了OOD视频理解性能。
点此查看论文截图
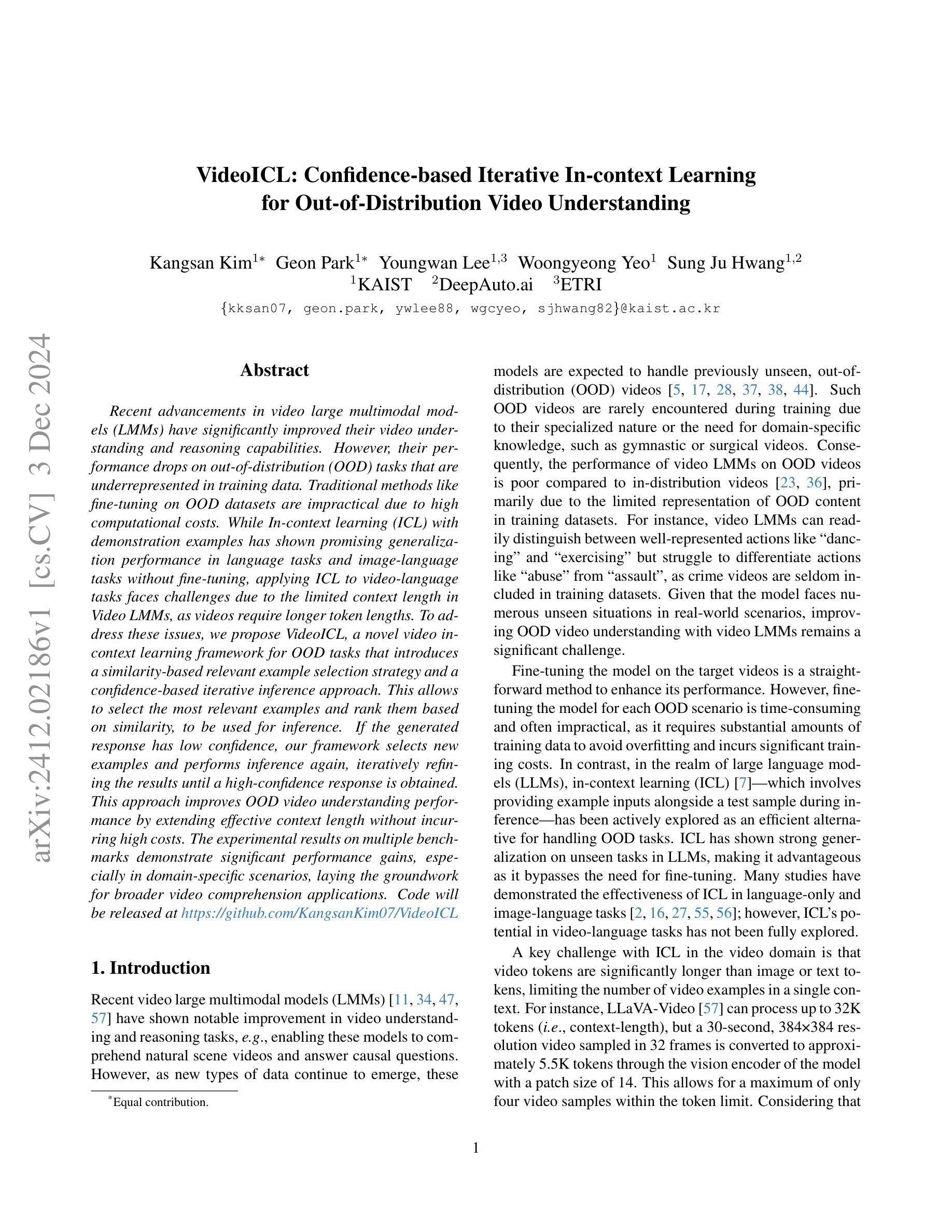
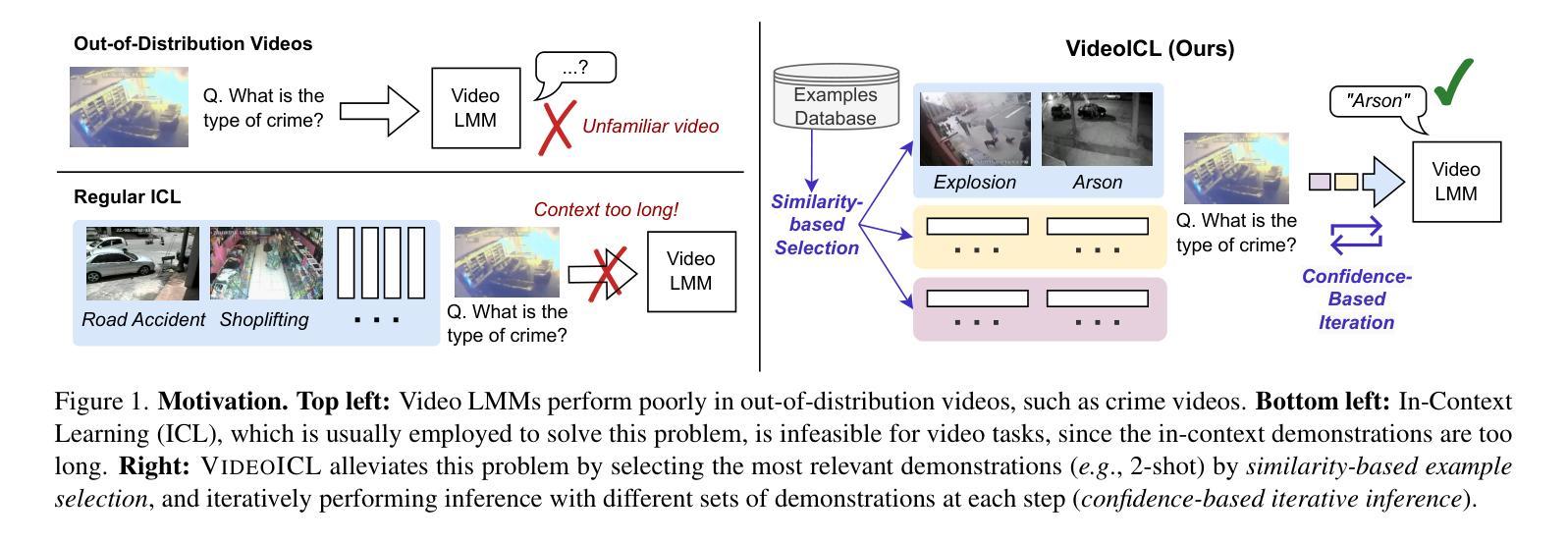
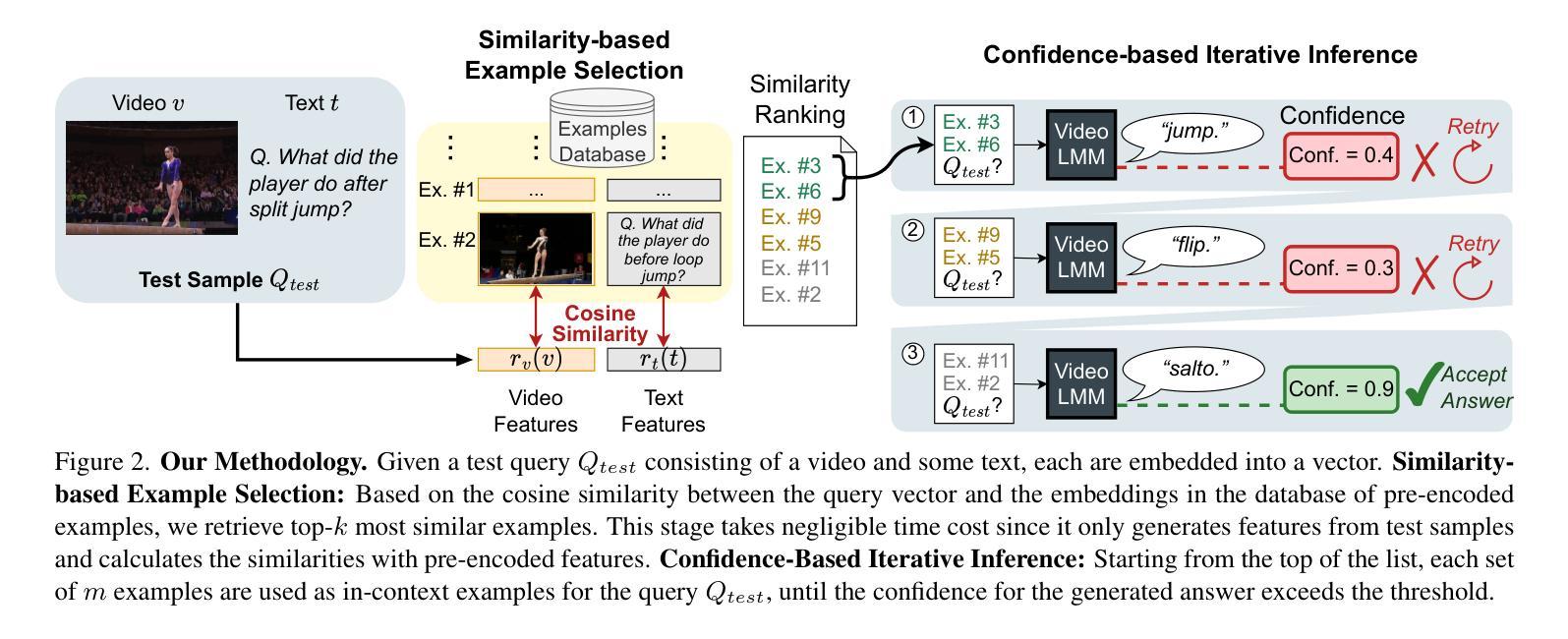
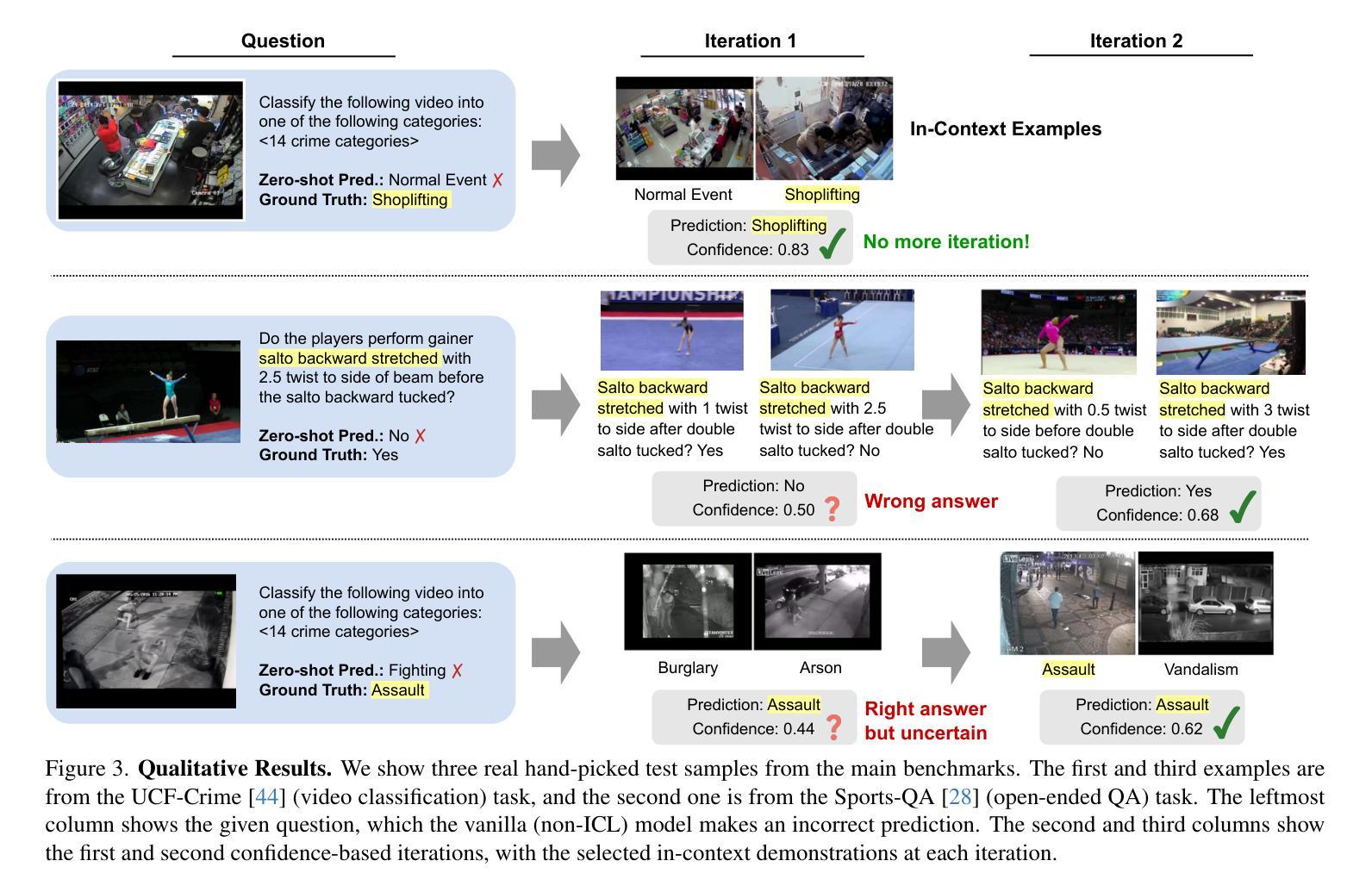
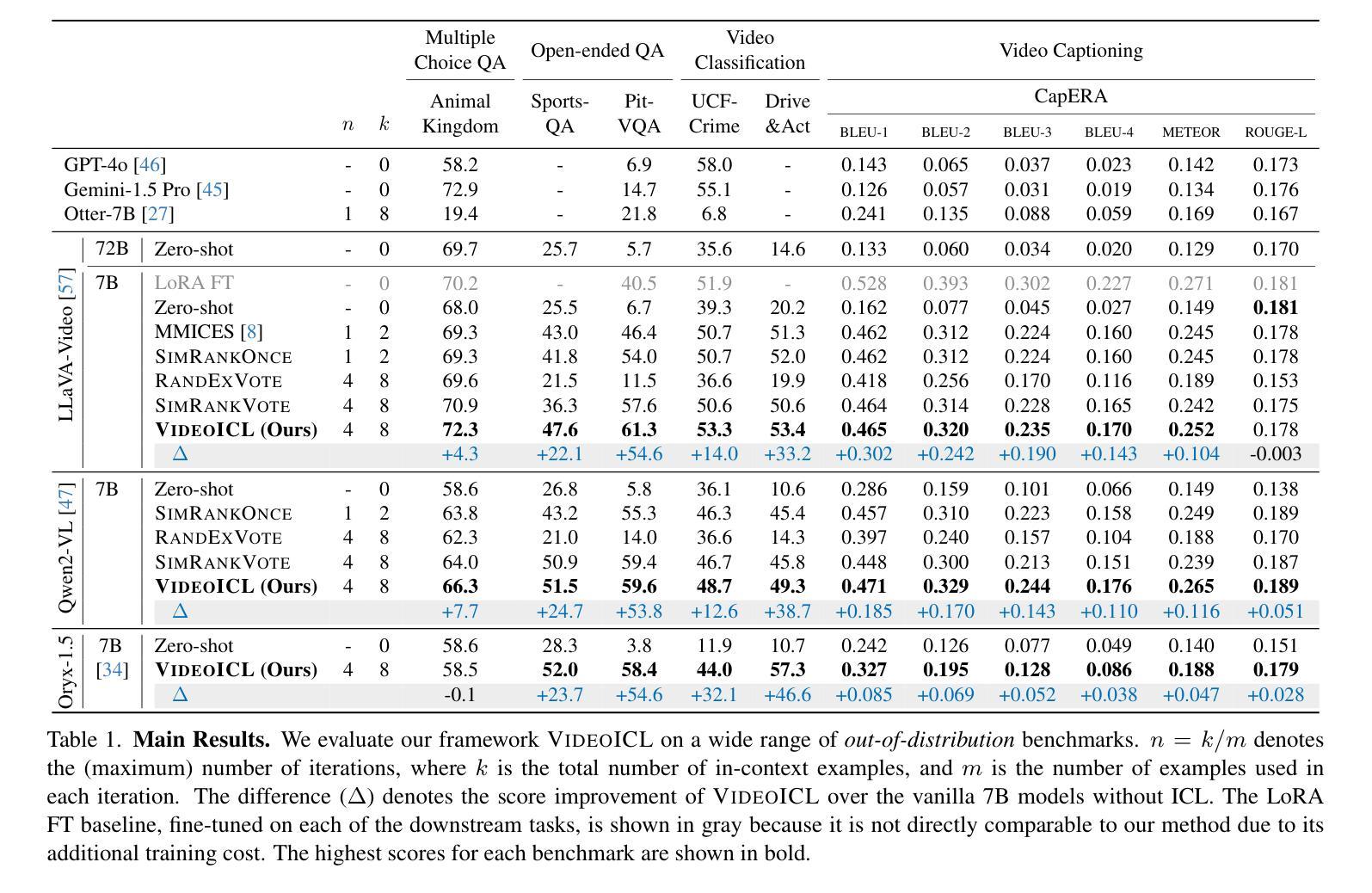
Understanding Particles From Video: Property Estimation of Granular Materials via Visuo-Haptic Learning
Authors:Zeqing Zhang, Guangze Zheng, Xuebo Ji, Guanqi Chen, Ruixing Jia, Wentao Chen, Guanhua Chen, Liangjun Zhang, Jia Pan
Granular materials (GMs) are ubiquitous in daily life. Understanding their properties is also important, especially in agriculture and industry. However, existing works require dedicated measurement equipment and also need large human efforts to handle a large number of particles. In this paper, we introduce a method for estimating the relative values of particle size and density from the video of the interaction with GMs. It is trained on a visuo-haptic learning framework inspired by a contact model, which reveals the strong correlation between GM properties and the visual-haptic data during the probe-dragging in the GMs. After training, the network can map the visual modality well to the haptic signal and implicitly characterize the relative distribution of particle properties in its latent embeddings, as interpreted in that contact model. Therefore, we can analyze GM properties using the trained encoder, and only visual information is needed without extra sensory modalities and human efforts for labeling. The presented GM property estimator has been extensively validated via comparison and ablation experiments. The generalization capability has also been evaluated and a real-world application on the beach is also demonstrated. Experiment videos are available at \url{https://sites.google.com/view/gmwork/vhlearning} .
颗粒材料(GMs)在日常生活中无处不在。了解它们的特性也非常重要,特别是在农业和工业中。然而,现有工作还需要专门的测量设备,并需要大量的人力来处理大量的颗粒。在本文中,我们介绍了一种方法,可以通过与颗粒材料的交互视频来估计颗粒尺寸和密度的相对值。它是基于视觉触觉学习框架进行训练的,该框架受到接触模型的启发,揭示了颗粒材料属性与探针拖动过程中的视觉触觉数据之间的强烈相关性。训练后,网络可以将视觉模式很好地映射到触觉信号,并隐含地在其潜在嵌入中描绘颗粒属性的相对分布,正如接触模型所解释的。因此,我们可以使用经过训练的编码器来分析颗粒材料的属性,仅需要视觉信息,而无需额外的感官模式和人力标注。所呈现的颗粒材料属性估计器已通过比较和消融实验进行了广泛验证。还评估了其泛化能力,并在沙滩上进行了实际应用演示。实验视频可在[https://sites.google.com/view/gmwork/vhlearning]上查看。
论文及项目相关链接
PDF IEEE Robotics and Automation Letters, with ICRA 2025
摘要
本文主要介绍了一种通过视频互动估计颗粒材料(GM)颗粒大小和密度相对值的方法。该研究采用视觉触觉学习框架,结合接触模型,揭示颗粒属性与视频触觉数据之间的强相关性。训练后的网络能够从视觉信息中映射出触觉信号,并隐含地描绘出颗粒属性的相对分布。因此,只需视觉信息即可分析GM属性,无需额外的感官模式和人工标注。该研究已通过对比和消融实验进行了广泛验证,并评估了其泛化能力,同时展示了在沙滩等实际场景的应用。相关实验视频可在链接观看。
关键要点
- 介绍了一种通过视频估计颗粒材料(GM)颗粒大小和密度相对值的方法。
- 采用视觉触觉学习框架和接触模型,展示颗粒材料与视觉触觉数据之间的强相关性。
- 训练后的网络能够从视觉信息映射出触觉信号,隐含描绘颗粒属性的相对分布。
- 无需额外的感官模式和人工标注即可分析GM属性。
- 该方法已通过对比和消融实验进行了验证,并展示了良好的泛化能力。
- 展示了在沙滩等实际场景的应用效果。
点此查看论文截图
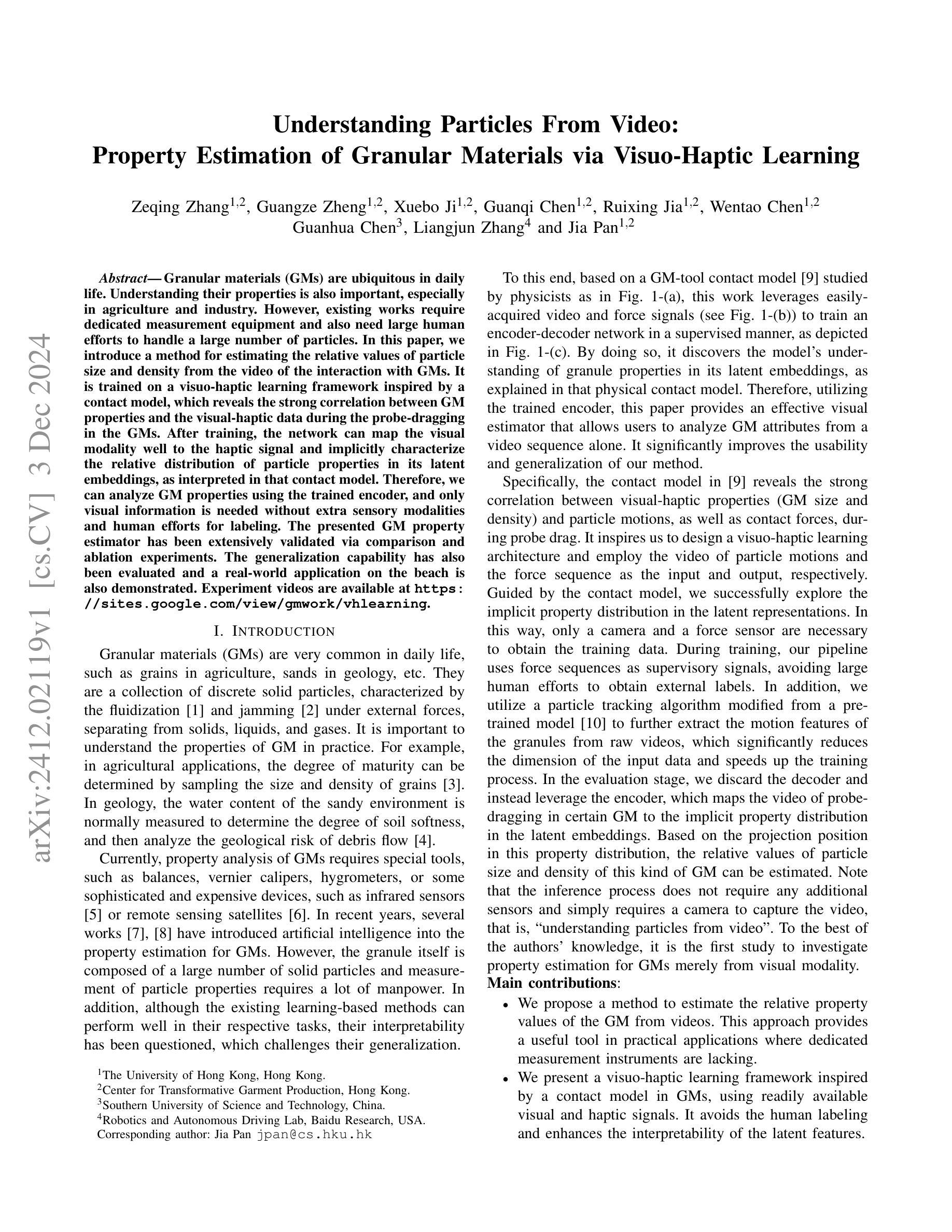
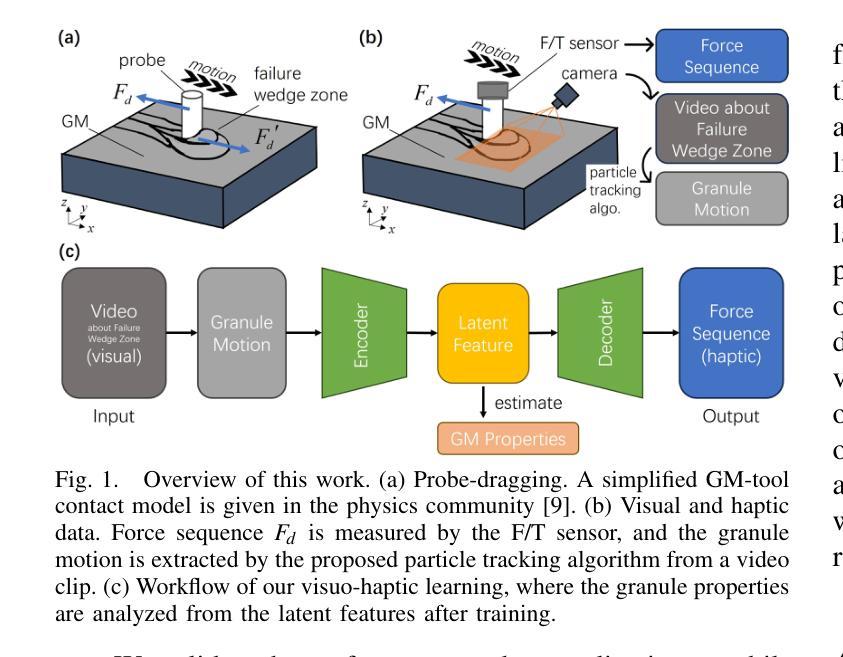
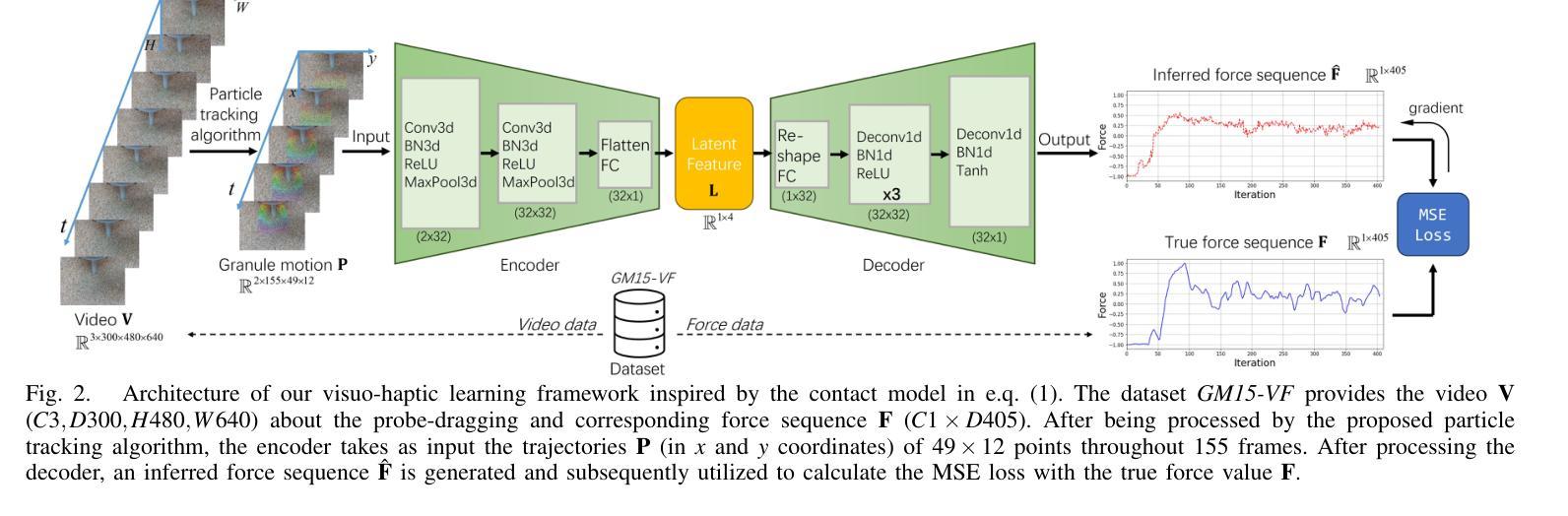
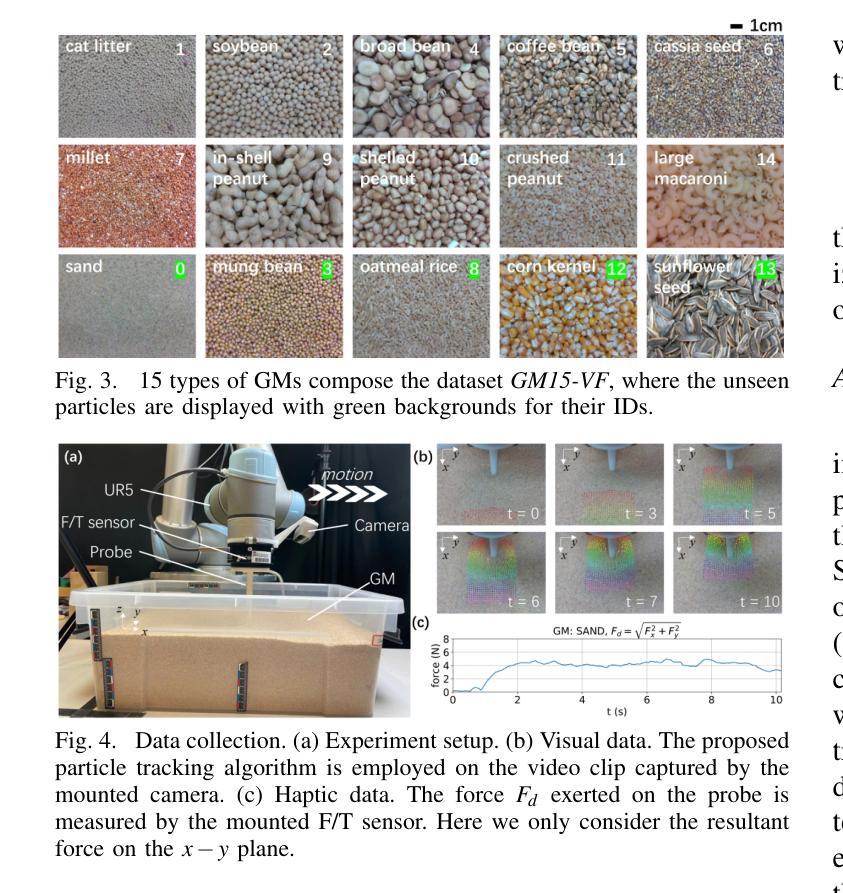
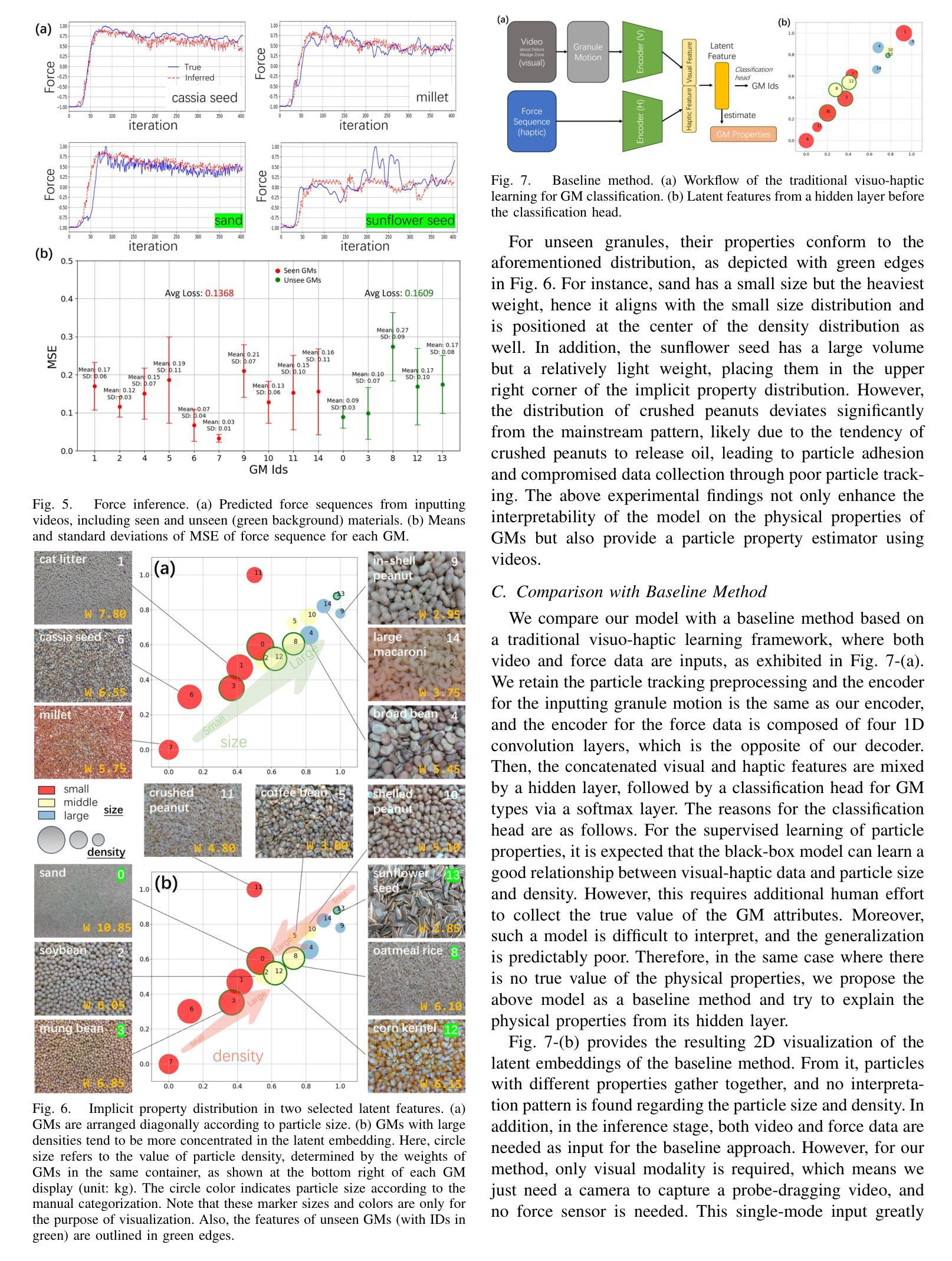
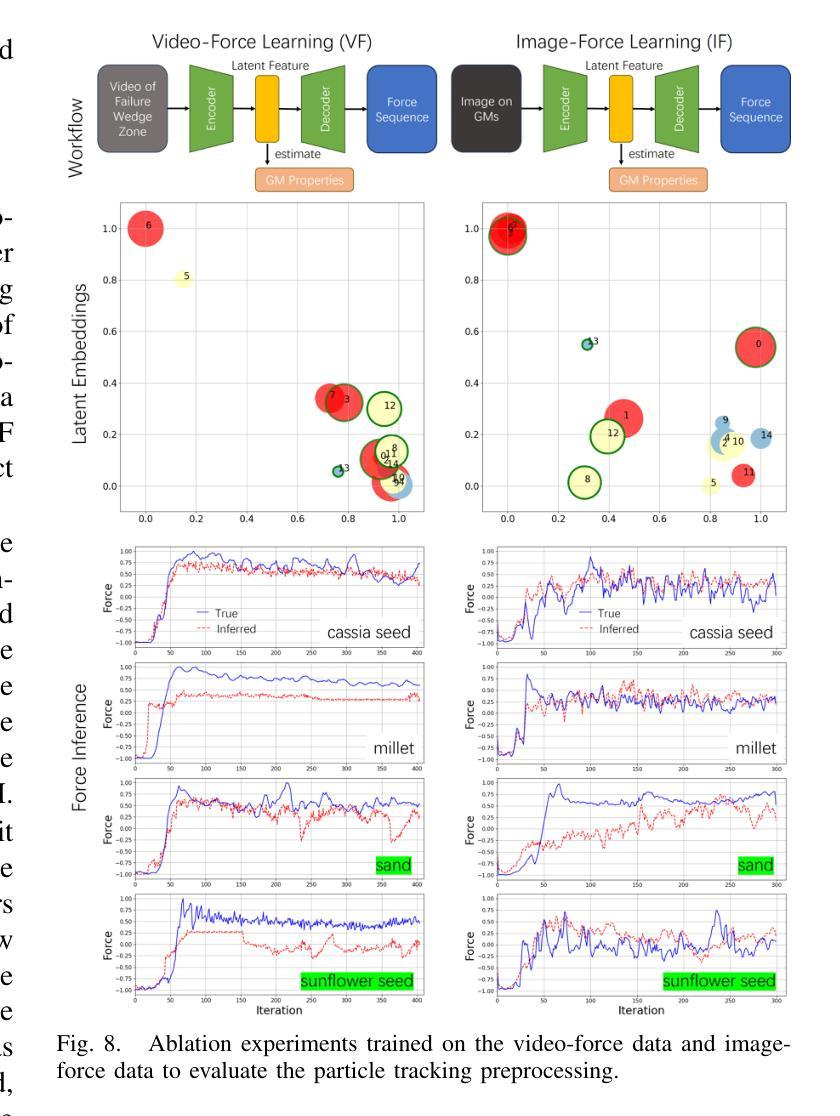
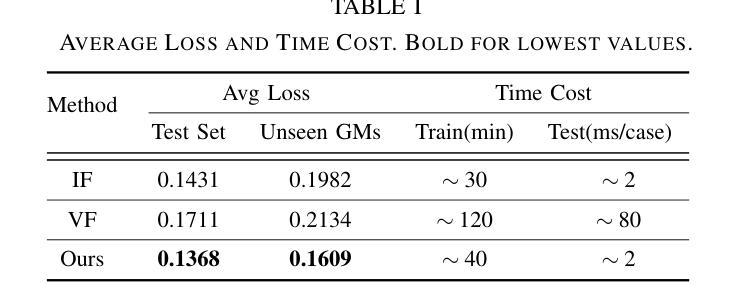
Towards Universal Soccer Video Understanding
Authors:Jiayuan Rao, Haoning Wu, Hao Jiang, Ya Zhang, Yanfeng Wang, Weidi Xie
As a globally celebrated sport, soccer has attracted widespread interest from fans all over the world. This paper aims to develop a comprehensive multi-modal framework for soccer video understanding. Specifically, we make the following contributions in this paper: (i) we introduce SoccerReplay-1988, the largest multi-modal soccer dataset to date, featuring videos and detailed annotations from 1,988 complete matches, with an automated annotation pipeline; (ii) we present the first visual-language foundation model in the soccer domain, MatchVision, which leverages spatiotemporal information across soccer videos and excels in various downstream tasks; (iii) we conduct extensive experiments and ablation studies on event classification, commentary generation, and multi-view foul recognition. MatchVision demonstrates state-of-the-art performance on all of them, substantially outperforming existing models, which highlights the superiority of our proposed data and model. We believe that this work will offer a standard paradigm for sports understanding research.
作为一项全球广受欢迎的运动,足球吸引了来自世界各地球迷的广泛关注。本文旨在开发一个用于足球视频理解的全面多模式框架。具体来说,本文做出了以下贡献:(i)我们介绍了SoccerReplay-1988,这是迄今为止最大的多模式足球数据集,它通过自动化注释管道对来自1988场完整比赛的视频和详细注释进行了介绍;(ii)我们展示了第一个在足球领域的视觉语言基础模型MatchVision,该模型利用足球视频中的时空信息,在各种下游任务中表现出色;(iii)我们在事件分类、评论生成和多视角犯规识别方面进行了广泛的实验和消去研究。MatchVision在所有任务上都表现出了最先进的性能,大幅优于现有模型,凸显了我们提出的数据和模型的优越性。我们相信这项工作将为体育理解研究提供一个标准范式。
论文及项目相关链接
PDF Technical Report; Project Page: https://jyrao.github.io/UniSoccer/
Summary
本文旨在开发一个全面的多模式框架,用于足球视频理解。主要贡献包括:引入迄今为止最大的多模式足球数据集SoccerReplay-1988,包含1988场完整比赛的自动注释视频;推出首个足球领域的视觉语言基础模型MatchVision,其在多个下游任务上表现出色;通过广泛的实验和对照研究验证了在事件分类、评论生成和多视角犯规识别等方面的先进性能。本文有望为体育理解研究提供标准范例。
Key Takeaways
- 引入多模式足球数据集SoccerReplay-1988,包含大量视频和详细注释。
- 提出首个针对足球领域的视觉语言基础模型MatchVision。
- MatchVision模型利用时空信息,在多种下游任务上表现优异。
- 通过实验和对照研究验证了模型在事件分类、评论生成和多视角犯规识别方面的性能。
- 该工作提供了全面的足球视频理解框架,展示了其在实际应用中的有效性。
- SoccerReplay-1988数据集的自动注释管道是一个重要的技术亮点。
点此查看论文截图
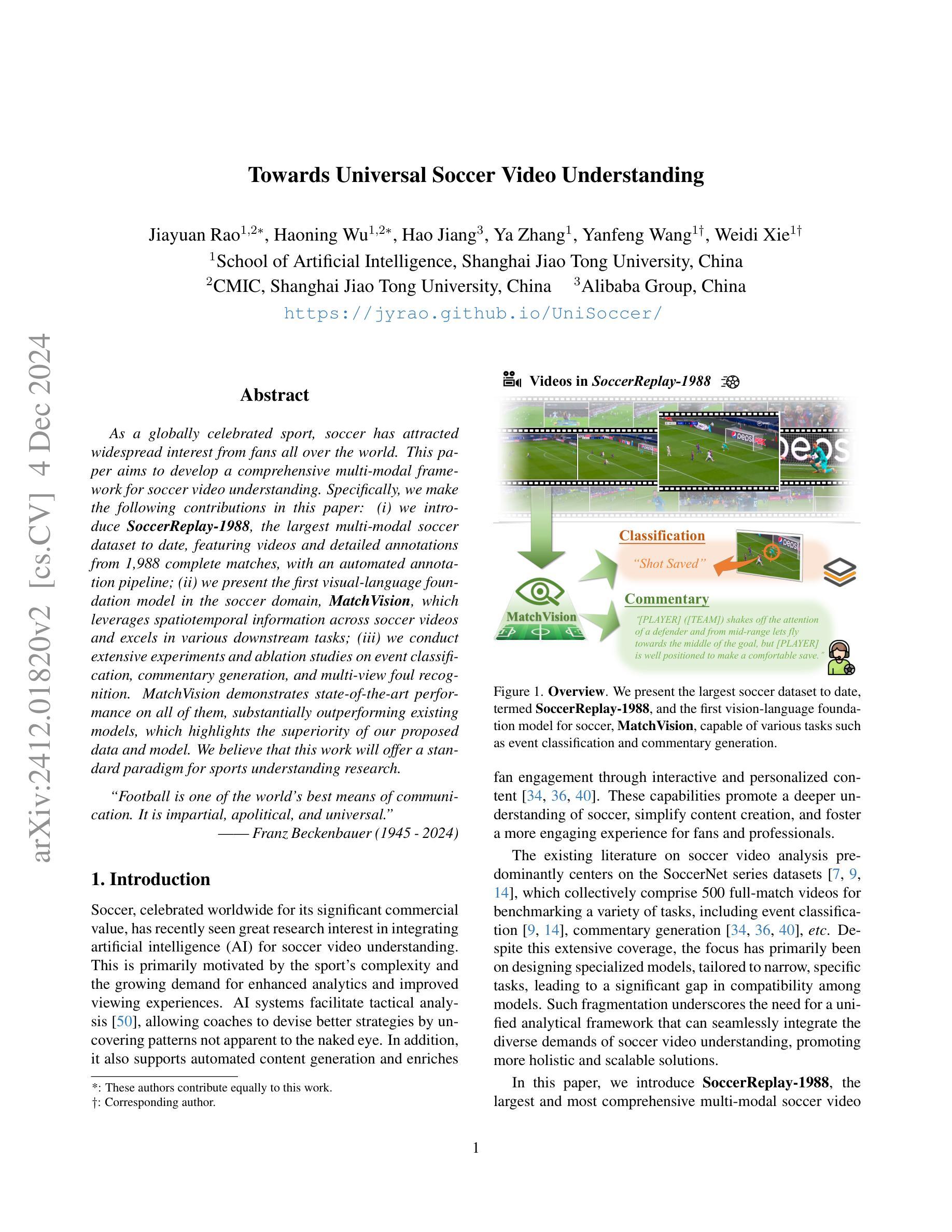
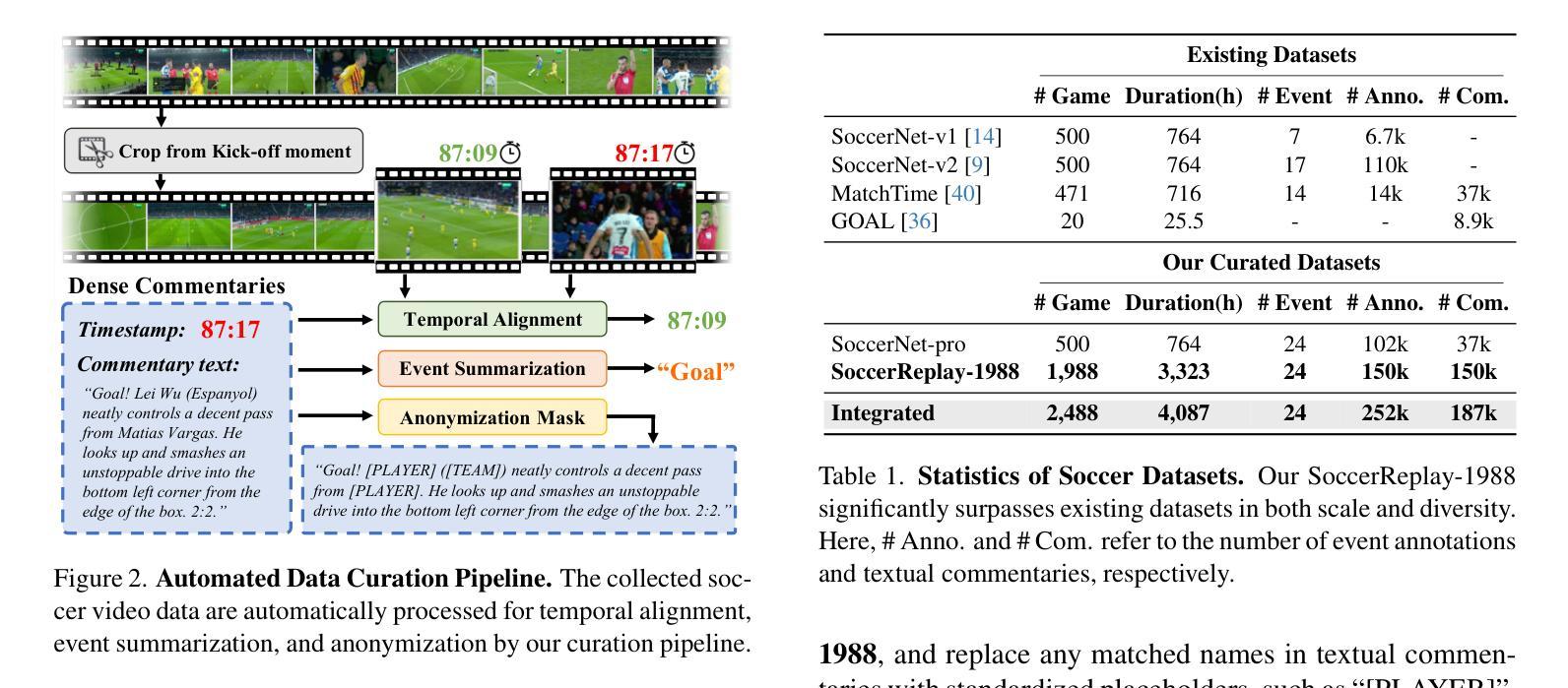
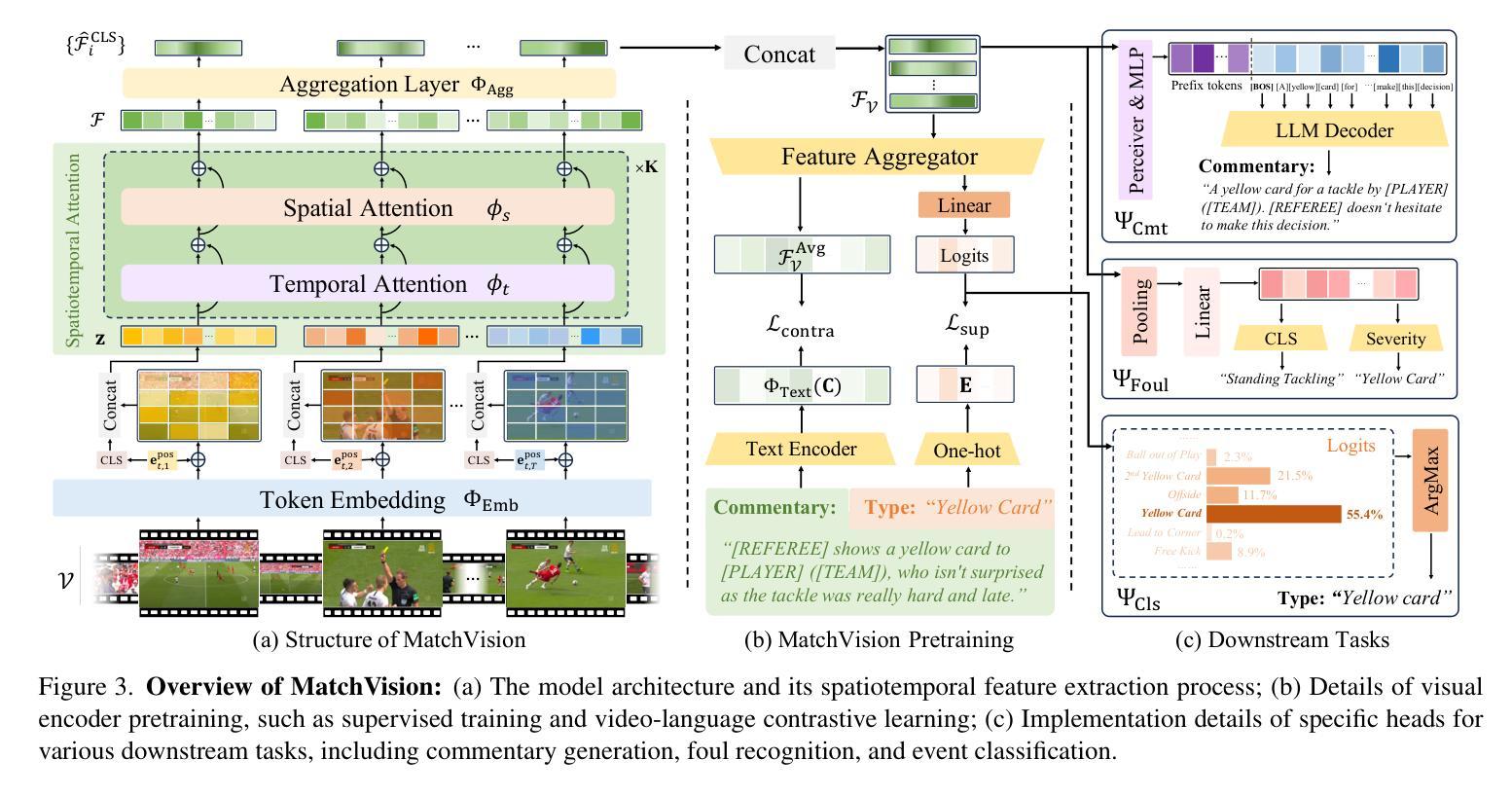
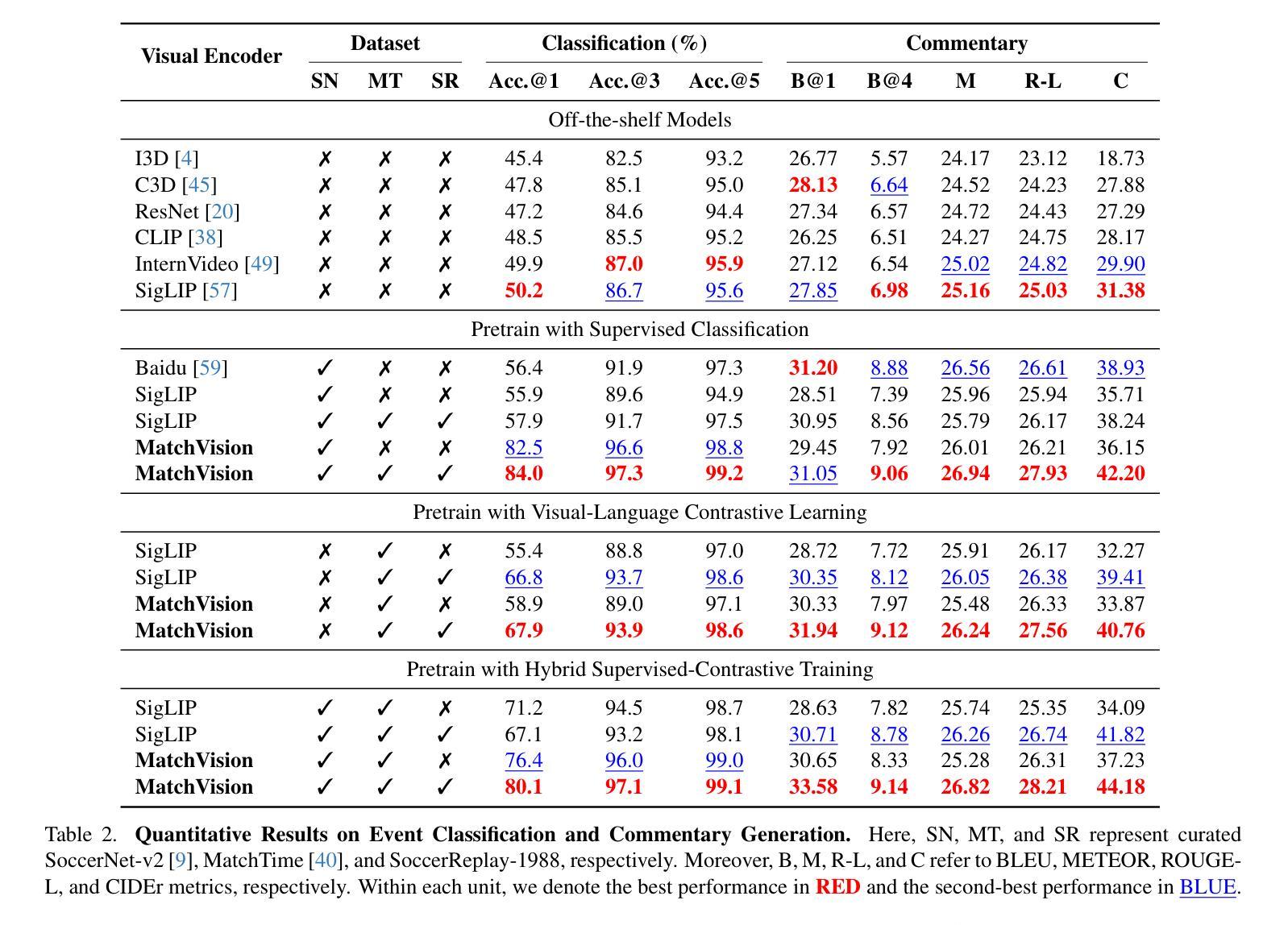
VISTA: Enhancing Long-Duration and High-Resolution Video Understanding by Video Spatiotemporal Augmentation
Authors:Weiming Ren, Huan Yang, Jie Min, Cong Wei, Wenhu Chen
Current large multimodal models (LMMs) face significant challenges in processing and comprehending long-duration or high-resolution videos, which is mainly due to the lack of high-quality datasets. To address this issue from a data-centric perspective, we propose VISTA, a simple yet effective Video Spatiotemporal Augmentation framework that synthesizes long-duration and high-resolution video instruction-following pairs from existing video-caption datasets. VISTA spatially and temporally combines videos to create new synthetic videos with extended durations and enhanced resolutions, and subsequently produces question-answer pairs pertaining to these newly synthesized videos. Based on this paradigm, we develop seven video augmentation methods and curate VISTA-400K, a video instruction-following dataset aimed at enhancing long-duration and high-resolution video understanding. Finetuning various video LMMs on our data resulted in an average improvement of 3.3% across four challenging benchmarks for long-video understanding. Furthermore, we introduce the first comprehensive high-resolution video understanding benchmark HRVideoBench, on which our finetuned models achieve a 6.5% performance gain. These results highlight the effectiveness of our framework.
当前的大型多模态模型(LMM)在处理和理解长时长或高分辨率视频时面临重大挑战,这主要是因为缺乏高质量的数据集。为了从数据中心的视角解决这个问题,我们提出了VISTA,这是一个简单有效的视频时空增强框架,它可以从现有的视频字幕数据集中合成长时长和高分辨率的视频指令对。VISTA在空间和时间上结合视频,以创建具有扩展时长和增强分辨率的新合成视频,并随后生成与这些新合成视频相关的问题答案对。基于此范式,我们开发了七种视频增强方法,并策划了VISTA-400K,这是一个旨在增强长时长和高分辨率视频理解能力的视频指令遵循数据集。在我们的数据集上进行微调的各种视频LMM在四个具有挑战性的长视频理解基准测试上平均提高了3.3%的性能。此外,我们还推出了首个全面高分辨率视频理解基准测试HRVideoBench,我们的微调模型在此基准测试上取得了6.5%的性能提升。这些结果凸显了我们框架的有效性。
论文及项目相关链接
PDF Project Page: https://tiger-ai-lab.github.io/VISTA/
Summary
该文本提出了一种名为VISTA的视频时空增强框架,用于从现有视频字幕数据集中合成长时和高清视频指令对。通过空间和时间上的视频组合,创建新的合成视频,并生成与之相关的问题答案对。基于这一框架,开发了七种视频增强方法,并创建了VISTA-400K视频指令遵循数据集,旨在提高长时和高清视频的理解能力。
Key Takeaways
- VISTA框架解决了大型多模态模型在处理长时或高清视频时面临的挑战,主要原因是缺乏高质量的数据集。
- VISTA通过空间和时间上的视频组合,合成长时和高清视频。
- VISTA能够生成与合成视频相关的问题答案对。
- 开发了七种视频增强方法,以提高视频数据的质量。
- 创建了VISTA-400K视频指令遵循数据集,旨在提高长时和高清视频的理解能力。
- 通过在VISTA-400K数据集上进行微调,大型多模态模型在长视频理解方面取得了平均3.3%的改进。
点此查看论文截图
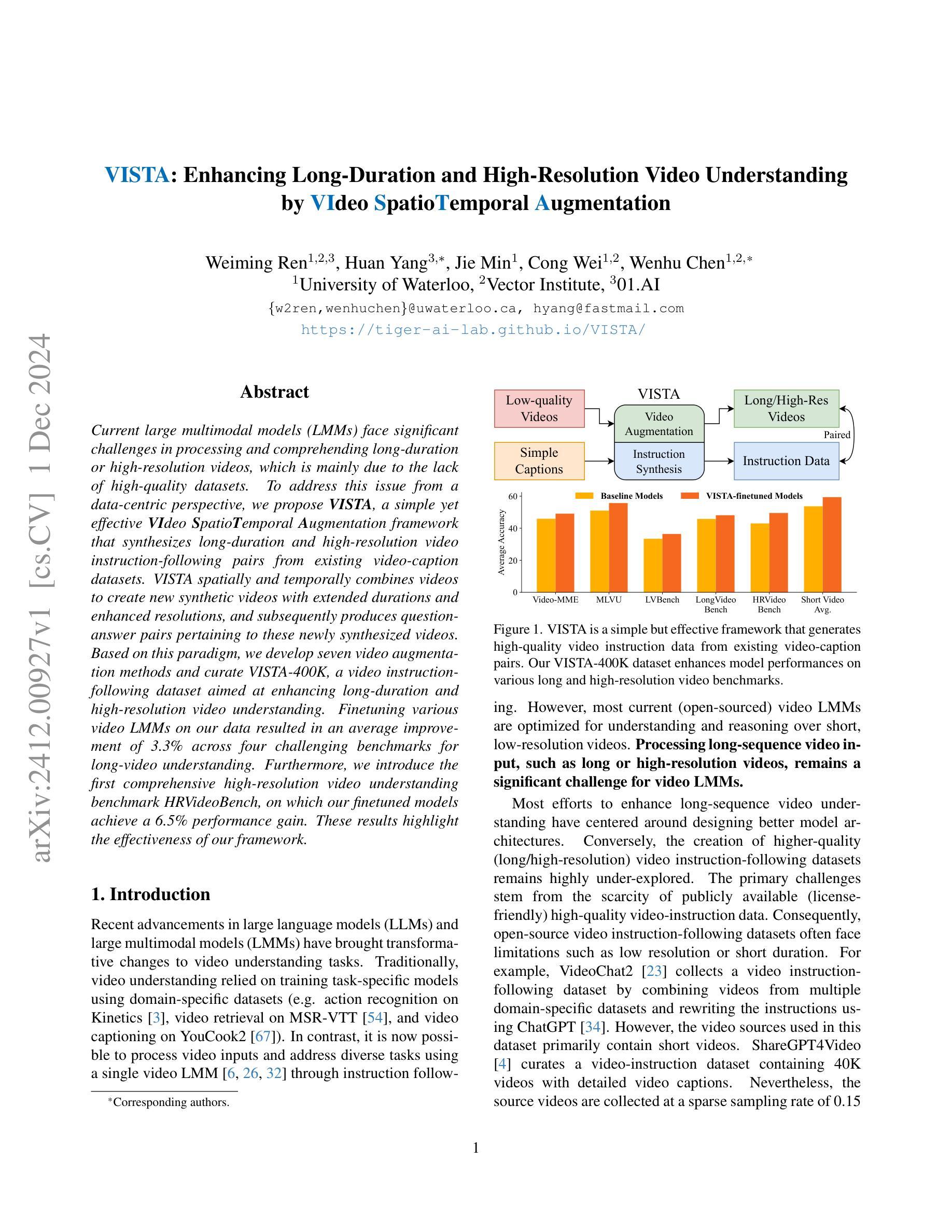
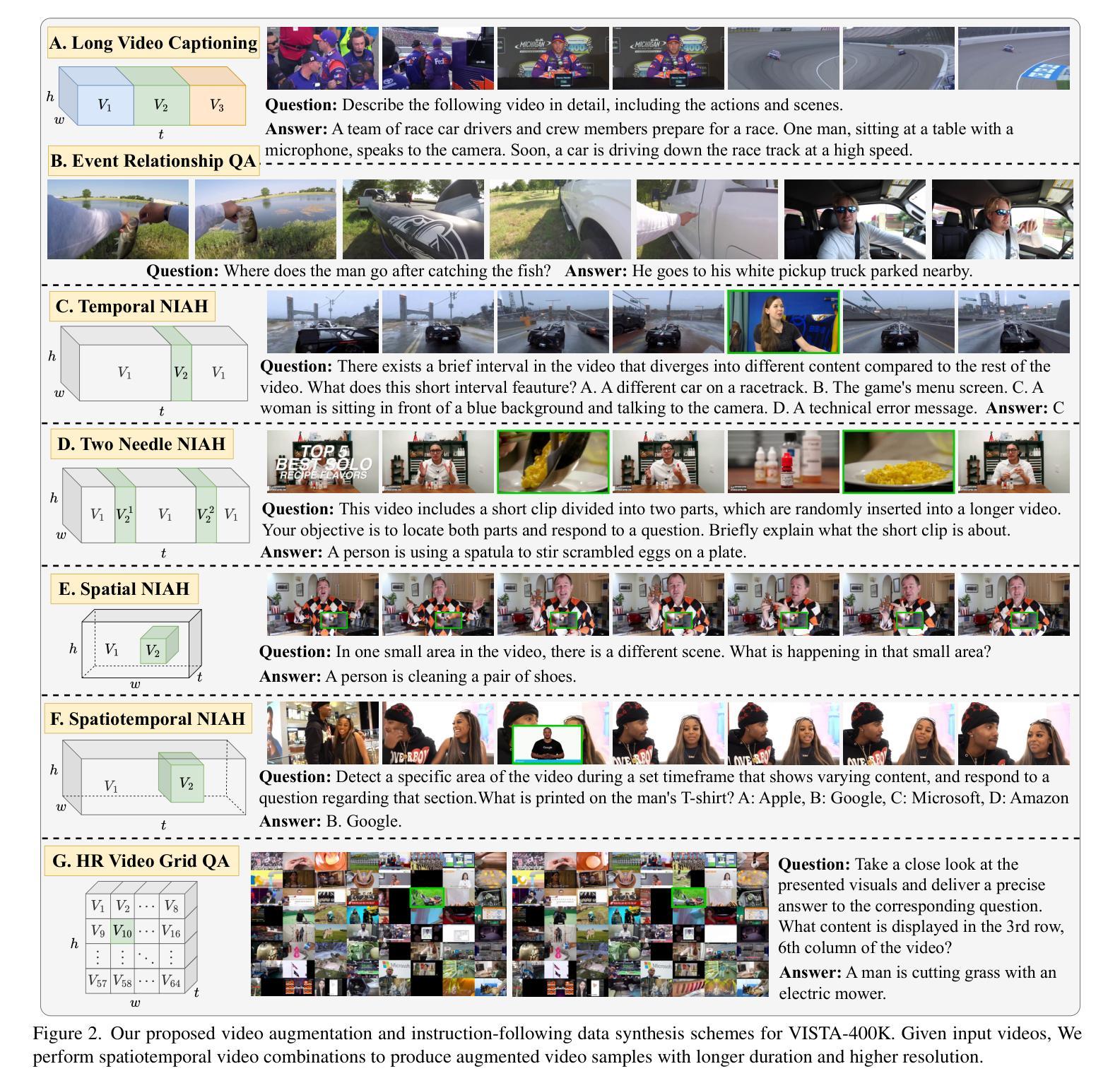
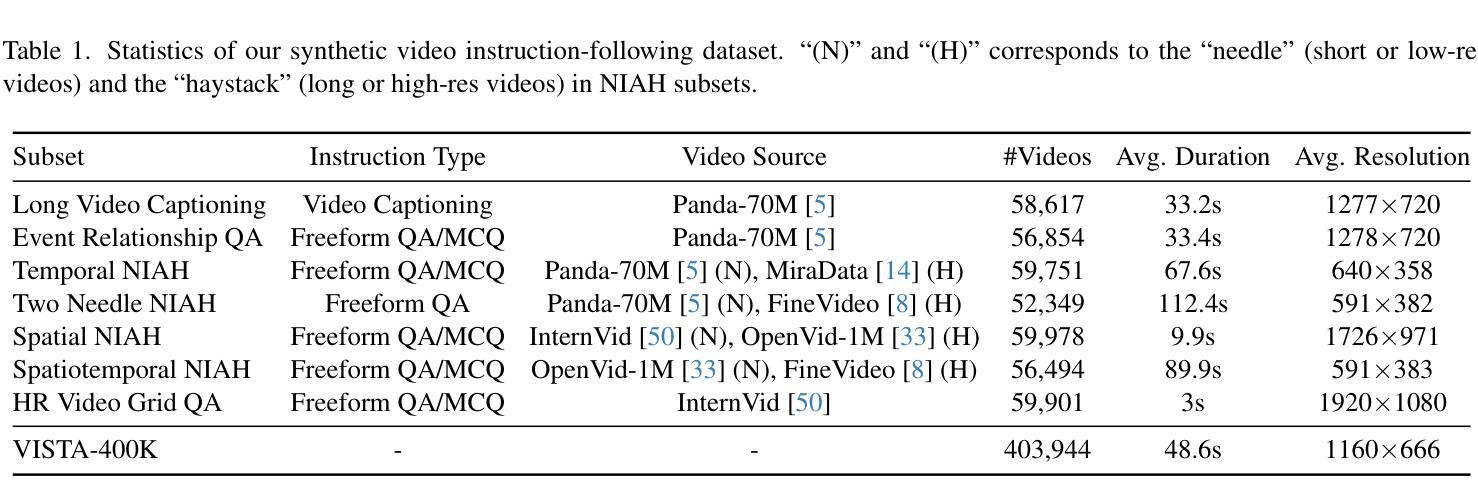
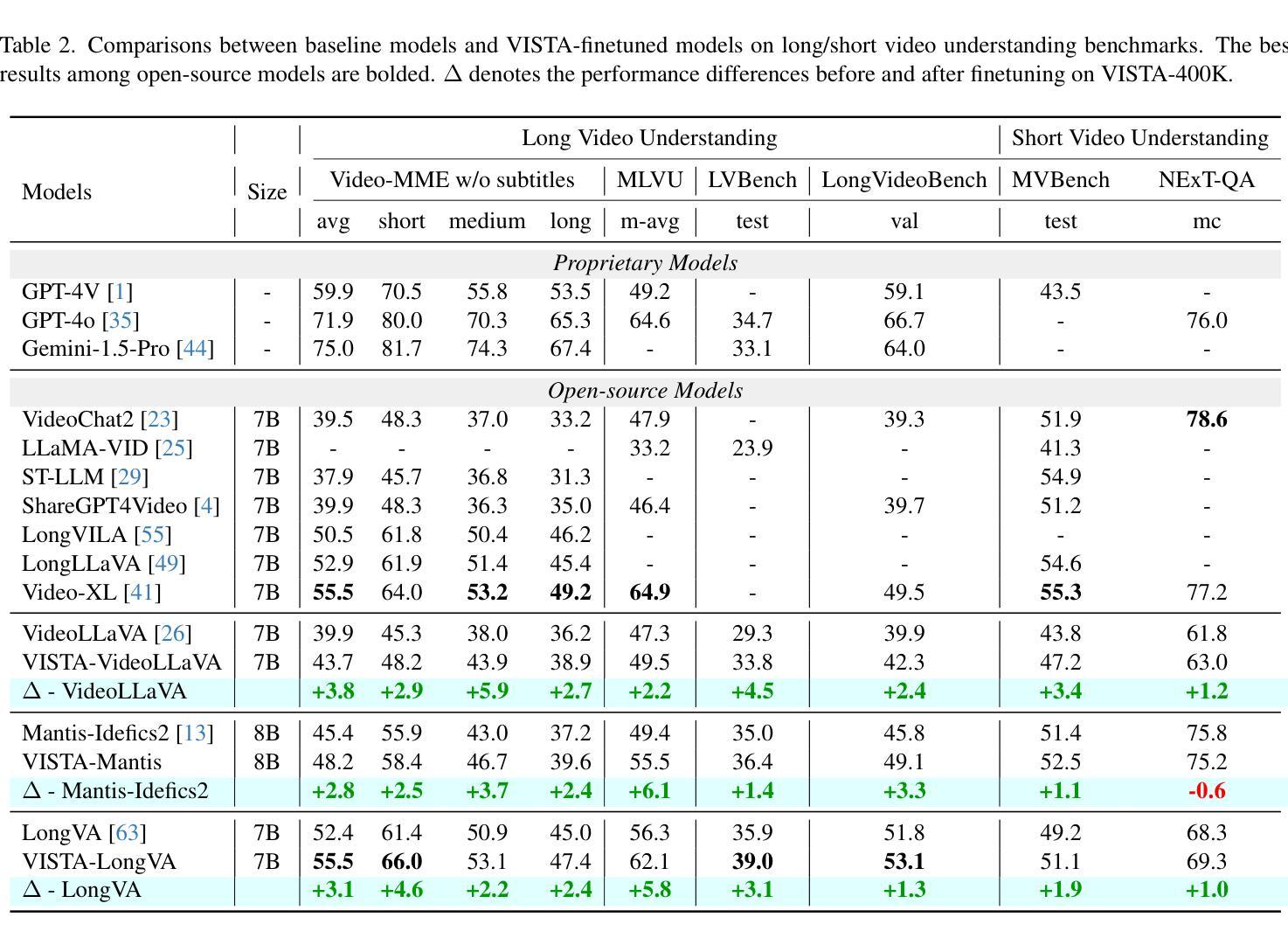
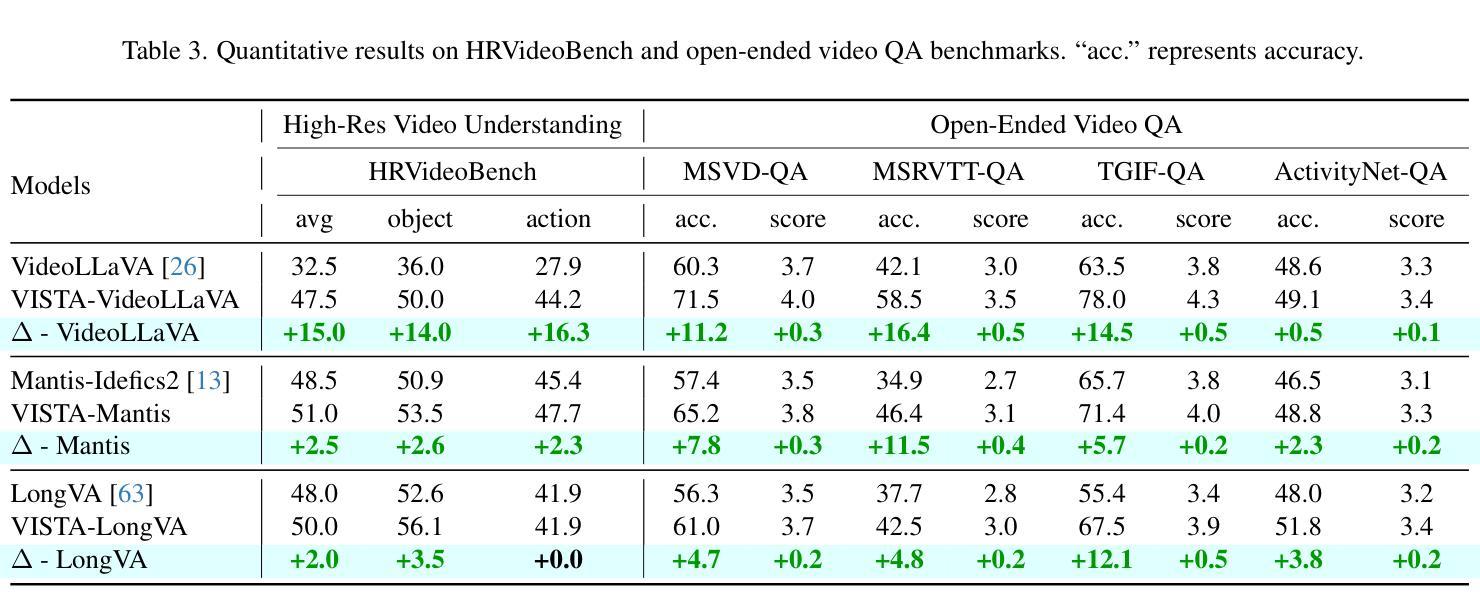
Video-3D LLM: Learning Position-Aware Video Representation for 3D Scene Understanding
Authors:Duo Zheng, Shijia Huang, Liwei Wang
The rapid advancement of Multimodal Large Language Models (MLLMs) has significantly impacted various multimodal tasks. However, these models face challenges in tasks that require spatial understanding within 3D environments. Efforts to enhance MLLMs, such as incorporating point cloud features, have been made, yet a considerable gap remains between the models’ learned representations and the inherent complexity of 3D scenes. This discrepancy largely stems from the training of MLLMs on predominantly 2D data, which restricts their effectiveness in comprehending 3D spaces. To address this issue, in this paper, we propose a novel generalist model, i.e., Video-3D LLM, for 3D scene understanding. By treating 3D scenes as dynamic videos and incorporating 3D position encoding into these representations, our Video-3D LLM aligns video representations with real-world spatial contexts more accurately. Additionally, we have implemented a maximum coverage sampling technique to optimize the balance between computational costs and performance efficiency. Extensive experiments demonstrate that our model achieves state-of-the-art performance on several 3D scene understanding benchmarks, including ScanRefer, Multi3DRefer, Scan2Cap, ScanQA, and SQA3D.
多模态大型语言模型(MLLMs)的快速发展对多种多模态任务产生了重大影响。然而,这些模型在处理需要在3D环境中进行空间理解的任务时面临挑战。为了增强MLLMs的功能,已经做出了努力,如融入点云特征,但模型所学习到的表示与3D场景固有复杂性之间仍存在较大差距。这种差异主要源于MLLMs主要在2D数据上的训练,这限制了它们在理解3D空间方面的有效性。为了解决这一问题,本文提出了一种新型通用模型,即Video-3D LLM,用于3D场景理解。我们将3D场景视为动态视频,并将3D位置编码融入这些表示中,我们的Video-3D LLM能够更准确地使视频表示与真实世界空间上下文对齐。此外,我们还实现了一种最大覆盖采样技术,以优化计算成本与性能效率之间的平衡。大量实验表明,我们的模型在多个3D场景理解基准测试上达到了最新技术水平,包括ScanRefer、Multi3DRefer、Scan2Cap、ScanQA和SQA3D。
论文及项目相关链接
PDF 14 pages, 4 figures
Summary
多媒体大型语言模型(MLLMs)在多模态任务中有显著影响,但在需要空间理解的3D环境中面临挑战。为解决现有模型的不足,本文提出了一种新型通用模型——Video-3D LLM,通过动态视频理解结合真实空间语境实现准确高效的3D场景理解。采用最大覆盖采样技术优化计算成本和性能效率之间的平衡。实验证明,该模型在多个3D场景理解基准测试中表现优异。
Key Takeaways
- MLLMs在多模态任务中有显著影响,但在处理需要空间理解的3D环境任务时面临挑战。
- MLLMs主要基于二维数据训练,限制了其在理解三维空间中的有效性。
- 为解决此问题,提出了一种新型通用模型Video-3D LLM用于理解三维场景。
- Video-3D LLM通过将三维场景视为动态视频并融入三维位置编码,更准确地将视频表现与真实空间语境对齐。
- Video-3D LLM采用最大覆盖采样技术优化计算成本和性能效率之间的平衡。
- 实验证明Video-3D LLM在多个三维场景理解基准测试中达到最新技术性能水平。
点此查看论文截图
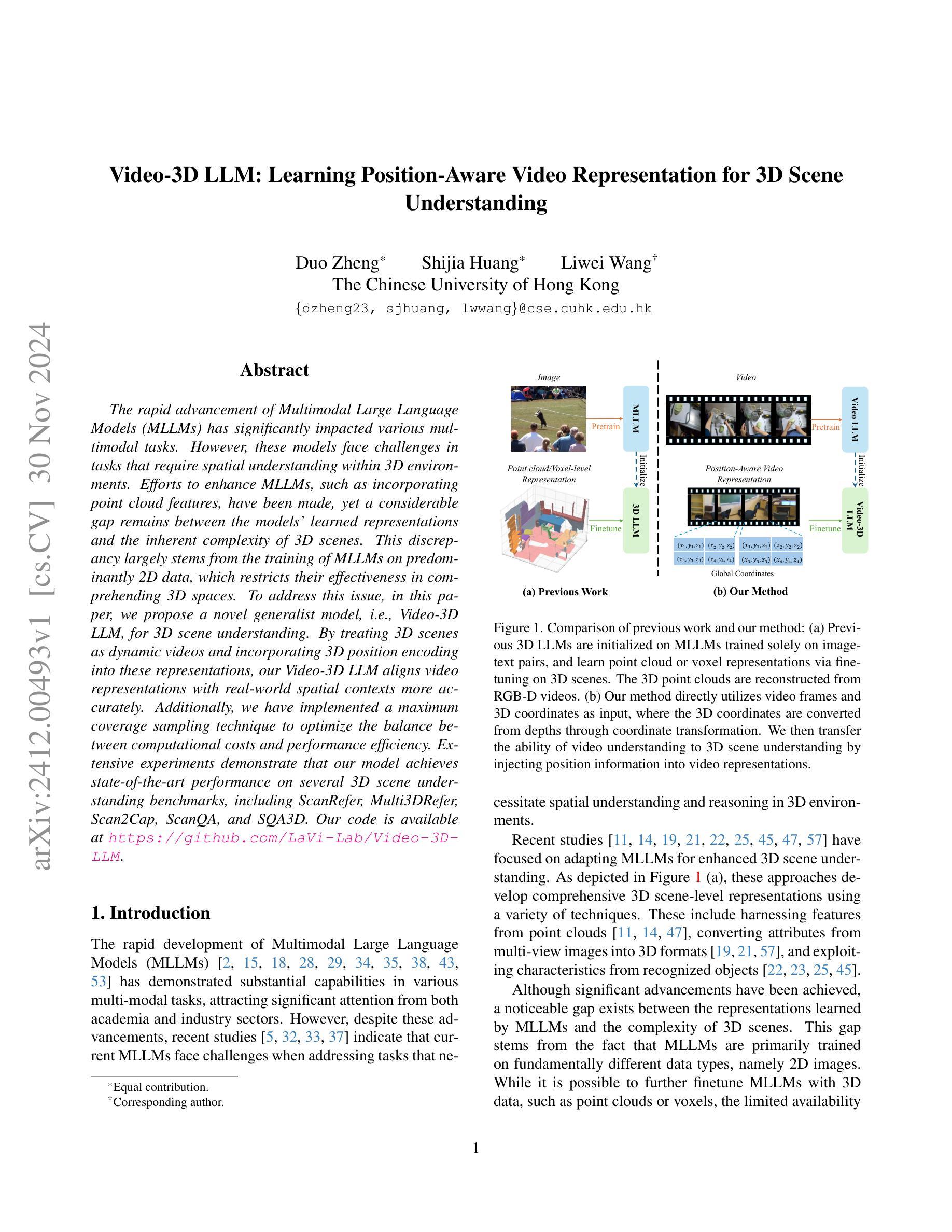
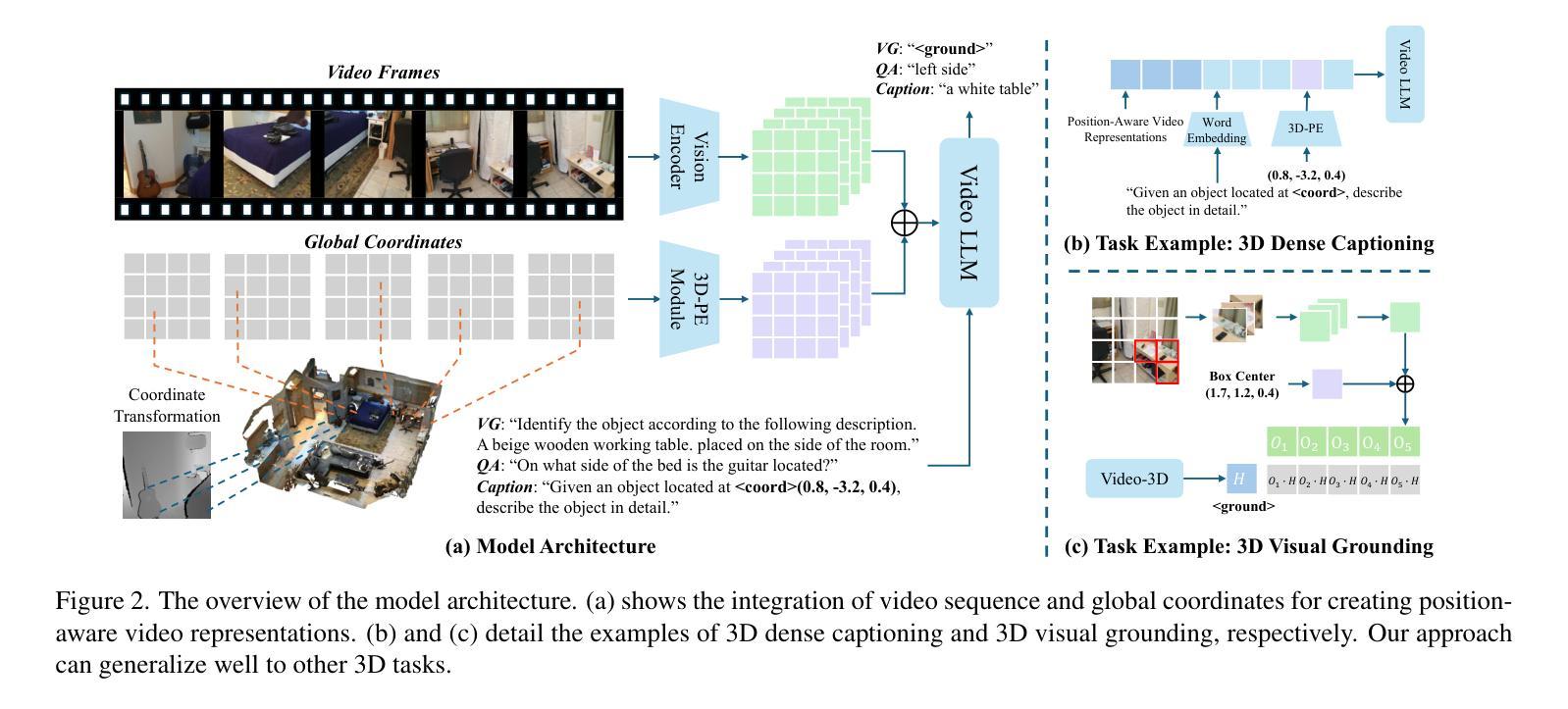
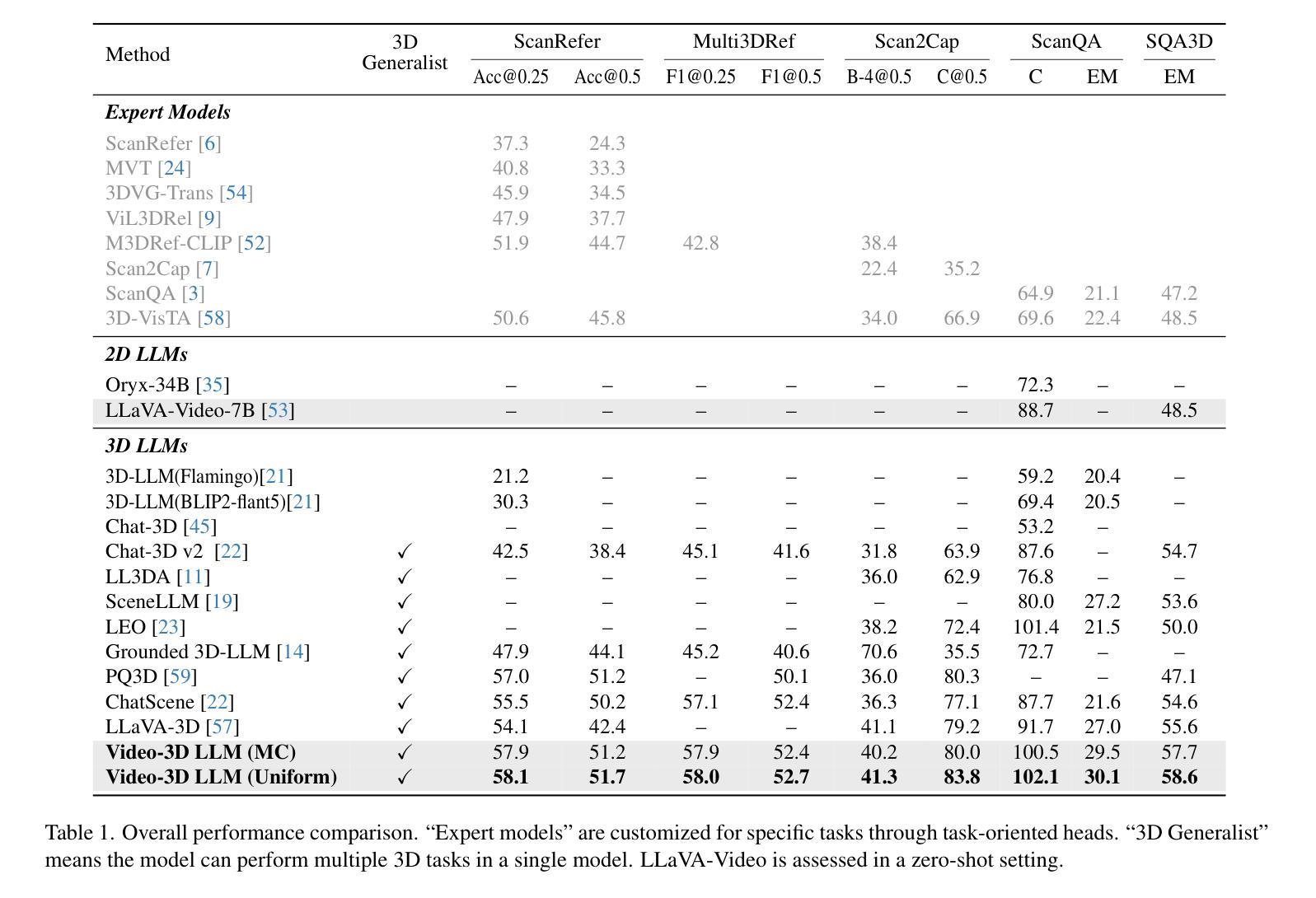
Look Every Frame All at Once: Video-Ma$^2$mba for Efficient Long-form Video Understanding with Multi-Axis Gradient Checkpointing
Authors:Hosu Lee, Junho Kim, Hyunjun Kim, Yong Man Ro
With the growing scale and complexity of video data, efficiently processing long video sequences poses significant challenges due to the quadratic increase in memory and computational demands associated with existing transformer-based Large Multi-modal Models (LMMs). To address these issues, we introduce Video-Ma$^2$mba, a novel architecture that incorporates State Space Models (SSMs) within the Mamba-2 framework, replacing the attention mechanisms. This allows the LMMs to scale linearly in terms of time and memory requirements, making it feasible to handle long-duration video content. Furthermore, we enhance the memory efficiency introducing the Multi-Axis Gradient Checkpointing (MA-GC) method, which strategically manages memory by retaining only essential activations across multiple computational axes. Our approach significantly reduces the memory footprint compared to standard gradient checkpointing. Empirical analyses show that Video-Ma$^2$mba can process extensive video sequences-equivalent to millions of tokens or over two hours of continuous sequences at 1 FPS-on a single GPU. By maintaining a detailed capture of temporal dynamics, our model improves the accuracy and relevance of responses in long video understanding tasks, demonstrating substantial advantages over existing frameworks.
随着视频数据的规模和复杂性不断增长,由于现有基于Transformer的大型多模态模型(LMMs)在内存和计算需求上的二次增长,有效地处理长视频序列带来了巨大的挑战。为了解决这些问题,我们引入了Video-Ma$^2$mba这一新型架构,它结合了Mamba-2框架中的状态空间模型(SSMs),替代了注意力机制。这使得LMM在时间和内存需求方面实现线性扩展,从而能够处理长时间的视频内容。此外,我们通过引入多轴梯度检查点(MA-GC)方法提高了内存效率,该方法通过保留多个计算轴上的重要激活来策略性地管理内存。我们的方法相比标准梯度检查点技术大大降低了内存占用。经验分析表明,Video-Ma$^2$mba可以在单个GPU上以每秒一帧的速度处理大量等效于数百万个标记或超过两小时的连续序列的视频序列。通过保持对时间动态的详细捕捉,我们的模型提高了长视频理解任务的准确性和响应的实用性,显示出相对于现有框架的巨大优势。
论文及项目相关链接
PDF Project page: https://ivy-lvlm.github.io/Video-MA2MBA/
Summary
视频数据的规模和复杂性不断增长,现有基于transformer的大型多模态模型在处理长视频序列时面临内存和计算需求呈二次方增长的挑战。为此,我们引入Video-Ma$^2$mba这一新型架构,通过状态空间模型(SSMs)在Mamba-2框架内替代注意力机制,使得大型多模态模型在时间需求上实现线性扩展。此外,通过引入多轴梯度检查点法(MA-GC),在多个计算轴上保留关键激活信息,提高内存使用效率。该方法的内存占用与标准梯度检查点法相比大幅减少。实验分析表明,Video-Ma$^2$mba能在单个GPU上处理相当于数百万令牌或超过两小时连续序列的视频数据。通过精确捕捉时间动态,该模型提高了长视频理解任务的准确性和响应相关性,展现出对现行框架的显著优势。
Key Takeaways
- 面对视频数据规模增长带来的挑战,现有基于transformer的大型多模态模型在处理长视频序列时存在内存和计算需求的问题。
- Video-Ma$^2$mba架构通过引入状态空间模型(SSMs)在Mamba-2框架内替代注意力机制,实现线性扩展。
- 多轴梯度检查点法(MA-GC)提高了内存使用效率,大幅减少内存占用。
- Video-Ma$^2$mba能在单个GPU上处理大量视频数据,相当于数百万令牌或超过两小时连续序列。
- 该模型能够精确捕捉视频的时间动态。
- Video-Ma$^2$mba提高了长视频理解任务的准确性和响应相关性。
点此查看论文截图
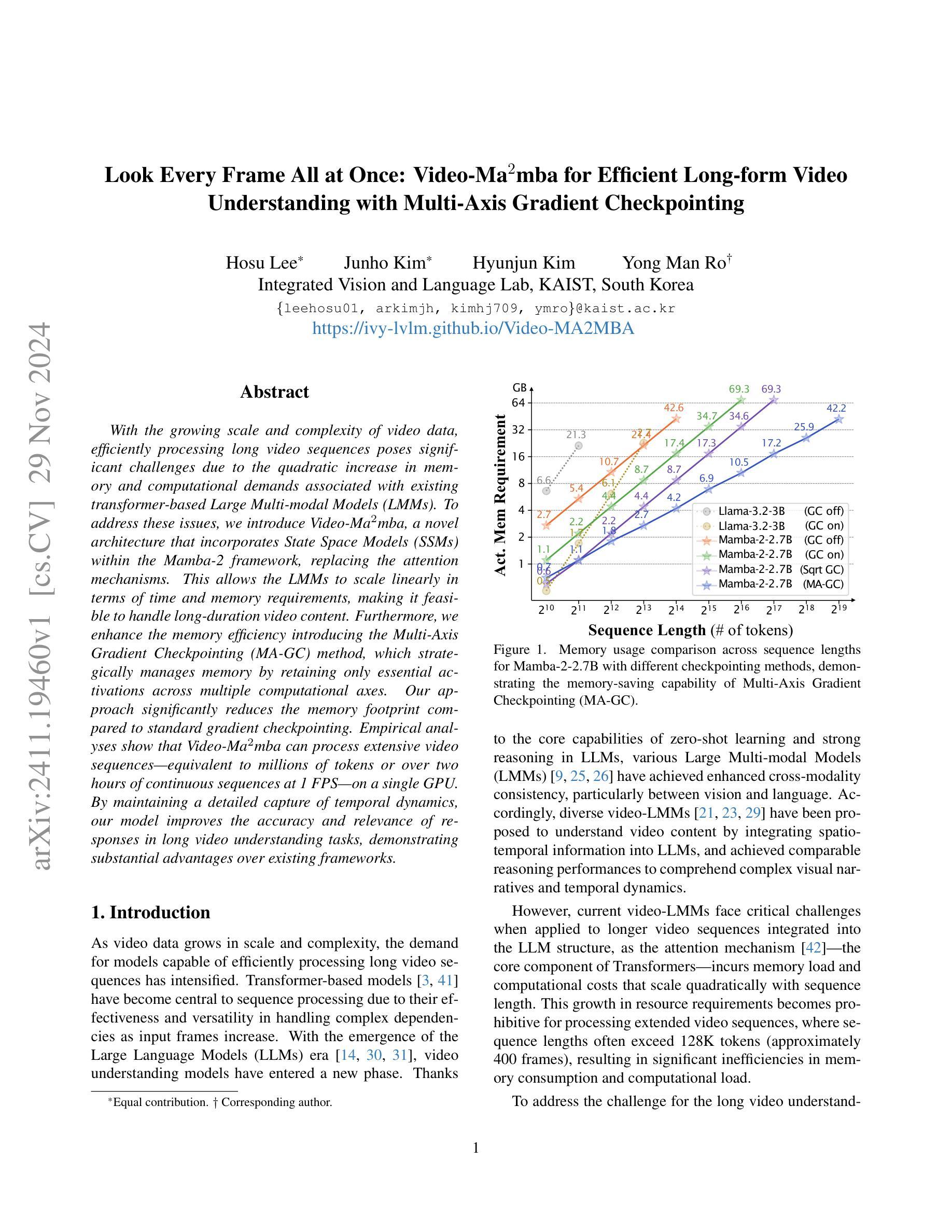
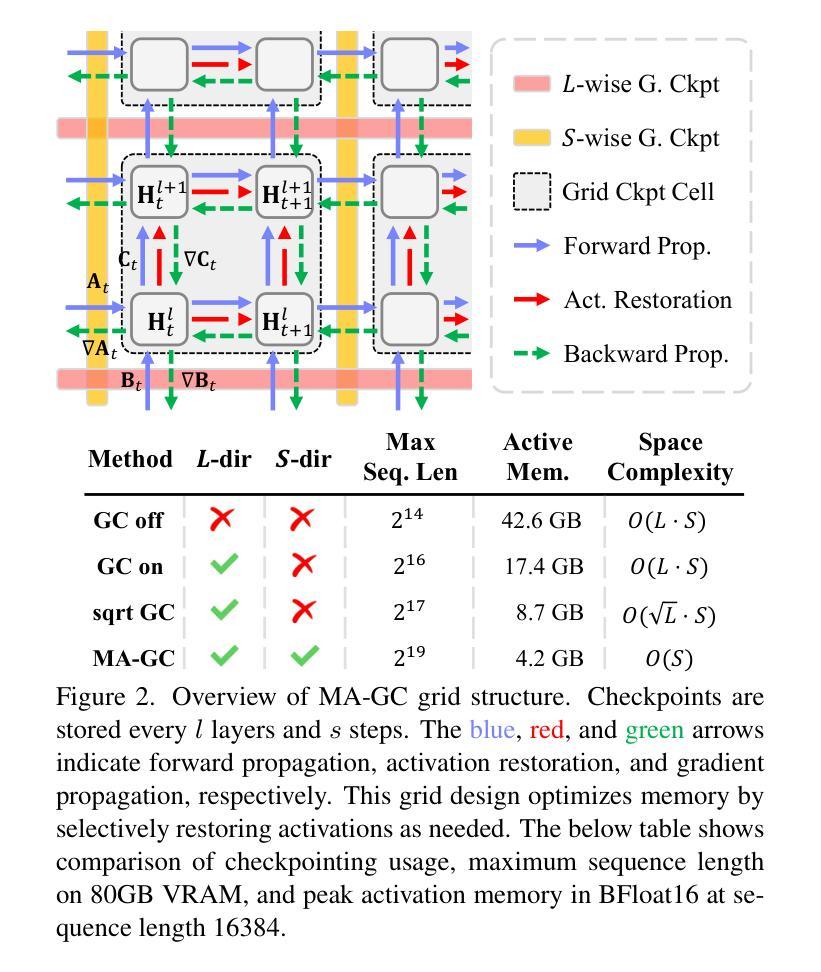
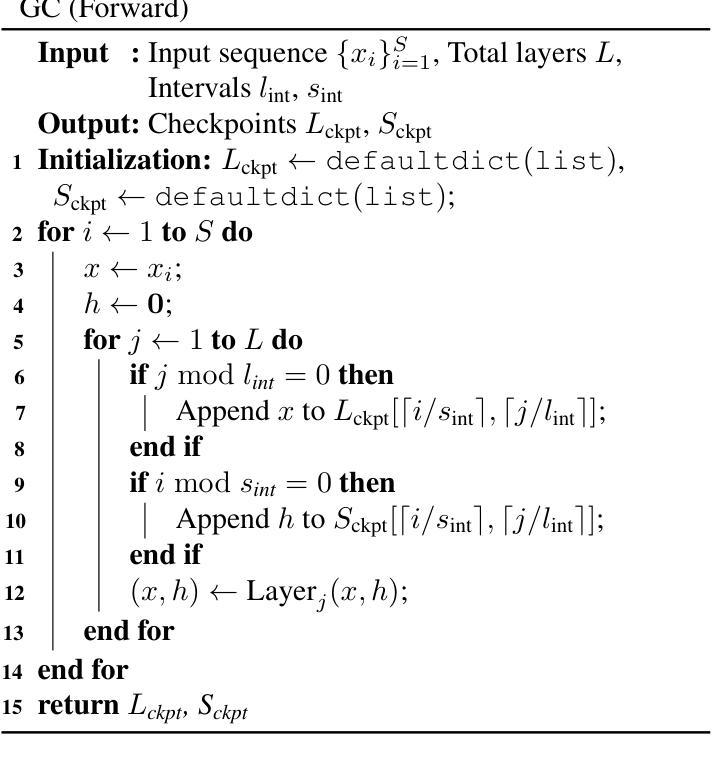
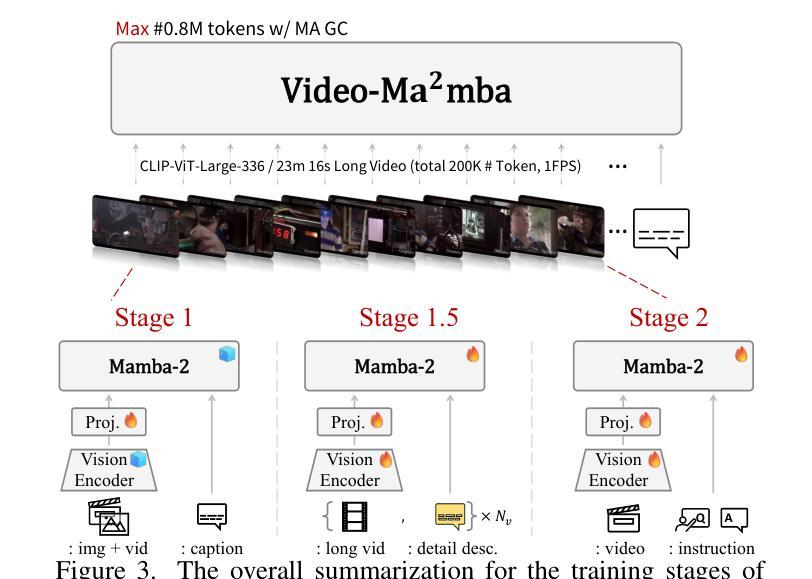
ObjectRelator: Enabling Cross-View Object Relation Understanding in Ego-Centric and Exo-Centric Videos
Authors:Yuqian Fu, Runze Wang, Yanwei Fu, Danda Pani Paudel, Xuanjing Huang, Luc Van Gool
In this paper, we focus on the Ego-Exo Object Correspondence task, an emerging challenge in the field of computer vision that aims to map objects across ego-centric and exo-centric views. We introduce ObjectRelator, a novel method designed to tackle this task, featuring two new modules: Multimodal Condition Fusion (MCFuse) and SSL-based Cross-View Object Alignment (XObjAlign). MCFuse effectively fuses language and visual conditions to enhance target object localization, while XObjAlign enforces consistency in object representations across views through a self-supervised alignment strategy. Extensive experiments demonstrate the effectiveness of ObjectRelator, achieving state-of-the-art performance on Ego2Exo and Exo2Ego tasks with minimal additional parameters. This work provides a foundation for future research in comprehensive cross-view object relation understanding highlighting the potential of leveraging multimodal guidance and cross-view alignment. Codes and models will be released to advance further research in this direction.
本文重点研究Ego-Exo对象对应关系任务,这是计算机视觉领域的新兴挑战,旨在实现跨自我中心视角和外在视角的对象映射。我们提出了ObjectRelator这一新方法来解决这一任务,其包含两个新模块:多模态条件融合(MCFuse)和基于SSL的跨视图对象对齐(XObjAlign)。MCFuse有效地融合了语言和视觉条件,增强了目标对象的定位,而XObjAlign则通过自我监督的对齐策略,实现了跨视图对象表示的一致性。大量实验证明了ObjectRelator的有效性,在Ego2Exo和Exo2Ego任务上达到了最新性能,且仅增加了极少量的参数。这项工作为全面跨视图对象关系理解的研究奠定了基础,突出了利用多模态指导和跨视图对齐的潜力。为了推动这一方向的研究进展,我们将发布相关代码和模型。
论文及项目相关链接
Summary
本文聚焦于Ego-Exo对象对应关系任务,这是计算机视觉领域的新兴挑战,旨在映射不同视点下的对象。引入了一种新方法ObjectRelator来解决此任务,包括两个新模块:多模态条件融合(MCFuse)和基于SSL的跨视图对象对齐(XObjAlign)。MCFuse有效地融合了语言和视觉条件,增强了目标对象的定位能力;而XObjAlign则通过一种自监督的对齐策略,确保跨视图的对象表示的一致性。实验证明ObjectRelator的有效性,在Ego2Exo和Exo2Ego任务上达到最新性能水平,并展示了在未来对全面跨视图对象关系理解的潜在贡献。
Key Takeaways
以下是七个关于该文本的关键见解:
- 本文主要讨论Ego-Exo对象对应关系任务,这是一个新兴挑战,旨在映射不同视点下的对象。
- 介绍了一种新方法ObjectRelator来解决这个任务,包括两个新模块:多模态条件融合(MCFuse)和基于SSL的跨视图对象对齐(XObjAlign)。
点此查看论文截图
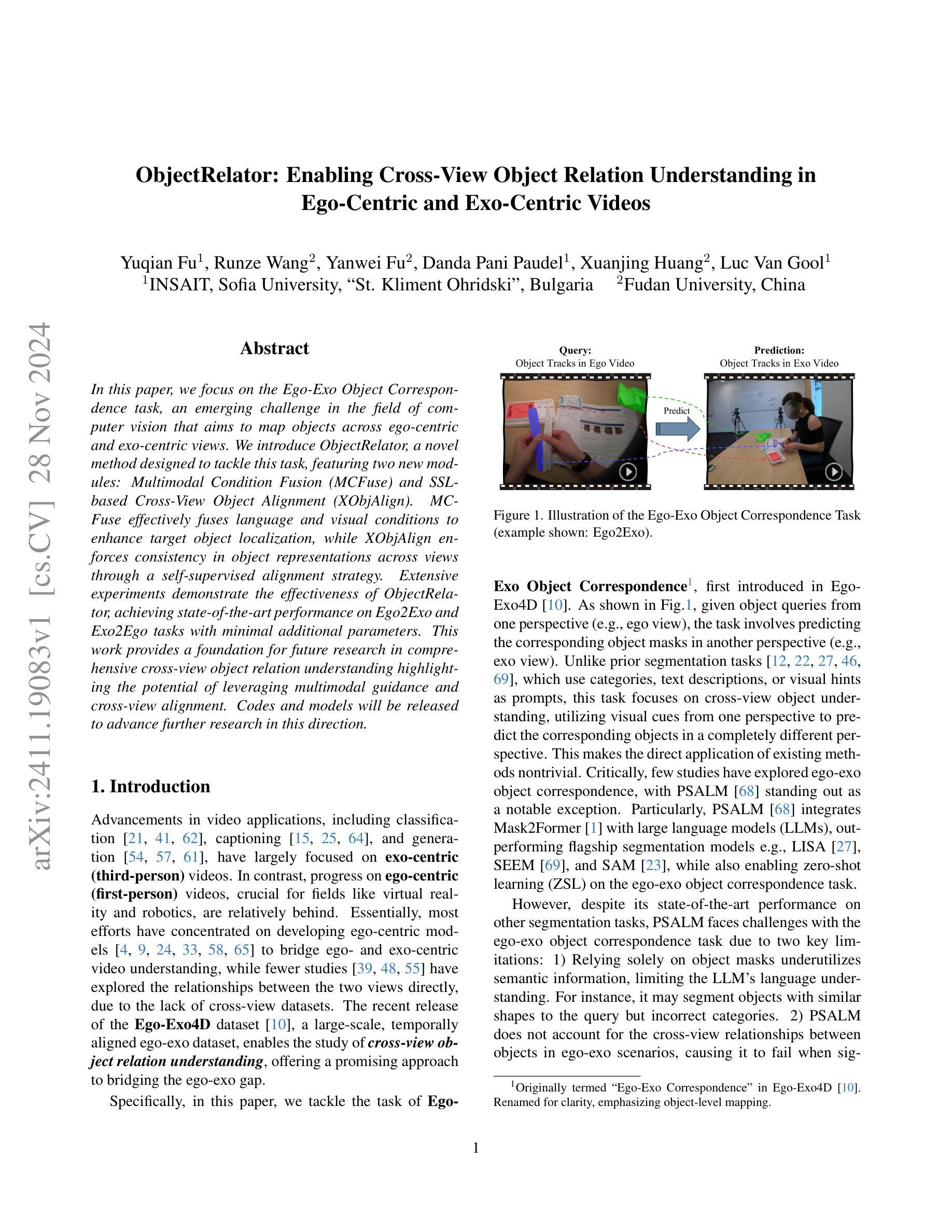

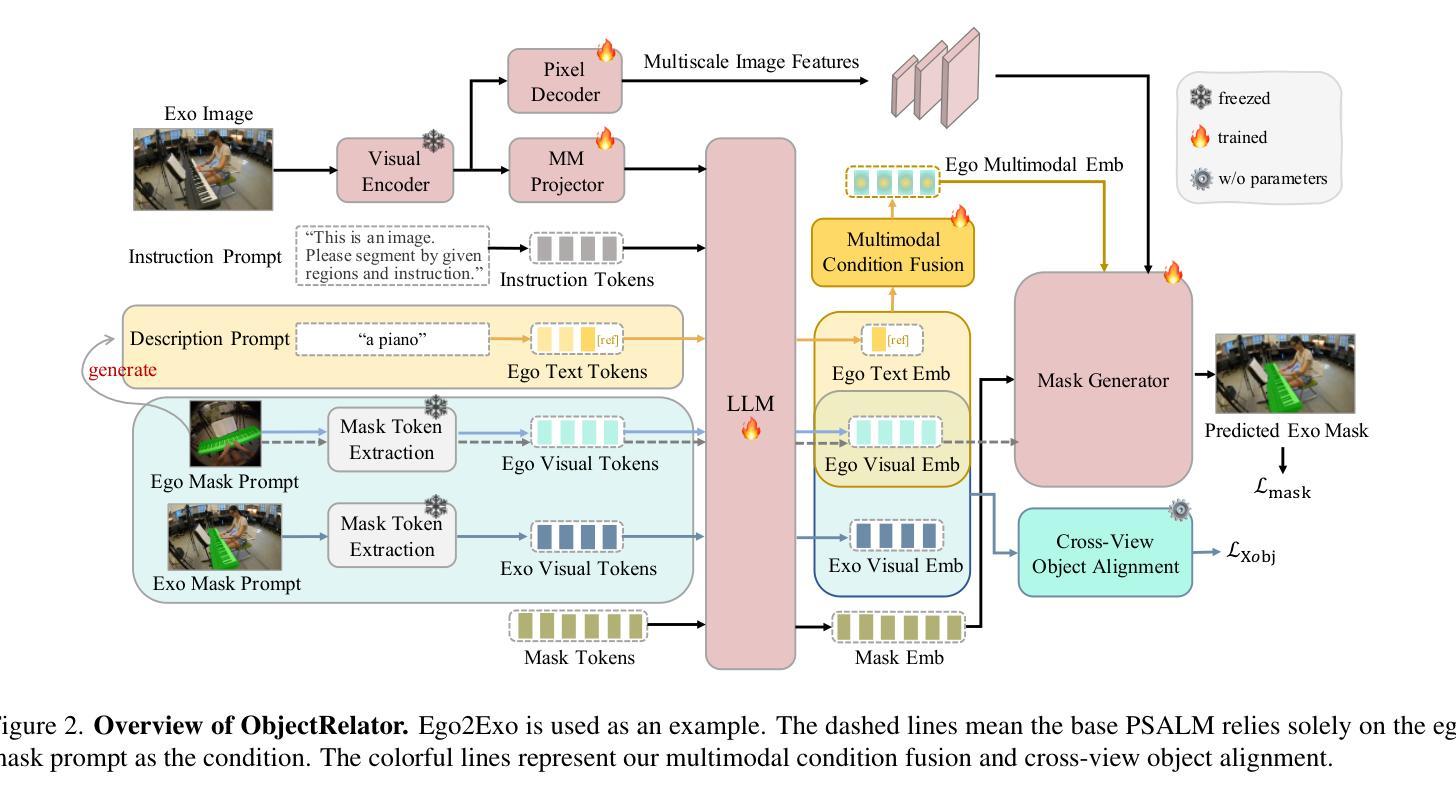
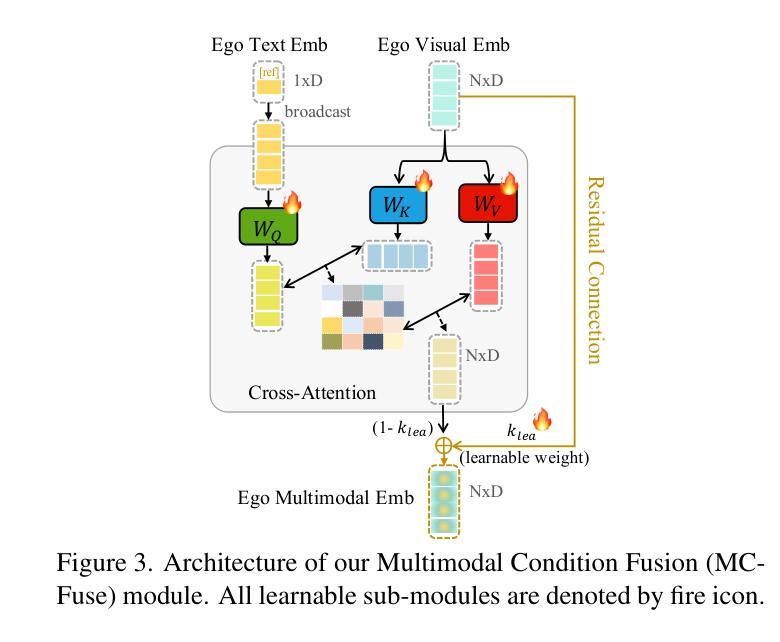
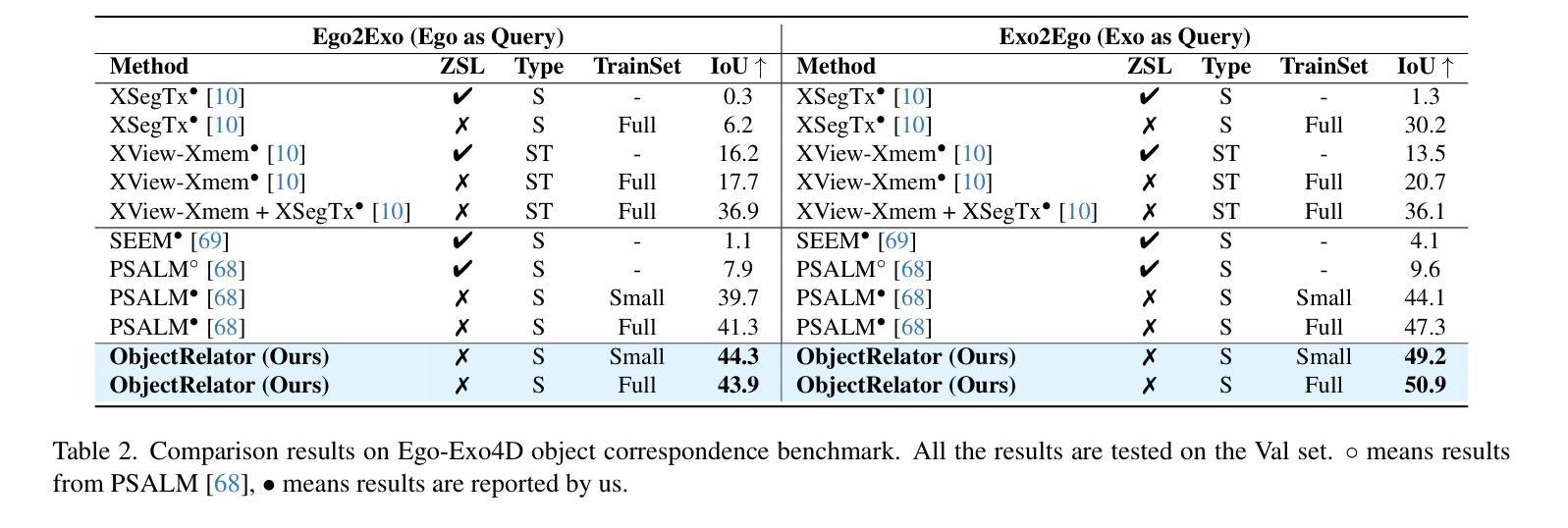
TimeMarker: A Versatile Video-LLM for Long and Short Video Understanding with Superior Temporal Localization Ability
Authors:Shimin Chen, Xiaohan Lan, Yitian Yuan, Zequn Jie, Lin Ma
Rapid development of large language models (LLMs) has significantly advanced multimodal large language models (LMMs), particularly in vision-language tasks. However, existing video-language models often overlook precise temporal localization and struggle with videos of varying lengths. We introduce TimeMarker, a versatile Video-LLM designed for high-quality dialogue based on video content, emphasizing temporal localization. TimeMarker integrates Temporal Separator Tokens to enhance temporal awareness, accurately marking specific moments within videos. It employs the AnyLength mechanism for dynamic frame sampling and adaptive token merging, enabling effective handling of both short and long videos. Additionally, TimeMarker utilizes diverse datasets, including further transformed temporal-related video QA datasets, to bolster its temporal understanding capabilities. Image and interleaved data are also employed to further enhance the model’s semantic perception ability. Evaluations demonstrate that TimeMarker achieves state-of-the-art performance across multiple benchmarks, excelling in both short and long video categories. Our project page is at \url{https://github.com/TimeMarker-LLM/TimeMarker/}.
随着大型语言模型(LLM)的快速发展,多模态大型语言模型(LMM)也取得了重大进展,特别是在视觉语言任务中。然而,现有的视频语言模型往往忽视了精确的时间定位,并且在处理不同长度的视频时面临困难。我们推出了TimeMarker,这是一款基于视频内容的高品质对话通用视频大型语言模型,强调时间定位。TimeMarker集成了时间分隔符令牌,以提高时间意识,准确标记视频中的特定时刻。它采用AnyLength机制进行动态帧采样和自适应令牌合并,能够有效地处理长短视频。此外,TimeMarker利用多样化的数据集,包括经过进一步时间变换的视频问答数据集,以增强其时间理解能力。图像和交错数据也被用来进一步提高模型的语义感知能力。评估表明,TimeMarker在多个基准测试中达到了最先进的性能,在长短视频类别中都表现出色。我们的项目页面是\url{https://github.com/TimeMarker-LLM/TimeMarker/}。
论文及项目相关链接
Summary
大型语言模型的快速发展推动了多模态语言模型在视觉语言任务上的进步,但现有视频语言模型在精确时间定位和不同长度视频的应对上存在局限。我们推出TimeMarker,一款针对视频内容的对话进行设计的多功能视频大型语言模型,强调时间定位的重要性。TimeMarker通过引入时间分隔符令牌增强时间感知,准确标记视频中的特定时刻。它采用任意长度机制进行动态帧采样和自适应令牌合并,有效处理长短不一的视频。此外,TimeMarker利用多样化的数据集,包括经过时间转化的视频问答数据集,增强时间理解的能力。评估和实验表明,TimeMarker在多个基准测试中达到领先水平,特别是在短长和长视频类别中表现出色。
Key Takeaways
- 大型语言模型的发展推动了多模态语言模型在视觉语言任务上的进步。
- 现有视频语言模型在时间定位上存在问题,无法很好地处理不同长度的视频。
- TimeMarker是一款针对视频内容的对话设计的多功能视频大型语言模型,强调时间定位的重要性。
- TimeMarker通过引入时间分隔符令牌和任意长度机制来处理长短不一的视频。
- TimeMarker利用多样化的数据集增强时间理解能力。
- TimeMarker采用图像和交错数据来进一步增强模型的语义感知能力。
- TimeMarker在多个基准测试中表现优秀,特别是在短长和长视频类别中表现突出。
点此查看论文截图
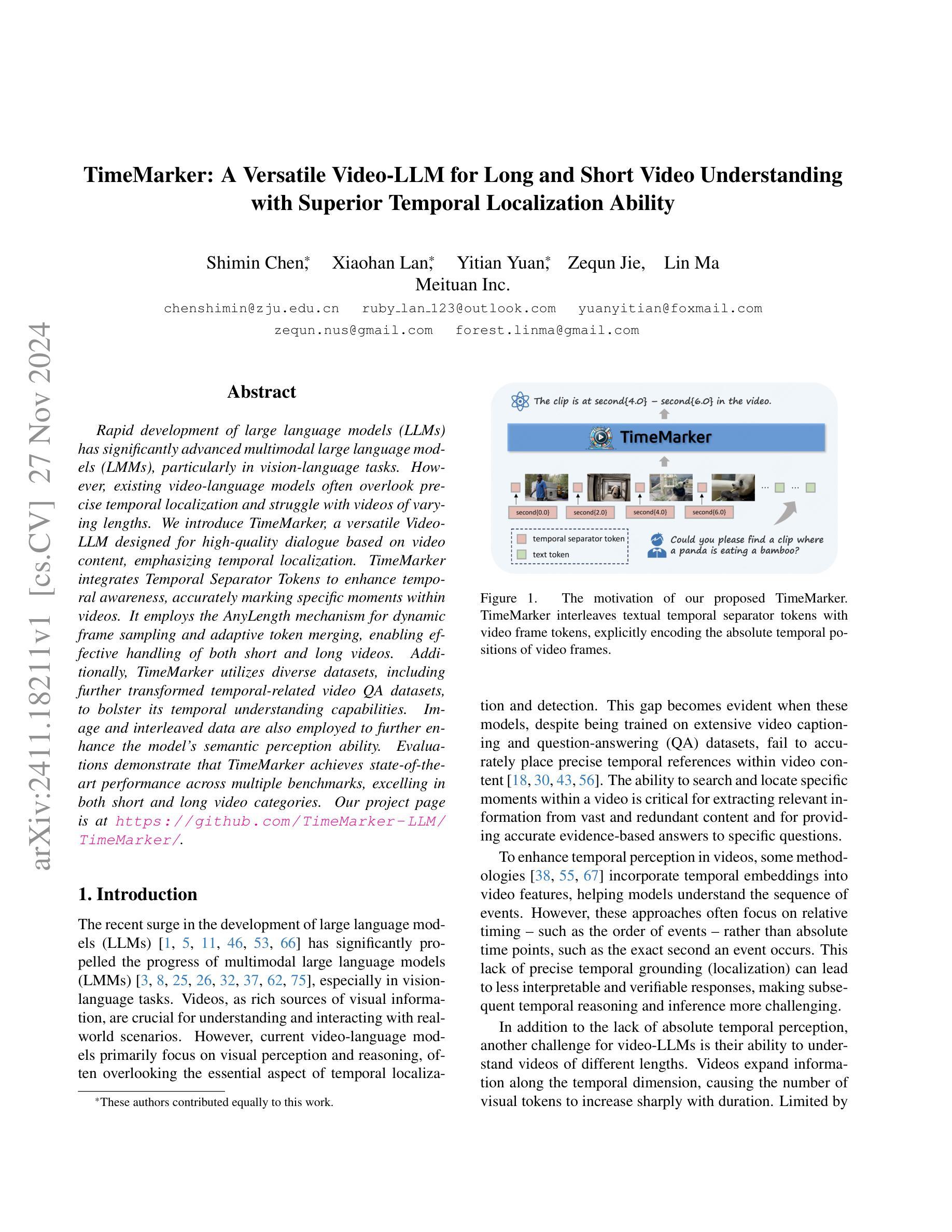
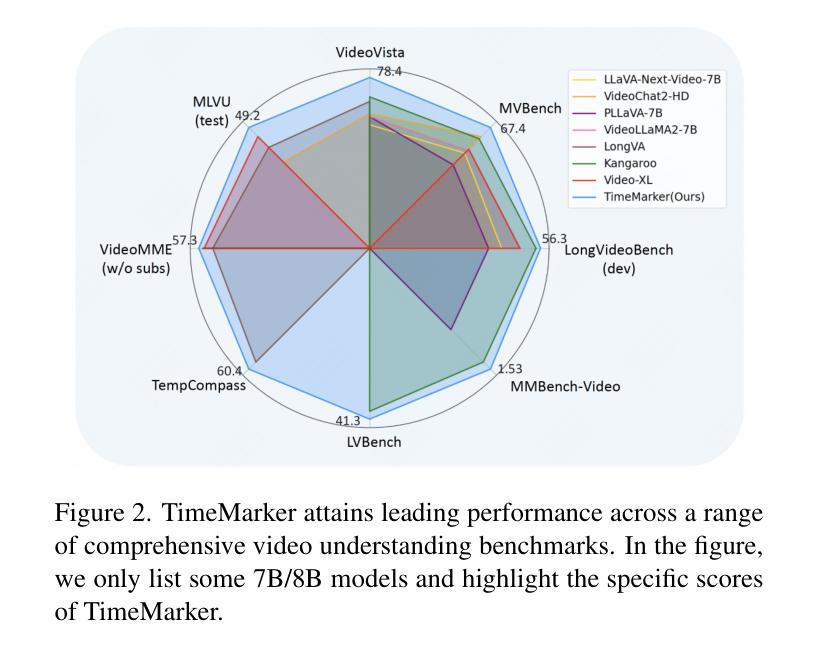
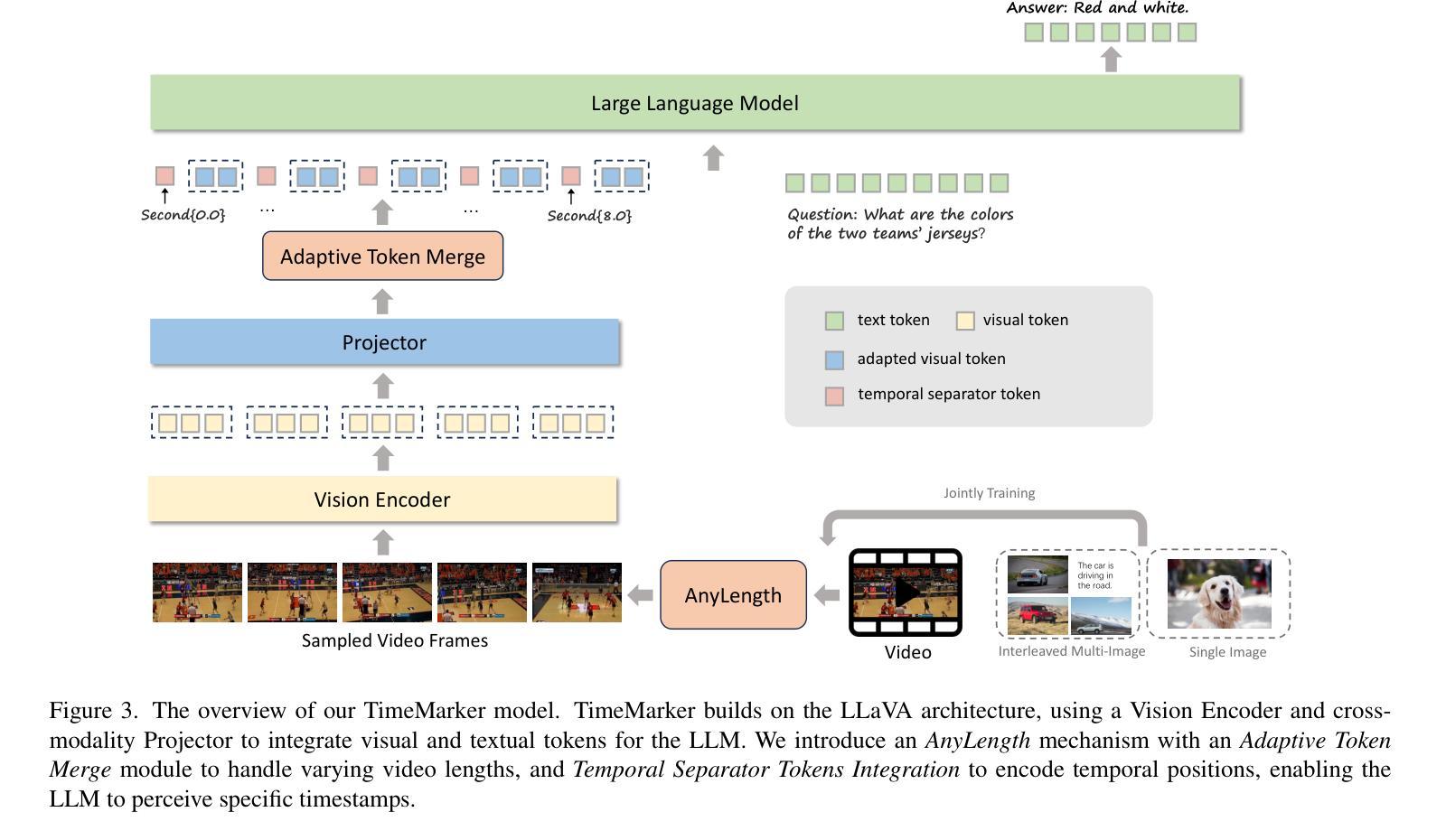
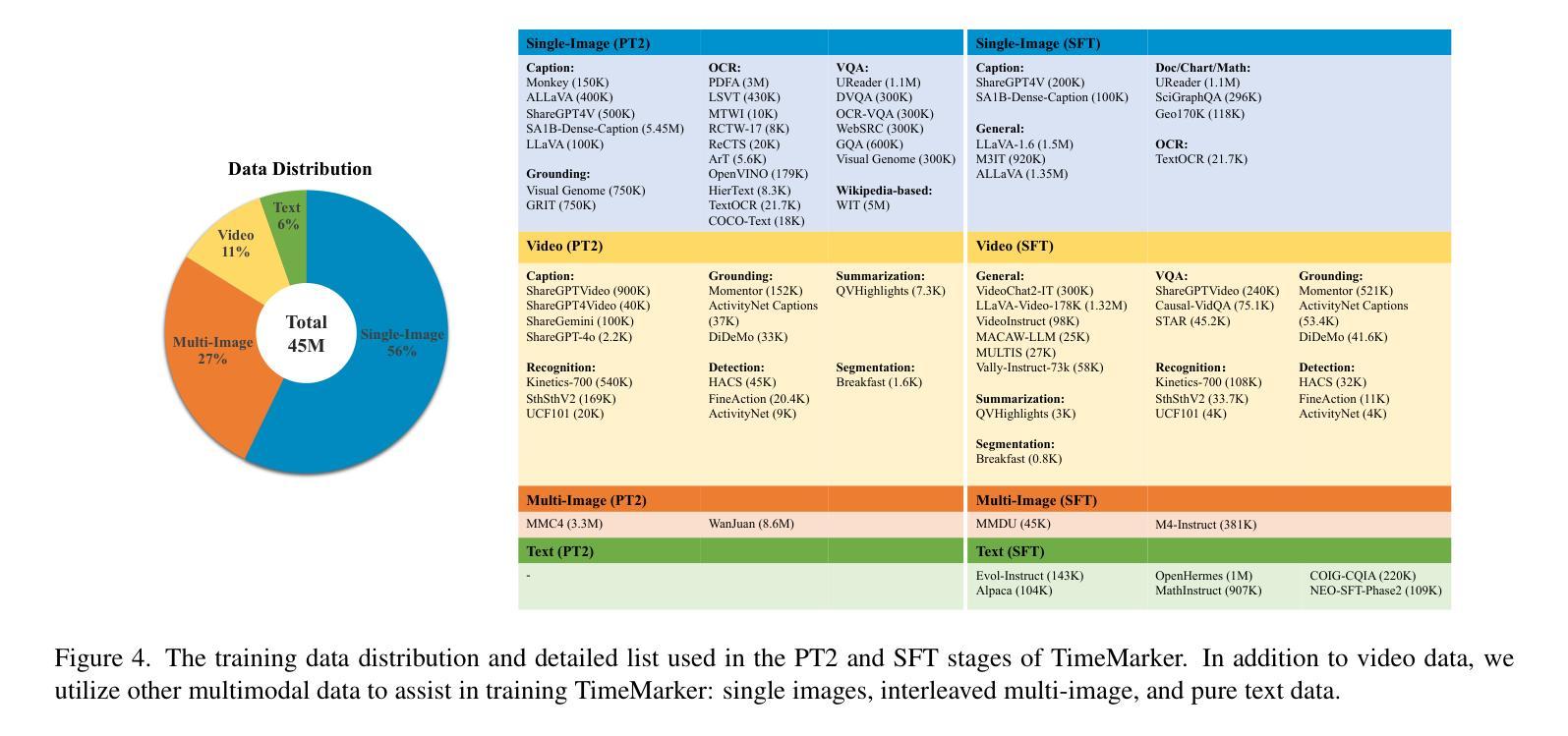
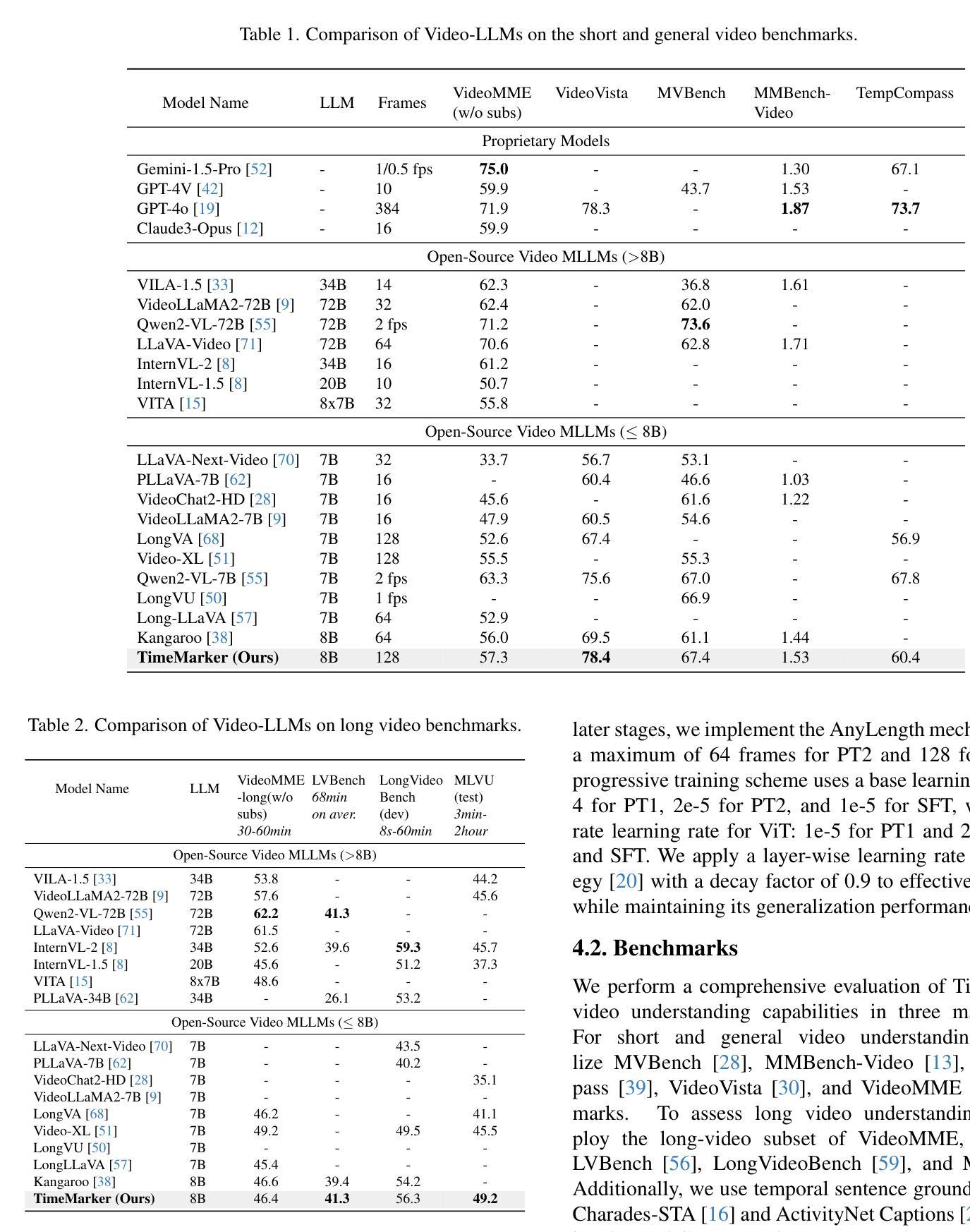
SAVEn-Vid: Synergistic Audio-Visual Integration for Enhanced Understanding in Long Video Context
Authors:Jungang Li, Sicheng Tao, Yibo Yan, Xiaojie Gu, Haodong Xu, Xu Zheng, Yuanhuiyi Lyu, Linfeng Zhang, Xuming Hu
Endeavors have been made to explore Large Language Models for video analysis (Video-LLMs), particularly in understanding and interpreting long videos. However, existing Video-LLMs still face challenges in effectively integrating the rich and diverse audio-visual information inherent in long videos, which is crucial for comprehensive understanding. This raises the question: how can we leverage embedded audio-visual information to enhance long video understanding? Therefore, (i) we introduce SAVEn-Vid, the first-ever long audio-visual video dataset comprising over 58k audio-visual instructions. (ii) From the model perspective, we propose a time-aware Audio-Visual Large Language Model (AV-LLM), SAVEnVideo, fine-tuned on SAVEn-Vid. (iii) Besides, we present AVBench, a benchmark containing 2,500 QAs designed to evaluate models on enhanced audio-visual comprehension tasks within long video, challenging their ability to handle intricate audio-visual interactions. Experiments on AVBench reveal the limitations of current AV-LLMs. Experiments also demonstrate that SAVEnVideo outperforms the best Video-LLM by 3.61% on the zero-shot long video task (Video-MME) and surpasses the leading audio-visual LLM by 1.29% on the zero-shot audio-visual task (Music-AVQA). Consequently, at the 7B parameter scale, SAVEnVideo can achieve state-of-the-art performance. Our dataset and code will be released at https://ljungang.github.io/SAVEn-Vid/ upon acceptance.
目前正努力探索用于视频分析的大型语言模型(Video-LLMs),特别是在理解和解释长视频方面。然而,现有的Video-LLMs仍然面临挑战,即如何有效地整合长视频中丰富的各种视听信息,这对于全面理解至关重要。这引发了以下问题:我们如何利用嵌入的视听信息来提高长视频的理解能力?因此,(i)我们推出了SAVEn-Vid,这是首个包含超过58,000条视听指令的长时间视听视频数据集。(ii)从模型角度来看,我们提出了时间感知视听大型语言模型(AV-LLM),即SAVEnVideo,并在SAVEn-Vid上进行微调。(iii)此外,我们还推出了AVBench,这是一个包含2500个问答的基准测试,旨在评估模型在处理长视频中的增强视听理解任务的能力,挑战它们处理复杂视听交互的能力。在AVBench上的实验揭示了当前AV-LLMs的局限性。实验还表明,在零样本长视频任务(Video-MME)上,SAVEnVideo的表现优于最佳Video-LLM达3.61%,在零样本视听任务(Music-AVQA)上,SAVEnVideo的表现也超过了领先的视听LLM达1.29%。因此,在7B参数规模下,SAVEnVideo可以达到最先进的性能。我们的数据集和代码将在https://ljungang.github.io/SAVEn-Vid/上发布,待审核通过后即可使用。
论文及项目相关链接
Summary
该研究探索了大型语言模型在视频分析中的应用,特别是针对长视频的理解和解释。研究面临如何有效整合长视频中的丰富视听信息的挑战。为此,研究者推出了SAVEn-Vid数据集和SAVEnVideo时间感知视听大型语言模型,并设立了AVBench基准测试。实验表明,SAVEnVideo在零样本长视频任务上表现出最佳性能,超越了现有视听语言模型。
Key Takeaways
- 大型语言模型在视频分析中的应用正在受到关注,特别是在理解和解释长视频方面。
- 现有大型语言模型在整合长视频的丰富视听信息方面面临挑战。
- SAVEn-Vid数据集的推出,旨在解决这一挑战,包含超过5.8万的视听指令。
- SAVEnVideo是一种时间感知的视听大型语言模型,经过SAVEn-Vid数据集的微调。
- AVBench基准测试的设立,旨在评估模型在处理长视频中的复杂视听交互任务的能力。
- 实验结果表明,SAVEnVideo在零样本长视频任务和视听任务上均表现出最佳性能,超越了其他大型语言模型。
点此查看论文截图
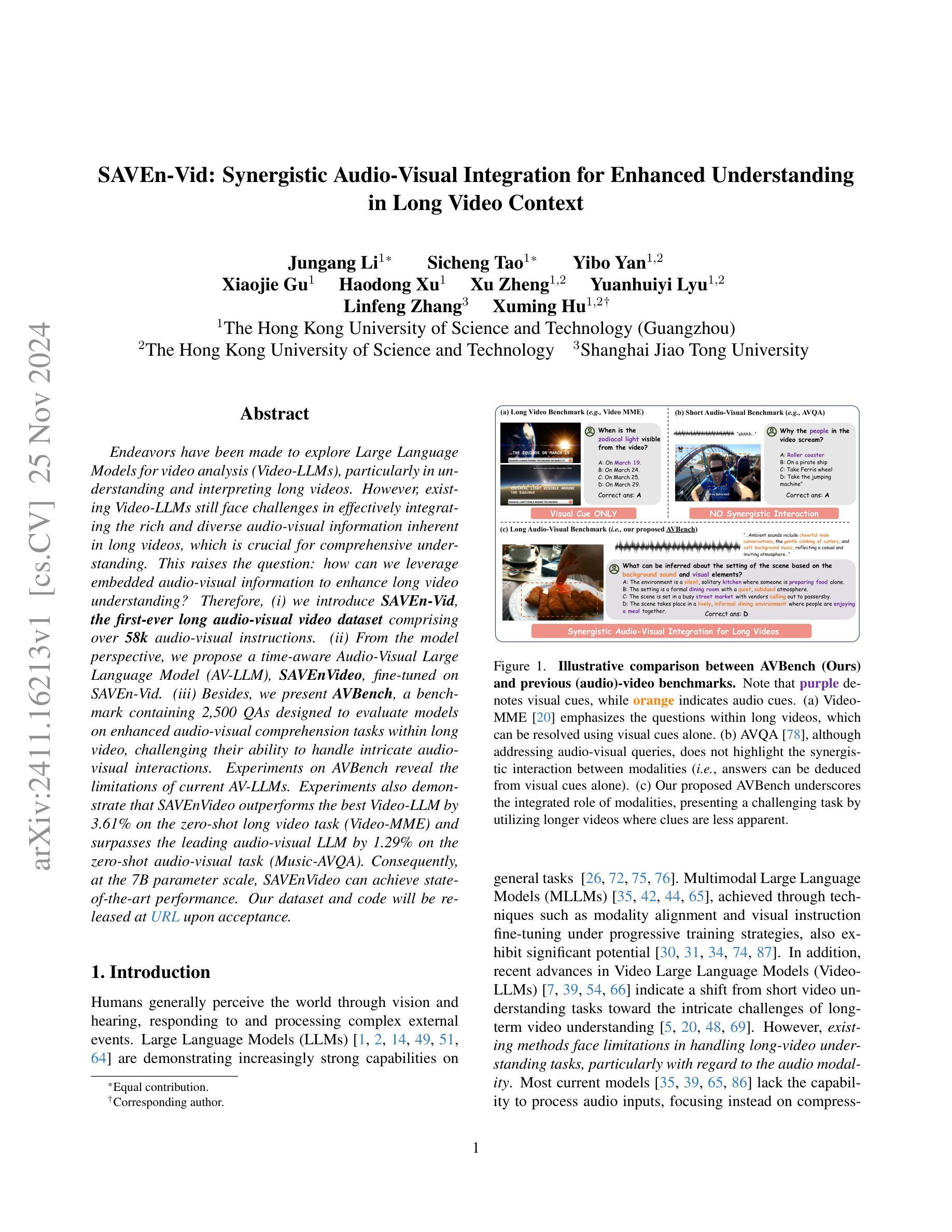
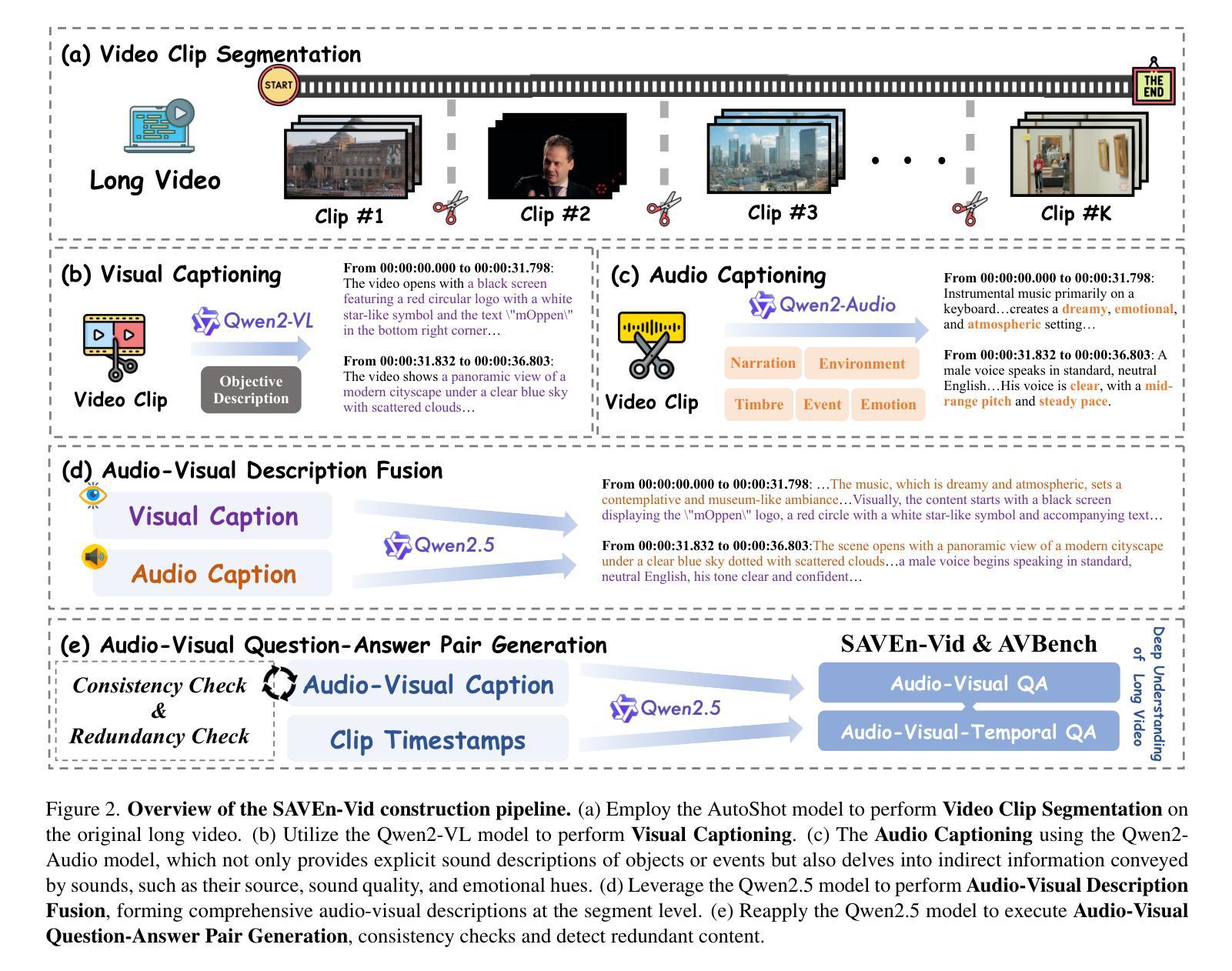
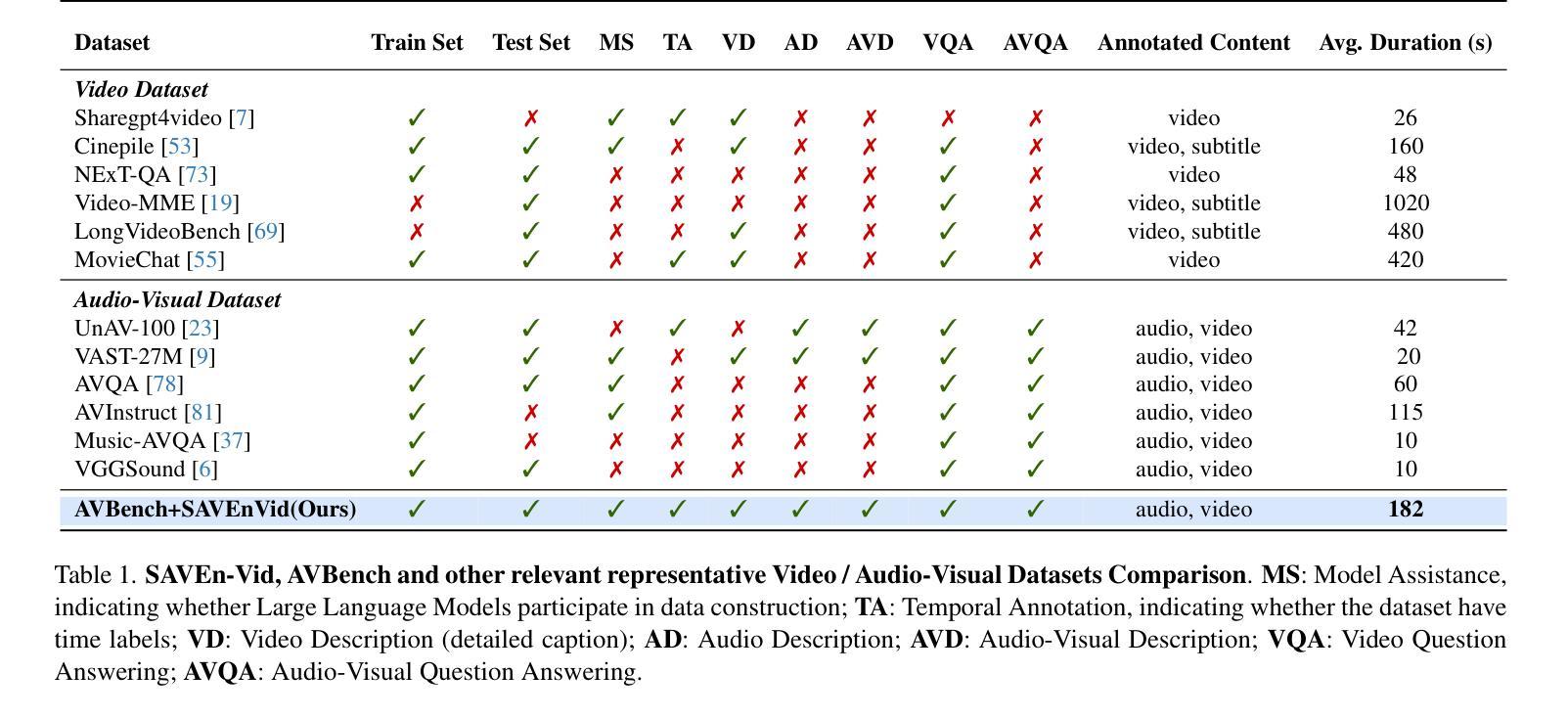
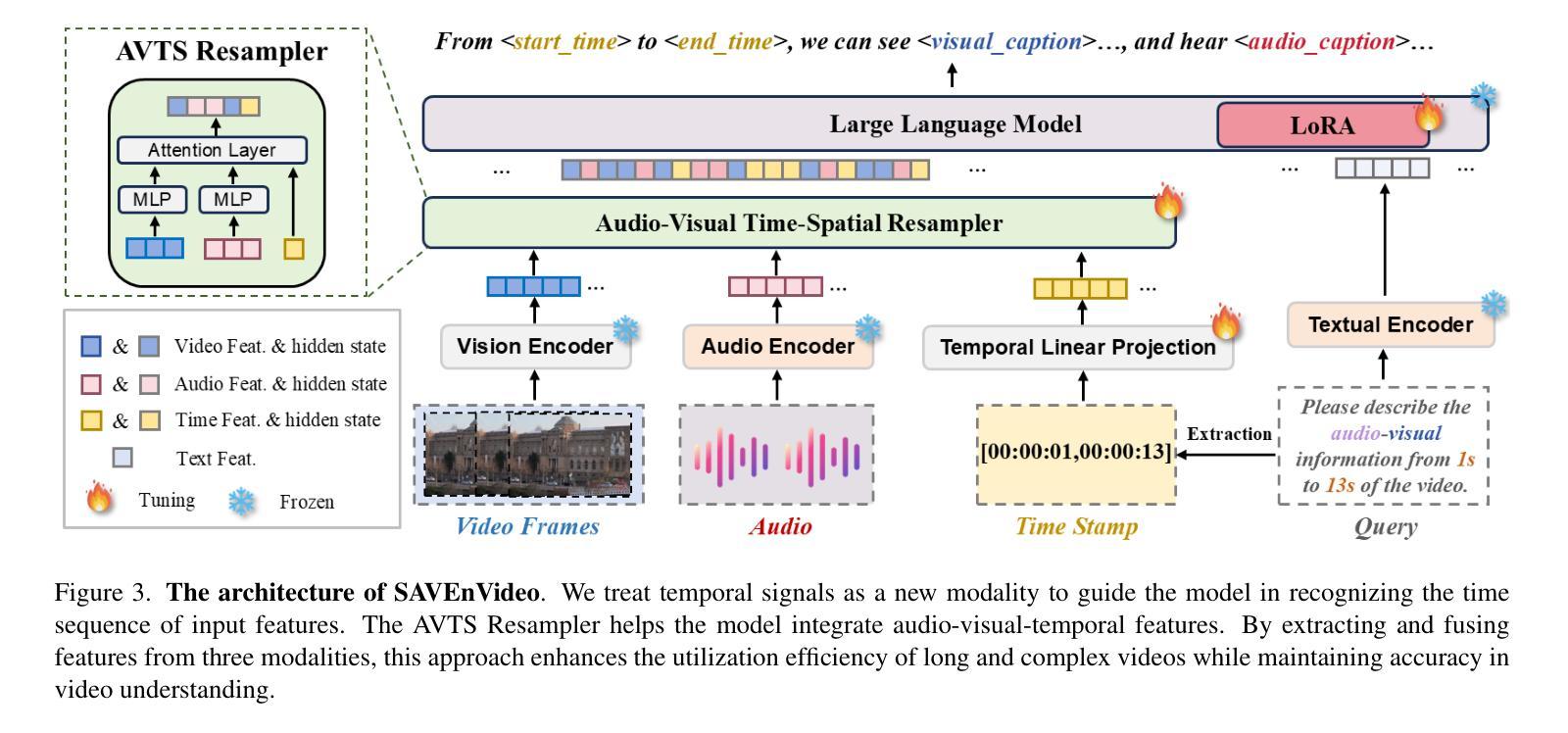
ReWind: Understanding Long Videos with Instructed Learnable Memory
Authors:Anxhelo Diko, Tinghuai Wang, Wassim Swaileh, Shiyan Sun, Ioannis Patras
Vision-Language Models (VLMs) are crucial for applications requiring integrated understanding textual and visual information. However, existing VLMs struggle with long videos due to computational inefficiency, memory limitations, and difficulties in maintaining coherent understanding across extended sequences. To address these challenges, we introduce ReWind, a novel memory-based VLM designed for efficient long video understanding while preserving temporal fidelity. ReWind operates in a two-stage framework. In the first stage, ReWind maintains a dynamic learnable memory module with a novel \textbf{read-perceive-write} cycle that stores and updates instruction-relevant visual information as the video unfolds. This module utilizes learnable queries and cross-attentions between memory contents and the input stream, ensuring low memory requirements by scaling linearly with the number of tokens. In the second stage, we propose an adaptive frame selection mechanism guided by the memory content to identify instruction-relevant key moments. It enriches the memory representations with detailed spatial information by selecting a few high-resolution frames, which are then combined with the memory contents and fed into a Large Language Model (LLM) to generate the final answer. We empirically demonstrate ReWind’s superior performance in visual question answering (VQA) and temporal grounding tasks, surpassing previous methods on long video benchmarks. Notably, ReWind achieves a +13% score gain and a +12% accuracy improvement on the MovieChat-1K VQA dataset and an +8% mIoU increase on Charades-STA for temporal grounding.
视觉语言模型(VLMs)对于需要综合理解文本和视觉信息的应用至关重要。然而,由于计算效率低下、内存限制以及维持扩展序列中连贯理解的困难,现有的VLMs在处理长视频时面临挑战。为了应对这些挑战,我们引入了Rewind,这是一种基于新型记忆设计的VLM,旨在实现高效的长视频理解,同时保持时间保真度。Rewind采用两阶段框架进行操作。在第一阶段,Rewind维护一个动态的可学习记忆模块,该模块具有新颖的“读-感知-写”循环,随着视频的展开,存储并更新与指令相关的视觉信息。该模块利用可学习查询和内存内容与输入流之间的交叉注意力,通过令牌数量进行线性缩放,确保低内存要求。在第二阶段,我们提出了一种由内存内容引导的自适应帧选择机制,以识别与指令相关的关键时刻。它通过选择一些高分辨率帧来丰富内存表示中的详细空间信息,然后将这些帧与内存内容相结合并输入大型语言模型(LLM)中,以生成最终答案。我们在视觉问答(VQA)和时间定位任务中进行了实证演示,Rewind在长风视频基准测试上的性能超越了以前的方法。值得注意的是,Rewind在MovieChat-1K VQA数据集上的得分提高了+13%,准确率提高了+12%,在时间定位任务Charades-STA上的mIoU提高了+8%。
论文及项目相关链接
Summary
ReWind是一种基于记忆的视频语言模型(VLM),旨在实现高效的长视频理解并保留时间真实性。它采用两阶段框架,通过动态学习记忆模块和自适应帧选择机制来处理长视频。该模型能够在视频展开时存储和更新与指令相关的视觉信息,并利用可学习的查询和内存内容与输入流之间的交叉注意力来确保低内存要求。自适应帧选择机制能够识别与指令相关的关键时刻,丰富内存表示并生成最终答案。ReWind在视觉问答(VQA)和时间定位任务中表现出卓越性能,在长视频基准测试中超越了以前的方法。
Key Takeaways
- ReWind是一个针对长视频理解的基于记忆的视频语言模型(VLM)。
- ReWind采用两阶段框架处理长视频,包括动态学习记忆模块和自适应帧选择机制。
- 动态学习记忆模块通过“读-感知-写”循环存储和更新与指令相关的视觉信息。
- 该模型利用可学习的查询和内存内容与输入流之间的交叉注意力来确保低内存要求。
- 自适应帧选择机制能够识别与指令相关的关键时刻,并丰富内存表示。
- ReWind在视觉问答(VQA)和时间定位任务中表现出卓越性能。
点此查看论文截图
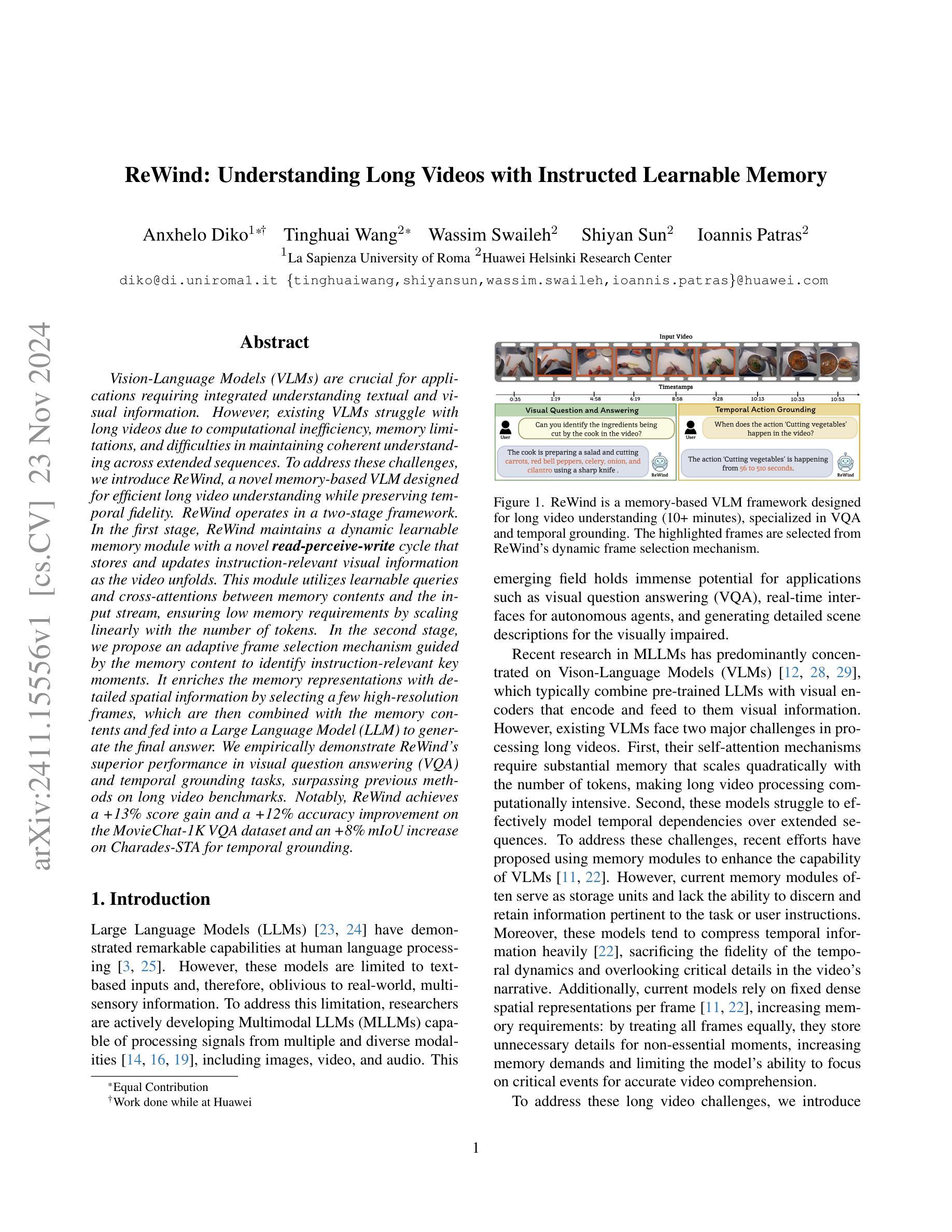
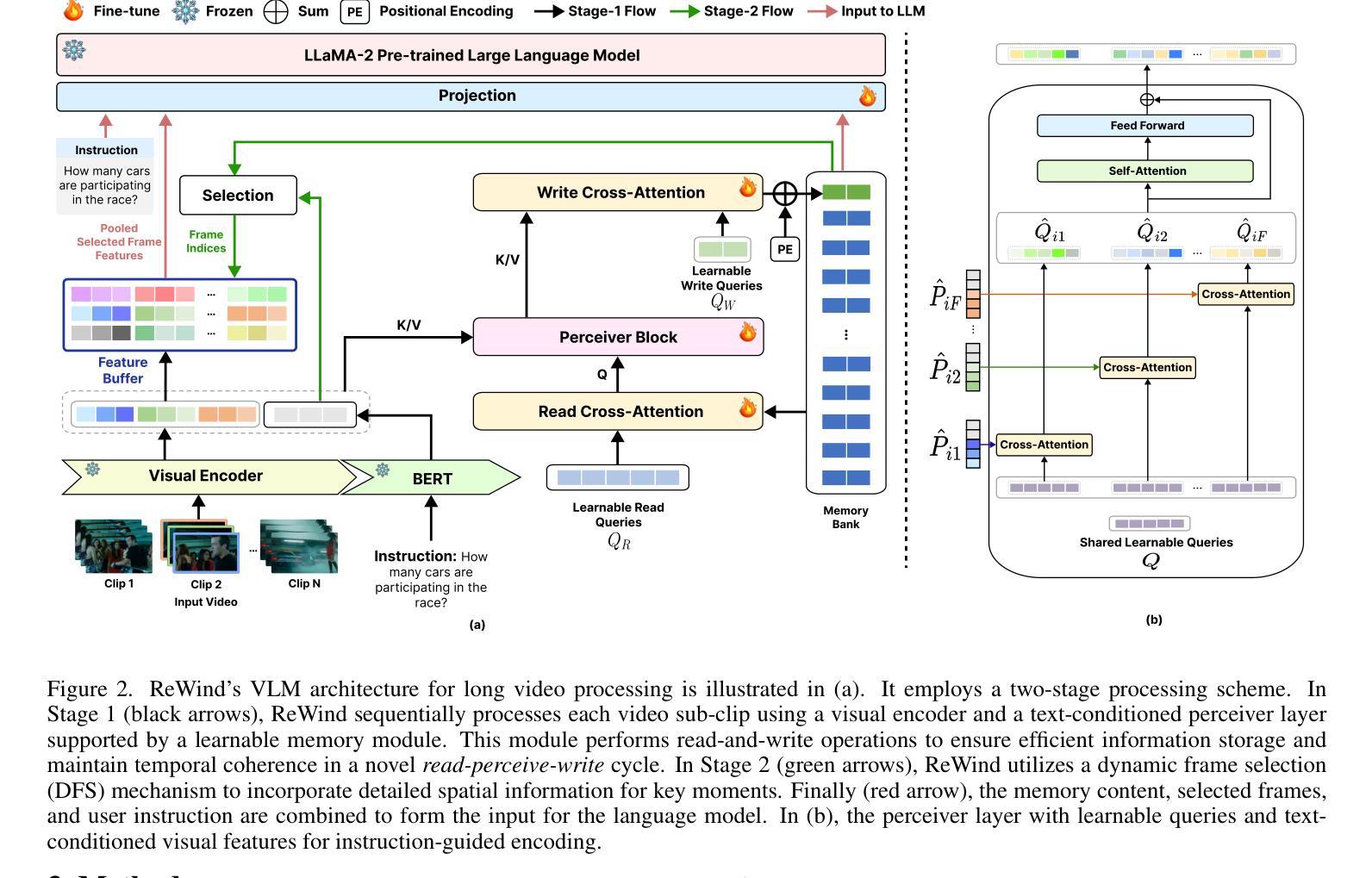
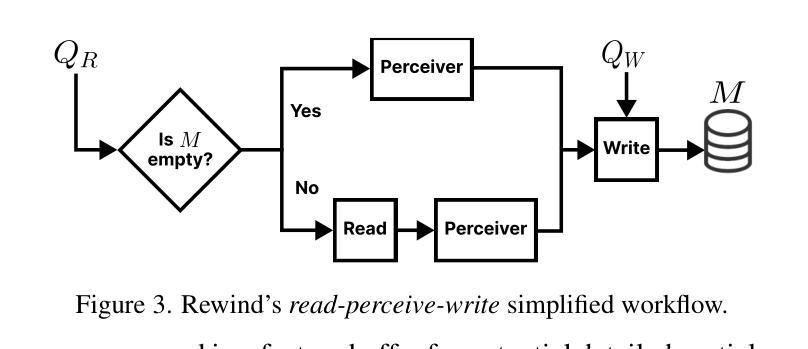
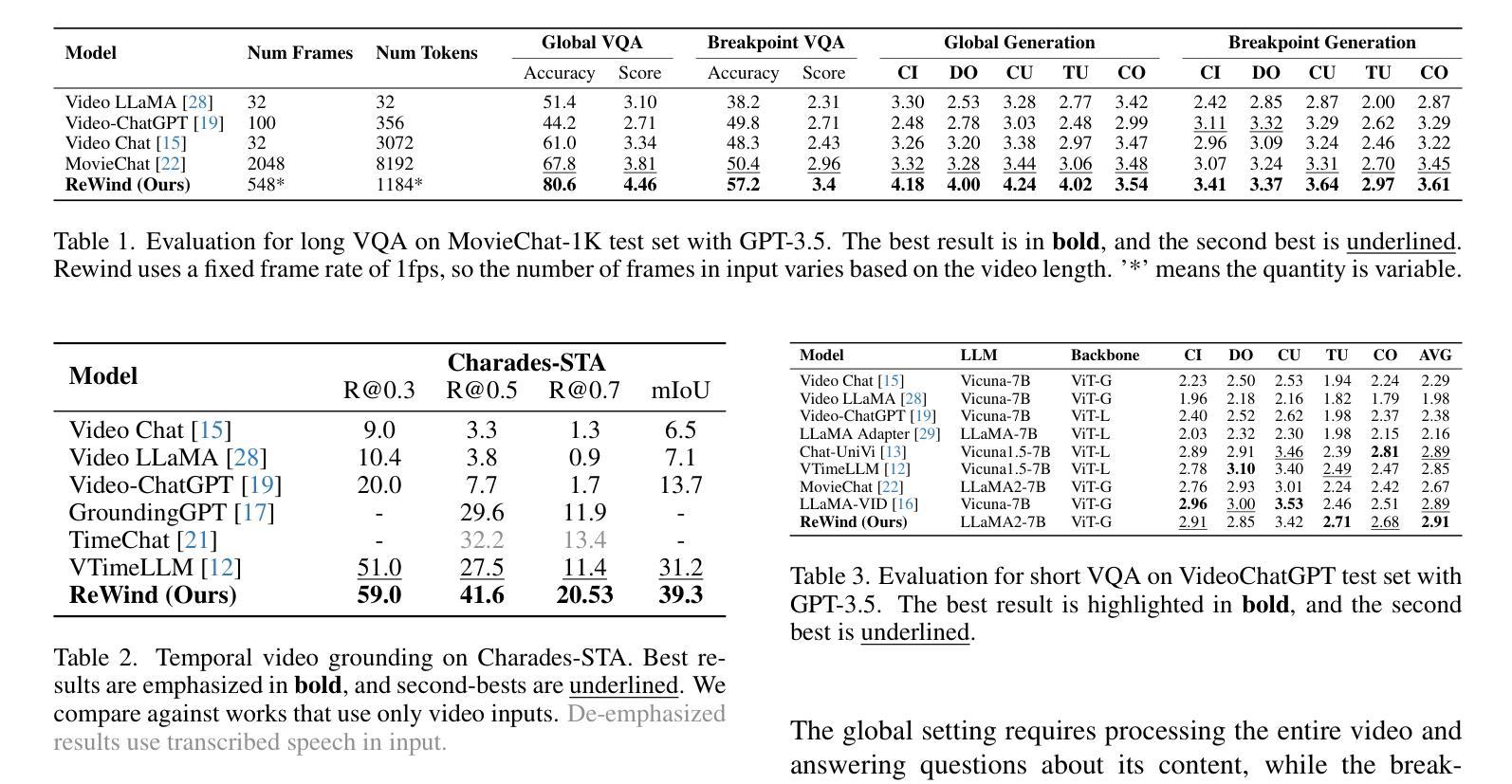
Beyond Training: Dynamic Token Merging for Zero-Shot Video Understanding
Authors:Yiming Zhang, Zhuokai Zhao, Zhaorun Chen, Zenghui Ding, Xianjun Yang, Yining Sun
Recent advancements in multimodal large language models (MLLMs) have opened new avenues for video understanding. However, achieving high fidelity in zero-shot video tasks remains challenging. Traditional video processing methods rely heavily on fine-tuning to capture nuanced spatial-temporal details, which incurs significant data and computation costs. In contrast, training-free approaches, though efficient, often lack robustness in preserving context-rich features across complex video content. To this end, we propose DYTO, a novel dynamic token merging framework for zero-shot video understanding that adaptively optimizes token efficiency while preserving crucial scene details. DYTO integrates a hierarchical frame selection and a bipartite token merging strategy to dynamically cluster key frames and selectively compress token sequences, striking a balance between computational efficiency with semantic richness. Extensive experiments across multiple benchmarks demonstrate the effectiveness of DYTO, achieving superior performance compared to both fine-tuned and training-free methods and setting a new state-of-the-art for zero-shot video understanding.
近期多模态大型语言模型(MLLMs)的进展为视频理解开辟了新的途径。然而,在零样本视频任务中实现高保真仍然具有挑战性。传统视频处理方法依赖于微调来捕捉微妙的时空细节,这产生了大量的数据和计算成本。相比之下,无训练的方法虽然效率高,但在保留复杂视频内容的丰富上下文特征方面往往缺乏稳健性。为此,我们提出了DYTO,这是一种用于零样本视频理解的新型动态令牌合并框架,它可以在保留关键场景细节的同时自适应地优化令牌效率。DYTO结合了分层帧选择和二分令牌合并策略,以动态聚类关键帧并选择性压缩令牌序列,在计算效率和语义丰富性之间达到平衡。在多个基准测试上的广泛实验证明了DYTO的有效性,与微调和无训练方法相比,它实现了卓越的性能,并为零样本视频理解设定了新的最新技术。
论文及项目相关链接
Summary
视频理解领域的新进展中,动态令牌合并框架DYTO能有效解决零样本视频任务的高保真度挑战。DYTO通过分层帧选择和二分令牌合并策略,实现关键帧的动态聚类和令牌序列的选择性压缩,平衡计算效率和语义丰富性。在多个基准测试中表现优异,相较于传统微调和无训练方法具有优越性,为零样本视频理解设定了新的技术高点。
Key Takeaways
- 多模态大型语言模型(MLLMs)的进步为视频理解开辟了新途径。
- 零样本视频任务的高保真度实现具有挑战性。
- 传统视频处理方法依赖微调捕捉细微时空细节,但数据计算成本高昂。
- 训练免费的方法虽然高效,但在复杂视频内容中缺乏保持丰富上下文特征的能力。
- DYTO是一个动态令牌合并框架,旨在解决零样本视频理解的挑战。
- DYTO通过分层帧选择和二分令牌合并策略,实现关键帧的动态聚类和令牌序列的选择性压缩。
点此查看论文截图
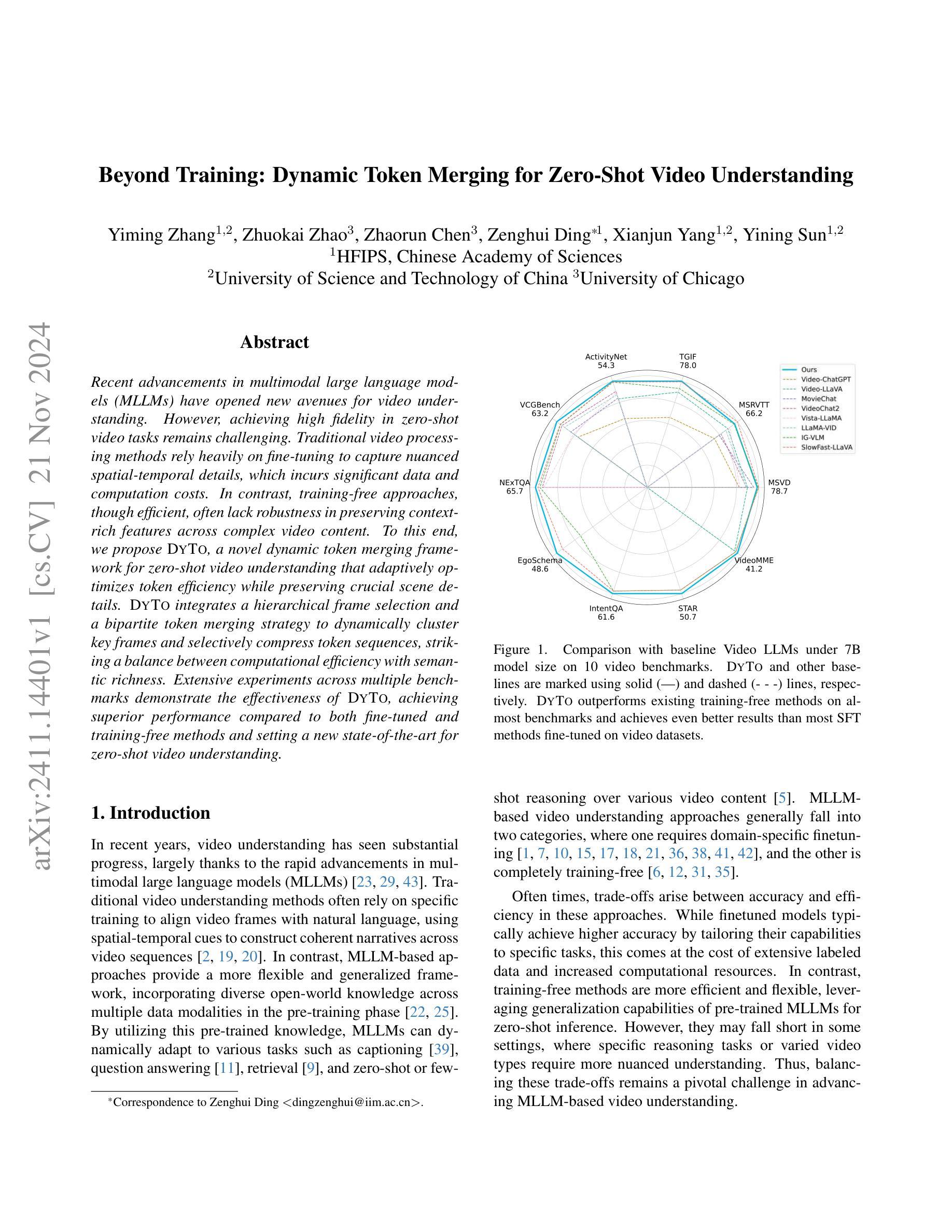
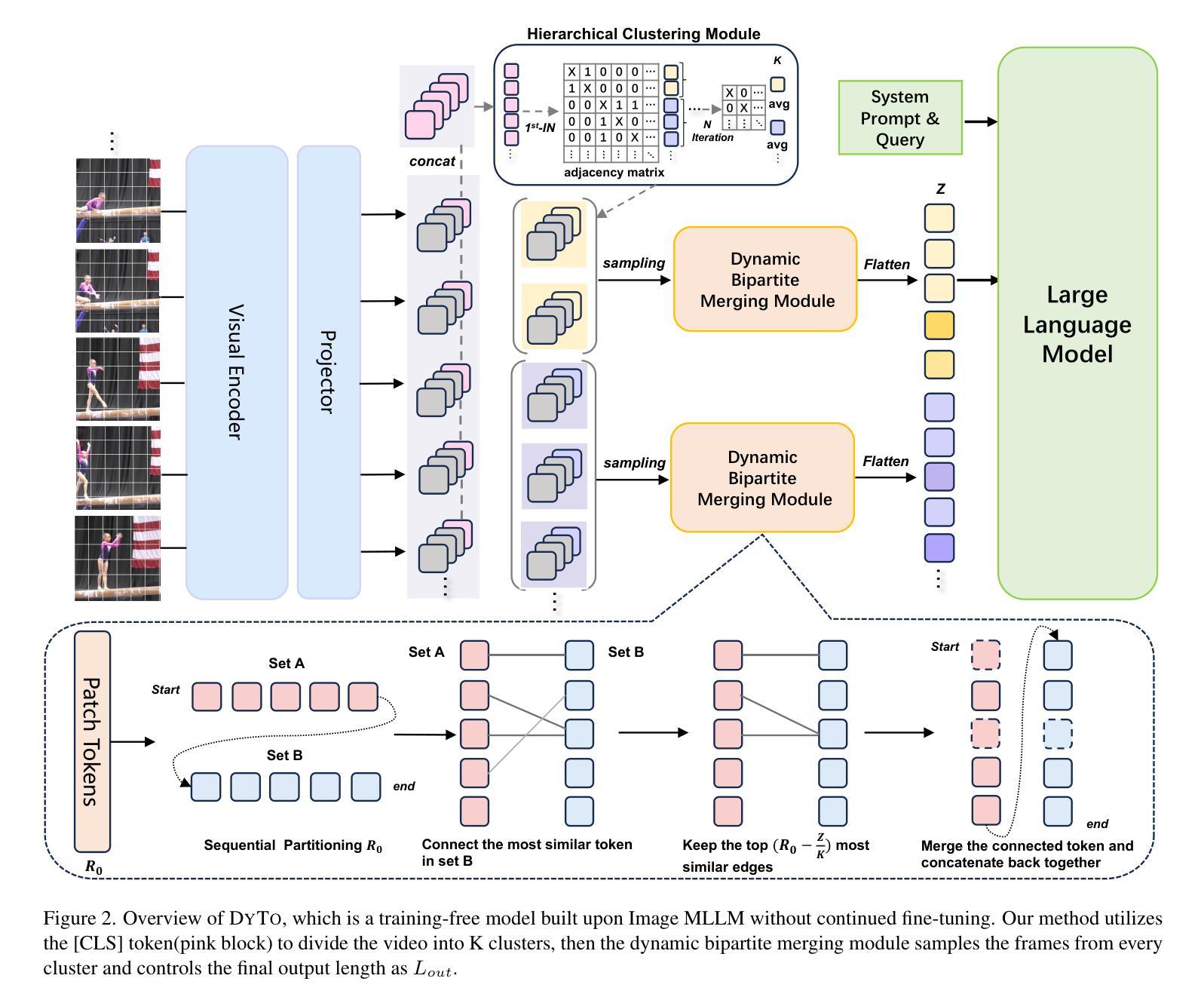
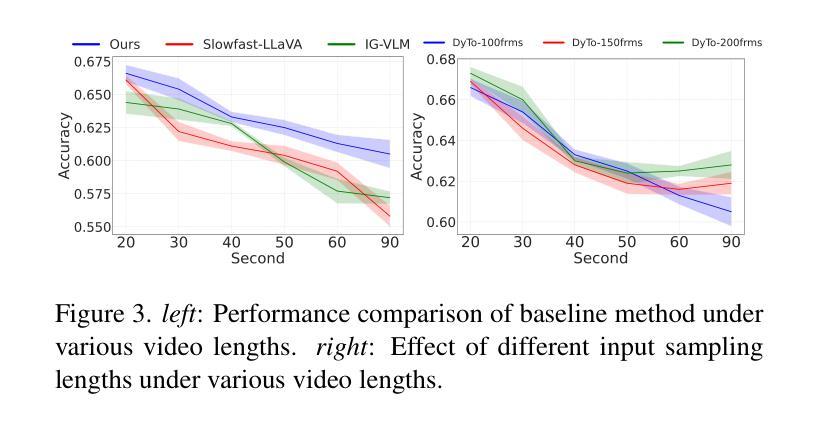
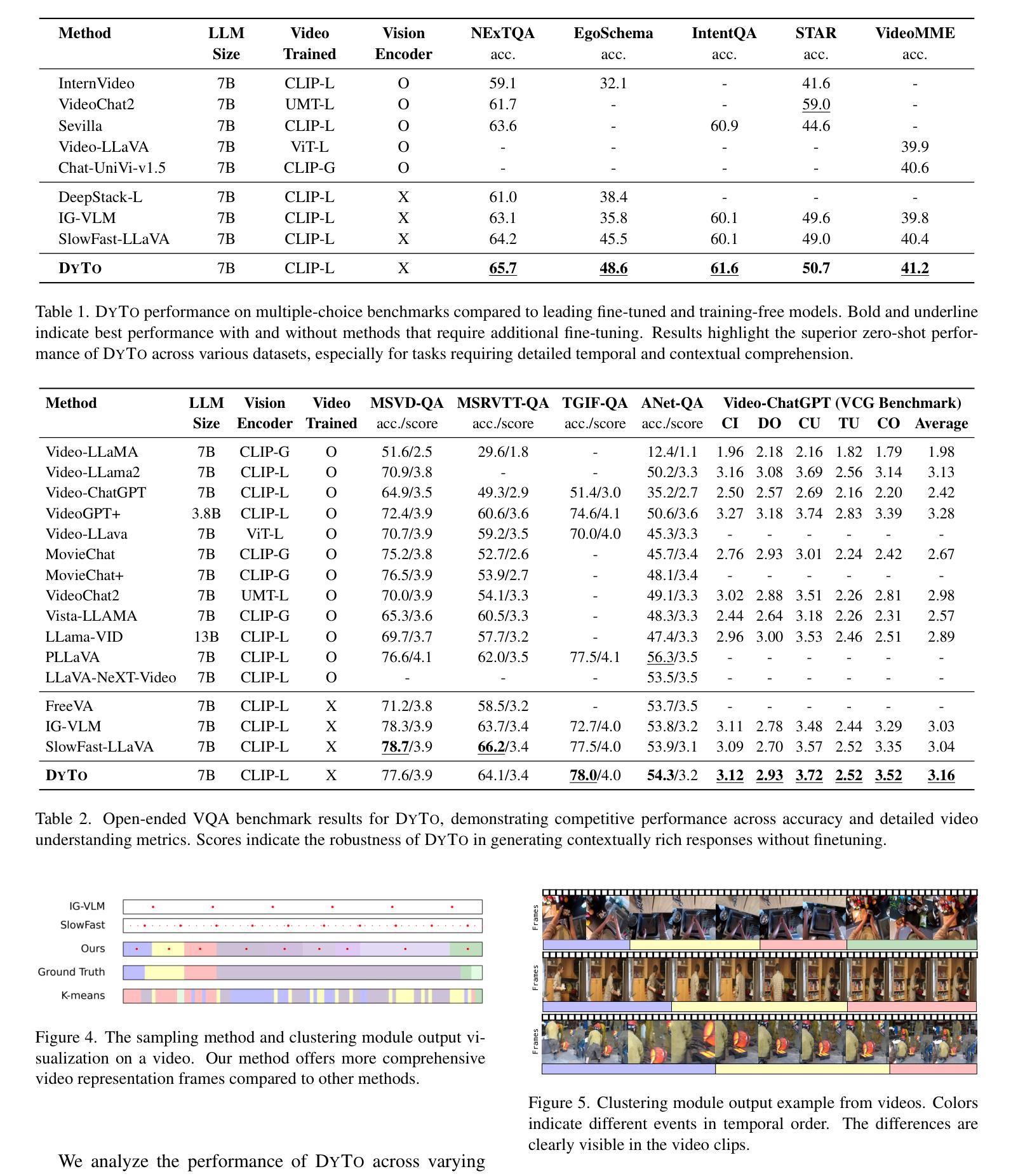
Principles of Visual Tokens for Efficient Video Understanding
Authors:Xinyue Hao, Gen Li, Shreyank N Gowda, Robert B Fisher, Jonathan Huang, Anurag Arnab, Laura Sevilla-Lara
Video understanding has made huge strides in recent years, relying largely on the power of the transformer architecture. As this architecture is notoriously expensive and video is highly redundant, research into improving efficiency has become particularly relevant. This has led to many creative solutions, including token merging and token selection. While most methods succeed in reducing the cost of the model and maintaining accuracy, an interesting pattern arises: most methods do not outperform the random sampling baseline. In this paper we take a closer look at this phenomenon and make several observations. First, we develop an oracle for the value of tokens which exposes a clear Pareto distribution where most tokens have remarkably low value, and just a few carry most of the perceptual information. Second, we analyze why this oracle is extremely hard to learn, as it does not consistently coincide with visual cues. Third, we observe that easy videos need fewer tokens to maintain accuracy. We build on these and further insights to propose a lightweight video model we call LITE that can select a small number of tokens effectively, outperforming state-of-the-art and existing baselines across datasets (Kinetics400 and Something-Something-V2) in the challenging trade-off of computation (GFLOPs) vs accuracy.
视频理解技术在近年来取得了巨大的进步,这主要得益于Transformer架构的强大性能。由于该架构非常昂贵且视频具有高度冗余性,关于提高效率的研究变得尤为重要。这导致了许多创造性的解决方案,包括令牌合并和令牌选择。虽然大多数方法在降低模型成本的同时保持了准确性,但出现了一个有趣的现象:大多数方法并没有超越随机抽样基线。在本文中,我们对这一现象进行了深入研究并进行了多次观察。首先,我们为令牌的价值开发了一个oracle,它暴露了一个清晰的帕累托分布,其中大多数令牌的价值相当低,只有少数令牌携带了大部分的感知信息。其次,我们分析了为什么这个oracle很难学习,因为它并不总是与视觉线索相吻合。第三,我们观察到简单的视频只需要更少的令牌就能维持准确性。我们基于这些观察以及其他进一步的见解,提出了一个轻量级的视频模型,我们称之为LITE。该模型可以有效地选择一小部分令牌,在数据集(Kinetics400和Something-Something-V2)的计算(GFLOPs)与准确性之间的权衡上具有挑战性,且表现优于最新技术和现有基线。
论文及项目相关链接
Summary
视频理解领域近年来取得了巨大进展,主要依赖于Transformer架构的力量。由于该架构成本高昂且视频冗余度高,提高效率的研究变得尤为重要。本文观察到一个现象:尽管许多方法成功降低了模型成本并保持了准确性,但它们并不优于随机抽样基线。本文对此现象进行了深入研究,并发现大多数令牌的价值极低,只有少数携带大部分感知信息。此外,本文分析了学习这种令牌价值困难的原因,发现其并不一致地与视觉线索相吻合。同时,本文观察到简单的视频维持准确性的令牌数量更少。基于这些和其他见解,本文提出了一种轻量级的视频模型LITE,它能够有效地选择少量令牌,在数据集(Kinetics400和Something-Something-V2)上实现了计算与准确性之间的权衡,优于现有基线和最新技术。
Key Takeaways
- 视频理解领域依赖Transformer架构取得进展,但成本高昂且视频冗余度高。
- 大多数令牌的价值极低,只有少数令牌携带大部分感知信息。
- 学习令牌价值困难的原因在于其与视觉线索的不一致性。
- 简单视频维持准确性的令牌数量更少。
- LITE模型能有效选择少量令牌。
- LITE模型在计算与准确性之间实现了权衡,优于现有基线和最新技术。
点此查看论文截图
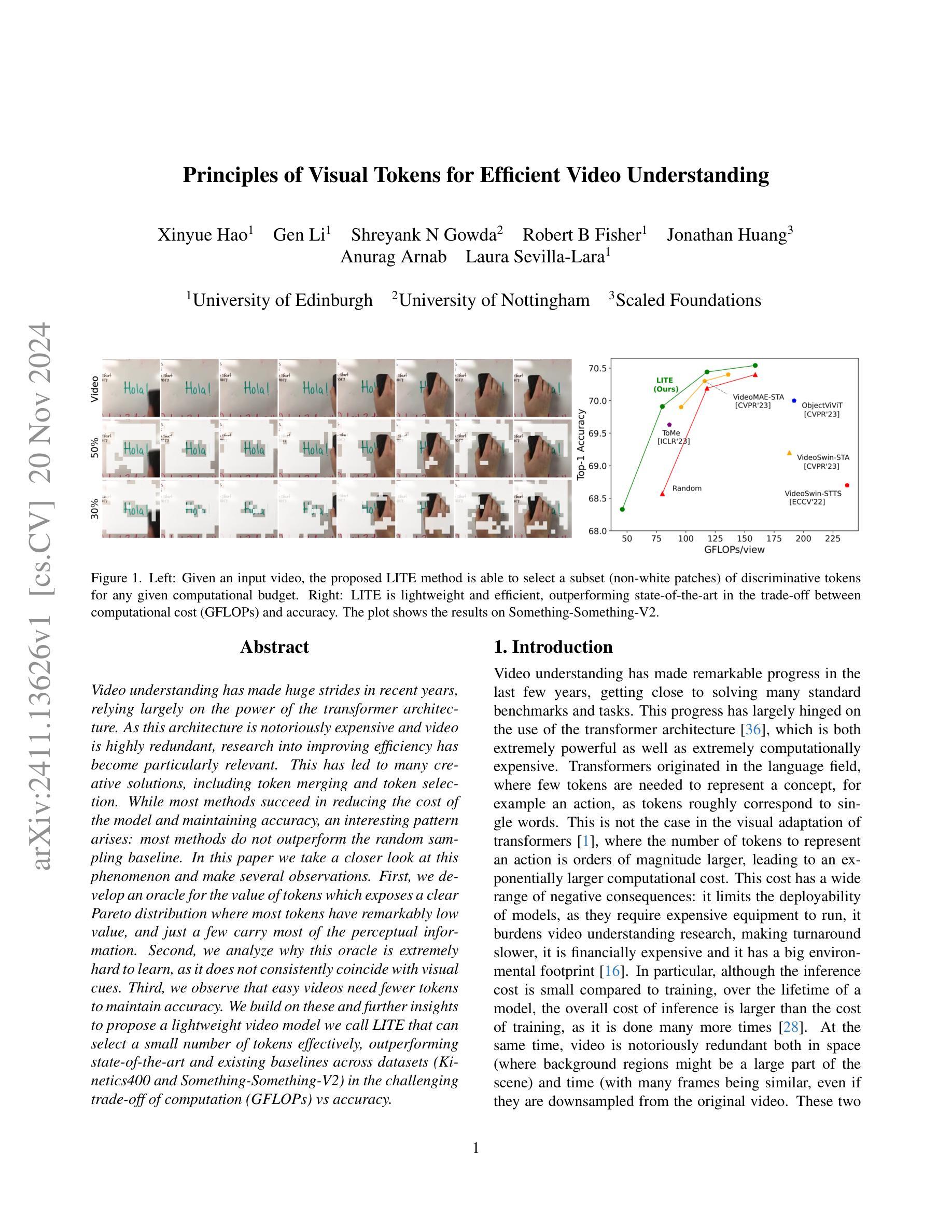
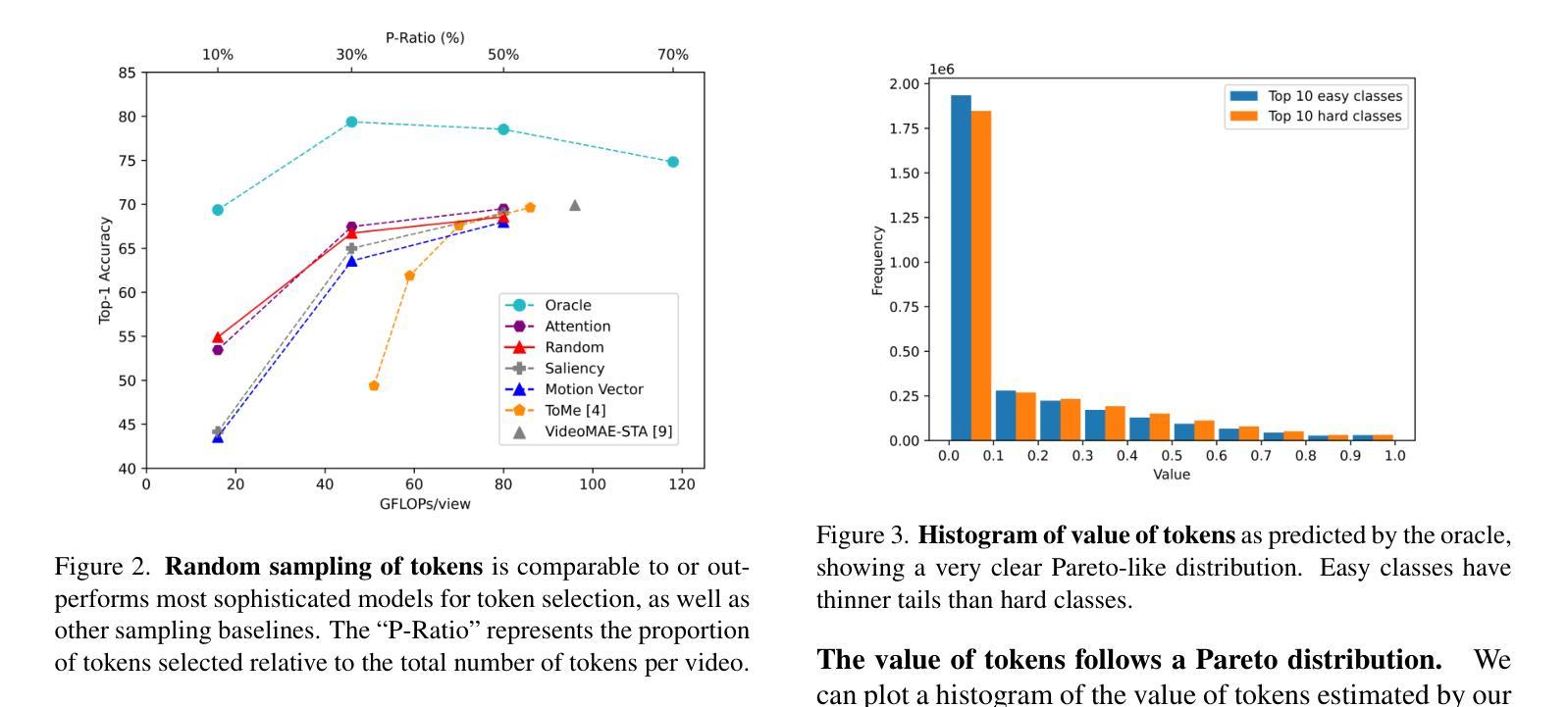
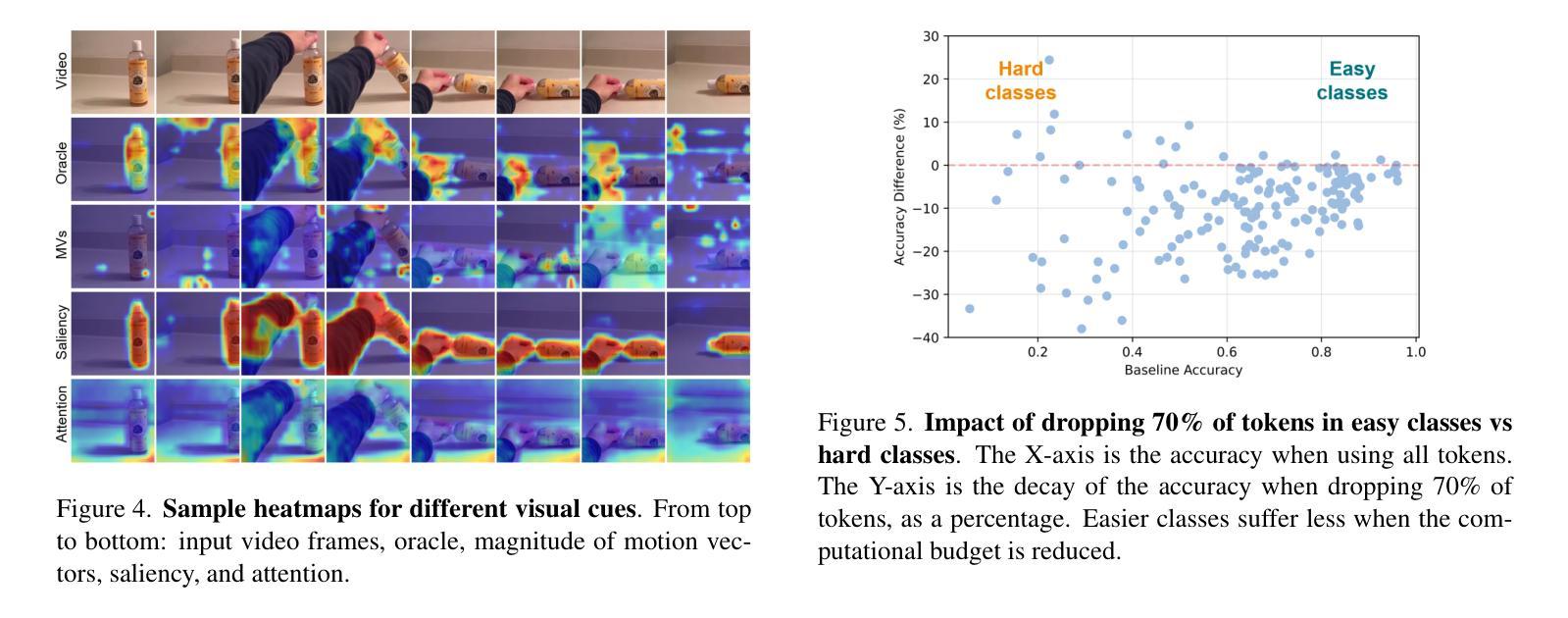
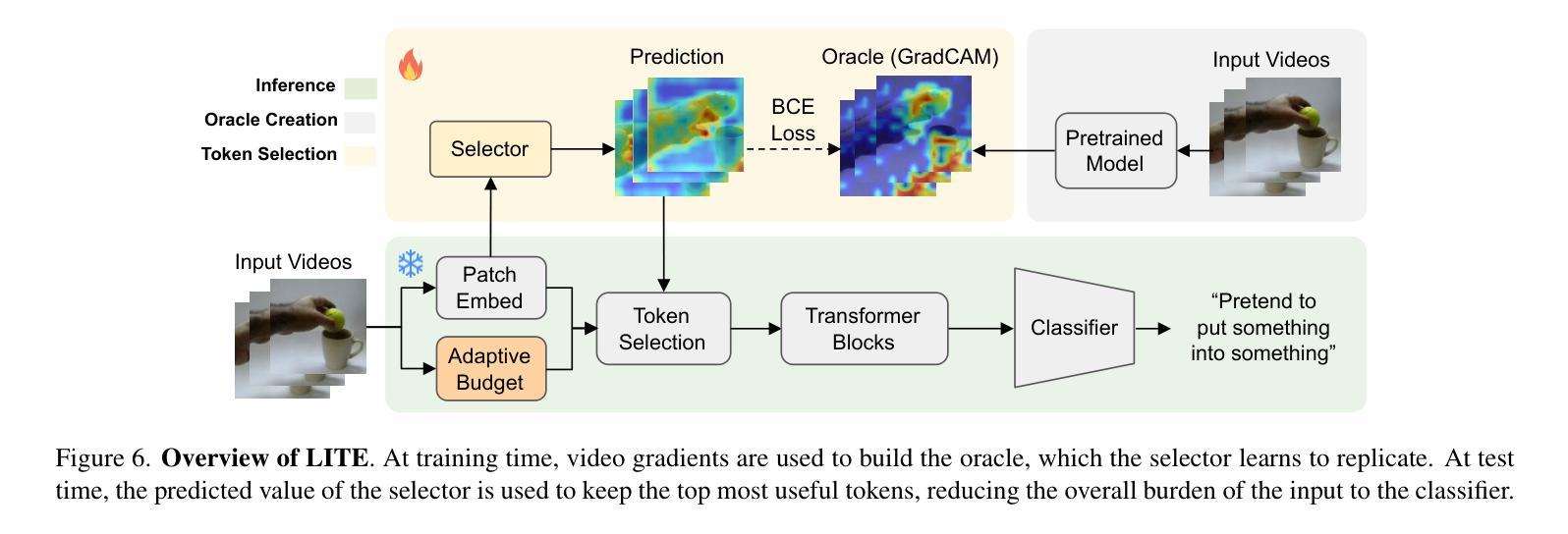
AdaCM$^2$: On Understanding Extremely Long-Term Video with Adaptive Cross-Modality Memory Reduction
Authors:Yuanbin Man, Ying Huang, Chengming Zhang, Bingzhe Li, Wei Niu, Miao Yin
The advancements in large language models (LLMs) have propelled the improvement of video understanding tasks by incorporating LLMs with visual models. However, most existing LLM-based models (e.g., VideoLLaMA, VideoChat) are constrained to processing short-duration videos. Recent attempts to understand long-term videos by extracting and compressing visual features into a fixed memory size. Nevertheless, those methods leverage only visual modality to merge video tokens and overlook the correlation between visual and textual queries, leading to difficulties in effectively handling complex question-answering tasks. To address the challenges of long videos and complex prompts, we propose AdaCM$^2$, which, for the first time, introduces an adaptive cross-modality memory reduction approach to video-text alignment in an auto-regressive manner on video streams. Our extensive experiments on various video understanding tasks, such as video captioning, video question answering, and video classification, demonstrate that AdaCM$^2$ achieves state-of-the-art performance across multiple datasets while significantly reducing memory usage. Notably, it achieves a 4.5% improvement across multiple tasks in the LVU dataset with a GPU memory consumption reduction of up to 65%.
随着大型语言模型(LLM)的进步,通过将LLM与视觉模型相结合,推动了视频理解任务的改进。然而,大多数现有的基于LLM的模型(例如VideoLLaMA、VideoChat)仅限于处理短时视频。最近有人尝试通过提取和压缩视觉特征到固定内存大小来理解长视频。然而,这些方法只利用视觉模式来合并视频令牌,忽视了视觉和文本查询之间的相关性,导致难以有效处理复杂的问答任务。为了解决长视频和复杂提示的挑战,我们提出了AdaCM$^2$,它首次引入了一种自适应跨模态内存缩减方法,以自回归的方式在视频流上进行视频文本对齐。我们在各种视频理解任务上进行了广泛实验,如视频描述、视频问答和视频分类,结果表明AdaCM$^2$在多个数据集上实现了最先进的性能,同时显著降低了内存使用。值得注意的是,它在LVU数据集的多项任务上实现了4.5%的改进,GPU内存消耗最多减少了65%。
论文及项目相关链接
Summary:大型语言模型(LLM)的进展推动了视频理解任务的进步,通过将LLM与视觉模型结合。然而,大多数现有的LLM模型主要用于处理短视频。对于长视频的认知,现有方法仅利用视觉模式合并视频符号,忽略了视频与文本查询之间的关联,难以应对复杂问答任务。为解决长视频和复杂提示的挑战,首次提出AdaCM$^2$模型,采用自适应跨模态记忆缩减方法,以自适应方式对齐视频文本。实验证明,AdaCM$^2$在多个视频理解任务上表现卓越,如视频描述、视频问答和视频分类等,并在LVU数据集上实现了高达4.5%的提升,同时GPU内存消耗降低了65%。
Key Takeaways:
- 大型语言模型的进步促进了视频理解任务的发展。
- 当前模型主要处理短视频,对长视频的认知存在挑战。
- 现有方法在处理长视频时仅利用视觉模式,忽略了视频与文本查询的关联。
- AdaCM$^2$模型首次采用自适应跨模态记忆缩减方法处理视频文本对齐。
- AdaCM$^2$在多个视频理解任务上表现优秀。
- AdaCM$^2$在LVU数据集上的性能提升显著。
点此查看论文截图
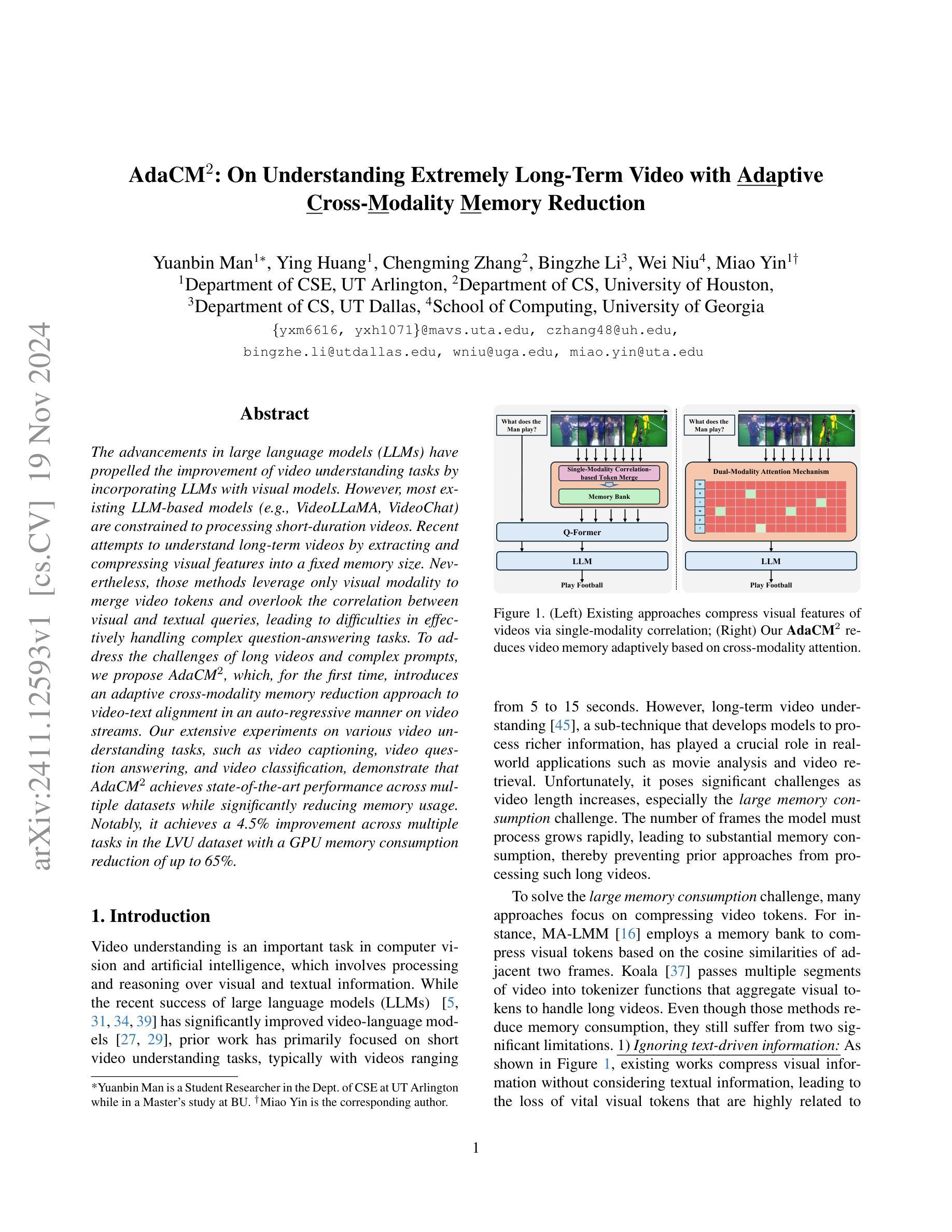
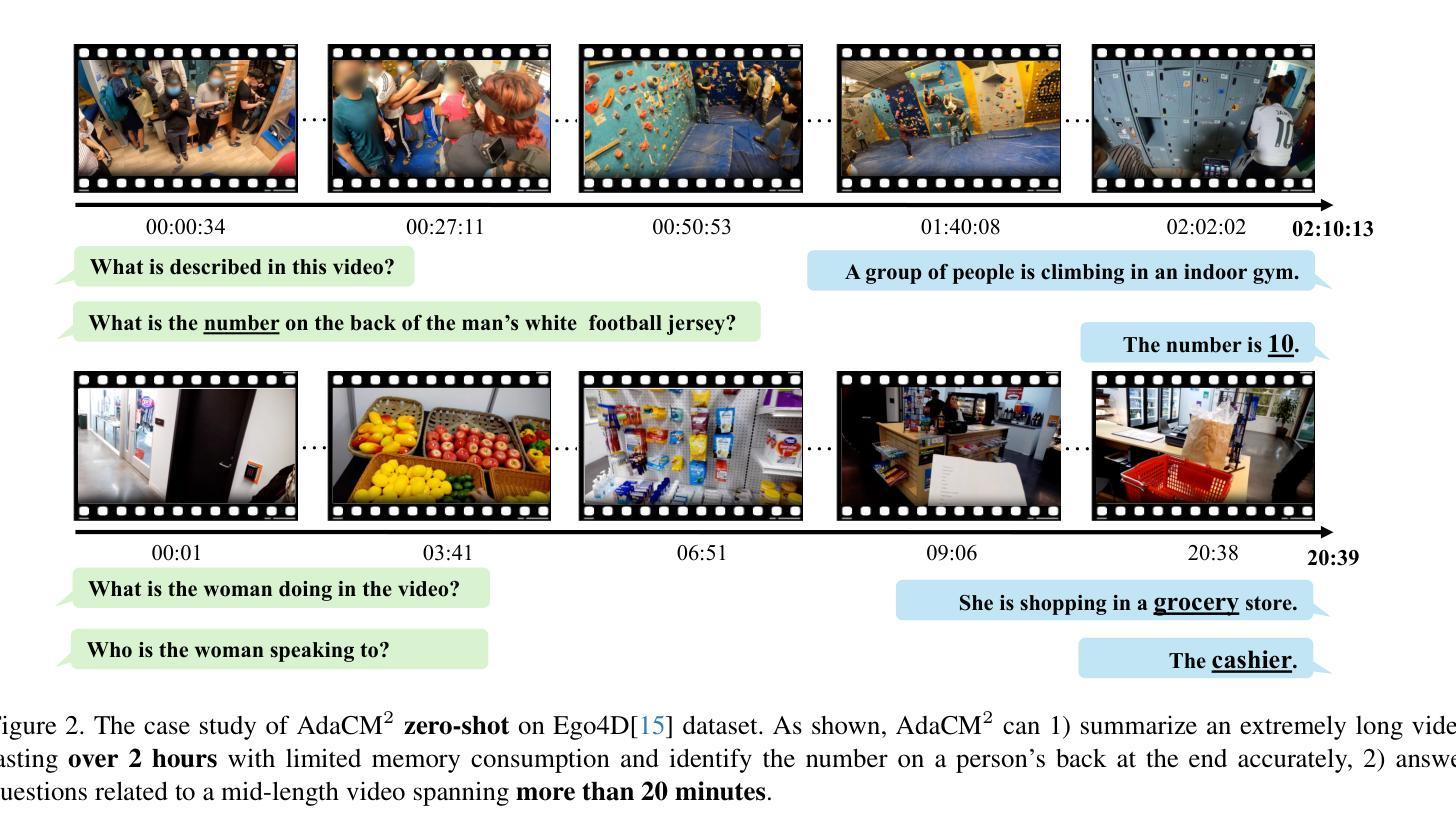
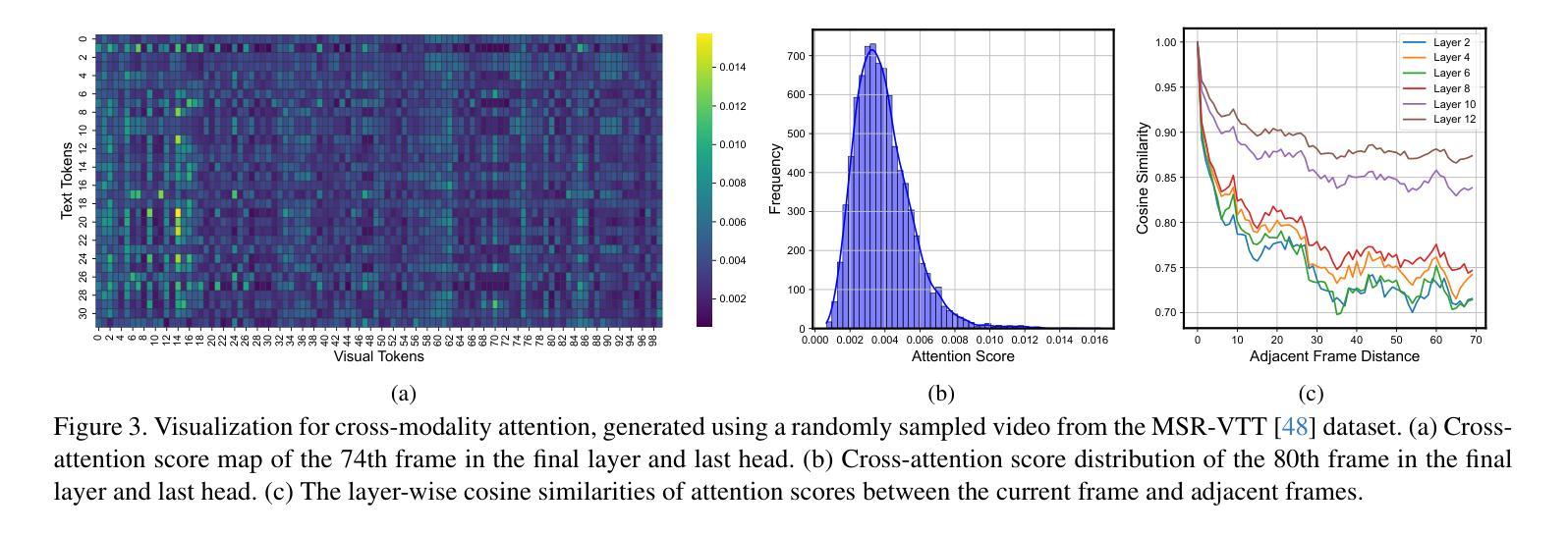
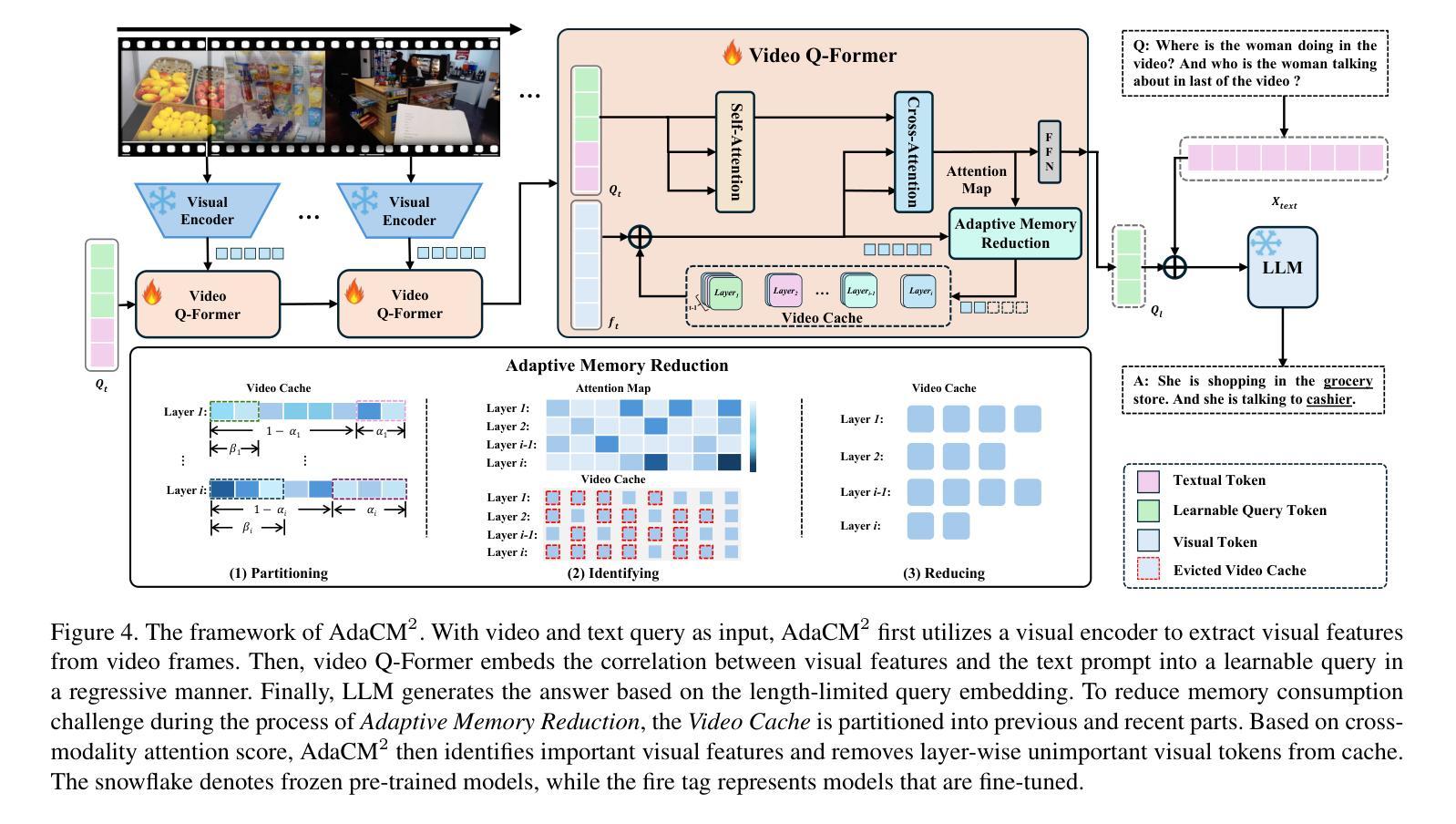
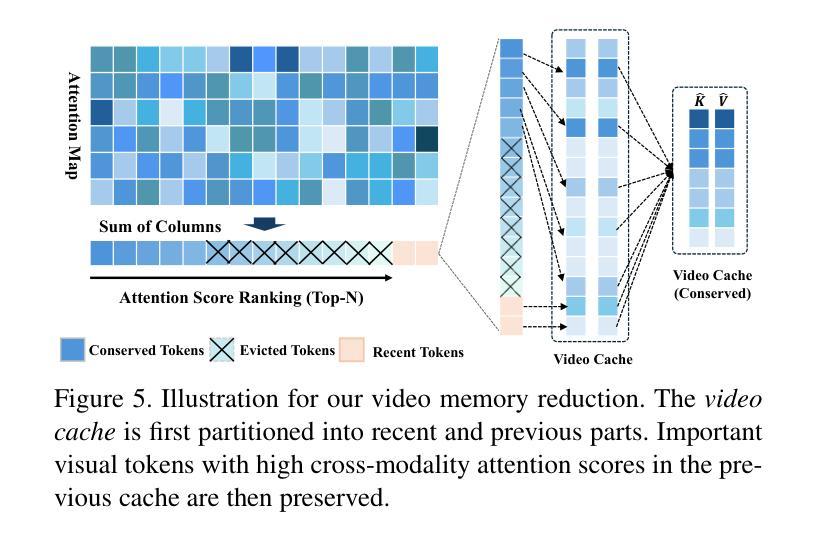
DynFocus: Dynamic Cooperative Network Empowers LLMs with Video Understanding
Authors:Yudong Han, Qingpei Guo, Liyuan Pan, Liu Liu, Yu Guan, Ming Yang
The challenge in LLM-based video understanding lies in preserving visual and semantic information in long videos while maintaining a memory-affordable token count. However, redundancy and correspondence in videos have hindered the performance potential of existing methods. Through statistical learning on current datasets, we observe that redundancy occurs in both repeated and answer-irrelevant frames, and the corresponding frames vary with different questions. This suggests the possibility of adopting dynamic encoding to balance detailed video information preservation with token budget reduction. To this end, we propose a dynamic cooperative network, DynFocus, for memory-efficient video encoding in this paper. Specifically, i) a Dynamic Event Prototype Estimation (DPE) module to dynamically select meaningful frames for question answering; (ii) a Compact Cooperative Encoding (CCE) module that encodes meaningful frames with detailed visual appearance and the remaining frames with sketchy perception separately. We evaluate our method on five publicly available benchmarks, and experimental results consistently demonstrate that our method achieves competitive performance.
基于大型语言模型(LLM)的视频理解挑战在于在长视频中保留视觉和语义信息的同时,保持可承受的令牌计数。然而,视频中的冗余和对应关系阻碍了现有方法的性能潜力。通过对当前数据集进行统计学习,我们发现冗余现象既出现在重复帧中也出现在与答案无关的帧中,并且对应帧会因不同问题而变化。这提示我们有可能采用动态编码来平衡保留详细的视频信息与减少令牌预算。为此,本文提出了一种用于内存高效视频编码的动态协同网络DynFocus。具体来说,一是对动态事件原型估计(DPE)模块进行动态选择有意义的帧用于问答;二是紧凑协同编码(CCE)模块,该模块对有意义的帧进行详细视觉外观编码,对其余帧进行草图感知编码。我们在五个公开可用基准测试上评估了我们的方法,实验结果表明我们的方法具有竞争力。
论文及项目相关链接
PDF 8 pages, 6 figures
Summary
本文指出基于大型语言模型(LLM)的视频理解的挑战在于如何在保持视频视觉和语义信息的同时,控制记忆负担。现有方法受到冗余和对应问题的困扰,本文通过当前数据集进行统计学习,发现冗余存在于重复和与答案无关的帧中,对应帧因不同问题而异。因此,本文提出采用动态编码以平衡视频信息的详细保存与令牌预算的减少。为此,提出了一种动态协作网络DynFocus用于内存高效的视频编码。具体而言,包括动态事件原型估计模块(DPE)选择对问答有意义的帧;紧凑合作编码模块(CCE)分别对有意义的帧进行详细的视觉外观编码,对其余帧进行草图感知编码。在五个公开基准上的实验结果表明,该方法具有竞争力。
Key Takeaways
- 基于大型语言模型(LLM)的视频理解的挑战在于保持视觉和语义信息的同时控制内存负担。
- 冗余存在于重复和与答案无关的帧中,影响现有方法的性能。
- 统计学习发现,不同问题对应的帧不同。
- 提出动态编码以平衡视频信息保存与令牌预算减少。
- 引入动态协作网络DynFocus,包括动态事件原型估计模块和紧凑合作编码模块。
- 方法在五个公开基准上进行评估,表现具有竞争力。
点此查看论文截图
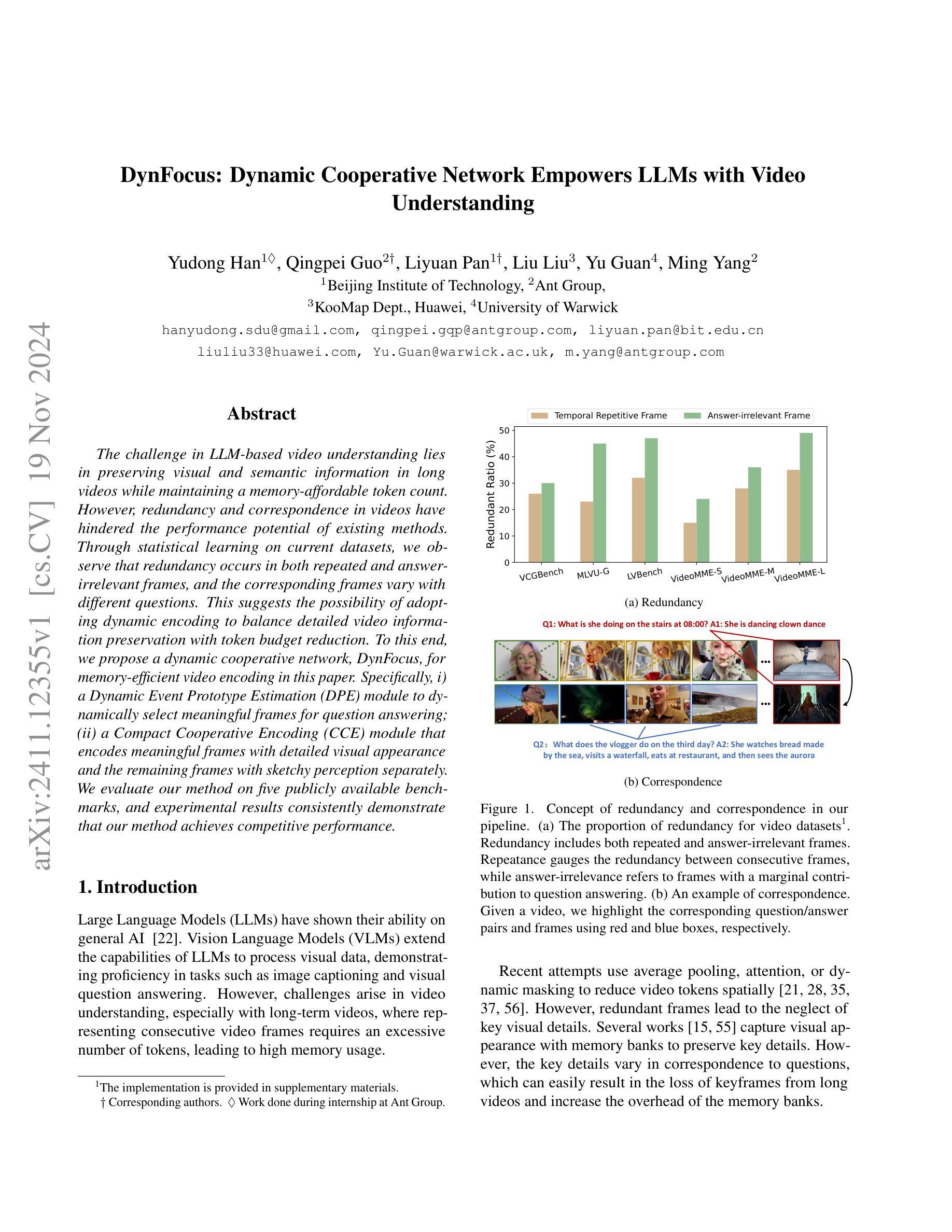
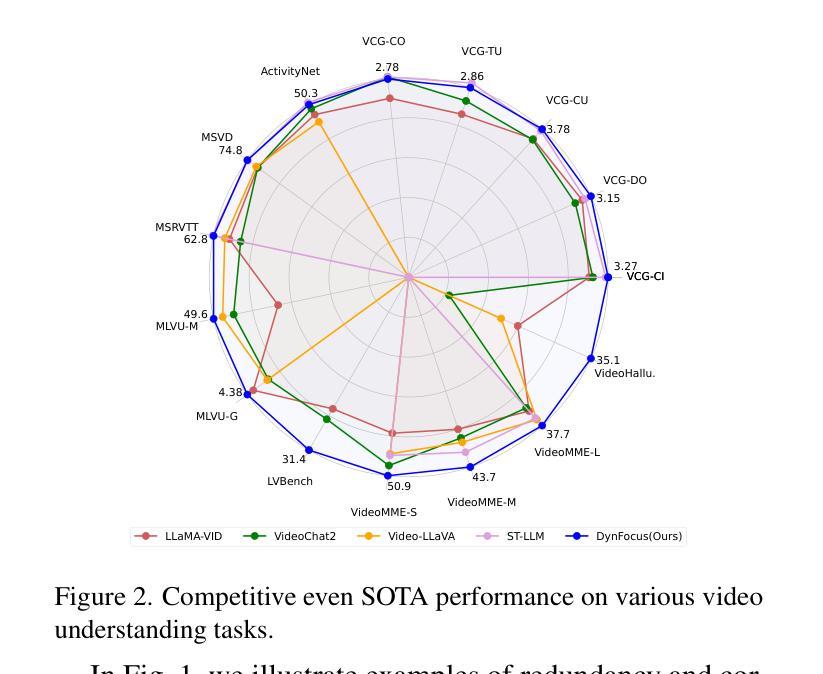
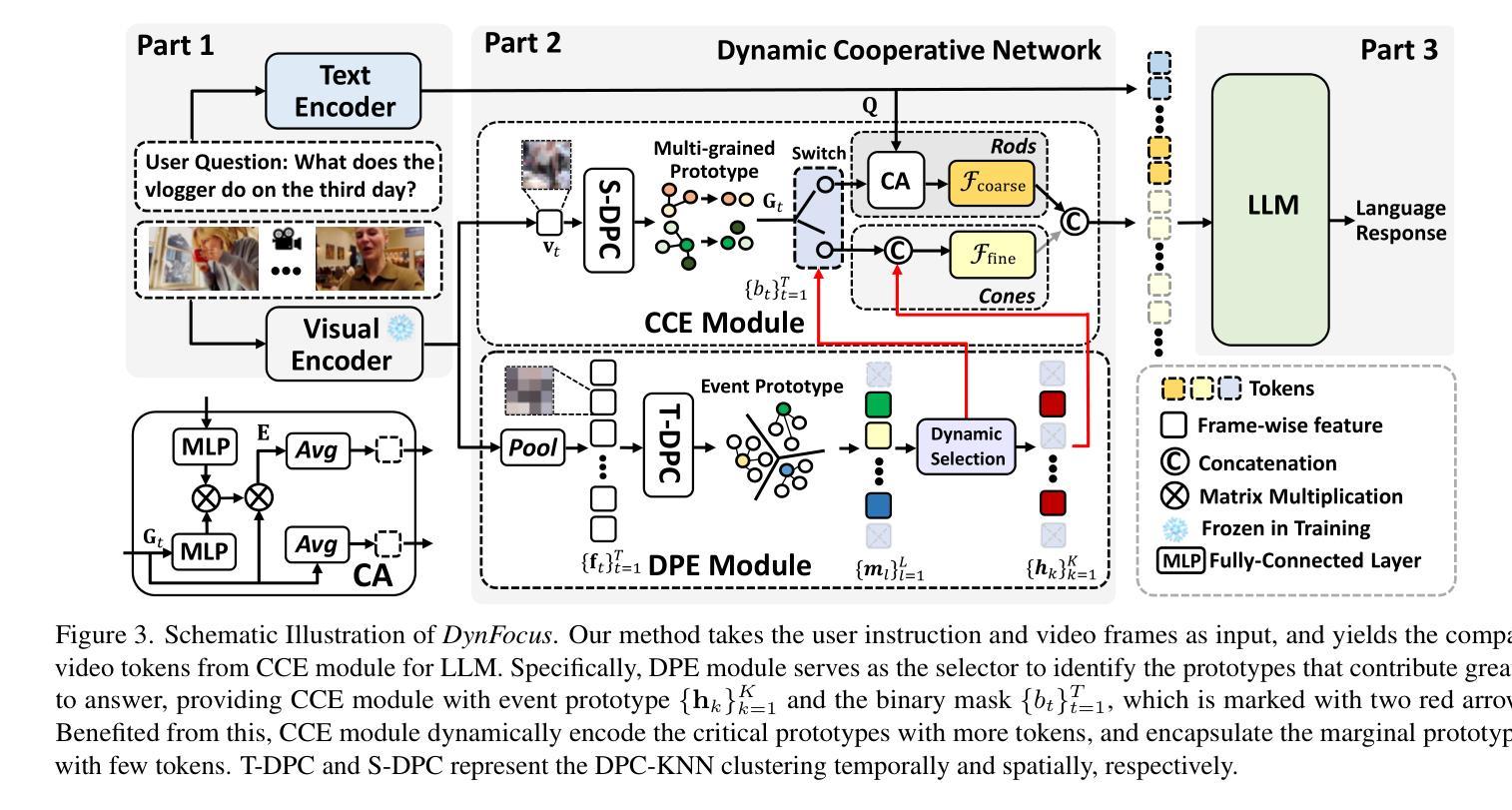
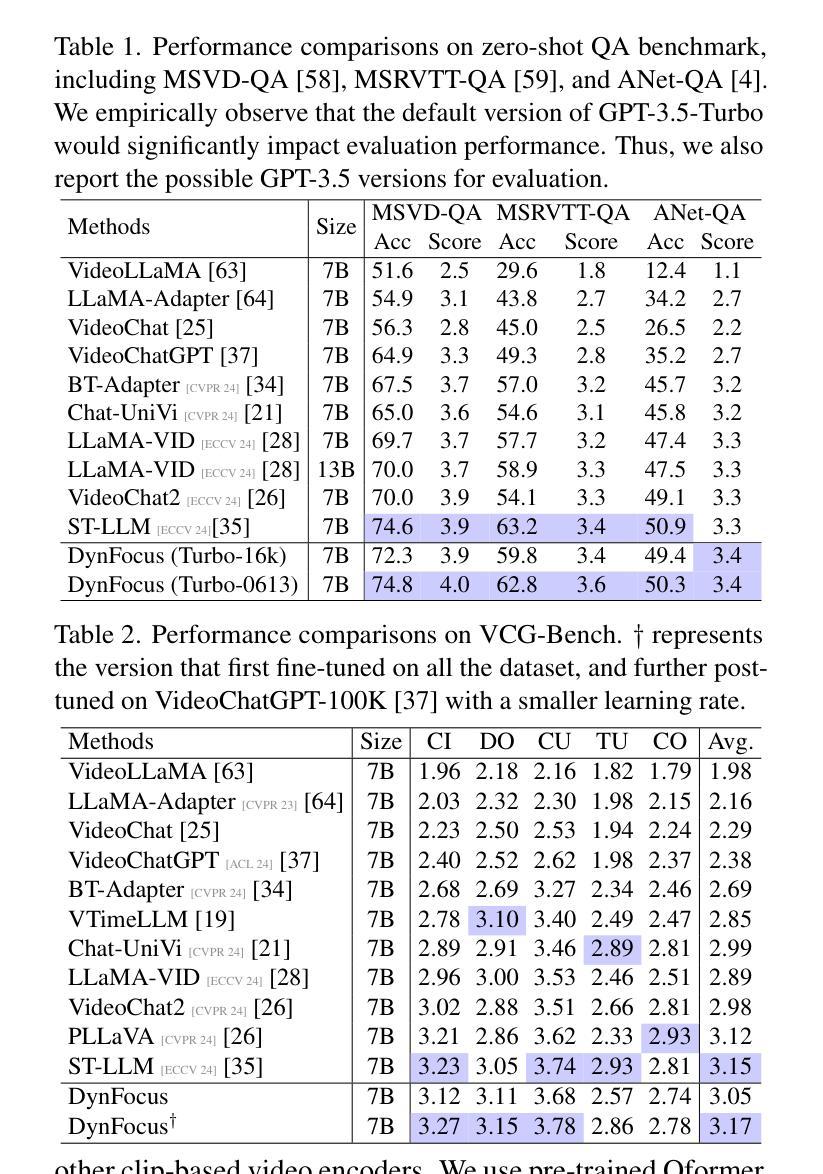
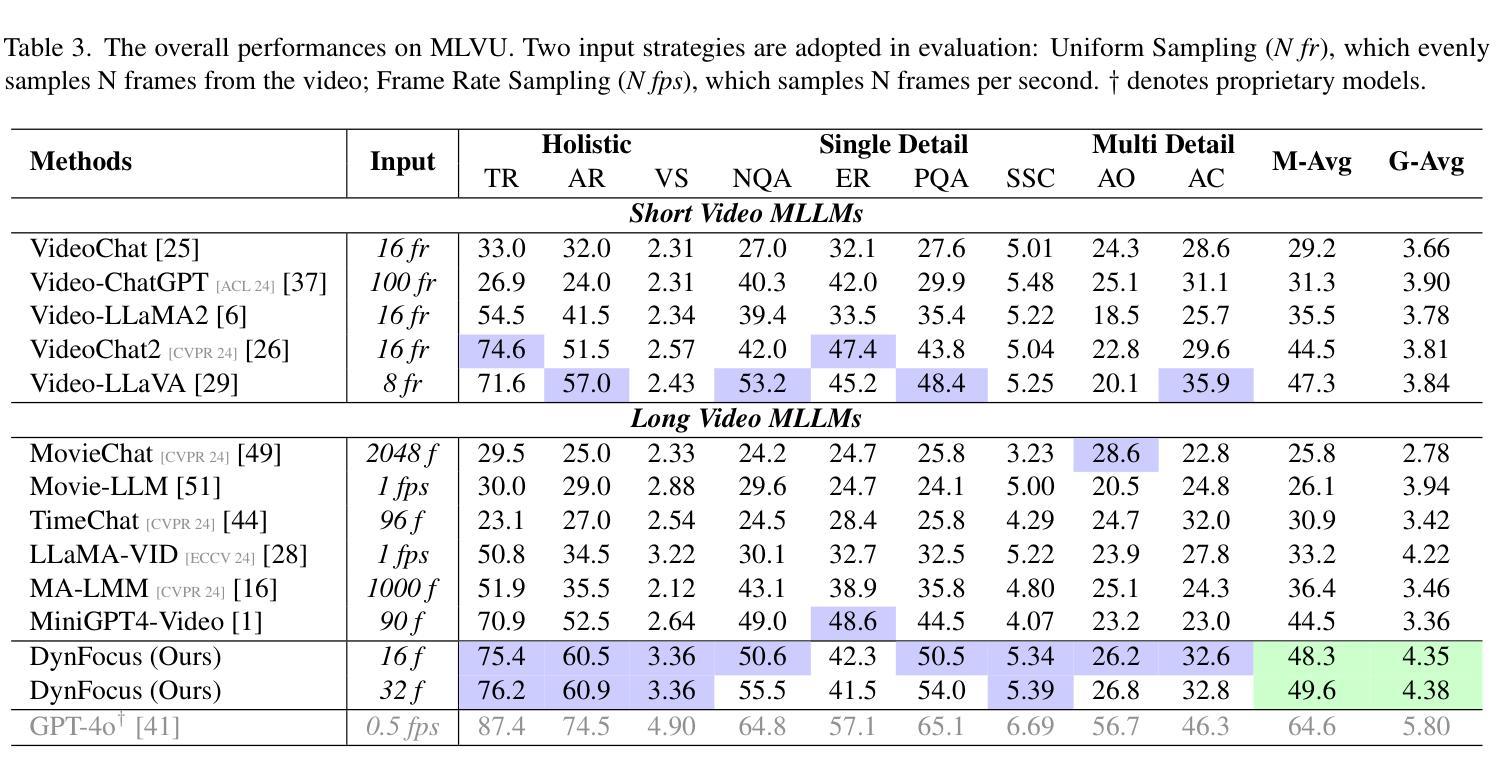
Motion-Grounded Video Reasoning: Understanding and Perceiving Motion at Pixel Level
Authors:Andong Deng, Tongjia Chen, Shoubin Yu, Taojiannan Yang, Lincoln Spencer, Yapeng Tian, Ajmal Saeed Mian, Mohit Bansal, Chen Chen
In this paper, we introduce Motion-Grounded Video Reasoning, a new motion understanding task that requires generating visual answers (video segmentation masks) according to the input question, and hence needs implicit spatiotemporal reasoning and grounding. This task extends existing spatiotemporal grounding work focusing on explicit action/motion grounding, to a more general format by enabling implicit reasoning via questions. To facilitate the development of the new task, we collect a large-scale dataset called GROUNDMORE, which comprises 1,715 video clips, 249K object masks that are deliberately designed with 4 question types (Causal, Sequential, Counterfactual, and Descriptive) for benchmarking deep and comprehensive motion reasoning abilities. GROUNDMORE uniquely requires models to generate visual answers, providing a more concrete and visually interpretable response than plain texts. It evaluates models on both spatiotemporal grounding and reasoning, fostering to address complex challenges in motion-related video reasoning, temporal perception, and pixel-level understanding. Furthermore, we introduce a novel baseline model named Motion-Grounded Video Reasoning Assistant (MORA). MORA incorporates the multimodal reasoning ability from the Multimodal LLM, the pixel-level perception capability from the grounding model (SAM), and the temporal perception ability from a lightweight localization head. MORA achieves respectable performance on GROUNDMORE outperforming the best existing visual grounding baseline model by an average of 21.5% relatively. We hope this novel and challenging task will pave the way for future advancements in robust and general motion understanding via video reasoning segmentation
本文介绍了基于动作的视频推理(Motion-Grounded Video Reasoning),这是一个新的动作理解任务。它要求根据输入问题生成视觉答案(视频分割掩码),因此需要隐式的时空推理和定位。这个任务扩展了现有的时空定位工作,从侧重于显式动作/运动定位,发展到了通过问题进行隐式推理的更通用格式。为了促进新任务的发展,我们收集了一个大规模数据集,名为GROUNDMORE。它包含1715个视频片段和24万多个对象掩码,这些掩码是专门设计的,包括因果、顺序、反事实和描述四种类型的问题,用于评估深度和综合运动推理能力。GROUNDMORE要求模型生成视觉答案,提供了一个比纯文本更具体和视觉可解释的响应。它评估了模型的时空定位和推理能力,有助于解决与运动相关的视频推理、时间感知和像素级理解的复杂挑战。此外,我们还引入了一种新型的基准模型,名为Motion-Grounded Video Reasoning Assistant(MORA)。MORA结合了多模态LLM的多模态推理能力、定位模型(SAM)的像素级感知能力和轻量化定位头的时序感知能力。在GROUNDMORE上,MORA取得了令人尊敬的业绩,平均比现有的最佳视觉定位基准模型高出21.5%。我们希望这个新颖而具有挑战性的任务将为未来通过视频推理分割进行稳健和通用运动理解的研究铺平道路。
论文及项目相关链接
Summary
本文提出了Motion-Grounded Video Reasoning任务,该任务需要依据输入问题生成视觉答案(视频分割掩膜),需要进行隐式时空推理和定位。为推进此新任务的发展,作者收集了一个大规模数据集GROUNDMORE,包含1715个视频片段和24.9万个对象掩膜,特意设计了四种问题类型(因果、顺序、反事实和描述)以评估深度和综合运动推理能力。此外,作者还介绍了一种新的基线模型MORA,该模型具备多模态推理能力、像素级感知能力和时间感知能力,在GROUNDMORE上表现优异,相较于现有最佳视觉定位基线模型,平均提高了21.5%的性能。
Key Takeaways
- 引入了Motion-Grounded Video Reasoning任务,要求根据输入问题生成视觉答案,需要隐式时空推理和定位。
- 推出大规模数据集GROUNDMORE,包含多样化视频片段和对象掩膜,用于评估深度和综合运动推理能力。
- GROUNDMORE数据集要求模型生成视觉答案,提供比纯文本更具体和可解释的反应。
- MORA模型具备多模态推理能力、像素级感知能力和时间感知能力。
- MORA在GROUNDMORE数据集上的性能表现优异。
- MORA相较于现有最佳视觉定位基线模型,平均提高了21.5%的性能。
点此查看论文截图
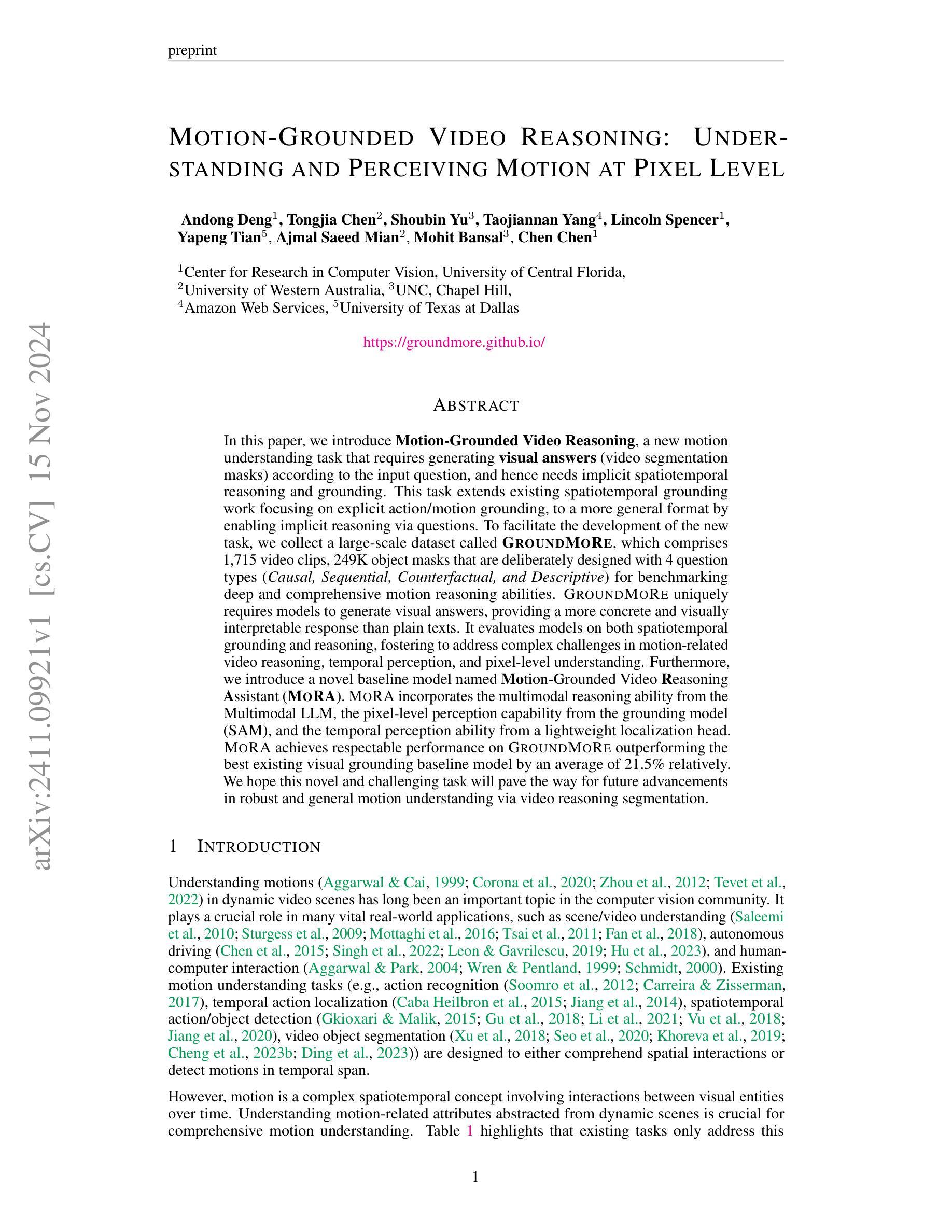
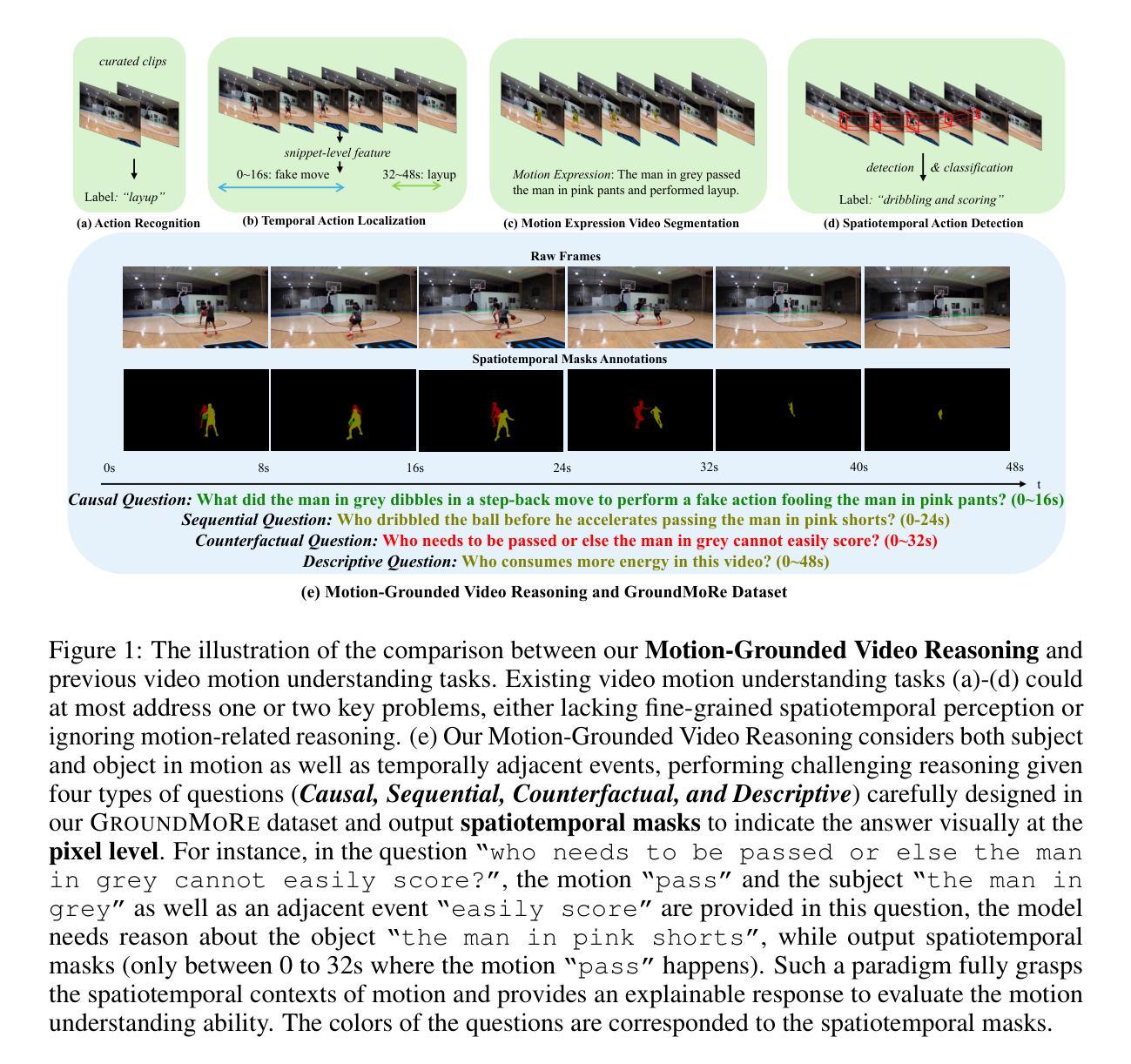
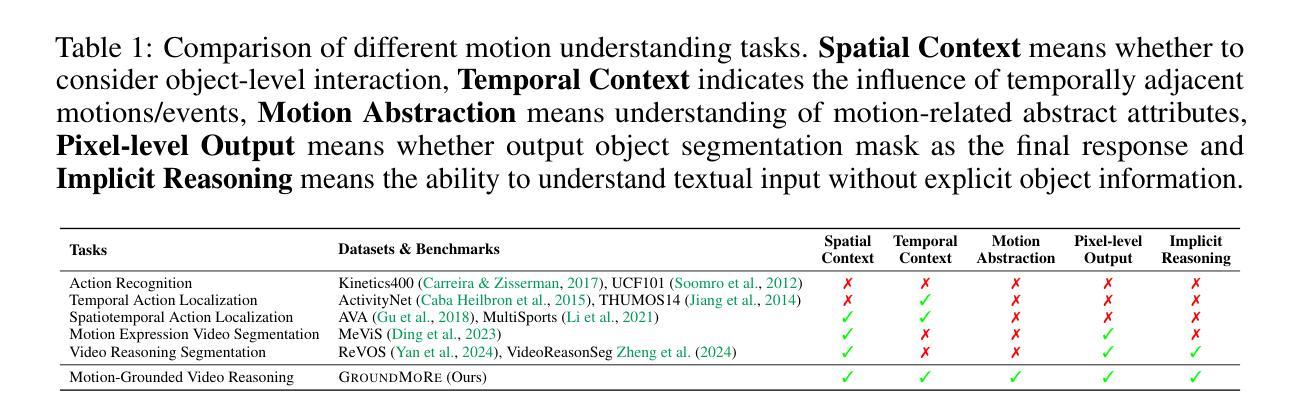
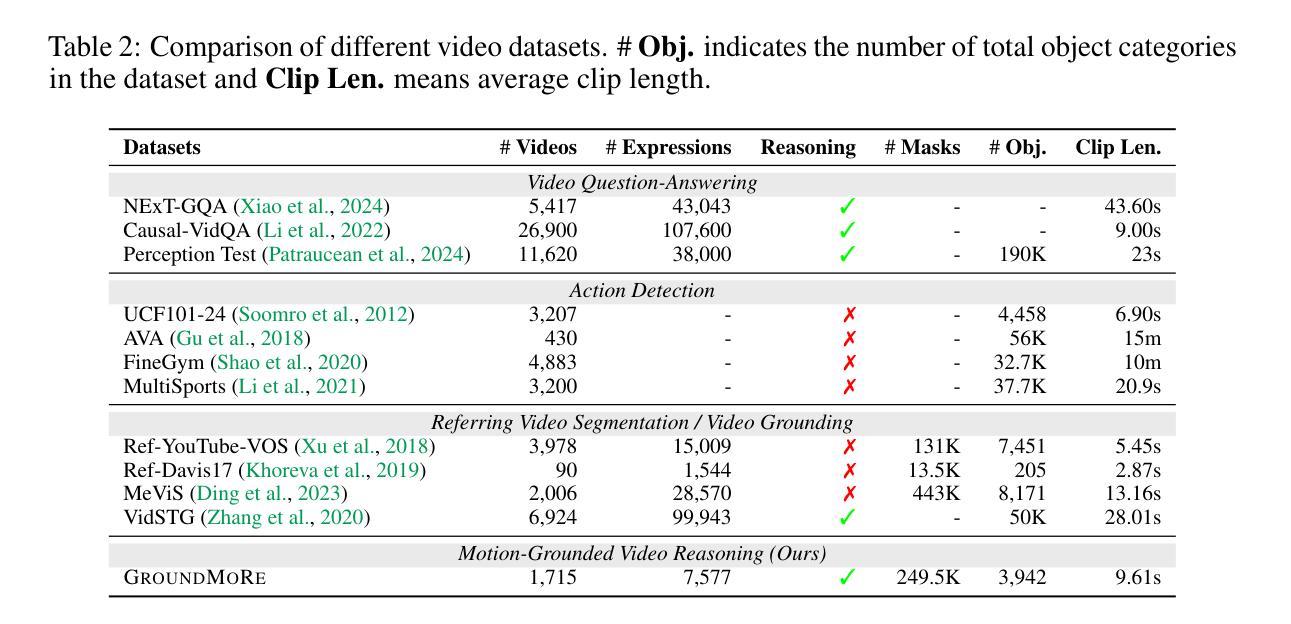
HourVideo: 1-Hour Video-Language Understanding
Authors:Keshigeyan Chandrasegaran, Agrim Gupta, Lea M. Hadzic, Taran Kota, Jimming He, Cristóbal Eyzaguirre, Zane Durante, Manling Li, Jiajun Wu, Li Fei-Fei
We present HourVideo, a benchmark dataset for hour-long video-language understanding. Our dataset consists of a novel task suite comprising summarization, perception (recall, tracking), visual reasoning (spatial, temporal, predictive, causal, counterfactual), and navigation (room-to-room, object retrieval) tasks. HourVideo includes 500 manually curated egocentric videos from the Ego4D dataset, spanning durations of 20 to 120 minutes, and features 12,976 high-quality, five-way multiple-choice questions. Benchmarking results reveal that multimodal models, including GPT-4 and LLaVA-NeXT, achieve marginal improvements over random chance. In stark contrast, human experts significantly outperform the state-of-the-art long-context multimodal model, Gemini Pro 1.5 (85.0% vs. 37.3%), highlighting a substantial gap in multimodal capabilities. Our benchmark, evaluation toolkit, prompts, and documentation are available at https://hourvideo.stanford.edu
我们推出HourVideo,这是一项针对长达一小时的视频语言理解的基准测试数据集。我们的数据集包含一组新颖的任务组合,包括摘要、感知(回忆、跟踪)、视觉推理(空间、时间、预测、因果、反事实)和导航(房间到房间、对象检索)任务。HourVideo包含来自Ego4D数据集的500个手动策划的以自我为中心的视频,时长从20分钟到120分钟不等,并设有高质量的五选一的多项选择题共计有特色方式组建成对套装一万二千九百七十六份评估模型发现各种模型基线方案随着其他备选路线增强带来了相较于随机抽取改善的轻微改变其中最显著的例子即为在构建简单逻辑推理电路这个任务场景中包占星控发挥了自己强势的一面对手旗舰型号Gemini Pro在平均正确率上的结果较差达到将近差五点五倍效能相比较结果惨淡的局面展现了AI产品在模态领域仍然有着巨大差距我们的基准测试评估工具提示和文档均可在https://hourvideo.stanford.edu找到。
论文及项目相关链接
PDF NeurIPS 2024 Datasets and Benchmarks Track; 28 pages
摘要
本文介绍了HourVideo数据集,该数据集是用于一小时视频语言理解的基准数据集。它包含多个任务,包括摘要、感知(回忆、跟踪)、视觉推理(空间、时间、预测、因果、反事实)和导航(房间对房间、对象检索)。HourVideo包括来自Ego4D数据集的500个手动策划的以自我为中心的视频,时长在20至120分钟之间,含有高质量五选一选择题共一万二千九百七十六题。基准测试结果表明,包括GPT-4和LLaVA-NeXT在内的多模式模型较随机结果仅稍有改善。相较最先进的长期上下文多模式模型双子座Pro 1.5而言,人类专家表现出显著差异的优异性能(准确率由85%对比至仅约三分之一),揭示了多模式能力存在显著差距。我们的基准测试、评估工具包、提示以及文档可以在http://hourvideo.stanford.edu查阅。
关键要点
以下是本文所列的七点主要洞见:
- 介绍HourVideo数据集,它是用于一小时视频语言理解的基准数据集。
- HourVideo数据集包含多种任务,涵盖摘要撰写、感知理解等多元技能挑战。
点此查看论文截图
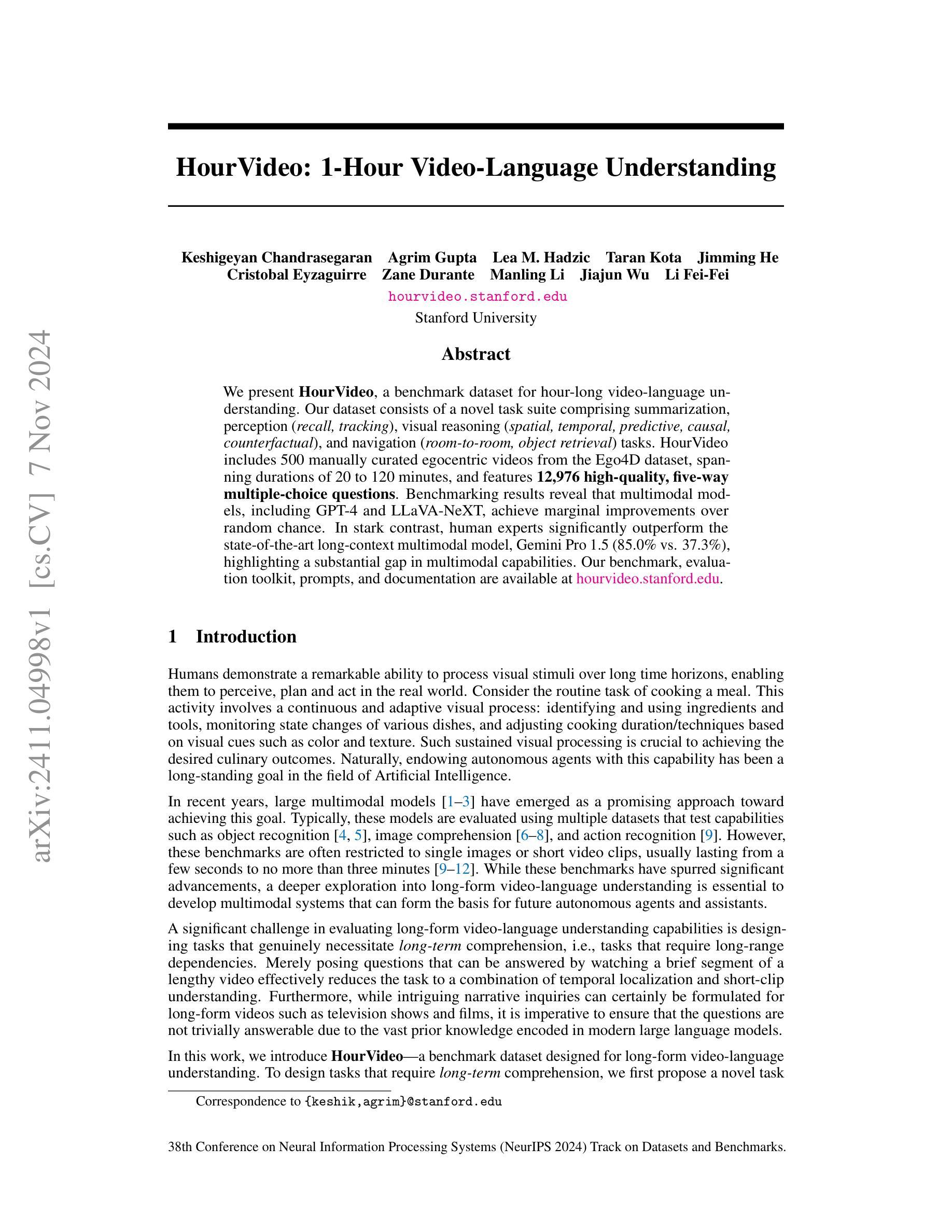
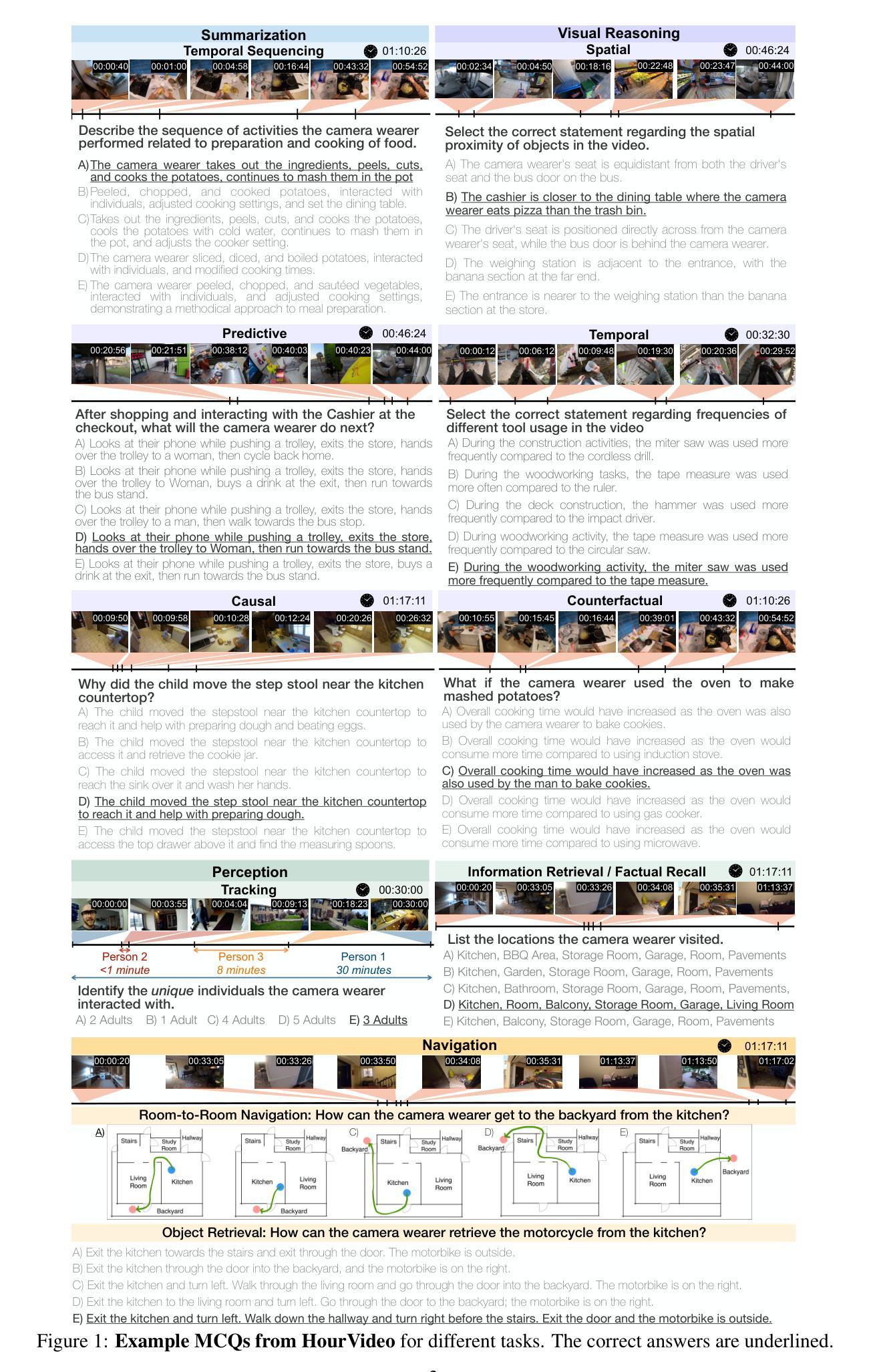
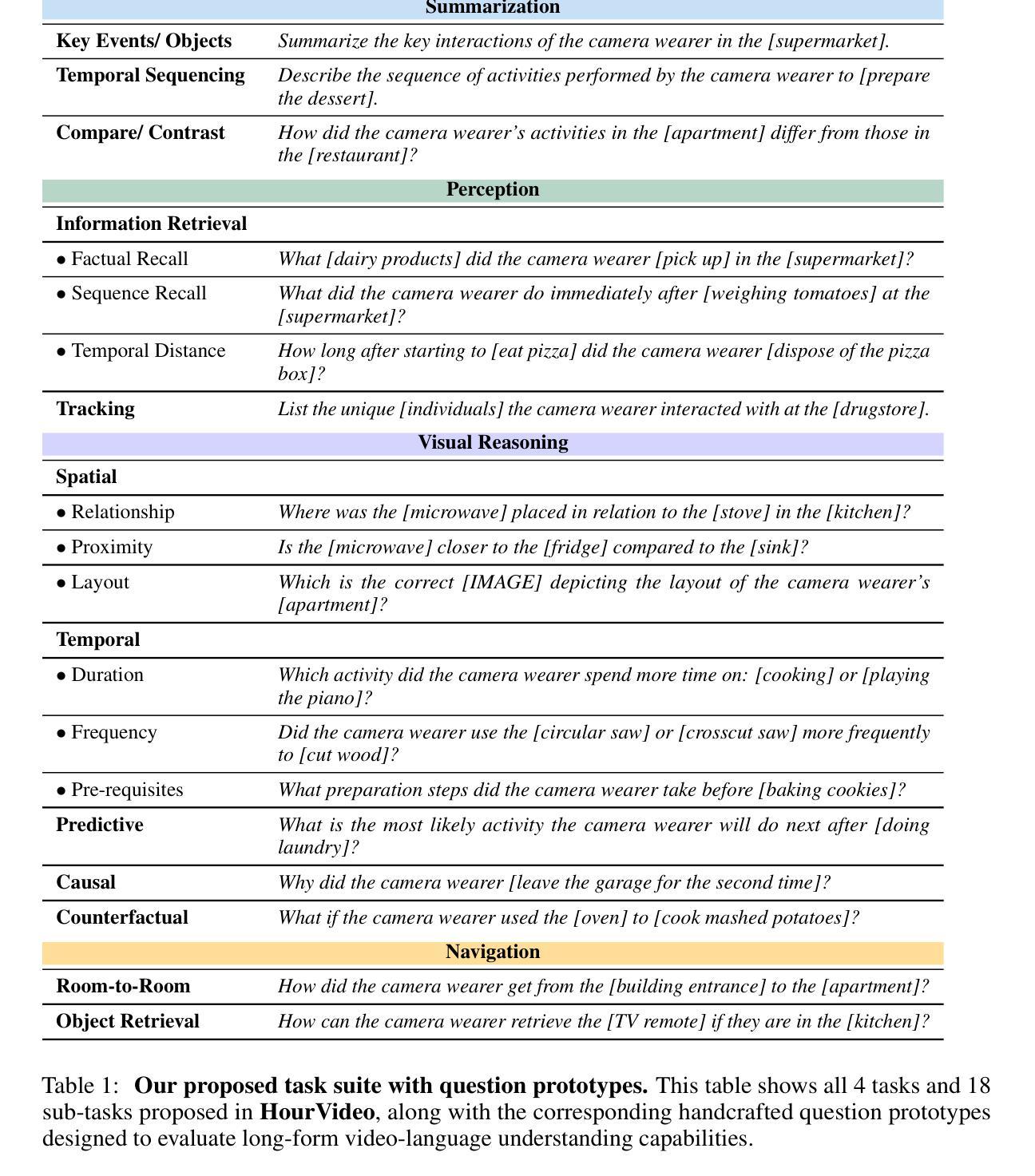
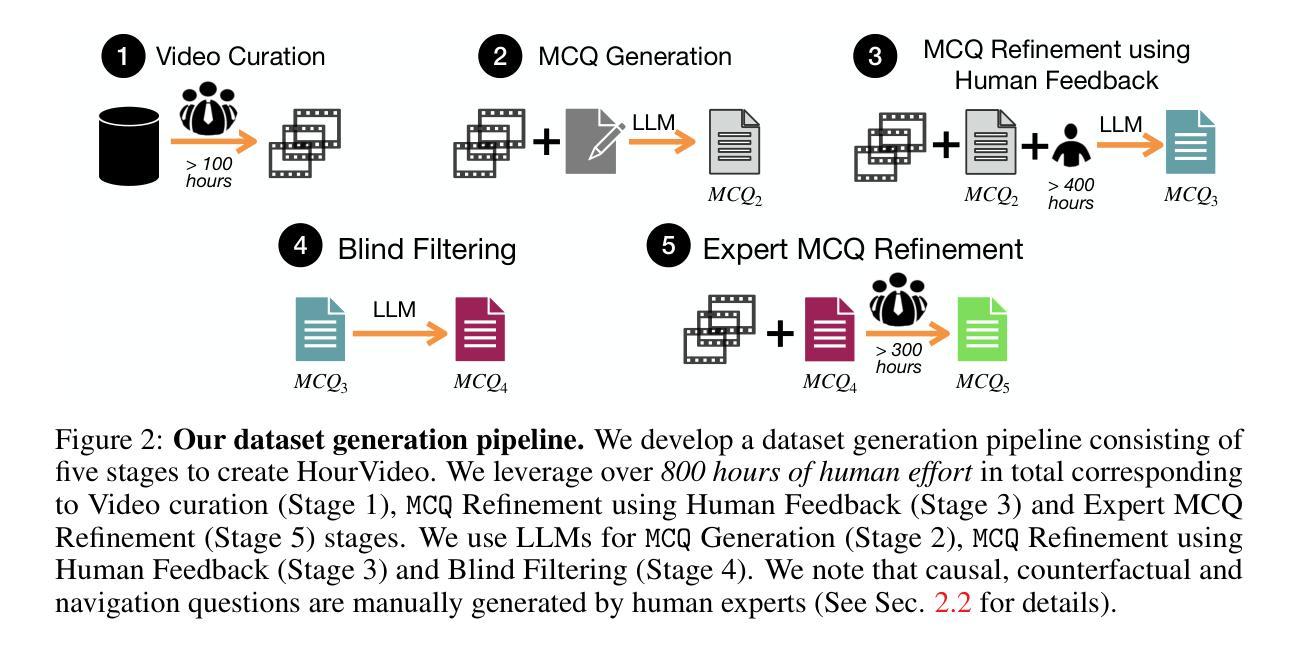
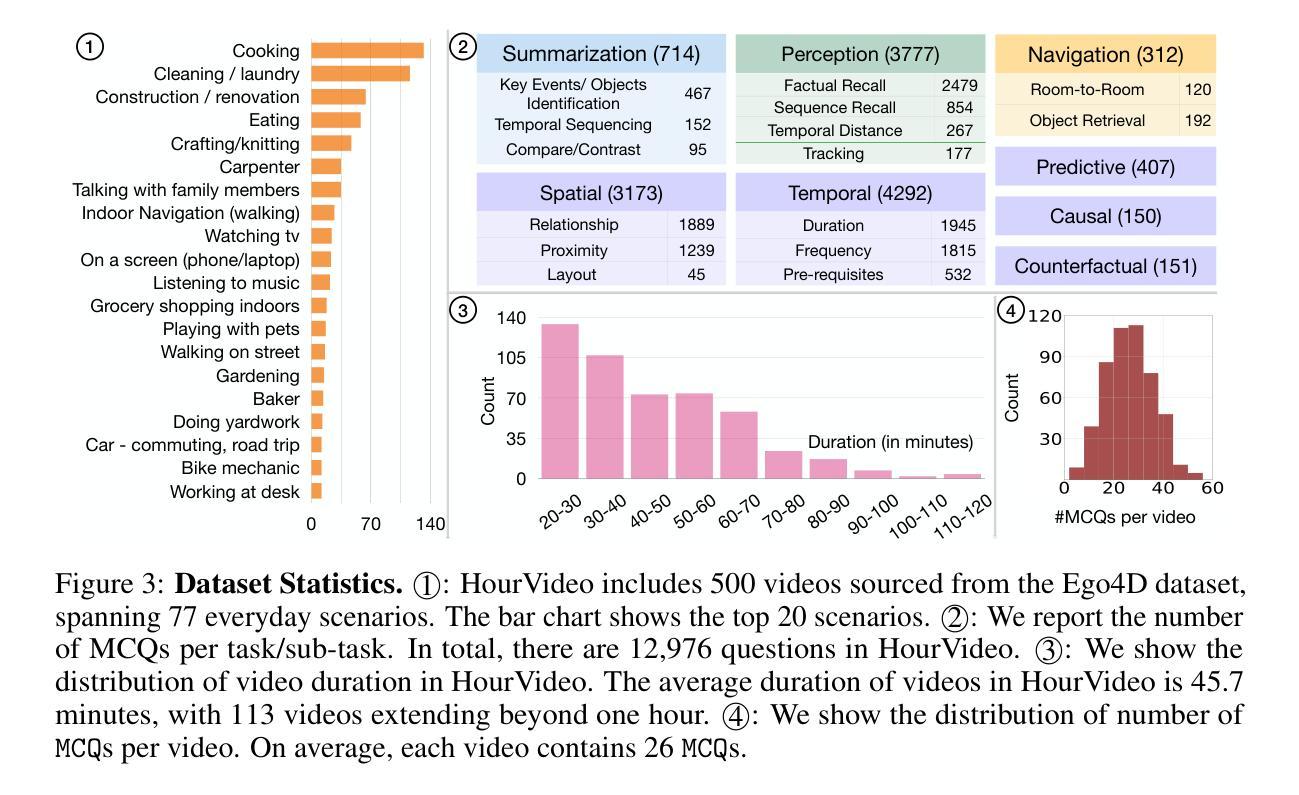
StreamingBench: Assessing the Gap for MLLMs to Achieve Streaming Video Understanding
Authors:Junming Lin, Zheng Fang, Chi Chen, Zihao Wan, Fuwen Luo, Peng Li, Yang Liu, Maosong Sun
The rapid development of Multimodal Large Language Models (MLLMs) has expanded their capabilities from image comprehension to video understanding. However, most of these MLLMs focus primarily on offline video comprehension, necessitating extensive processing of all video frames before any queries can be made. This presents a significant gap compared to the human ability to watch, listen, think, and respond to streaming inputs in real time, highlighting the limitations of current MLLMs. In this paper, we introduce StreamingBench, the first comprehensive benchmark designed to evaluate the streaming video understanding capabilities of MLLMs. StreamingBench assesses three core aspects of streaming video understanding: (1) real-time visual understanding, (2) omni-source understanding, and (3) contextual understanding. The benchmark consists of 18 tasks, featuring 900 videos and 4,500 human-curated QA pairs. Each video features five questions presented at different time points to simulate a continuous streaming scenario. We conduct experiments on StreamingBench with 13 open-source and proprietary MLLMs and find that even the most advanced proprietary MLLMs like Gemini 1.5 Pro and GPT-4o perform significantly below human-level streaming video understanding capabilities. We hope our work can facilitate further advancements for MLLMs, empowering them to approach human-level video comprehension and interaction in more realistic scenarios.
随着多模态大型语言模型(MLLMs)的快速发展,其能力已从图像理解扩展到视频理解。然而,大多数MLLM主要关注离线视频理解,需要在提出任何查询之前对所有视频帧进行大量处理。这与人类实时观看、聆听、思考和回应流媒体输入的能力相比存在显著差距,突显了当前MLLM的局限性。在本文中,我们介绍了StreamingBench,这是第一个旨在评估MLLM的流媒体视频理解能力的综合基准测试。StreamingBench评估流媒体理解的三个核心方面:(1)实时视觉理解、(2)全源理解和(3)上下文理解。该基准测试包含18项任务,以900个视频和4500个人工策划的问答对为特色。每个视频都在不同的时间点呈现五个问题,以模拟连续的流媒体场景。我们在StreamingBench上进行了实验,使用了包括开源和专有在内的共13种MLLM,发现最先进的专有MLLM如Gemini 1.5 Pro和GPT-4o在流媒体视频理解能力方面与人类水平存在显著差异。我们希望这项工作能够促进MLLM的进一步发展,使其能够在更现实的场景中接近人类水平的视频理解和交互能力。
论文及项目相关链接
Summary
随着多模态大型语言模型(MLLMs)的快速发展,其能力已从图像理解扩展到视频理解。然而,大多数MLLMs主要关注离线视频理解,在提出任何查询之前需要对所有视频帧进行大量处理,这与人能够实时观看、聆听、思考和回应流媒体输入的能力相比存在显著差距。本文介绍了StreamingBench,这是第一个旨在评估MLLMs流媒体视频理解能力的综合基准测试。StreamingBench评估流媒体视频理解的核心三个方面:实时视觉理解、全源理解和上下文理解。该基准测试包含18个任务,涉及900个视频和4500个人工制作的问答对。每个视频在不同时间点呈现五个问题,以模拟连续流媒体场景。在StreamingBench上进行的实验表明,即使是最先进的专有MLLMs,如Gemini 1.5 Pro和GPT-4o,在流媒体视频理解方面的表现也远远低于人类水平。我们希望通过这项工作促进MLLMs的进一步发展,使其能够在更现实的场景中实现接近人类水平的视频理解和交互。
Key Takeaways
- 多模态大型语言模型(MLLMs)已能从图像理解扩展到视频理解。
- 当前的MLLMs主要关注离线视频理解,并需预先处理所有视频帧,这与人类实时处理流媒体的能力存在差距。
- StreamingBench是首个评估MLLMs在流媒体视频理解方面的能力的综合基准测试。
- StreamingBench评估了流媒体视频理解的三个核心方面:实时视觉理解、全源理解和上下文理解。
- 实验显示,即使是最先进的MLLMs,在流媒体视频理解方面的表现也远低于人类水平。
- StreamingBench包含18个任务,900个视频和4500个人工制作的问答对,以模拟连续流媒体场景。
点此查看论文截图
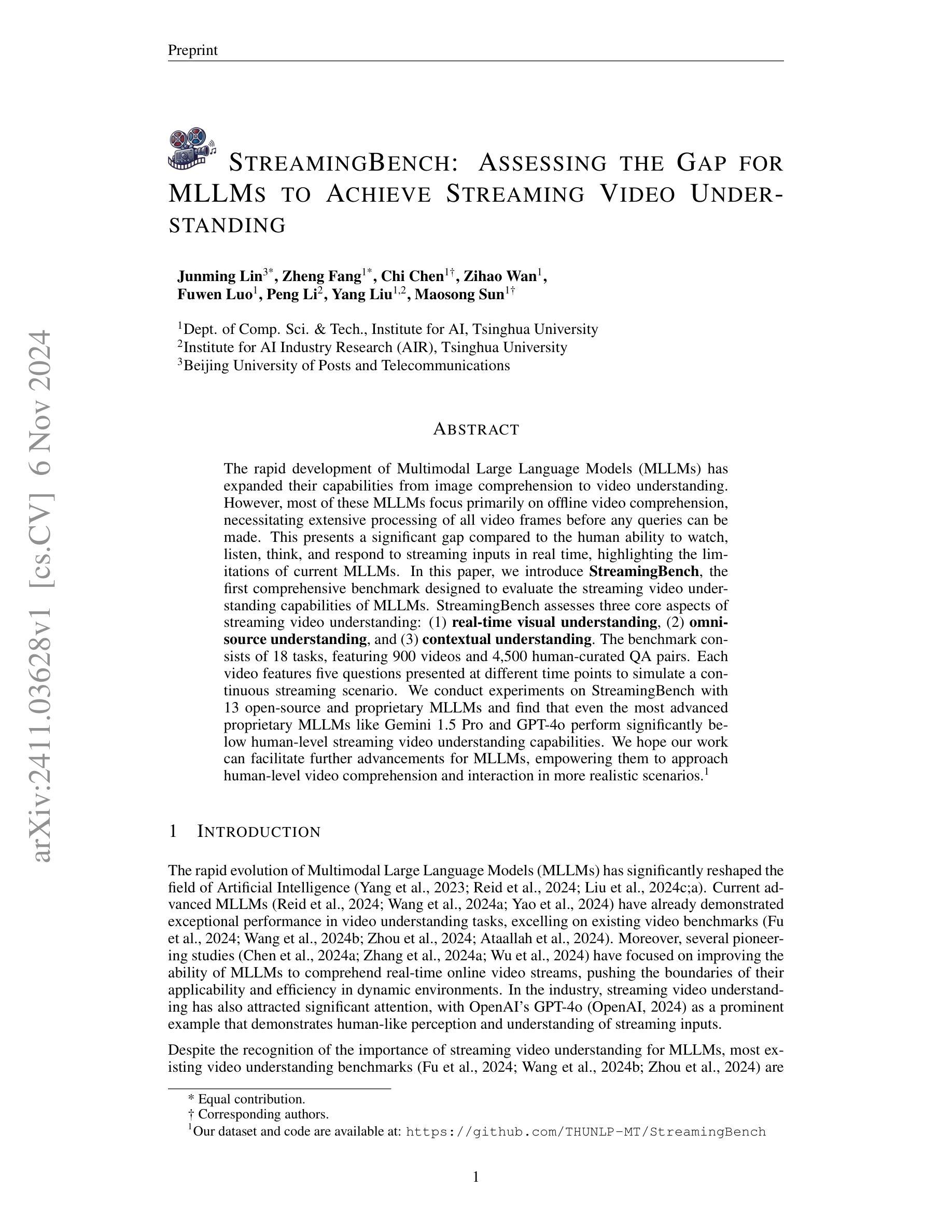
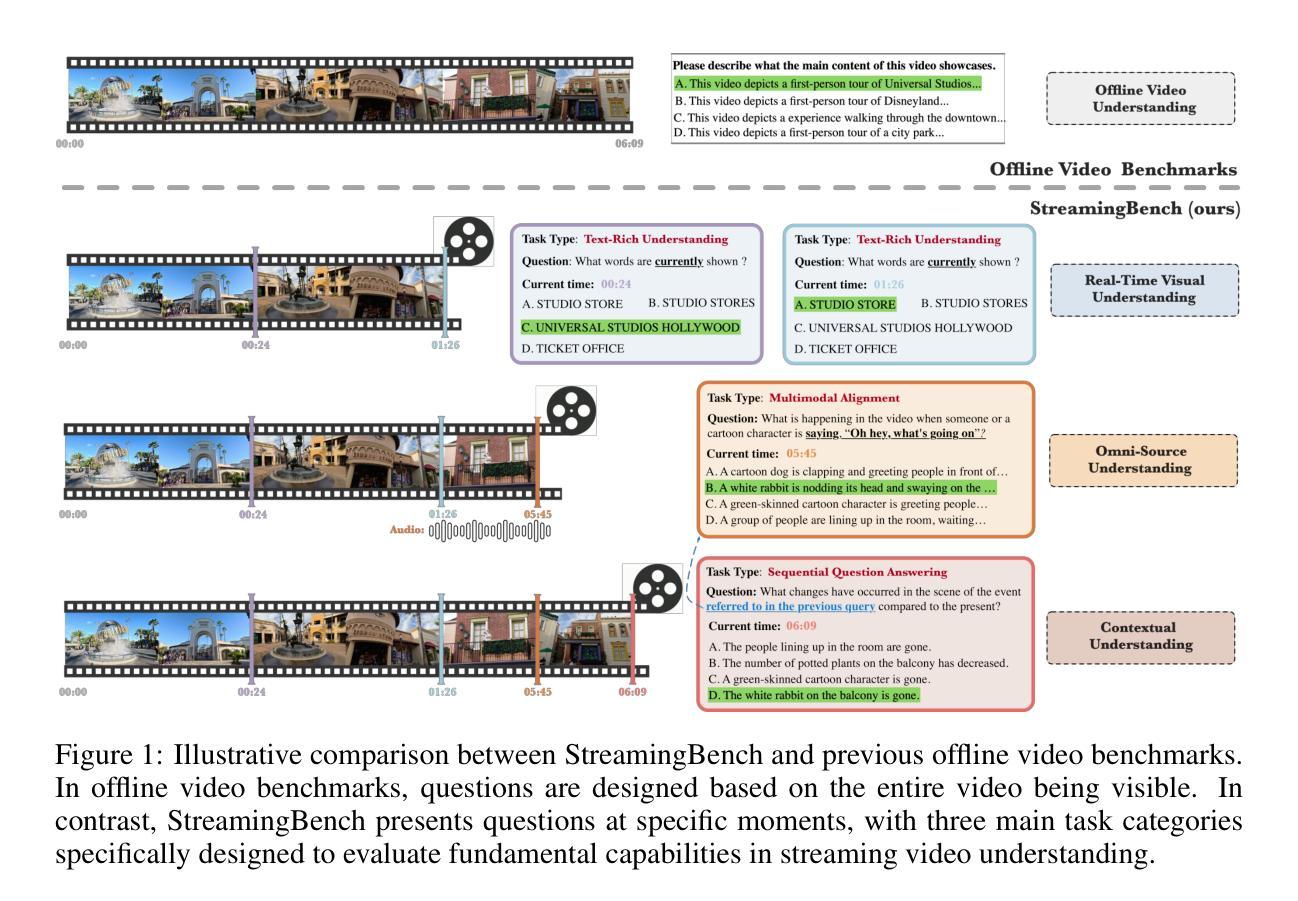
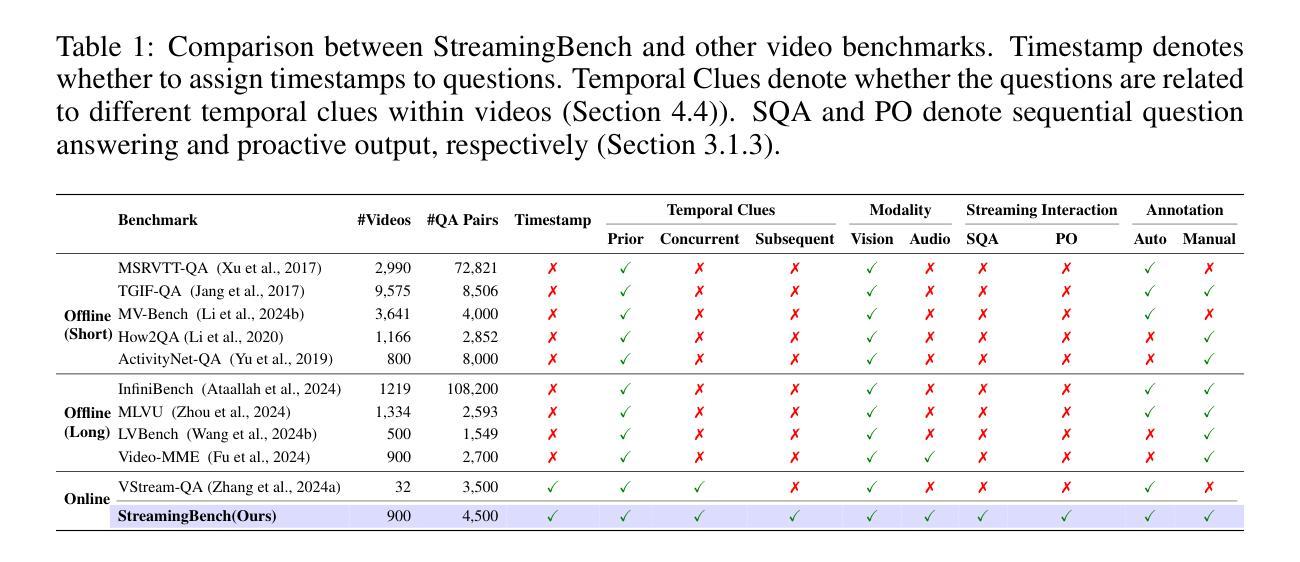
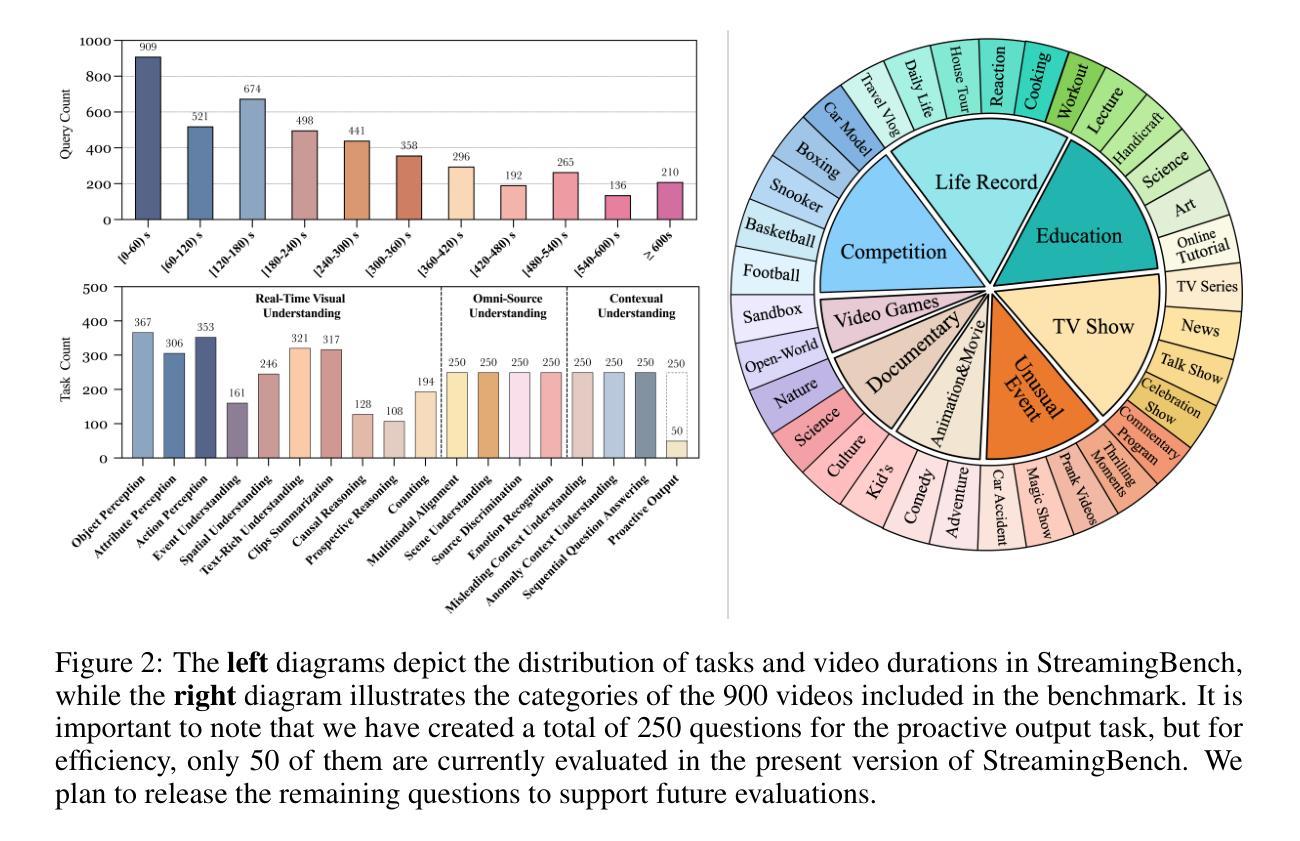
Personalized Video Summarization by Multimodal Video Understanding
Authors:Brian Chen, Xiangyuan Zhao, Yingnan Zhu
Video summarization techniques have been proven to improve the overall user experience when it comes to accessing and comprehending video content. If the user’s preference is known, video summarization can identify significant information or relevant content from an input video, aiding them in obtaining the necessary information or determining their interest in watching the original video. Adapting video summarization to various types of video and user preferences requires significant training data and expensive human labeling. To facilitate such research, we proposed a new benchmark for video summarization that captures various user preferences. Also, we present a pipeline called Video Summarization with Language (VSL) for user-preferred video summarization that is based on pre-trained visual language models (VLMs) to avoid the need to train a video summarization system on a large training dataset. The pipeline takes both video and closed captioning as input and performs semantic analysis at the scene level by converting video frames into text. Subsequently, the user’s genre preference was used as the basis for selecting the pertinent textual scenes. The experimental results demonstrate that our proposed pipeline outperforms current state-of-the-art unsupervised video summarization models. We show that our method is more adaptable across different datasets compared to supervised query-based video summarization models. In the end, the runtime analysis demonstrates that our pipeline is more suitable for practical use when scaling up the number of user preferences and videos.
视频摘要技术已被证明在访问和理解视频内容时,能够提升整体用户体验。如果了解用户的偏好,视频摘要可以从输入的视频中识别出重要信息或相关内容,帮助用户获得必要的信息或确定他们对原始视频的兴趣。适应各种类型视频和用户偏好的视频摘要需要大量的训练数据和昂贵的人工标注。为了推动相关研究,我们为视频摘要提出了一个新的基准测试,该基准测试能够捕捉各种用户偏好。此外,我们提出了一种基于预训练视觉语言模型的用户偏好视频摘要管道(Video Summarization with Language (VSL))。该管道避免了需要在大量训练数据集上训练视频摘要系统的需求,以视频和字幕作为输入,通过将视频帧转换为文本,在场景级别进行语义分析。然后,使用用户的类型偏好作为选择相关文本场景的基础。实验结果表明,我们提出的管道优于当前最先进的无监督视频摘要模型。我们证明,我们的方法在不同的数据集上比监督查询基于的视频摘要模型更具适应性。最后,运行时间分析表明,当扩大用户偏好和视频数量时,我们的管道更适合实际应用。
论文及项目相关链接
PDF In Proceedings of CIKM 2024 Applied Research Track
Summary
视频摘要技术能提升用户访问和理解视频内容的体验。通过识别用户偏好,视频摘要可从输入视频中识别重要信息或相关内容,帮助用户获取必要信息或决定是否需要观看原视频。为应对不同视频类型和用户需求,需大量训练数据和昂贵的人力标注。我们提出了新的视频摘要基准测试,反映各种用户偏好,并提出基于预训练视觉语言模型的VSL管道,无需在大训练数据集上训练视频摘要系统。管道同时处理视频和字幕,通过场景级别的语义分析将视频帧转换为文本,并根据用户喜好选择相关文本场景。实验证明,我们的管道性能优于当前先进的无监督视频摘要模型,且比监督查询型视频摘要模型更适用于不同数据集。运行分析表明,在用户偏好和视频数量增加时,我们的管道更适合实际应用。
Key Takeaways
- 视频摘要技术可以增强用户理解和体验视频内容的效果。
- 通过识别用户偏好,可以更有效地从视频中提取关键信息。
- 适应不同类型的视频和用户需求的视频摘要需要大规模的训练数据和标注。
- 提出了一种新的视频摘要基准测试,反映不同的用户偏好。
- 引入VSL管道,利用预训练的视觉语言模型进行用户偏好的视频摘要。
- VSL管道通过场景级别的语义分析处理视频和字幕。
点此查看论文截图
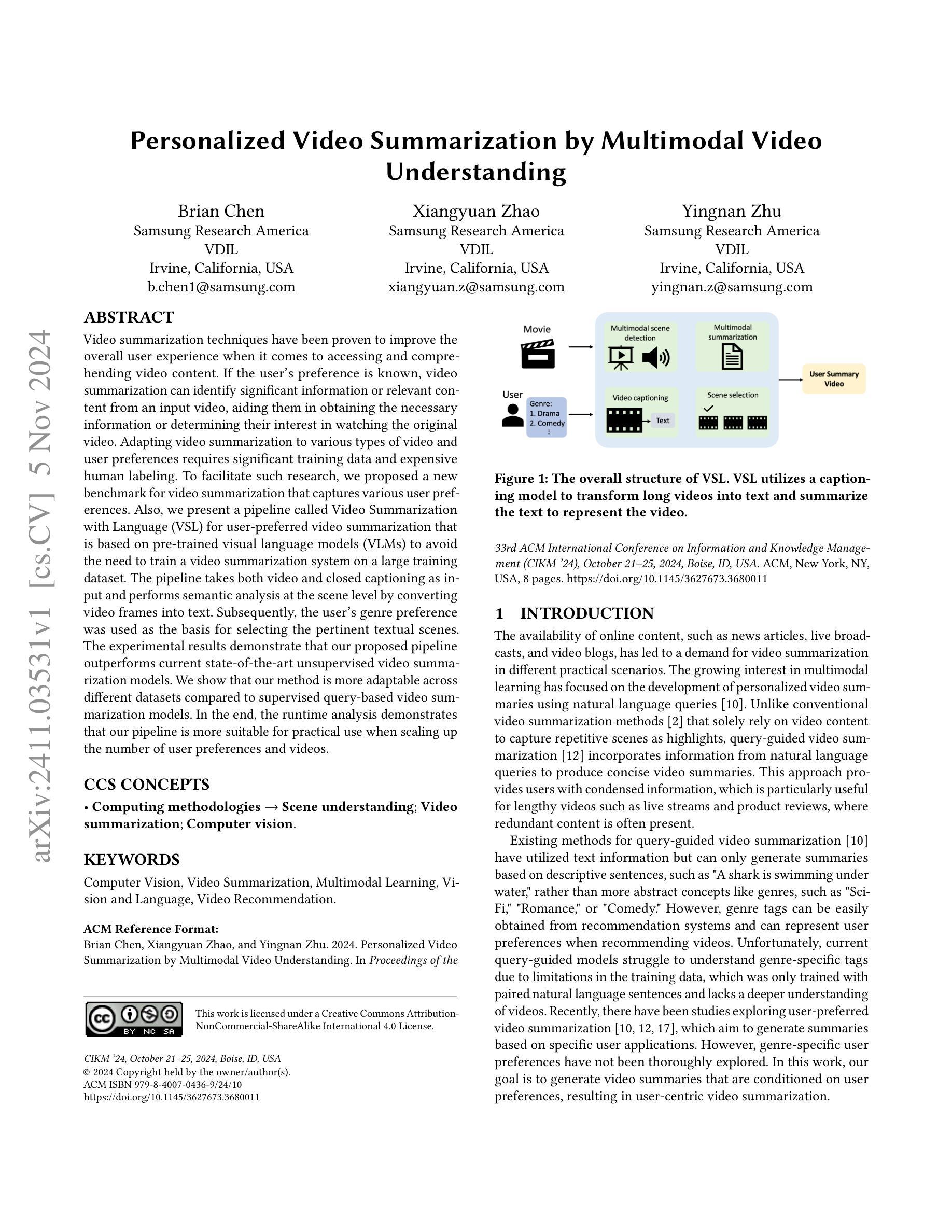
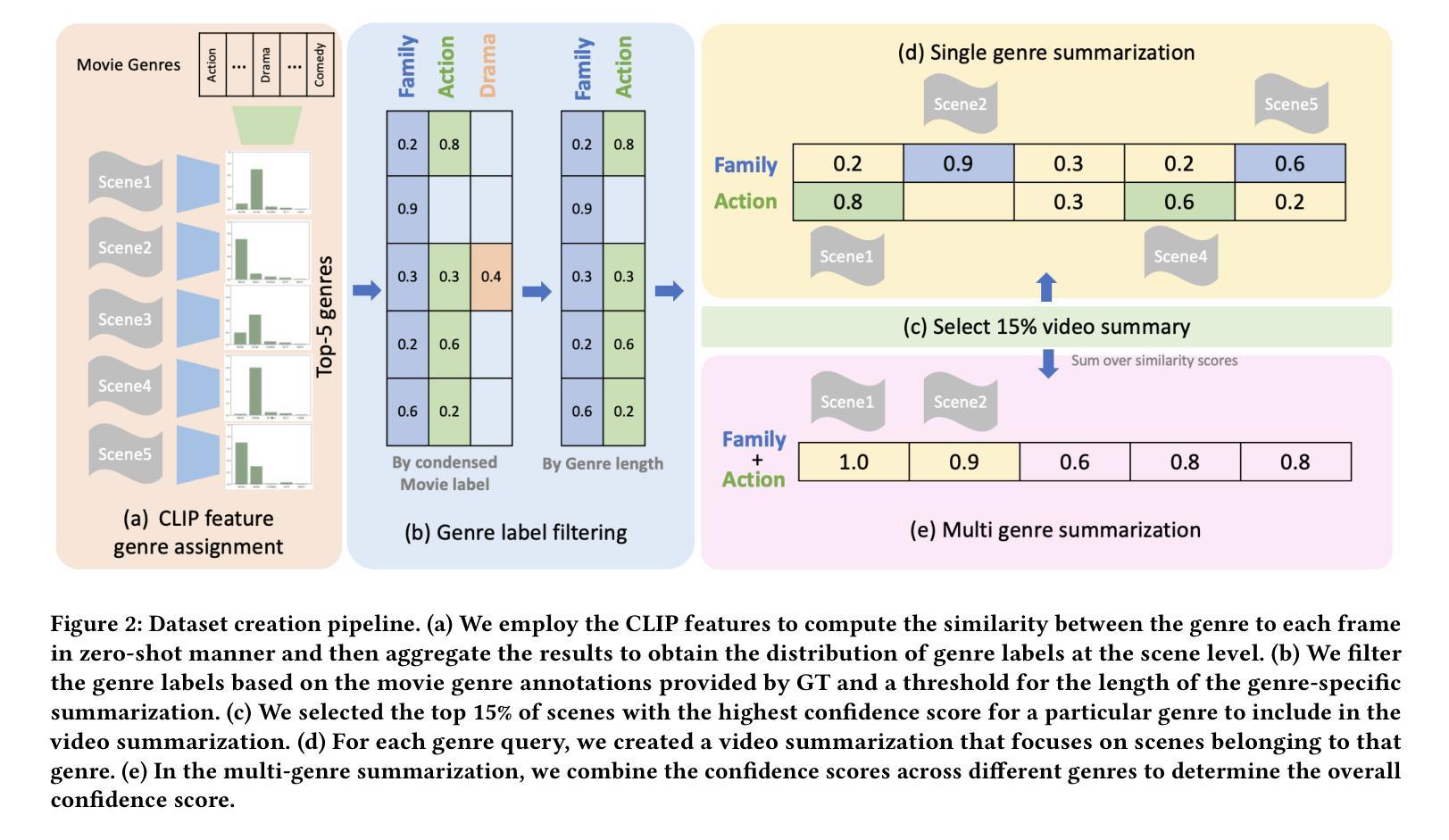
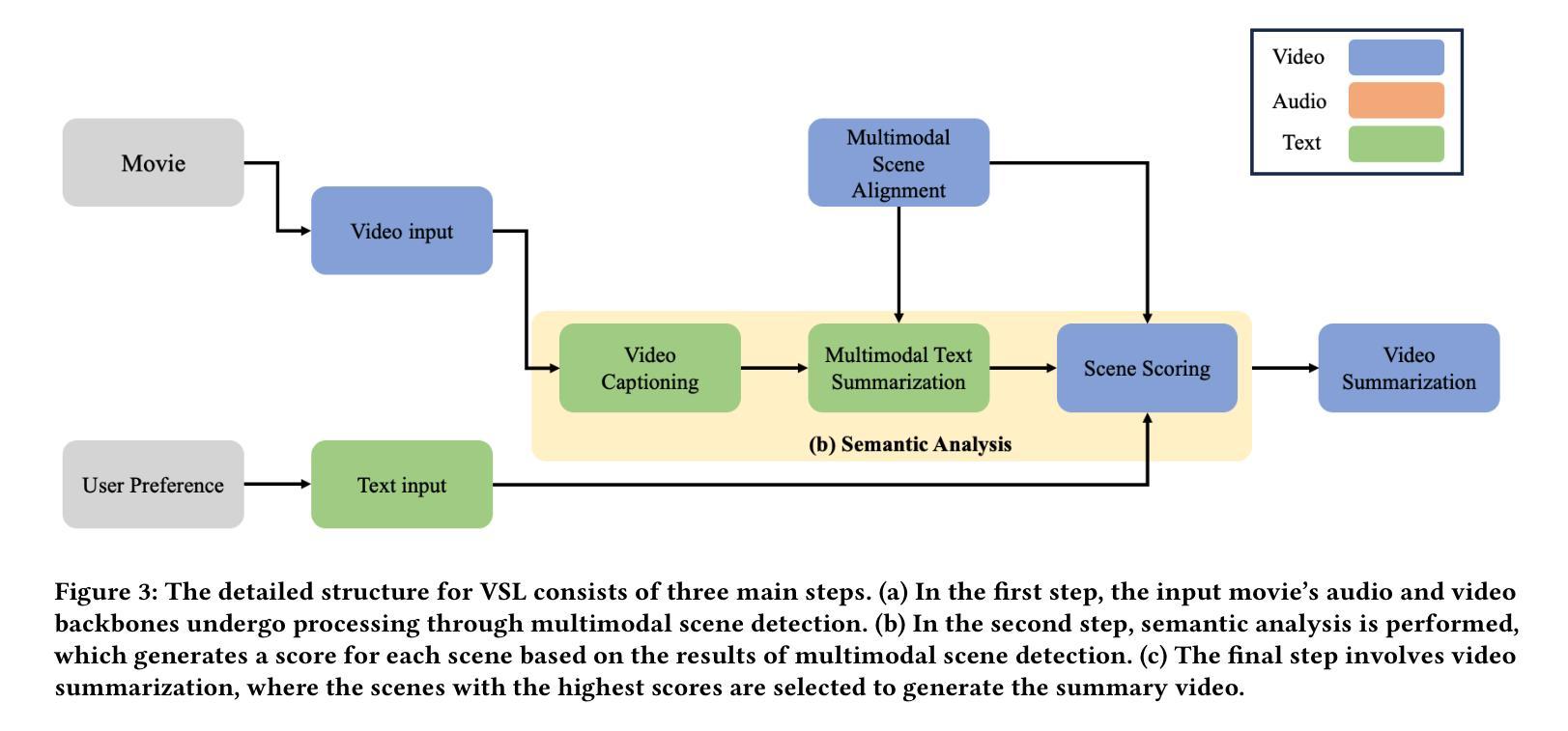
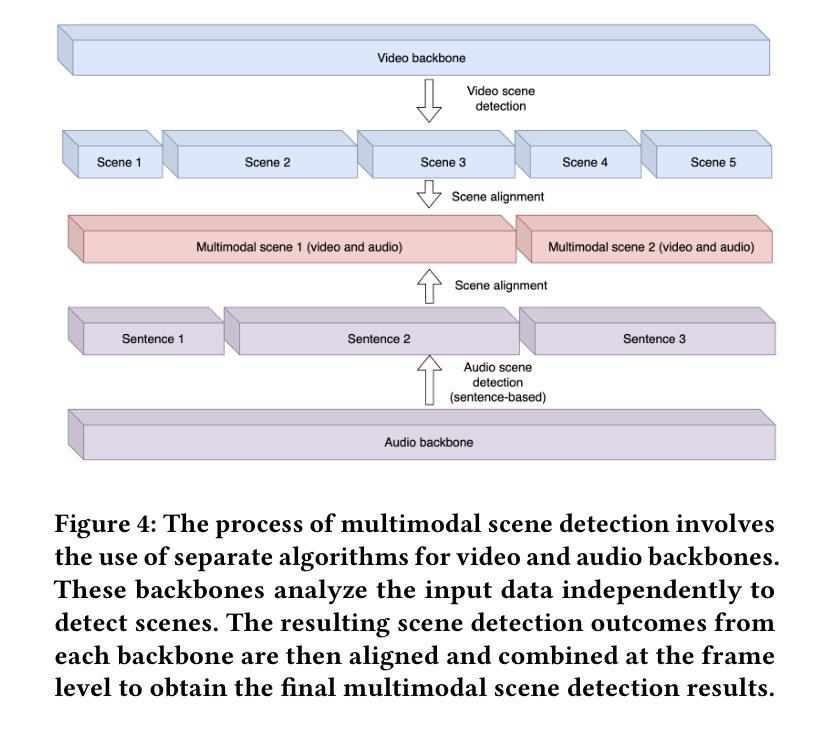
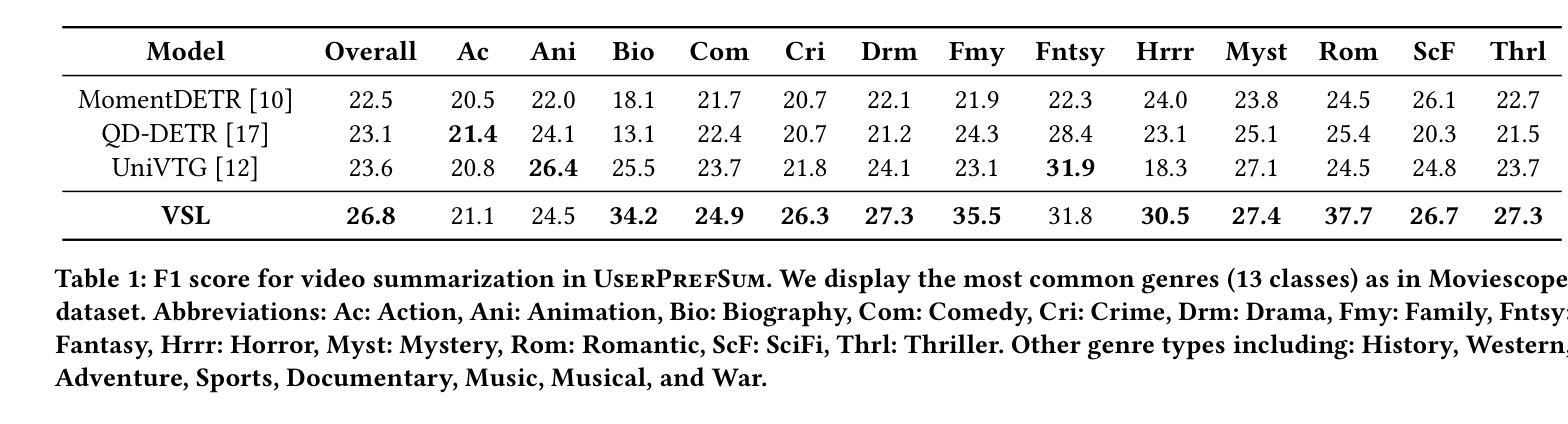
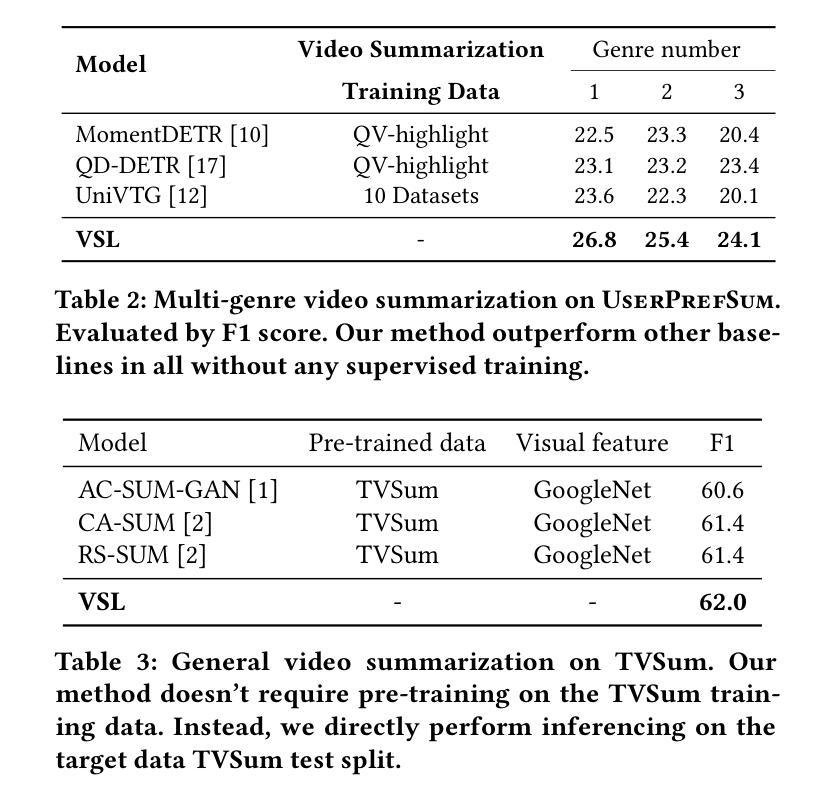
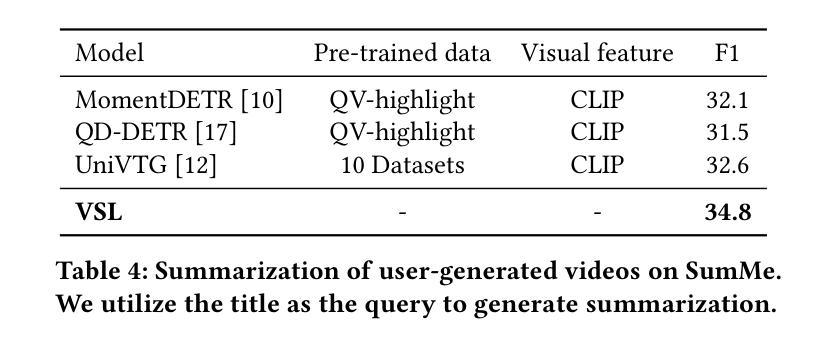
PPLLaVA: Varied Video Sequence Understanding With Prompt Guidance
Authors:Ruyang Liu, Haoran Tang, Haibo Liu, Yixiao Ge, Ying Shan, Chen Li, Jiankun Yang
The past year has witnessed the significant advancement of video-based large language models. However, the challenge of developing a unified model for both short and long video understanding remains unresolved. Most existing video LLMs cannot handle hour-long videos, while methods custom for long videos tend to be ineffective for shorter videos and images. In this paper, we identify the key issue as the redundant content in videos. To address this, we propose a novel pooling strategy that simultaneously achieves token compression and instruction-aware visual feature aggregation. Our model is termed Prompt-guided Pooling LLaVA, or PPLLaVA for short. Specifically, PPLLaVA consists of three core components: the CLIP-based visual-prompt alignment that extracts visual information relevant to the user’s instructions, the prompt-guided pooling that compresses the visual sequence to arbitrary scales using convolution-style pooling, and the clip context extension designed for lengthy prompt common in visual dialogue. Moreover, our codebase also integrates the most advanced video Direct Preference Optimization (DPO) and visual interleave training. Extensive experiments have validated the performance of our model. With superior throughput and only 1024 visual context, PPLLaVA achieves better results on image benchmarks as a video LLM, while achieving state-of-the-art performance across various video benchmarks, excelling in tasks ranging from caption generation to multiple-choice questions, and handling video lengths from seconds to hours. Codes have been available at https://github.com/farewellthree/PPLLaVA.
过去一年,基于视频的的大型语言模型取得了显著进展。然而,开发一种同时适用于短视频和长视频理解的统一模型仍然是一个挑战。大多数现有的视频LLM无法处理长达一小时的视频,而针对长视频的定制方法对于短视频和图像则往往效果不佳。在本文中,我们将关键问题确定为视频中的冗余内容。为了解决这一问题,我们提出了一种新的池化策略,可以同时实现令牌压缩和指令感知的视觉特征聚合。我们的模型被称为Prompt引导池化LLaVA,简称PPLLaVA。具体来说,PPLLaVA包括三个核心组件:基于CLIP的视觉提示对齐,用于提取与用户指令相关的视觉信息;提示引导池化,使用卷积式池化将视觉序列压缩到任意规模;以及针对视觉对话中常见冗长提示设计的CLIP上下文扩展。此外,我们的代码库还集成了最先进的视频直接偏好优化(DPO)和视觉交替训练。大量实验验证了模型性能。PPLLaVA具有出色的吞吐量和仅使用1024个视觉上下文,作为视频LLM在图像基准测试中取得了更好的结果,同时在各种视频基准测试中实现了最先进的性能,擅长从生成字幕到多项选择题的各种任务,并能处理从几秒到几小时的视频长度。代码可通过https://github.com/farewellthree/PPLLaVA获取。
论文及项目相关链接
Summary
本文提出一种针对视频理解的新型统一模型PPLLaVA,通过识别视频冗余内容的问题并引入新的池化策略,实现了对长短视频的全面理解。该模型包含三个核心组件:基于CLIP的视觉提示对齐、引导式池化和用于视频对话的剪辑上下文扩展。此外,它结合了最新的视频直接偏好优化和视觉交替训练技术,实验结果显示其性能优越,仅使用少量视觉上下文就能在处理视频长短不同任务上达到顶尖表现。
Key Takeaways
- 视频理解的现状和挑战:当前视频大语言模型在长短视频理解上存在统一性问题。大多数模型无法处理长时间视频,而针对长视频的特定方法对于短视频和图像则效果不佳。
- 核心问题识别:视频冗余内容是导致模型表现不佳的关键问题。
- PPLLaVA模型及其组件:介绍新型模型PPLLaVA及其三个核心组件——基于CLIP的视觉提示对齐、引导式池化和用于视频对话的剪辑上下文扩展。
- 模型性能优化策略:结合最新的视频直接偏好优化和视觉交替训练技术来提升模型性能。
- 实验结果:PPLLaVA模型性能优越,能在仅使用少量视觉上下文的情况下,在处理长短不一的视频任务上达到顶尖表现。
- 模型可用性和代码共享:PPLLaVA模型的代码已公开分享在GitHub上。
点此查看论文截图
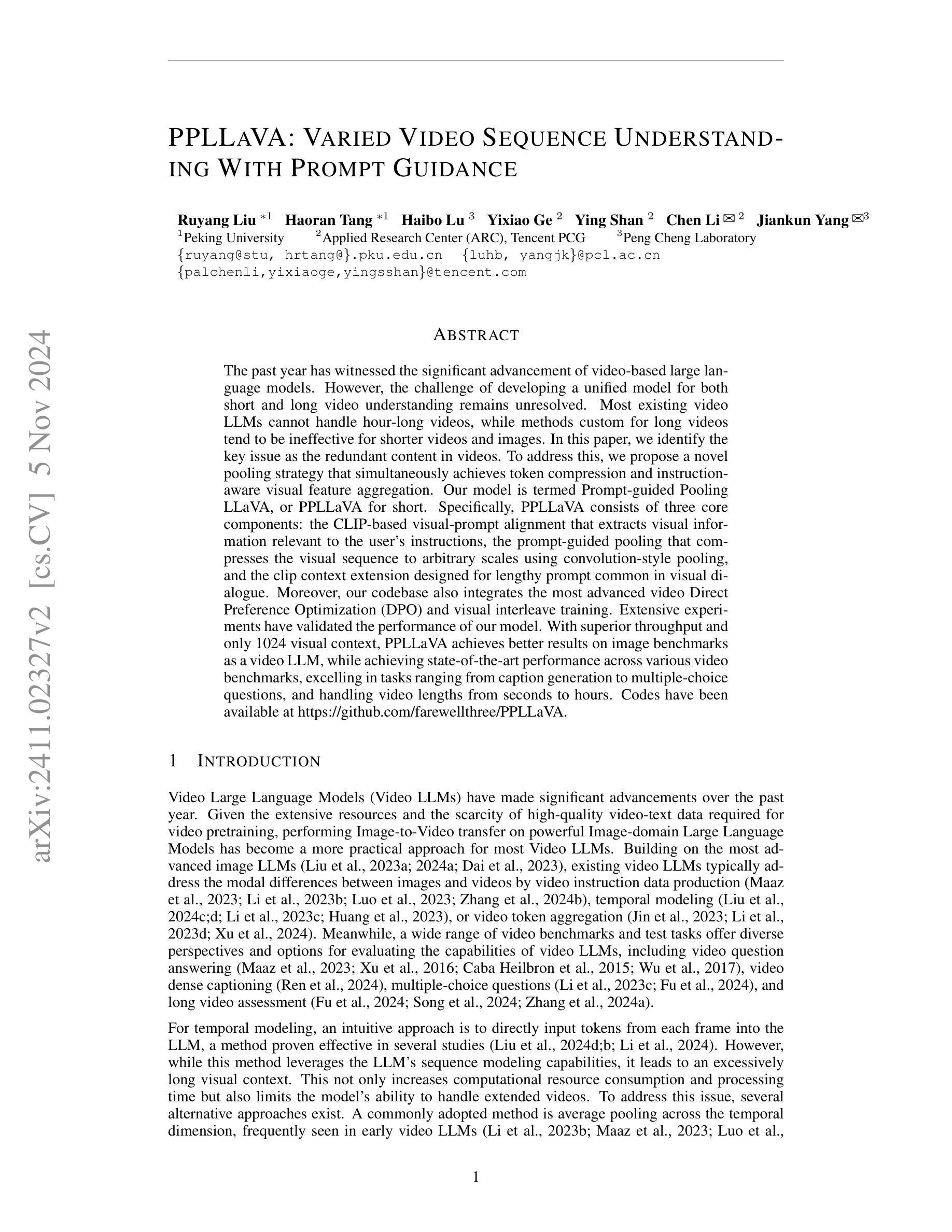
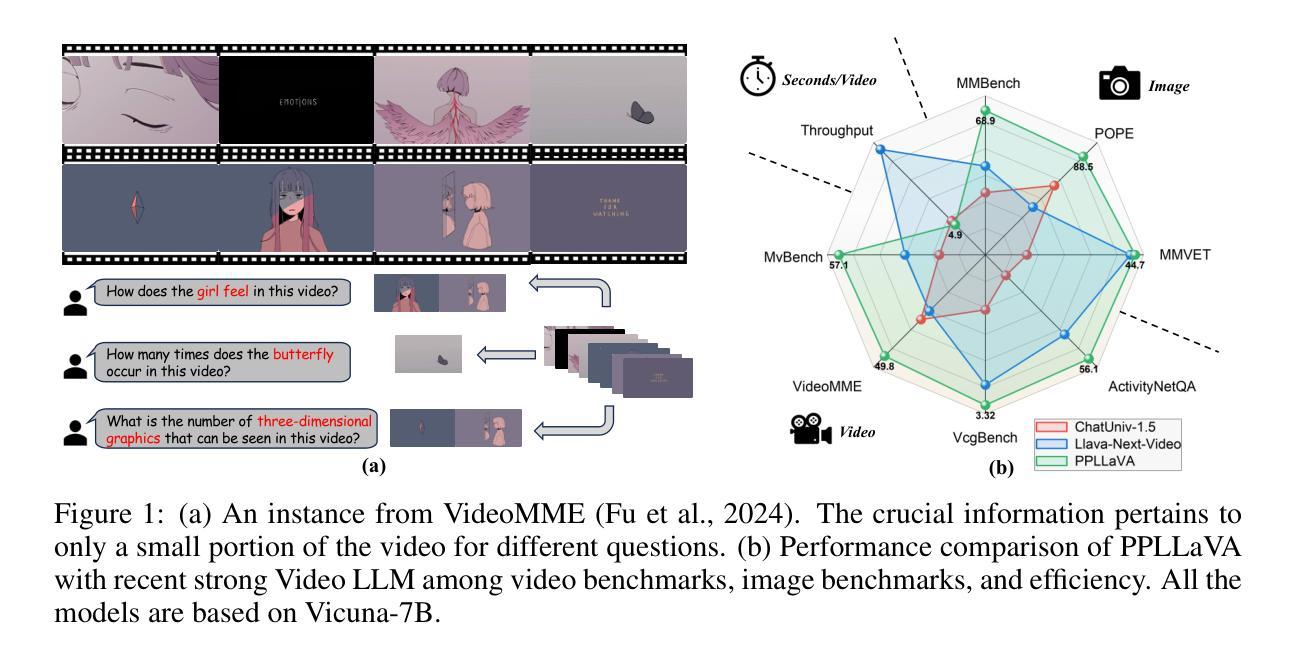
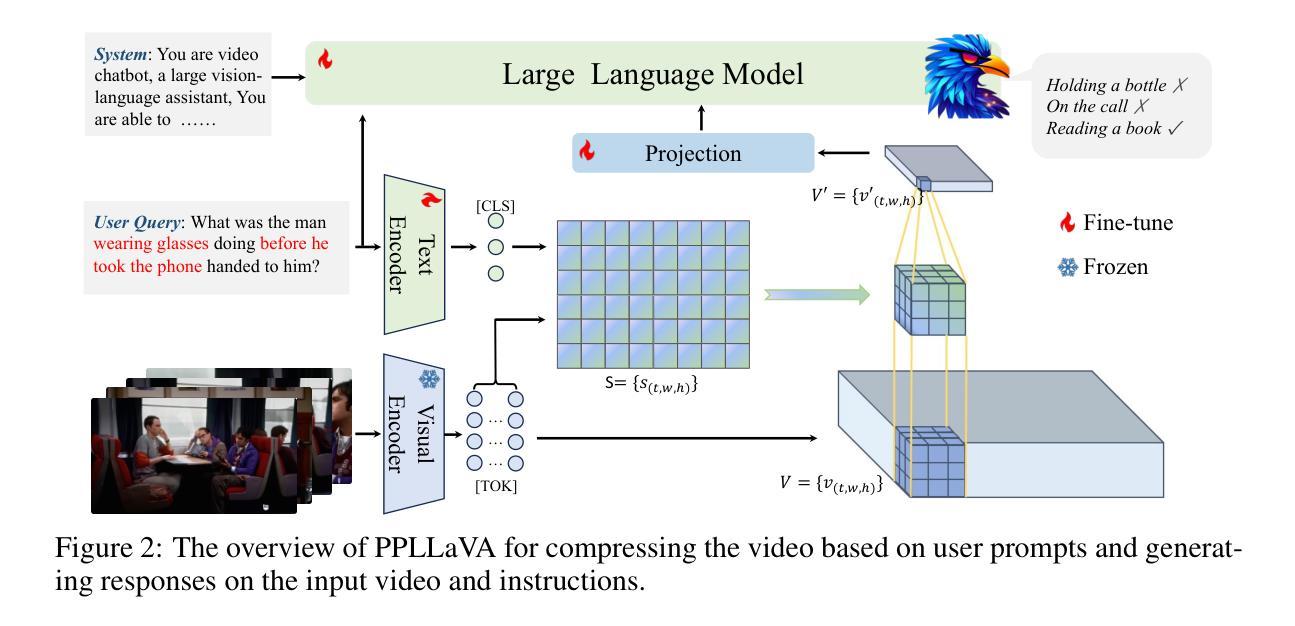
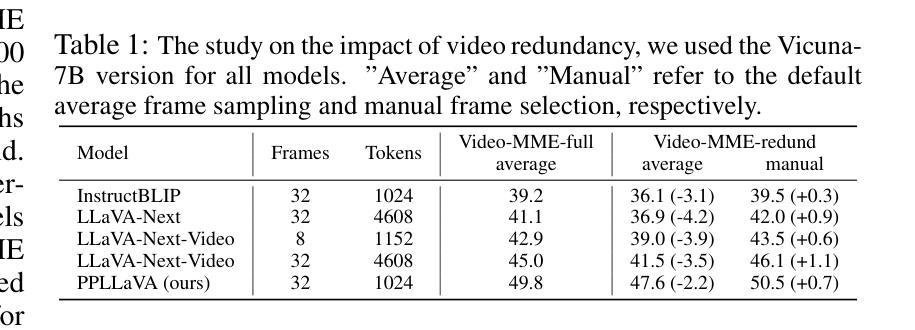
From Seconds to Hours: Reviewing MultiModal Large Language Models on Comprehensive Long Video Understanding
Authors:Heqing Zou, Tianze Luo, Guiyang Xie, Victor, Zhang, Fengmao Lv, Guangcong Wang, Junyang Chen, Zhuochen Wang, Hansheng Zhang, Huaijian Zhang
The integration of Large Language Models (LLMs) with visual encoders has recently shown promising performance in visual understanding tasks, leveraging their inherent capability to comprehend and generate human-like text for visual reasoning. Given the diverse nature of visual data, MultiModal Large Language Models (MM-LLMs) exhibit variations in model designing and training for understanding images, short videos, and long videos. Our paper focuses on the substantial differences and unique challenges posed by long video understanding compared to static image and short video understanding. Unlike static images, short videos encompass sequential frames with both spatial and within-event temporal information, while long videos consist of multiple events with between-event and long-term temporal information. In this survey, we aim to trace and summarize the advancements of MM-LLMs from image understanding to long video understanding. We review the differences among various visual understanding tasks and highlight the challenges in long video understanding, including more fine-grained spatiotemporal details, dynamic events, and long-term dependencies. We then provide a detailed summary of the advancements in MM-LLMs in terms of model design and training methodologies for understanding long videos. Finally, we compare the performance of existing MM-LLMs on video understanding benchmarks of various lengths and discuss potential future directions for MM-LLMs in long video understanding.
大型语言模型(LLM)与视觉编码器的结合在视觉理解任务中展现出了有前景的性能,利用它们理解和生成用于视觉推理的人类文本的能力。考虑到视觉数据的多样性,多模态大型语言模型(MM-LLM)在理解图像、短视频和长视频时,在模型设计和训练方面展现出差异。我们的论文重点关注长视频理解与静态图像和短视频理解相比存在的巨大差异和独特挑战。不同于静态图像,短视频包含具有空间和时间信息的连续帧,而长视频则由多个事件组成,具有事件间和长期的时间信息。在这篇综述中,我们旨在追踪并总结MM-LLM从图像理解到长视频理解的进展。我们回顾了不同视觉理解任务之间的差异,并强调了长视频理解中的挑战,包括更精细的时空细节、动态事件和长期依赖关系。然后,我们对MM-LLM在理解长视频方面的模型设计和训练方法的进展进行了详细总结。最后,我们比较了现有MM-LLM在各种长度的视频理解基准测试上的性能,并讨论了MM-LLM在未来长视频理解方面的潜在发展方向。
论文及项目相关链接
PDF 11 pages
Summary:
大型语言模型与视觉编码器的结合在视觉理解任务中展现出良好性能,尤其擅长利用模型固有的文本理解和生成能力进行视觉推理。针对长视频理解,本文重点探讨了与静态图像和短视频理解相比,长视频理解中的显著差异和独特挑战。长视频包含多个事件,涉及跨事件和长期时间信息。本文旨在回顾并总结多模态大型语言模型从图像理解到长视频理解的进展,同时介绍了设计模型和训练方法的最新进展。最后对现有模型的性能进行了对比,并探讨了未来多模态大型语言模型在长视频理解领域的发展方向。
Key Takeaways:
- 大型语言模型与视觉编码器的结合在视觉理解任务中表现良好。
- 长视频理解相较于静态图像和短视频理解存在显著差异和独特挑战。
- 长视频包含跨事件和长期时间信息。
- 多模态大型语言模型在视频理解方面取得进展,包括模型设计和训练方法的进步。
- 长视频理解需要更精细的时空细节、动态事件和长期依赖关系。
- 现有模型在长视频理解方面的性能有所差异,未来需要进一步提高模型的鲁棒性和效率。
点此查看论文截图
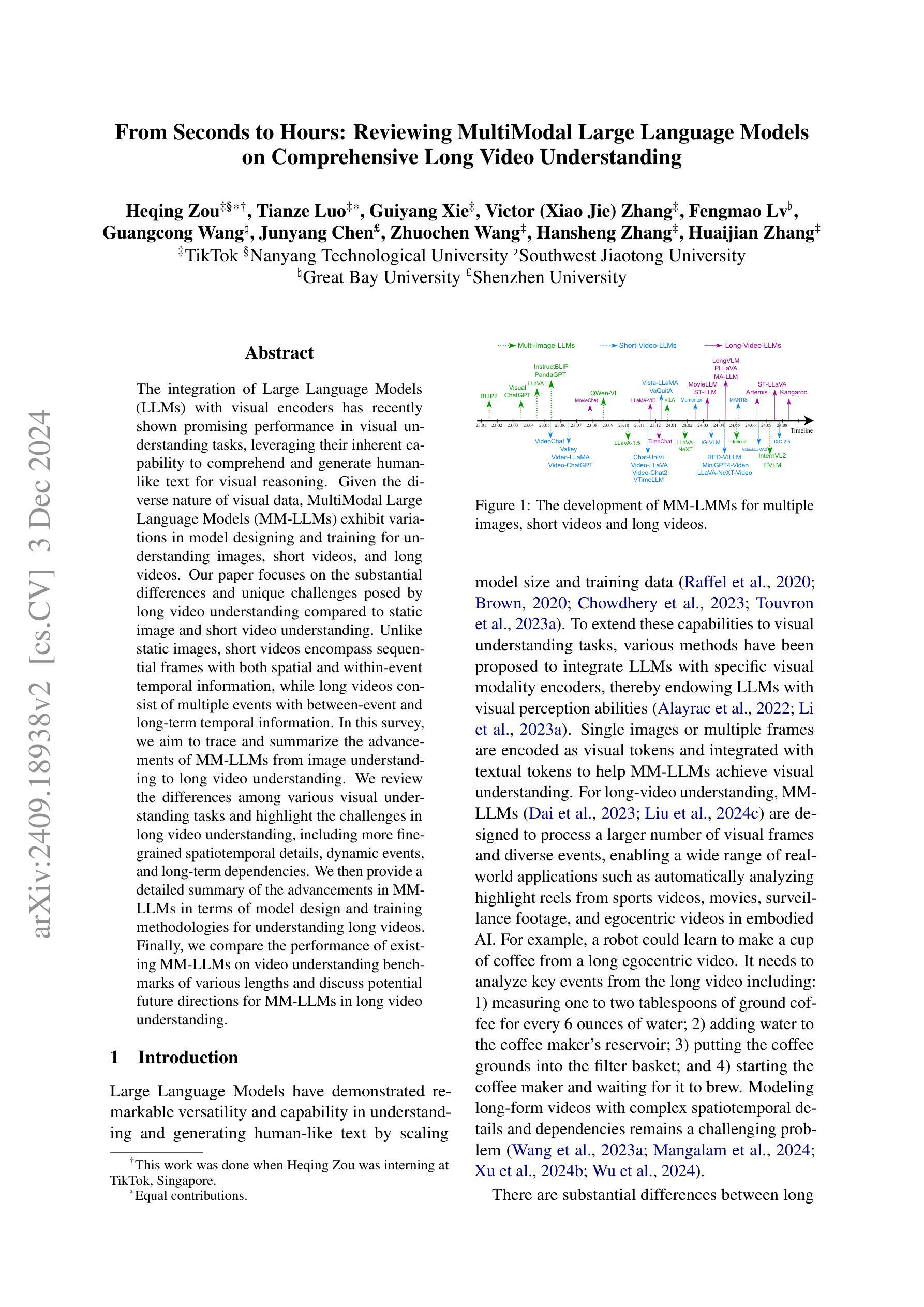
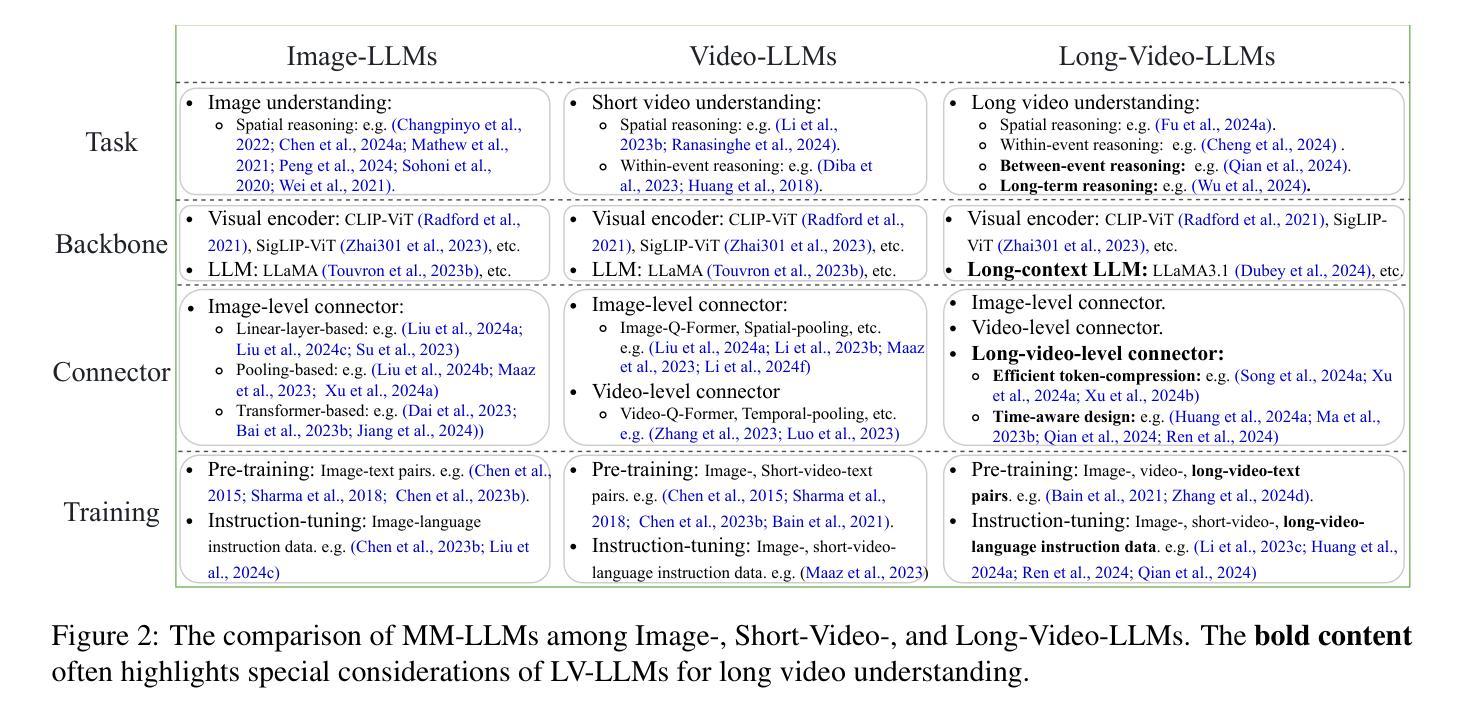
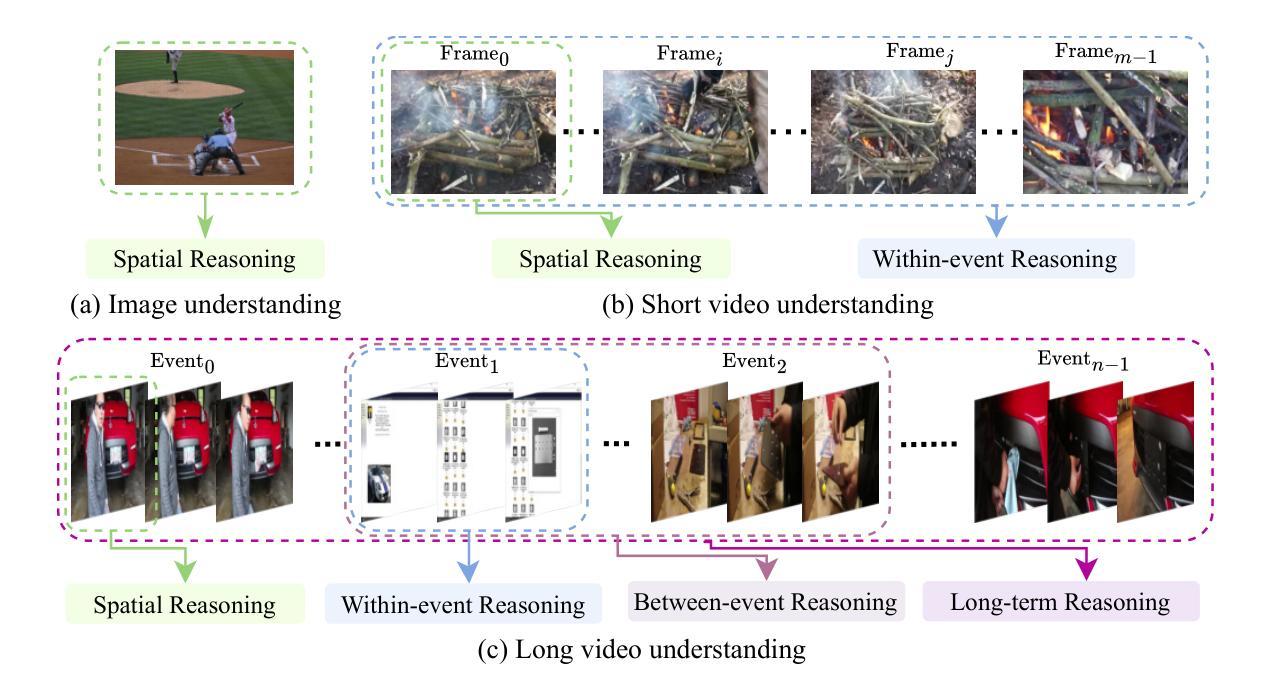
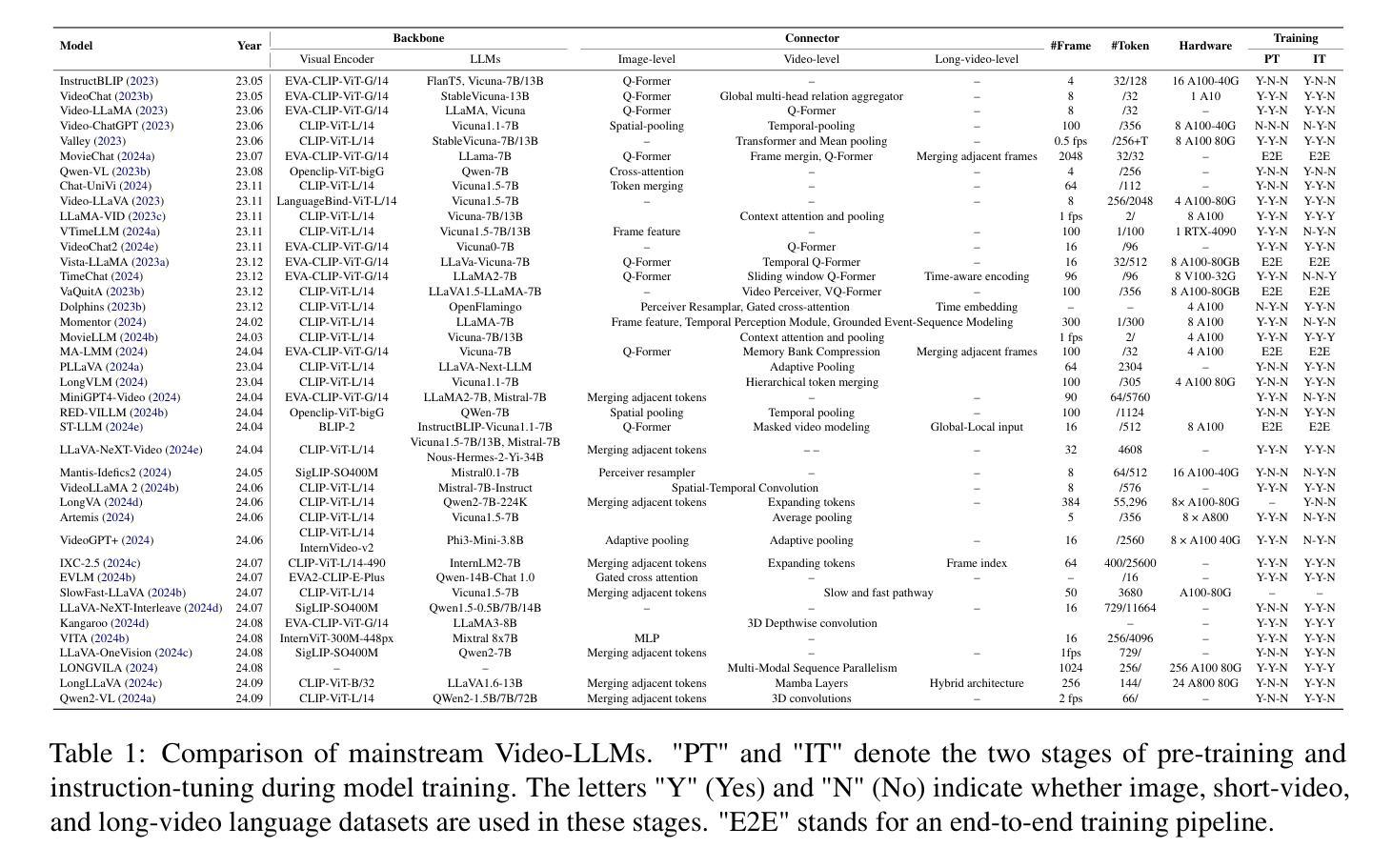
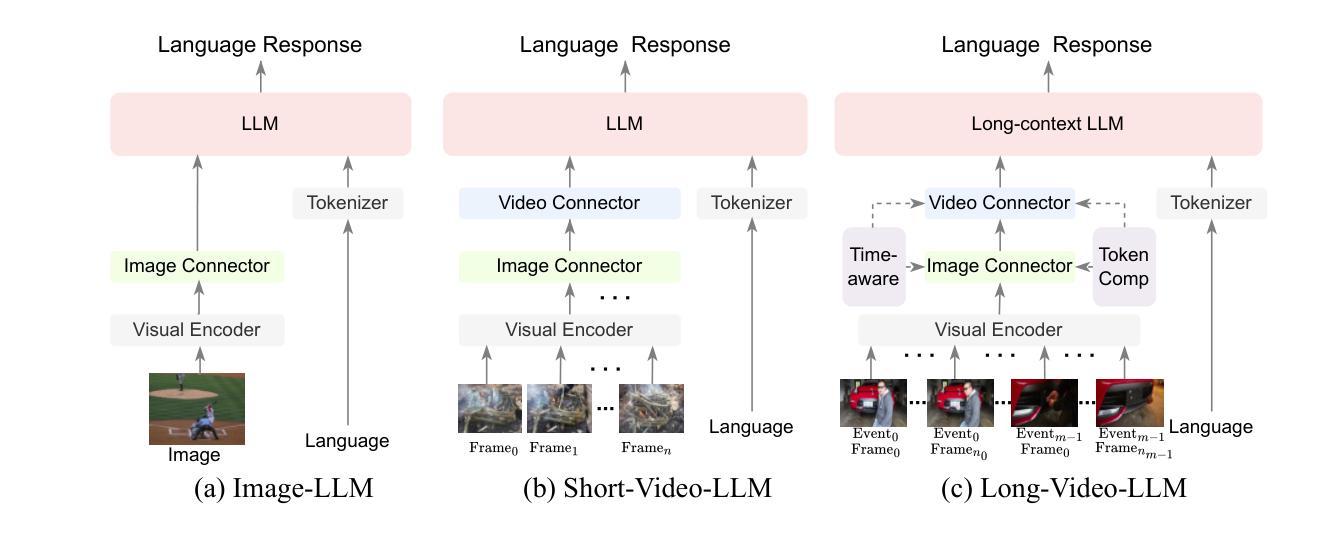
OmAgent: A Multi-modal Agent Framework for Complex Video Understanding with Task Divide-and-Conquer
Authors:Lu Zhang, Tiancheng Zhao, Heting Ying, Yibo Ma, Kyusong Lee
Recent advancements in Large Language Models (LLMs) have expanded their capabilities to multimodal contexts, including comprehensive video understanding. However, processing extensive videos such as 24-hour CCTV footage or full-length films presents significant challenges due to the vast data and processing demands. Traditional methods, like extracting key frames or converting frames to text, often result in substantial information loss. To address these shortcomings, we develop OmAgent, efficiently stores and retrieves relevant video frames for specific queries, preserving the detailed content of videos. Additionally, it features an Divide-and-Conquer Loop capable of autonomous reasoning, dynamically invoking APIs and tools to enhance query processing and accuracy. This approach ensures robust video understanding, significantly reducing information loss. Experimental results affirm OmAgent’s efficacy in handling various types of videos and complex tasks. Moreover, we have endowed it with greater autonomy and a robust tool-calling system, enabling it to accomplish even more intricate tasks.
近期大型语言模型(LLM)的进步已经将其能力扩展到了多模态上下文,包括全面的视频理解。然而,处理长达24小时的监控录像或全长电影等大量视频由于巨大的数据和处理需求而面临重大挑战。传统的方法,如提取关键帧或将帧转换为文本,通常会导致大量信息丢失。为了克服这些缺点,我们开发了OmAgent,它能有效地存储和检索与特定查询相关的视频帧,同时保留视频的详细内容。此外,它还具有一种分而治之循环能力,能够进行自主推理,动态调用API和工具以提高查询处理和准确性。这种方法确保了稳健的视频理解,显著减少了信息丢失。实验结果表明,OmAgent在处理各种视频和复杂任务方面的有效性。此外,我们还赋予了它更大的自主性和强大的工具调用系统,使其能够完成更复杂的任务。
论文及项目相关链接
Summary:随着大型语言模型(LLM)的最新进展,其在多模态上下文中的能力得到了扩展,包括对视频的综合理解。然而,处理长篇视频如24小时监控录像或全长电影时,由于大量数据和处理需求,存在巨大挑战。传统方法常常导致信息大量丢失。为解决这些问题,我们开发了OmAgent,它能高效存储和检索相关视频帧以应对特定查询,同时保留视频的详细内容。此外,它还具有一种分而治之循环能力,可自主推理、动态调用API和工具以提高查询处理和准确性。这种方法确保了稳健的视频理解,显著减少了信息丢失。实验结果表明,OmAgent在处理各种视频和复杂任务方面的有效性。我们还赋予它更大的自主性和强大的工具调用系统,使其能够完成更复杂的任务。
Key Takeaways:
- 大型语言模型(LLM)现在能够应用于视频理解。
- 处理长篇视频时存在巨大的挑战,传统方法常常导致信息丢失。
- OmAgent能够高效存储和检索相关视频帧以应对特定查询。
- OmAgent具有分而治之循环能力,可以自主推理并动态调用API和工具。
- 这种新的方法确保了稳健的视频理解,显著减少了信息丢失。
- 实验结果表明OmAgent在处理各种视频和复杂任务方面的有效性。
点此查看论文截图
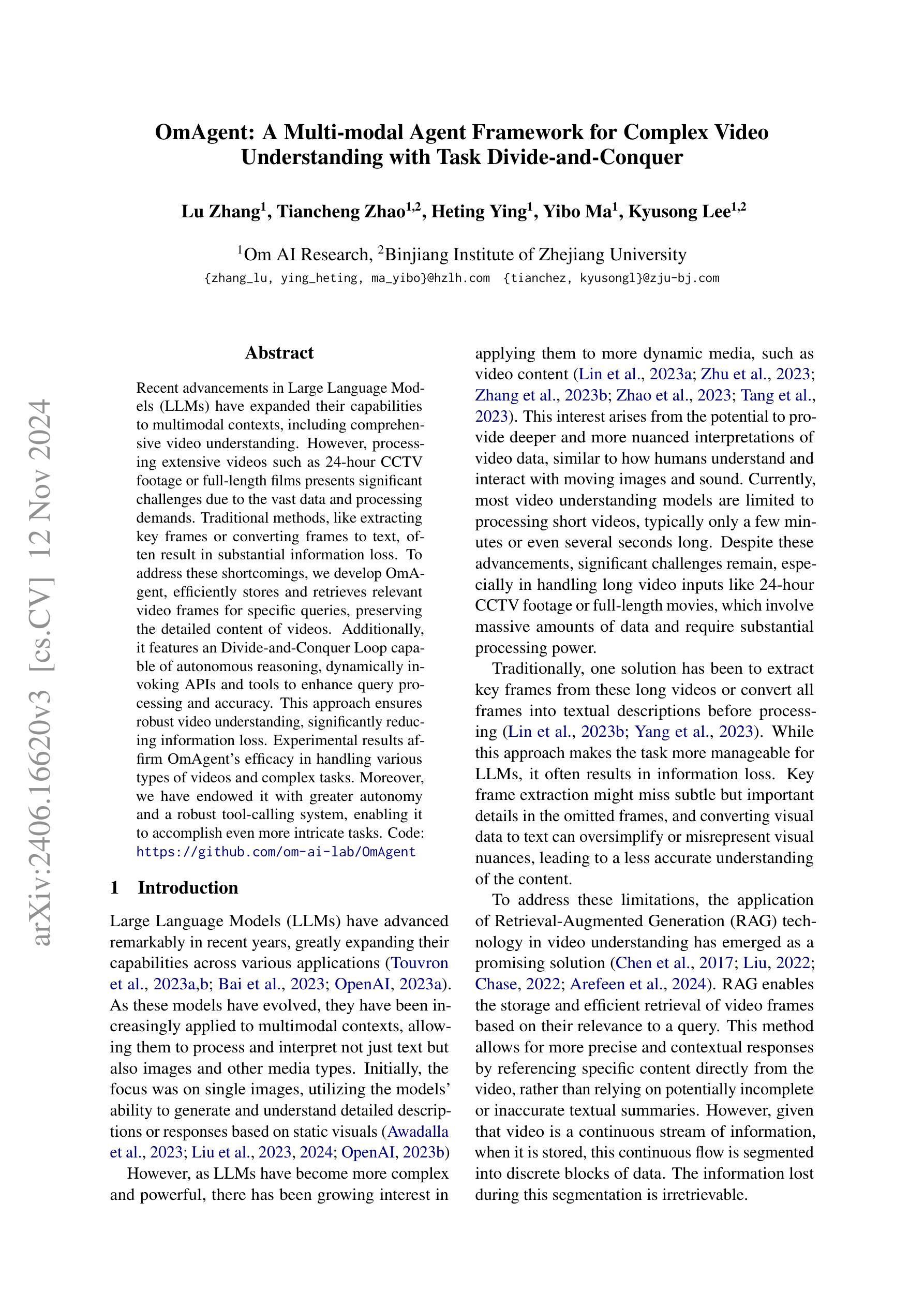
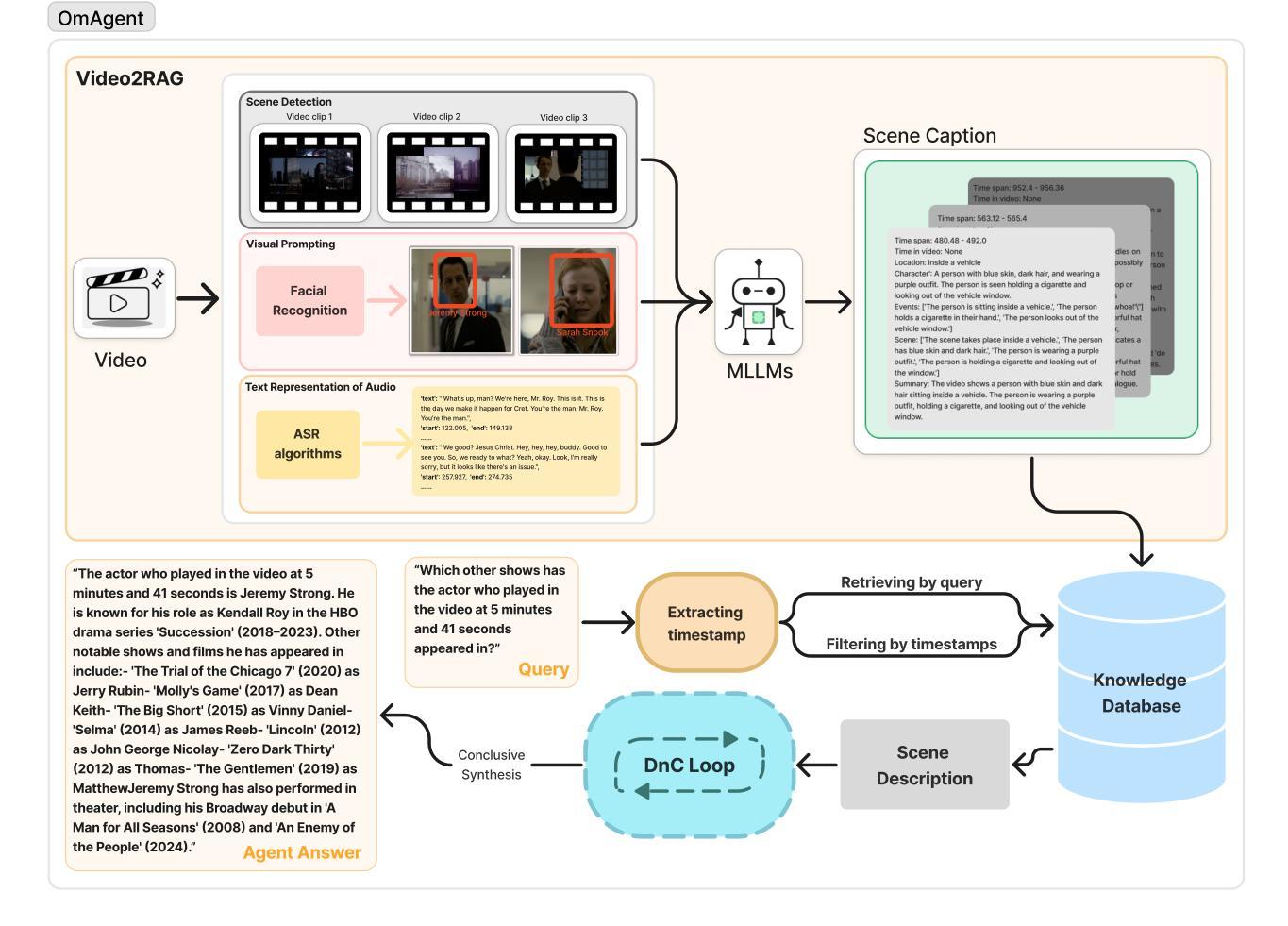
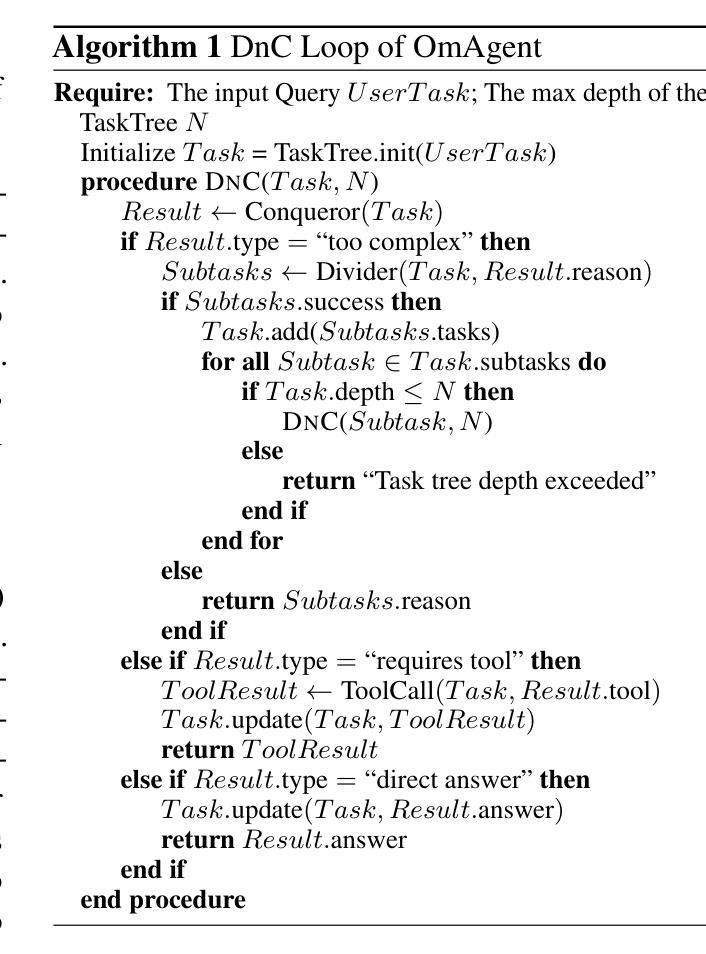
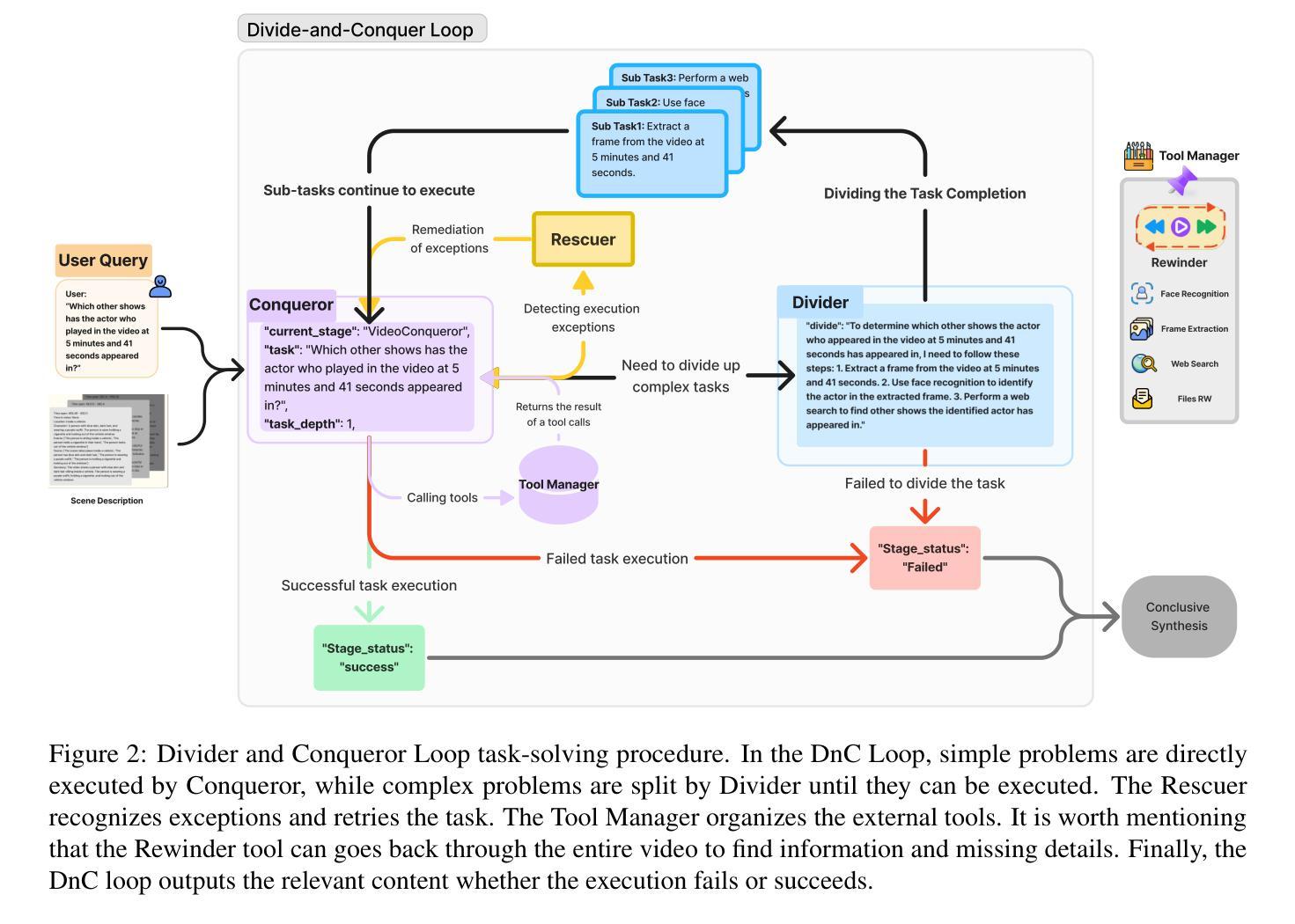
DrVideo: Document Retrieval Based Long Video Understanding
Authors:Ziyu Ma, Chenhui Gou, Hengcan Shi, Bin Sun, Shutao Li, Hamid Rezatofighi, Jianfei Cai
Most of the existing methods for video understanding primarily focus on videos only lasting tens of seconds, with limited exploration of techniques for handling long videos. The increased number of frames in long videos poses two main challenges: difficulty in locating key information and performing long-range reasoning. Thus, we propose DrVideo, a document-retrieval-based system designed for long video understanding. Our key idea is to convert the long-video understanding problem into a long-document understanding task so as to effectively leverage the power of large language models. Specifically, DrVideo first transforms a long video into a coarse text-based long document to initially retrieve key frames and then updates the documents with the augmented key frame information. It then employs an agent-based iterative loop to continuously search for missing information and augment the document until sufficient question-related information is gathered for making the final predictions in a chain-of-thought manner. Extensive experiments on long video benchmarks confirm the effectiveness of our method. DrVideo significantly outperforms existing LLM-based state-of-the-art methods on EgoSchema benchmark (3 minutes), MovieChat-1K benchmark (10 minutes), and the long split of Video-MME benchmark (average of 44 minutes).
现有的视频理解方法大多主要关注持续时间只有几十秒的视频,对于处理长视频的技术探索有限。长视频中帧数的增加带来了两个主要挑战:难以定位关键信息进行长程推理。因此,我们提出了DrVideo,一个基于文档检索的系统,专为长视频理解而设计。我们的核心思想是将长视频理解问题转化为长文档理解任务,以便有效利用大型语言模型的威力。具体来说,DrVideo首先将长视频转换为基于文本的粗略长文档,以初步检索关键帧,然后使用增强后的关键帧信息更新文档。接着,它采用基于代理的迭代循环来不断搜索缺失信息并更新文档,直到收集到足够与问题相关的信息,以进行最终的预测。在大型视频基准测试上的广泛实验证实了我们的方法的有效性。DrVideo在EgoSchema基准测试(3分钟)、MovieChat-1K基准测试(10分钟)和视频MME基准测试的长片段(平均44分钟)上的表现都显著优于现有的基于大型语言模型的最先进的方法。
论文及项目相关链接
PDF 17 pages
Summary
本文提出一种名为DrVideo的文档检索系统,用于处理长时间视频的理解问题。该系统将长时间视频转化为基于文本的文档,并利用大型语言模型的能力进行处理。DrVideo通过迭代搜索和更新文档的方式收集与问题相关的信息,在思想链中形成最终的预测结果。它在多个长时间视频基准测试中效果显著,如EgoSchema、MovieChat-1K和视频MME的长分割等。
Key Takeaways
- 现有视频理解方法主要关注时长几十秒的短视频,对于处理长视频的技术探索有限。
- 长视频由于帧数增多,存在定位关键信息和进行长距离推理两大挑战。
- DrVideo系统通过将长视频转化为基于文本的文档来解决这一问题,从而利用大型语言模型的力量。
- DrVideo首先会将长视频转化为粗略的文本文档以检索关键帧。
- 之后,系统会利用增强后的关键帧信息更新文档。
- DrVideo采用基于代理的迭代循环来持续搜索缺失信息并更新文档,直至收集到足够与问题相关的信息以做出最终预测。
点此查看论文截图
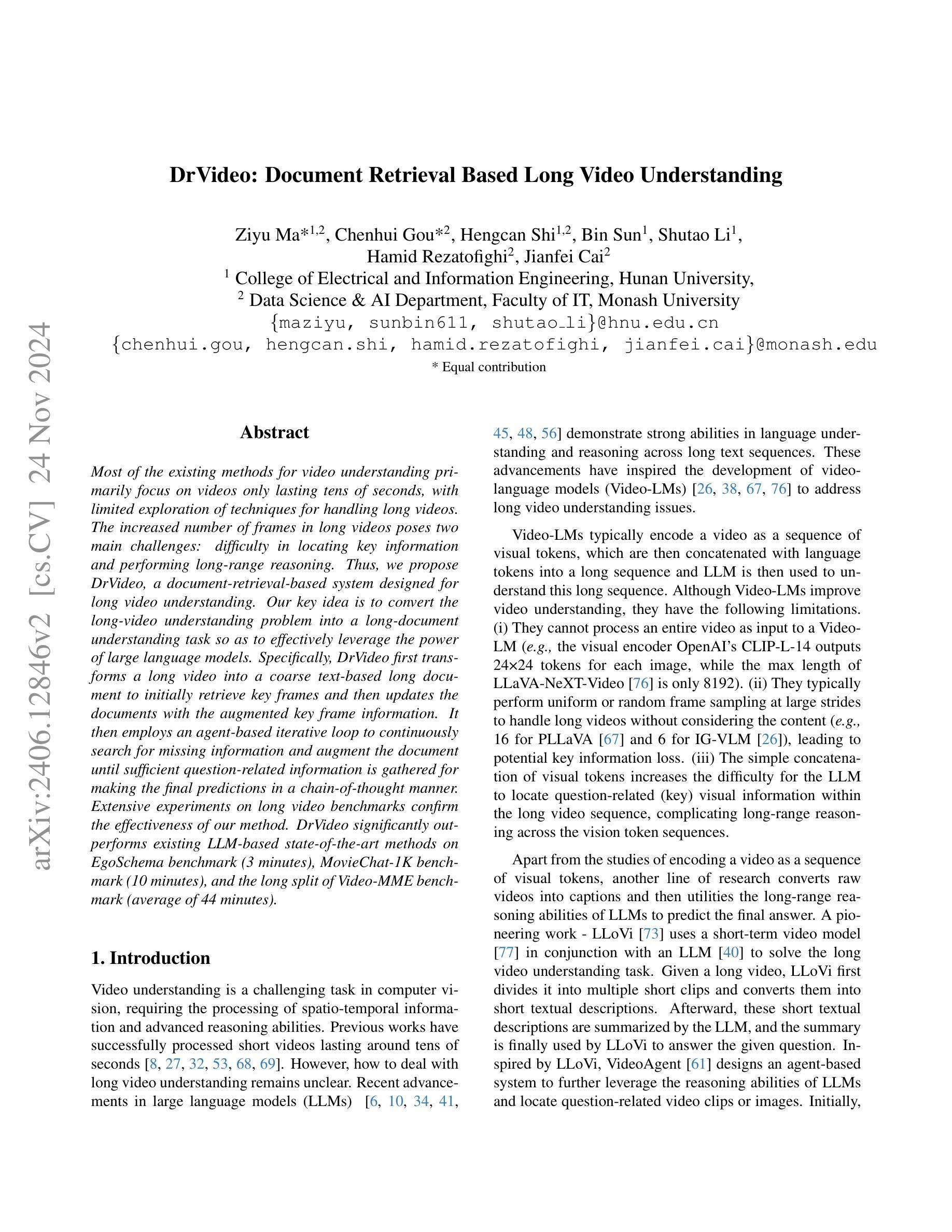
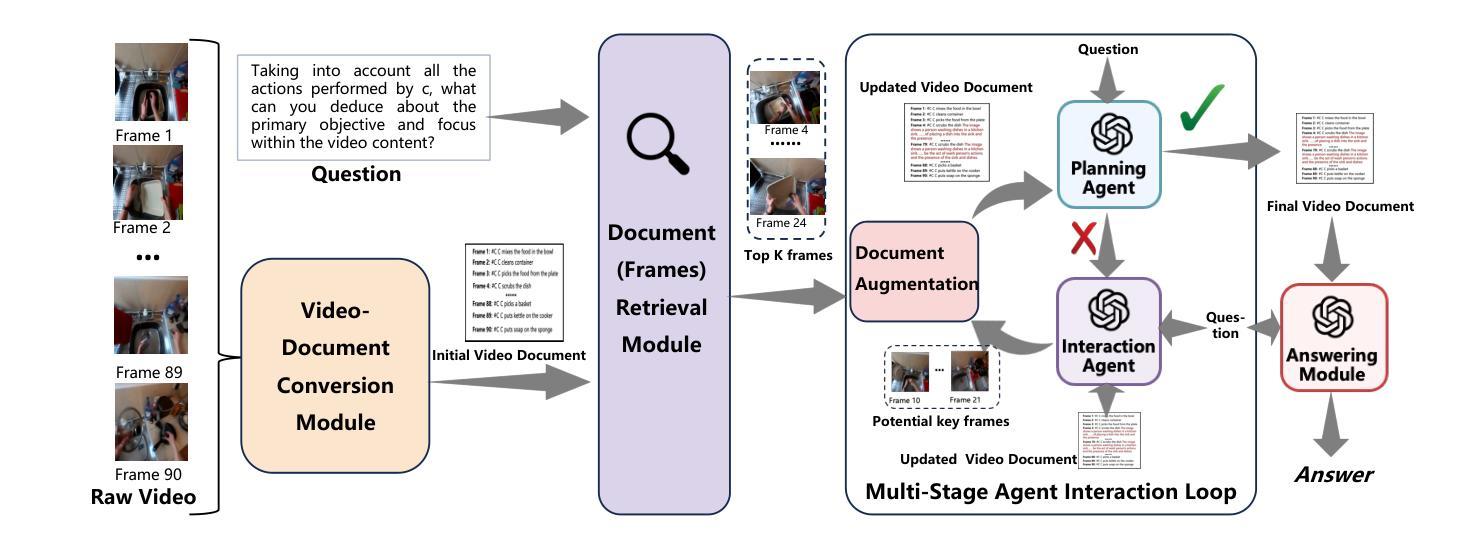
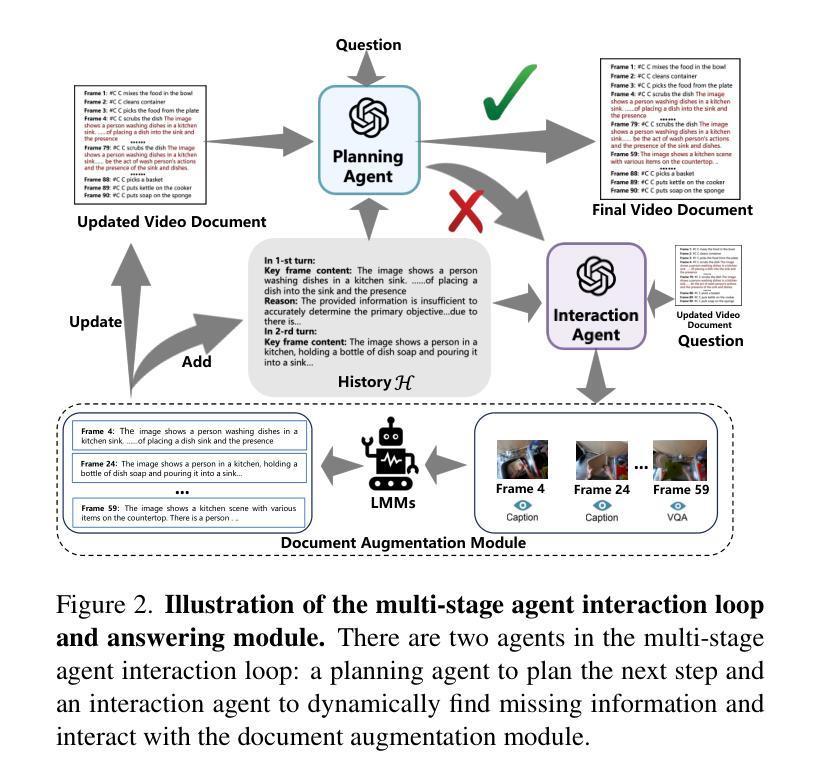
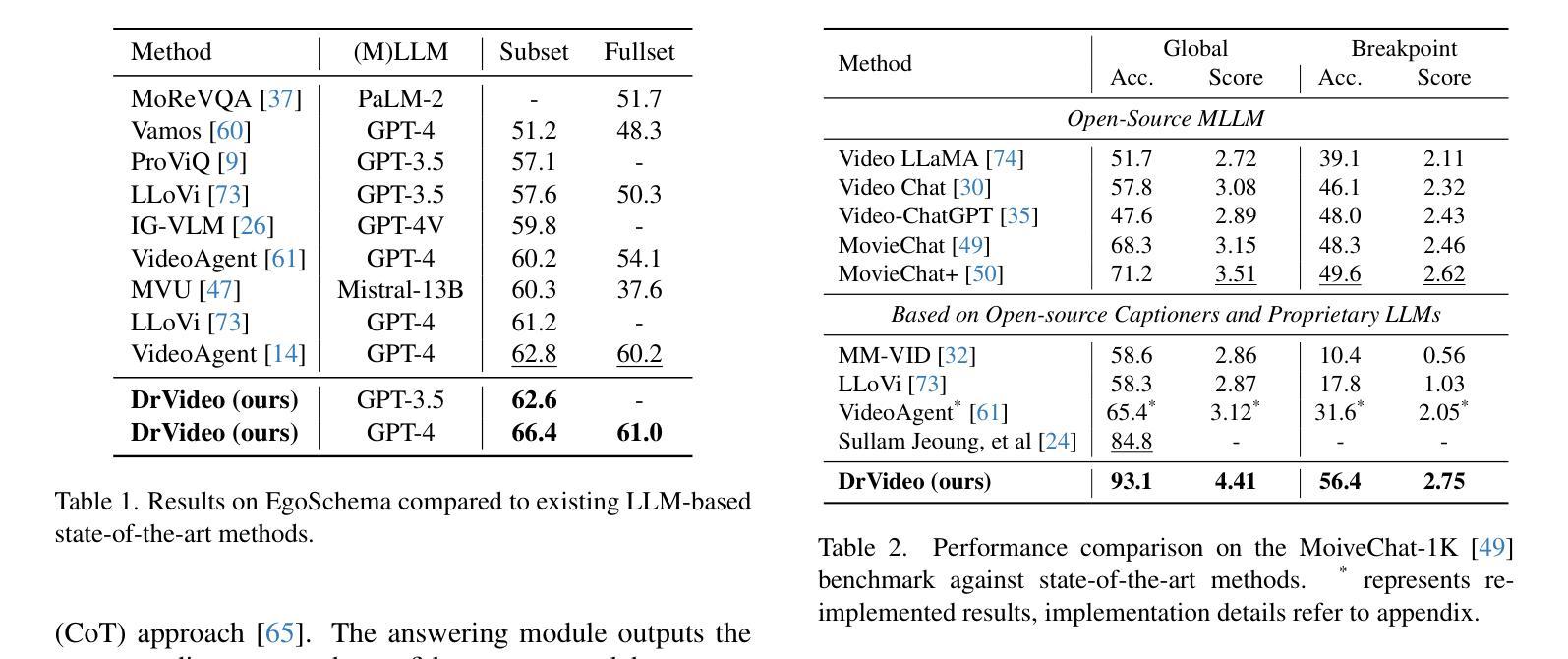
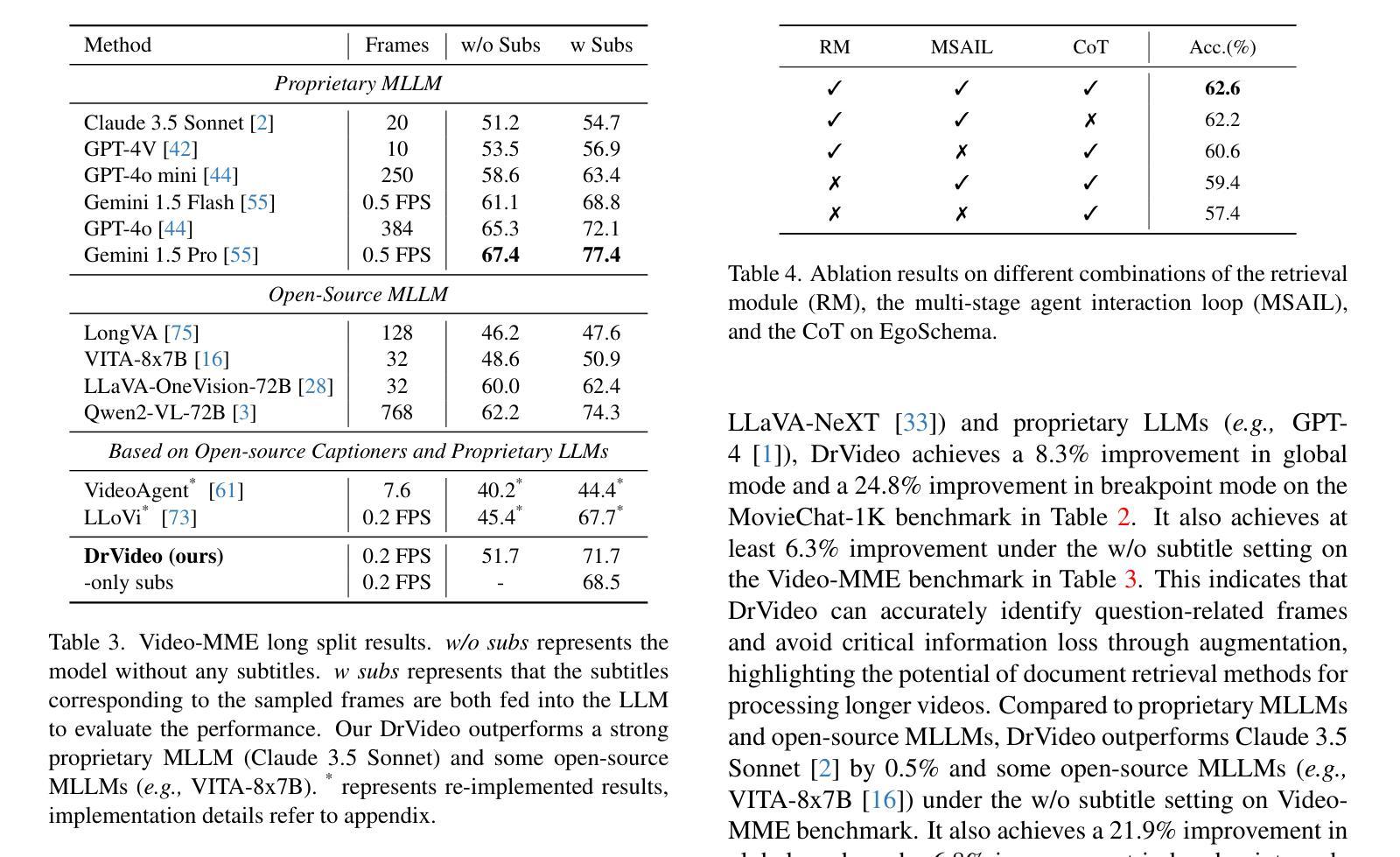
MAMBA4D: Efficient Long-Sequence Point Cloud Video Understanding with Disentangled Spatial-Temporal State Space Models
Authors:Jiuming Liu, Jinru Han, Lihao Liu, Angelica I. Aviles-Rivero, Chaokang Jiang, Zhe Liu, Hesheng Wang
Point cloud videos can faithfully capture real-world spatial geometries and temporal dynamics, which are essential for enabling intelligent agents to understand the dynamically changing world. However, designing an effective 4D backbone remains challenging, mainly due to the irregular and unordered distribution of points and temporal inconsistencies across frames. Also, recent transformer-based 4D backbones commonly suffer from large computational costs due to their quadratic complexity, particularly for long video sequences.To address these challenges, we propose a novel point cloud video understanding backbone purely based on the State Space Models (SSMs). Specifically, we first disentangle space and time in 4D video sequences and then establish the spatio-temporal correlation with our designed Mamba blocks. The Intra-frame Spatial Mamba module is developed to encode locally similar geometric structures within a certain temporal stride. Subsequently, locally correlated tokens are delivered to the Inter-frame Temporal Mamba module, which integrates long-term point features across the entire video with linear complexity. Our proposed Mamba4d achieves competitive performance on the MSR-Action3D action recognition (+10.4% accuracy), HOI4D action segmentation (+0.7 F1 Score), and Synthia4D semantic segmentation (+0.19 mIoU) datasets. Especially, for long video sequences, our method has a significant efficiency improvement with 87.5% GPU memory reduction and 5.36 times speed-up.
点云视频能够忠实捕捉现实世界中的空间几何和动态时序信息,这对于智能主体理解动态变化的世界至关重要。然而,设计有效的四维主干网络仍然是一个挑战,主要是由于点的分布不规则且无序,以及帧之间的时间不一致性。此外,基于最新变换器的四维主干网络通常因为其二次复杂性而面临巨大的计算成本,特别是对于长视频序列。为了解决这些挑战,我们提出了一种全新的点云视频理解主干网络,该网络完全基于状态空间模型(SSMs)。具体来说,我们首先解开四维视频序列中的空间和时间元素,然后通过设计的Mamba块建立时空关联。我们开发了帧内空间Mamba模块,以编码某一时间步长内局部相似的几何结构。随后,局部相关令牌被传递给帧间时间Mamba模块,该模块以线性复杂度整合整个视频的点特征。我们提出的Mamba4d在MSR-Action3D动作识别(提高10.4%的准确性)、HOI4D动作分割(提高0.7的F1分数)和Synthia4D语义分割(提高0.19的mIoU)等数据集上实现了有竞争力的性能。特别是对于长视频序列,我们的方法在GPU内存方面减少了87.5%,并实现了5.36倍的速度提升,具有显著的效率改进。
论文及项目相关链接
Summary
点云视频能够捕捉真实世界的空间几何和时态动态,这对于智能代理理解动态变化的世界至关重要。然而,设计有效的4D主干网络面临挑战,主要源于点的分布不规则、无序以及跨帧的时空不一致性。为解决这些问题,我们提出了一种基于状态空间模型(SSMs)的点云视频理解主干网络。通过设计Mamba块建立时空关联,实现帧内空间Mamba模块和帧间时间Mamba模块的协同工作,有效提升了点云视频处理的效率和性能。
Key Takeaways
- 点云视频能够捕捉真实世界的空间几何和时态动态,对智能代理理解动态世界至关重要。
- 设计有效的4D主干网络面临挑战,主要包括点的分布不规则、无序以及跨帧的时空不一致性。
- 基于状态空间模型(SSMs)的点云视频理解主干网络能够有效解决这些问题。
- 提出的Mamba块通过建立时空关联,实现帧内空间Mamba模块和帧间时间Mamba模块的协同工作。
- Mamba4d在MSR-Action3D动作识别、HOI4D动作分割和Synthia4D语义分割数据集上实现了具有竞争力的性能。
- 对于长视频序列,所提方法具有显著的效率提升,实现了87.5%的GPU内存减少和5.36倍的速度提升。
点此查看论文截图
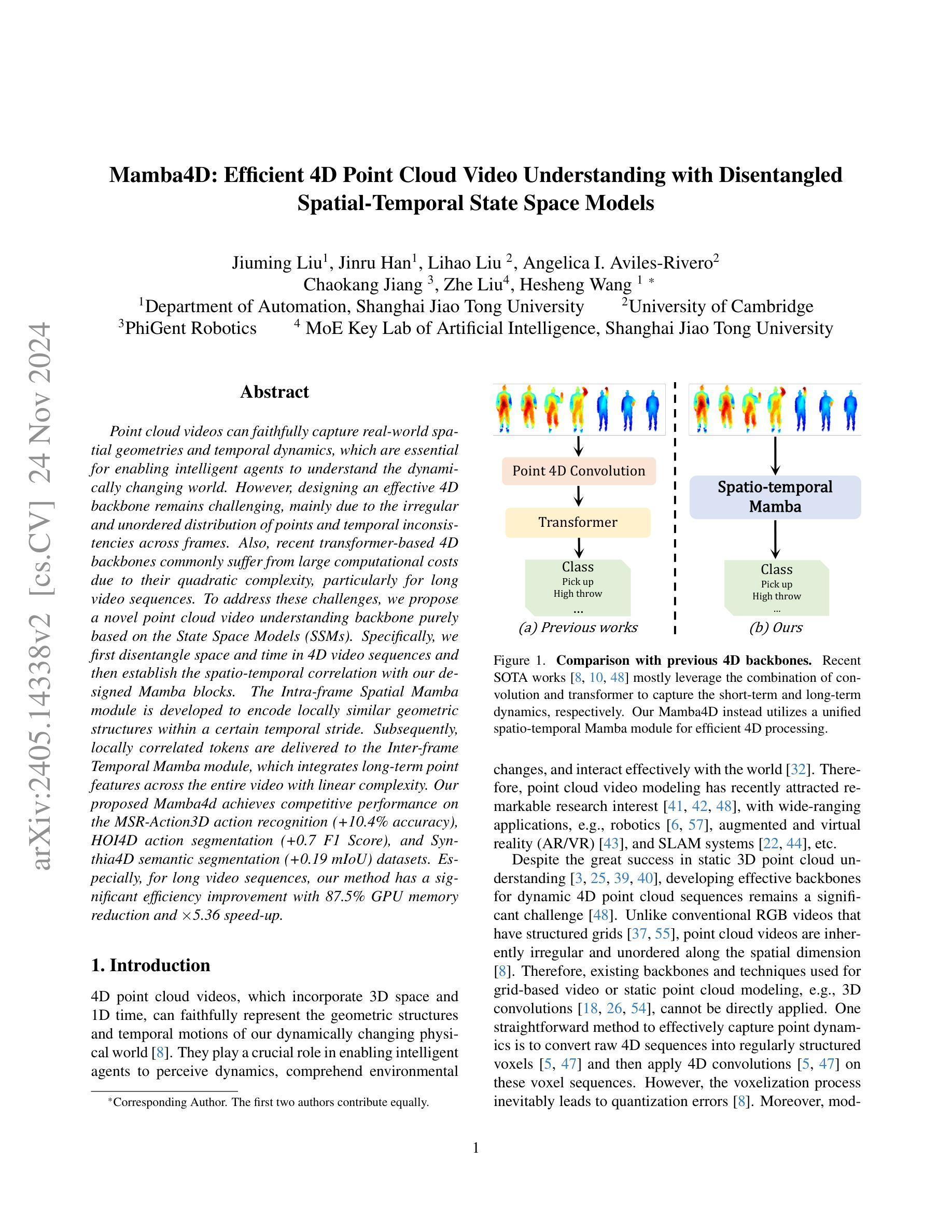
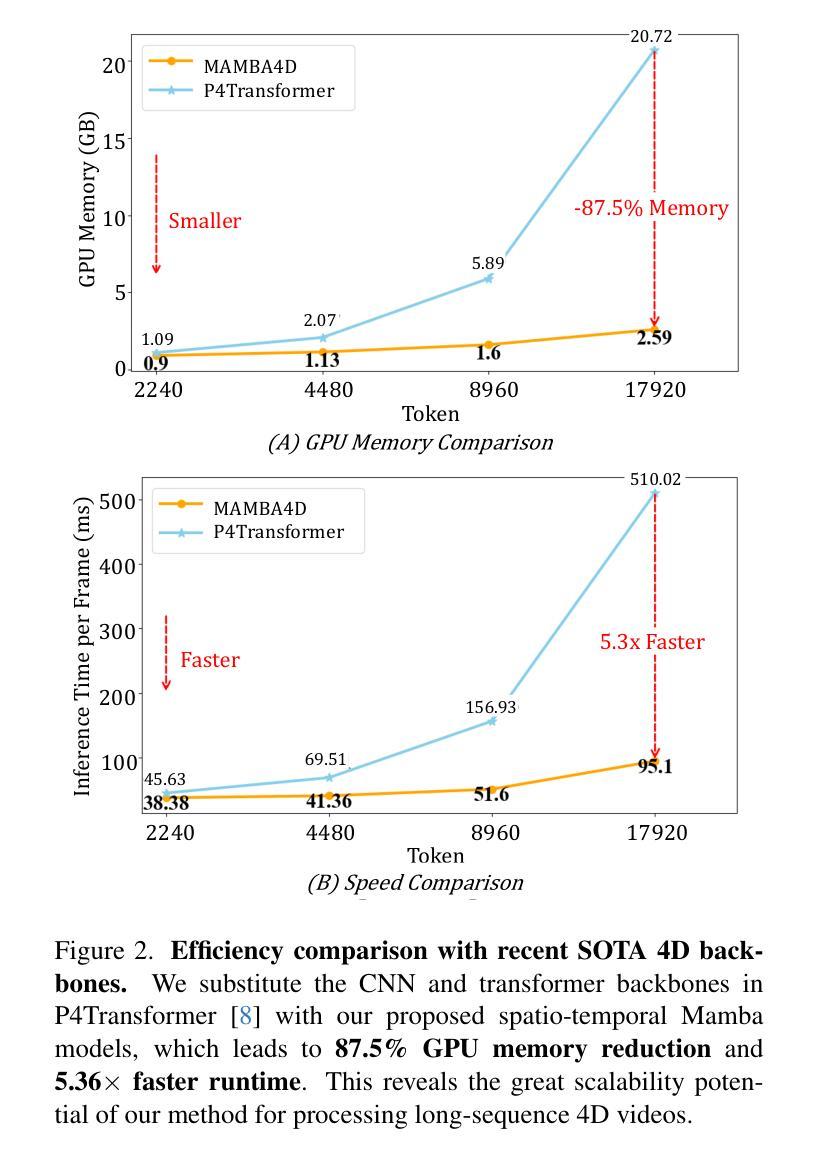
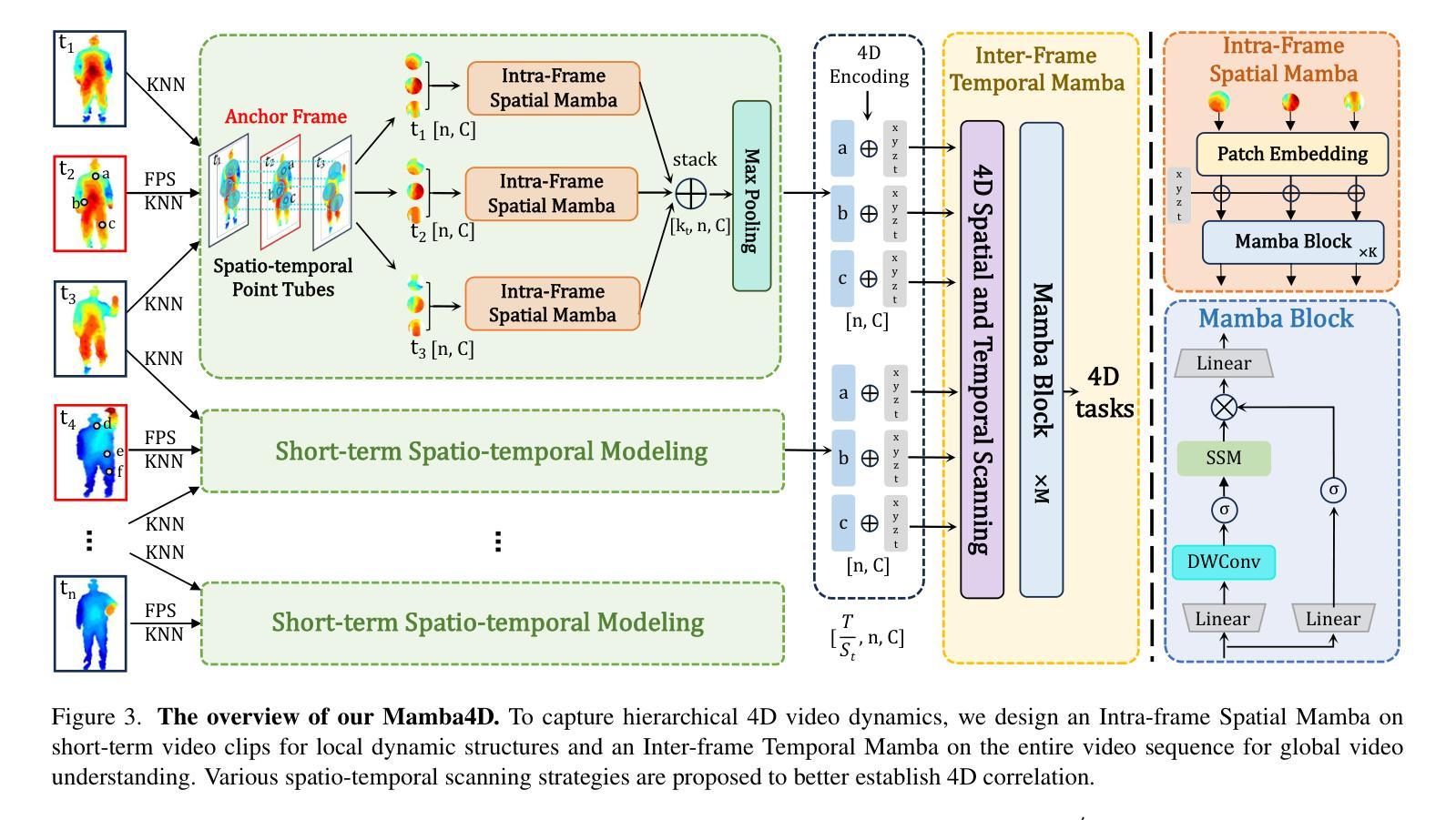
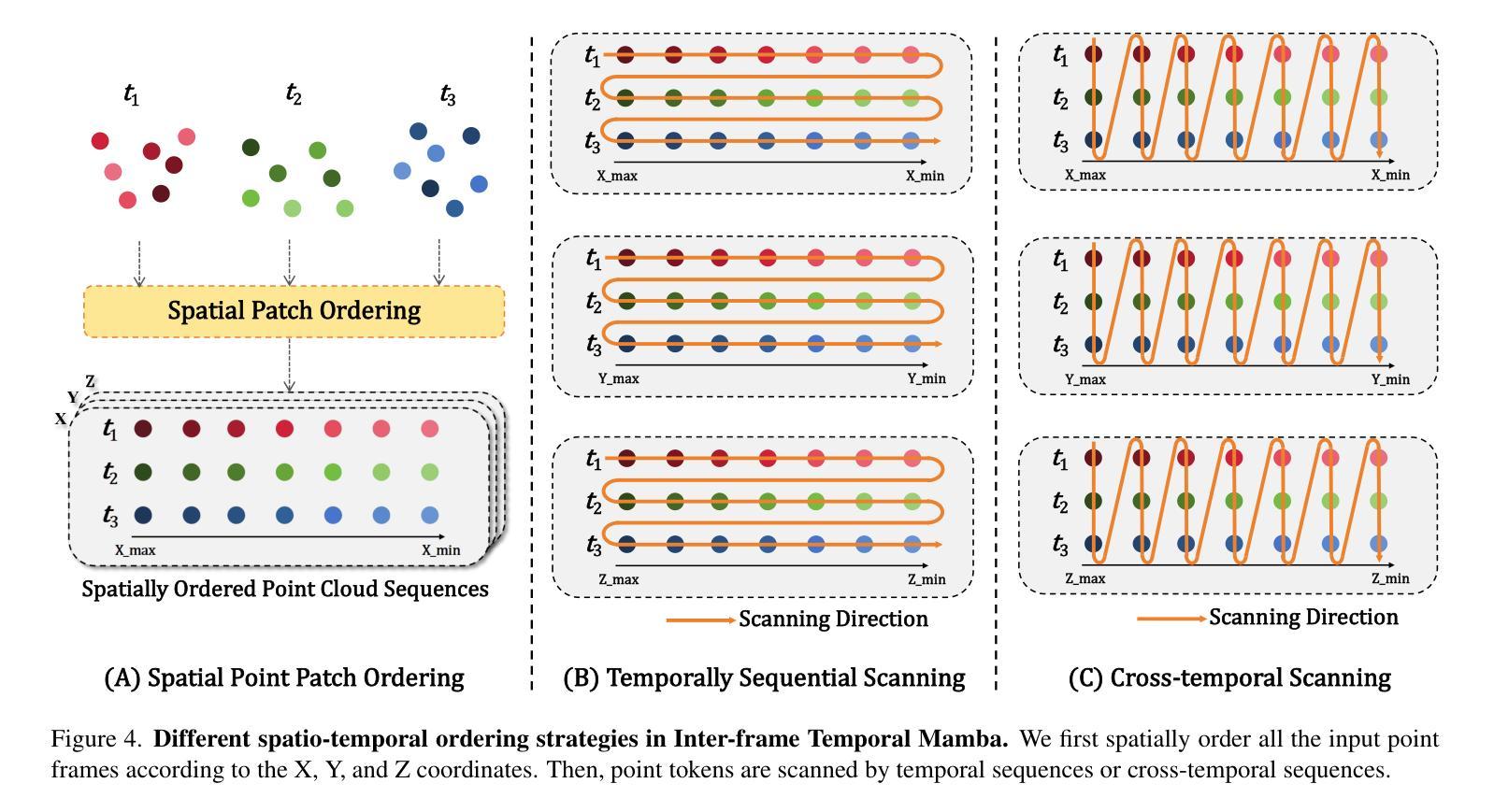
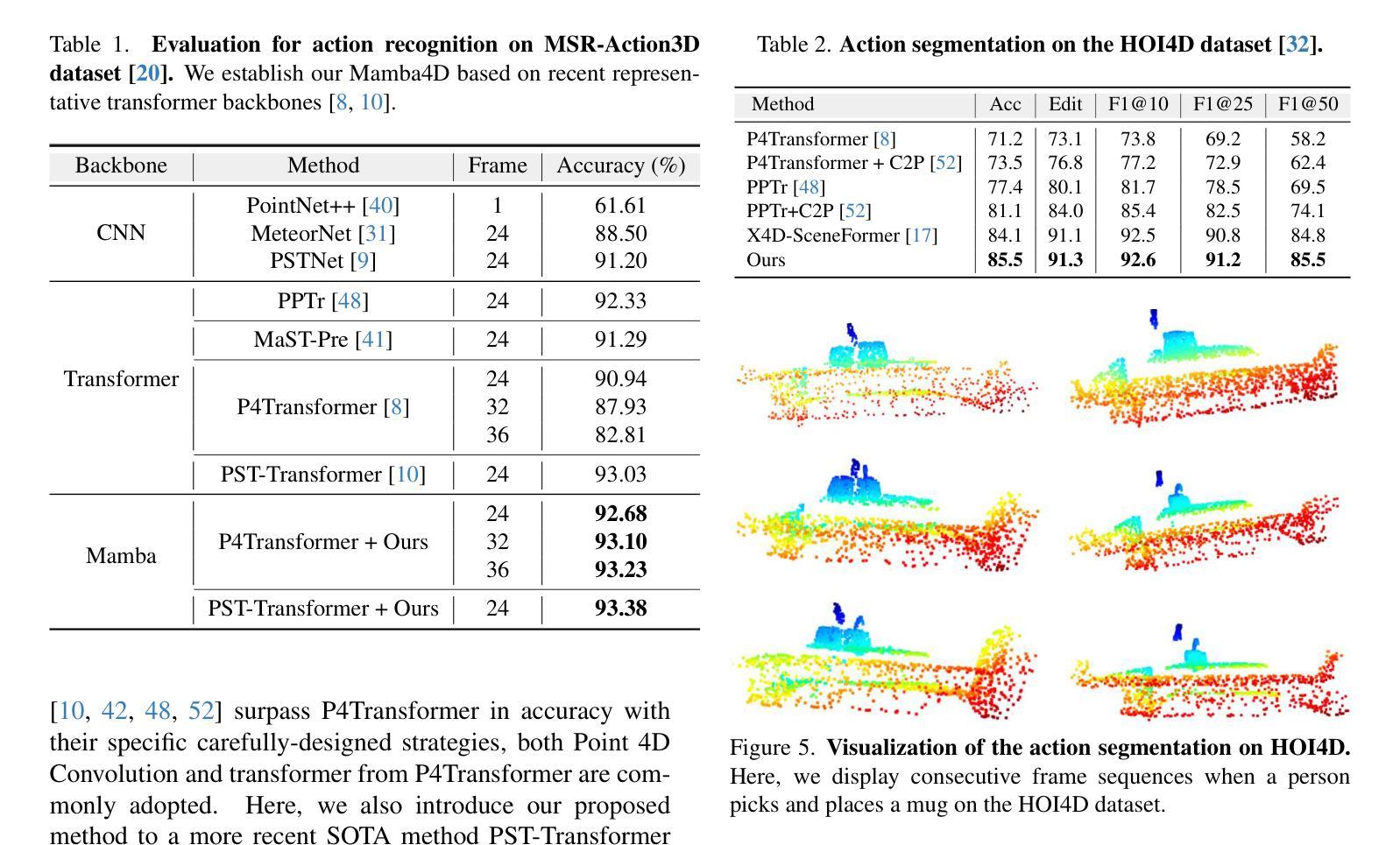
Understanding Long Videos with Multimodal Language Models
Authors:Kanchana Ranasinghe, Xiang Li, Kumara Kahatapitiya, Michael S. Ryoo
Large Language Models (LLMs) have allowed recent LLM-based approaches to achieve excellent performance on long-video understanding benchmarks. We investigate how extensive world knowledge and strong reasoning skills of underlying LLMs influence this strong performance. Surprisingly, we discover that LLM-based approaches can yield surprisingly good accuracy on long-video tasks with limited video information, sometimes even with no video specific information. Building on this, we exploring injecting video-specific information into an LLM-based framework. We utilize off-the-shelf vision tools to extract three object-centric information modalities from videos and then leverage natural language as a medium for fusing this information. Our resulting Multimodal Video Understanding (MVU) framework demonstrates state-of-the-art performance across multiple video understanding benchmarks. Strong performance also on robotics domain tasks establish its strong generality. Our code will be released publicly.
大型语言模型(LLM)使得最近的基于LLM的方法在长时间视频理解基准测试中取得了卓越的性能。我们研究基础LLM的广泛世界知识和强大的推理技能如何影响这一出色性能。令人惊讶的是,我们发现基于LLM的方法在有限的视频信息上,甚至在没有任何特定视频信息的情况下,都能在长时间视频任务上产生令人惊讶的良好准确性。基于此,我们探索将特定视频信息注入到基于LLM的框架中。我们使用现成的视觉工具从视频中提取三种以对象为中心的信息模式,然后利用自然语言作为融合这些信息的媒介。我们由此产生的多模态视频理解(MVU)框架在多个视频理解基准测试中展示了卓越的性能。在机器人领域任务上的出色表现也证明了其强大的通用性。我们的代码将公开发布。
论文及项目相关链接
PDF Code available at https://github.com/kahnchana/mvu
Summary
大规模语言模型(LLM)在视频理解方面展现出卓越性能。研究指出,LLM的丰富知识和强大推理能力是关键。令人惊讶的是,即便视频信息有限甚至毫无视频特定信息,LLM方法也能实现良好的准确性。通过注入视频特定信息到LLM框架中,并结合现成的视觉工具提取视频的三对象中心信息模式,再利用自然语言融合这些信息,研发出的多模式视频理解(MVU)框架在多个视频理解基准测试中表现卓越,且在机器人领域任务中表现同样出色。代码将公开发布。
Key Takeaways
- 大规模语言模型(LLM)在视频理解方面表现出色。
- LLM的丰富知识和推理能力对性能至关重要。
- LLM方法能在视频信息有限甚至毫无特定信息的情况下实现良好准确性。
- 通过注入视频特定信息到LLM框架中,能提高性能。
- 利用现成的视觉工具提取视频的三对象中心信息模式。
- 自然语言被用于融合这些视频信息模式。
点此查看论文截图
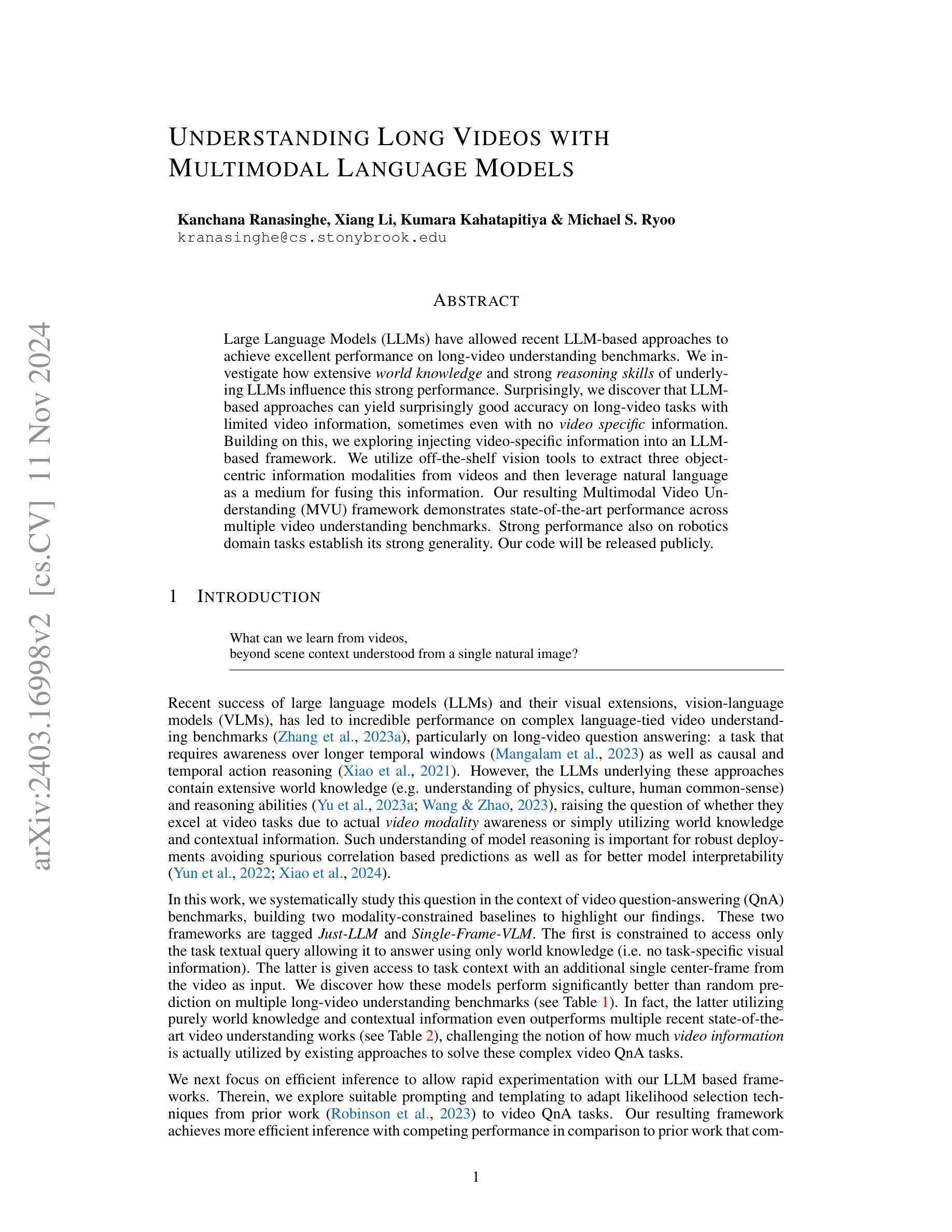
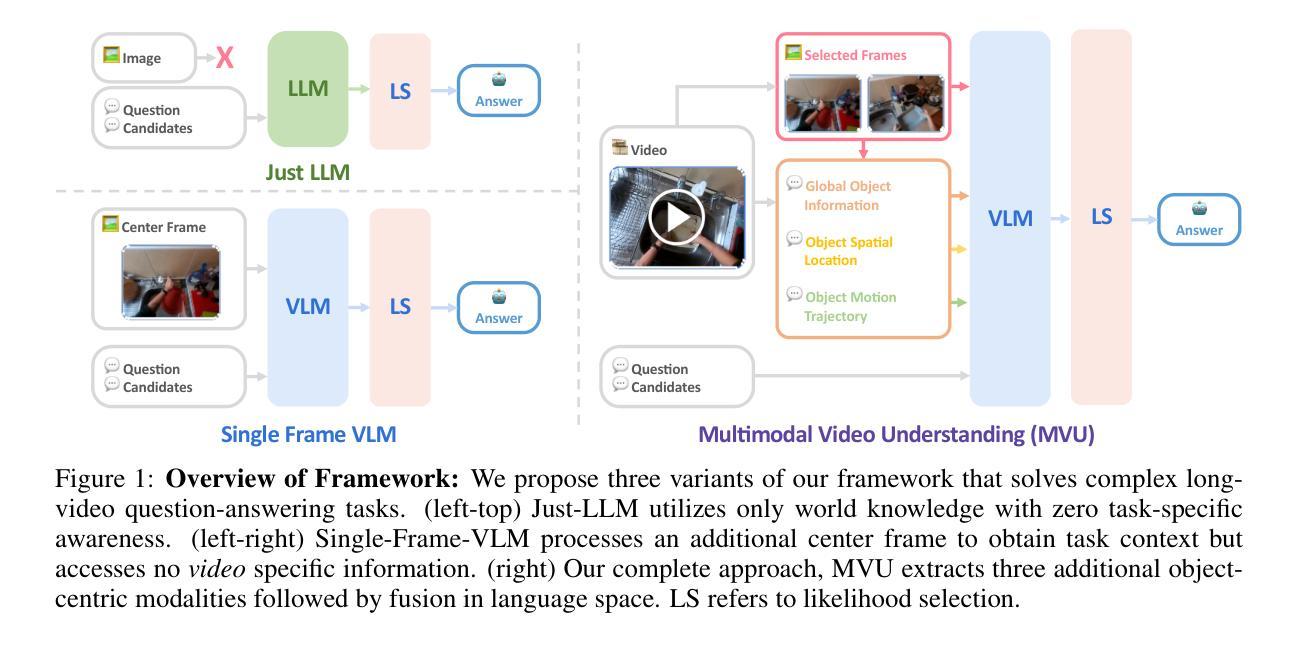
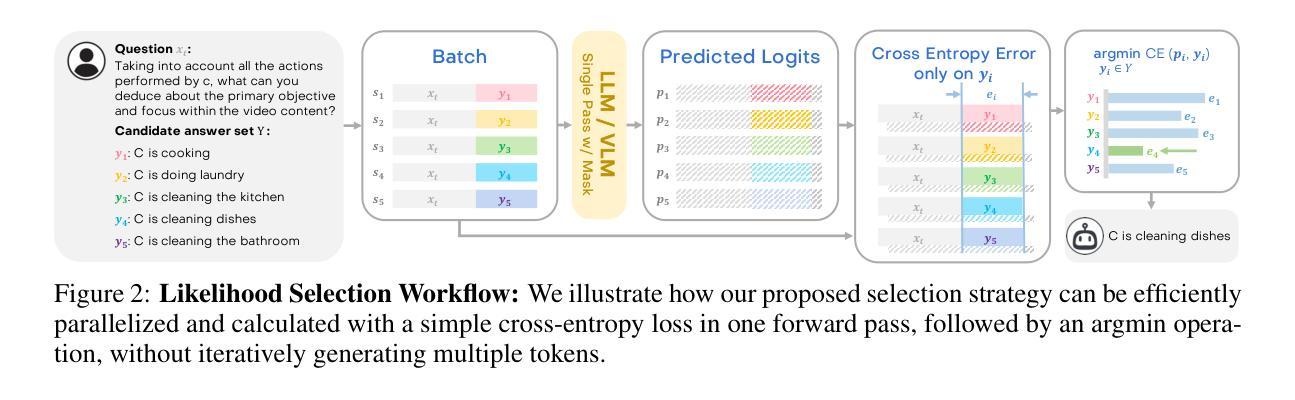
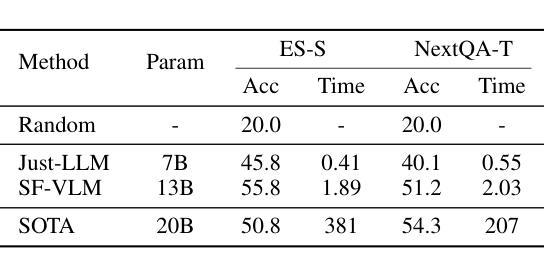
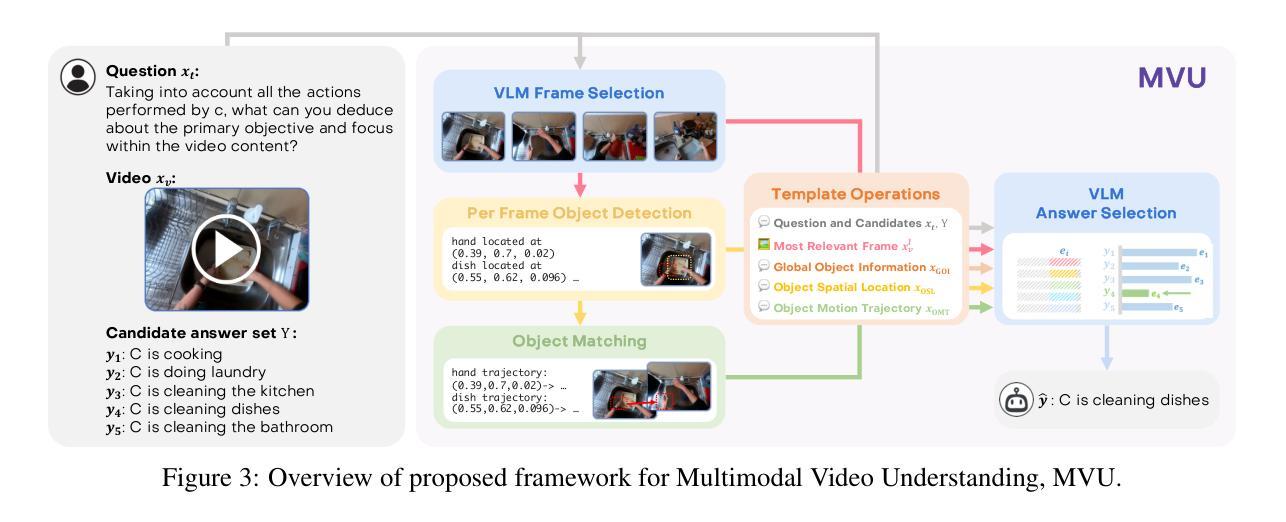
Towards Neuro-Symbolic Video Understanding
Authors:Minkyu Choi, Harsh Goel, Mohammad Omama, Yunhao Yang, Sahil Shah, Sandeep Chinchali
The unprecedented surge in video data production in recent years necessitates efficient tools to extract meaningful frames from videos for downstream tasks. Long-term temporal reasoning is a key desideratum for frame retrieval systems. While state-of-the-art foundation models, like VideoLLaMA and ViCLIP, are proficient in short-term semantic understanding, they surprisingly fail at long-term reasoning across frames. A key reason for this failure is that they intertwine per-frame perception and temporal reasoning into a single deep network. Hence, decoupling but co-designing semantic understanding and temporal reasoning is essential for efficient scene identification. We propose a system that leverages vision-language models for semantic understanding of individual frames but effectively reasons about the long-term evolution of events using state machines and temporal logic (TL) formulae that inherently capture memory. Our TL-based reasoning improves the F1 score of complex event identification by 9-15% compared to benchmarks that use GPT4 for reasoning on state-of-the-art self-driving datasets such as Waymo and NuScenes.
近年来,视频数据生产的空前增长需要大量有效的工具从视频中提取有意义的帧以供下游任务使用。长期时间推理是帧检索系统的关键需求。虽然最先进的基础模型,如VideoLLaMA和ViCLIP,擅长短期语义理解,但它们在长期跨帧推理方面却出人意料地失败了。失败的一个关键原因是它们将逐帧感知和时间推理交织在一个深度网络中。因此,解耦但协同设计语义理解和时间推理对于有效场景识别至关重要。我们提出了一个系统,该系统利用视觉语言模型对单个帧进行语义理解,并利用状态机和时间逻辑(TL)公式有效地推理长期事件的演变,这些公式可以固有地捕获记忆。与在Waymo和NuScenes等先进自动驾驶数据集上使用GPT4进行推理的基准测试相比,我们的基于TL的推理将复杂事件识别的F1分数提高了9-15%。
论文及项目相关链接
PDF Accepted by The European Conference on Computer Vision (ECCV) 2024
Summary
视频数据量的激增要求开发有效的工具从视频中提取有意义的帧以供下游任务使用。长期时间推理是帧检索系统的关键要素。尽管最前沿的基础模型,如VideoLLaMA和ViCLIP,擅长短期语义理解,但在长期跨帧推理方面却表现不佳。其原因在于它们将单帧感知和时间推理交织在一个深度网络中。因此,对语义理解和时间推理进行解耦和协同设计对于有效场景识别至关重要。我们提出了一种系统,该系统利用视觉语言模型对单个帧进行语义理解,同时使用状态机和时间逻辑(TL)公式有效推理长期事件的发展,这些公式能固有地捕捉记忆。与在前沿自动驾驶数据集(如Waymo和NuScenes)上使用GPT4进行推理的基准测试相比,我们的TL基于推理将复杂事件识别的F1分数提高了9-15%。
Key Takeaways
- 视频数据量的激增需要有效的工具从视频中提取有意义的帧。
- 长期时间推理是帧检索系统的关键要素。
- 最新的基础模型(如VideoLLaMA和ViCLIP)在短期语义理解方面表现出色,但在长期跨帧推理方面存在不足。
- 当前模型将单帧感知和时间推理交织在一起,需要解耦和协同设计。
- 提出的系统利用视觉语言模型进行语义理解,并结合状态机和时间逻辑公式进行长期事件推理。
- 时间逻辑公式能固有地捕捉记忆,这是提高复杂事件识别性能的关键。
- 与使用GPT4的基准测试相比,系统在复杂事件识别方面的F1分数提高了9-15%。
点此查看论文截图
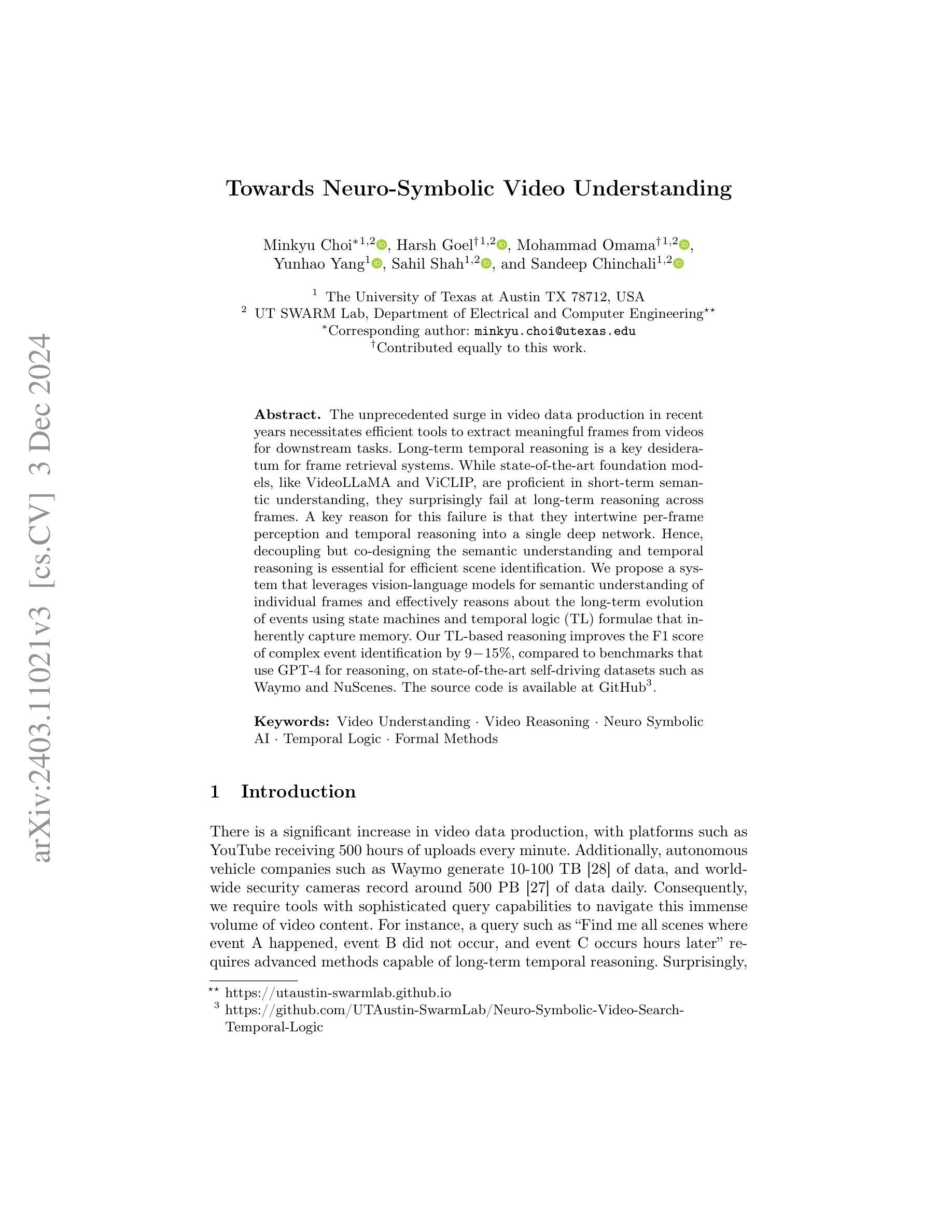
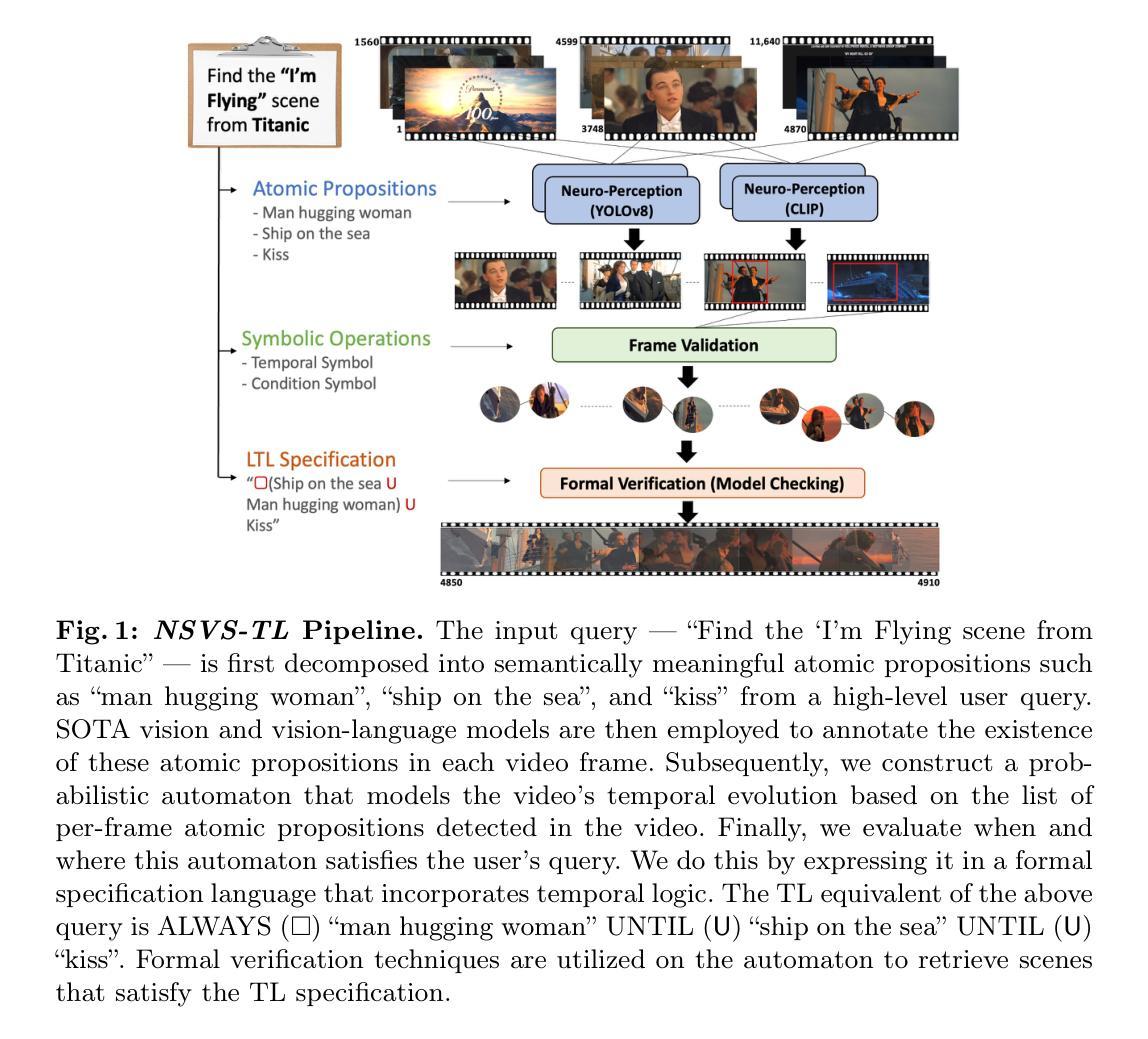
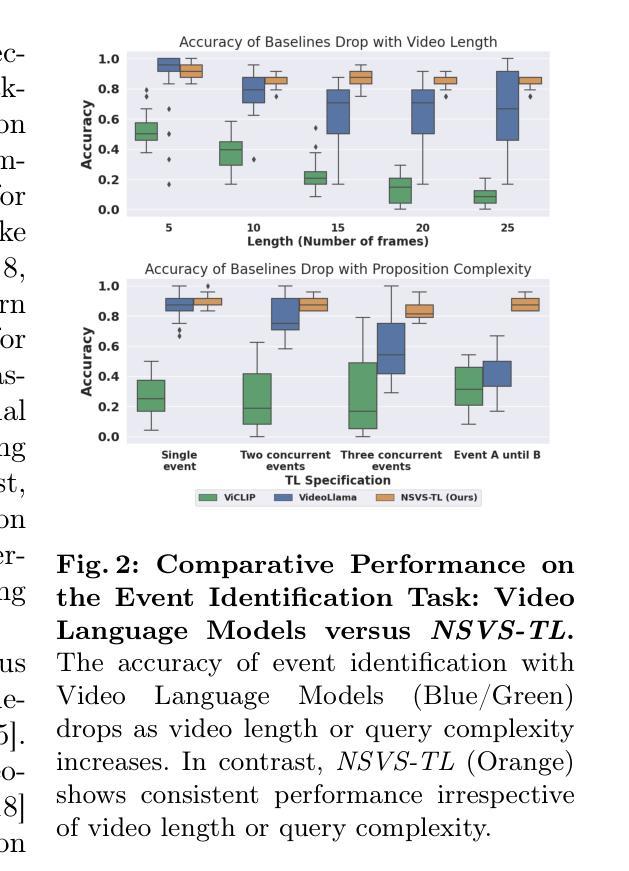