⚠️ 以下所有内容总结都来自于 大语言模型的能力,如有错误,仅供参考,谨慎使用
🔴 请注意:千万不要用于严肃的学术场景,只能用于论文阅读前的初筛!
💗 如果您觉得我们的项目对您有帮助 ChatPaperFree ,还请您给我们一些鼓励!⭐️ HuggingFace免费体验
2024-12-12 更新
Local Features Meet Stochastic Anonymization: Revolutionizing Privacy-Preserving Face Recognition for Black-Box Models
Authors:Yuanwei Liu, Chengyu Jia, Ruqi Xiao, Xuemai Jia, Hui Wei, Kui Jiang, Zheng Wang
The task of privacy-preserving face recognition (PPFR) currently faces two major unsolved challenges: (1) existing methods are typically effective only on specific face recognition models and struggle to generalize to black-box face recognition models; (2) current methods employ data-driven reversible representation encoding for privacy protection, making them susceptible to adversarial learning and reconstruction of the original image. We observe that face recognition models primarily rely on local features ({e.g., face contour, skin texture, and so on) for identification. Thus, by disrupting global features while enhancing local features, we achieve effective recognition even in black-box environments. Additionally, to prevent adversarial models from learning and reversing the anonymization process, we adopt an adversarial learning-based approach with irreversible stochastic injection to ensure the stochastic nature of the anonymization. Experimental results demonstrate that our method achieves an average recognition accuracy of 94.21% on black-box models, outperforming existing methods in both privacy protection and anti-reconstruction capabilities.
隐私保护人脸识别(PPFR)的任务当前面临两大未解决的挑战:(1)现有方法通常仅对特定的人脸识别模型有效,在难以推广至黑箱人脸识别模型;(2)当前的方法采用数据驱动的可逆表示编码进行隐私保护,使其容易受到对抗学习和原始图像的重构。我们观察到人脸识别模型主要依赖局部特征(例如脸部轮廓、皮肤纹理等)进行识别。因此,通过干扰全局特征同时增强局部特征,我们即使在黑箱环境中也能实现有效识别。此外,为了防止对抗模型学习和反转匿名化过程,我们采用基于对抗学习的不可逆随机注入方法,以确保匿名化的随机性。实验结果表明,我们的方法在黑箱模型上平均识别准确率为94.21%,在隐私保护和防重建能力方面都优于现有方法。
论文及项目相关链接
Summary
人脸识别技术面临隐私保护难题,当前两大挑战在于缺乏普遍适用性和安全性。该研究提出新方法应对,干扰全局特征强化局部特征以适配黑箱模型环境,同时采用对抗学习防止隐私信息泄露和反匿名化。实验结果证实其有效性。
Key Takeaways
- 人脸识别面临隐私保护难题,存在两大挑战:模型通用性和安全性。
- 当前方法主要依赖特定模型,难以应用于黑箱模型。
- 人脸识别主要依赖局部特征进行识别。
- 研究通过干扰全局特征强化局部特征以实现黑箱环境下的有效识别。
- 采用对抗学习防止匿名化过程被学习和逆转。
点此查看论文截图
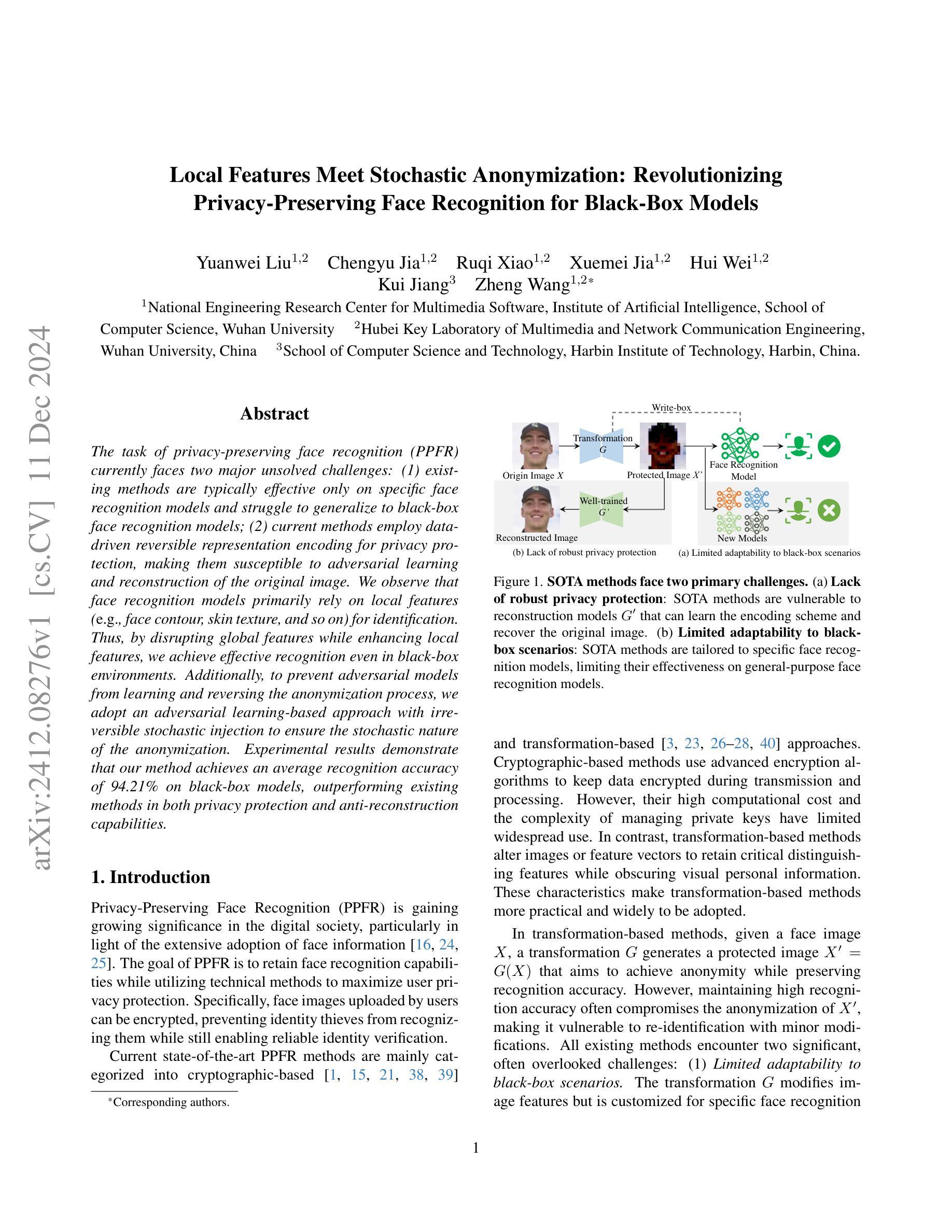
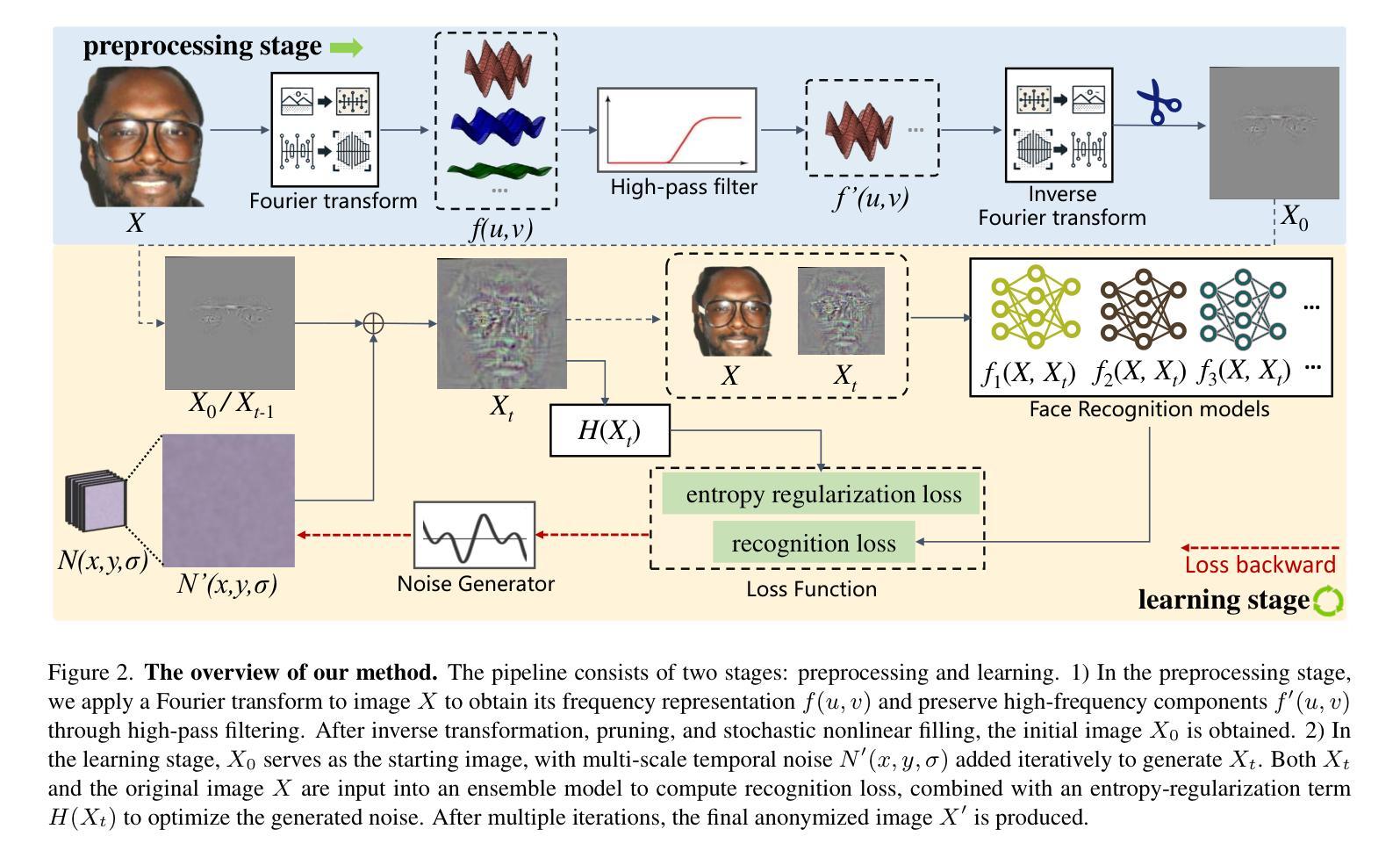
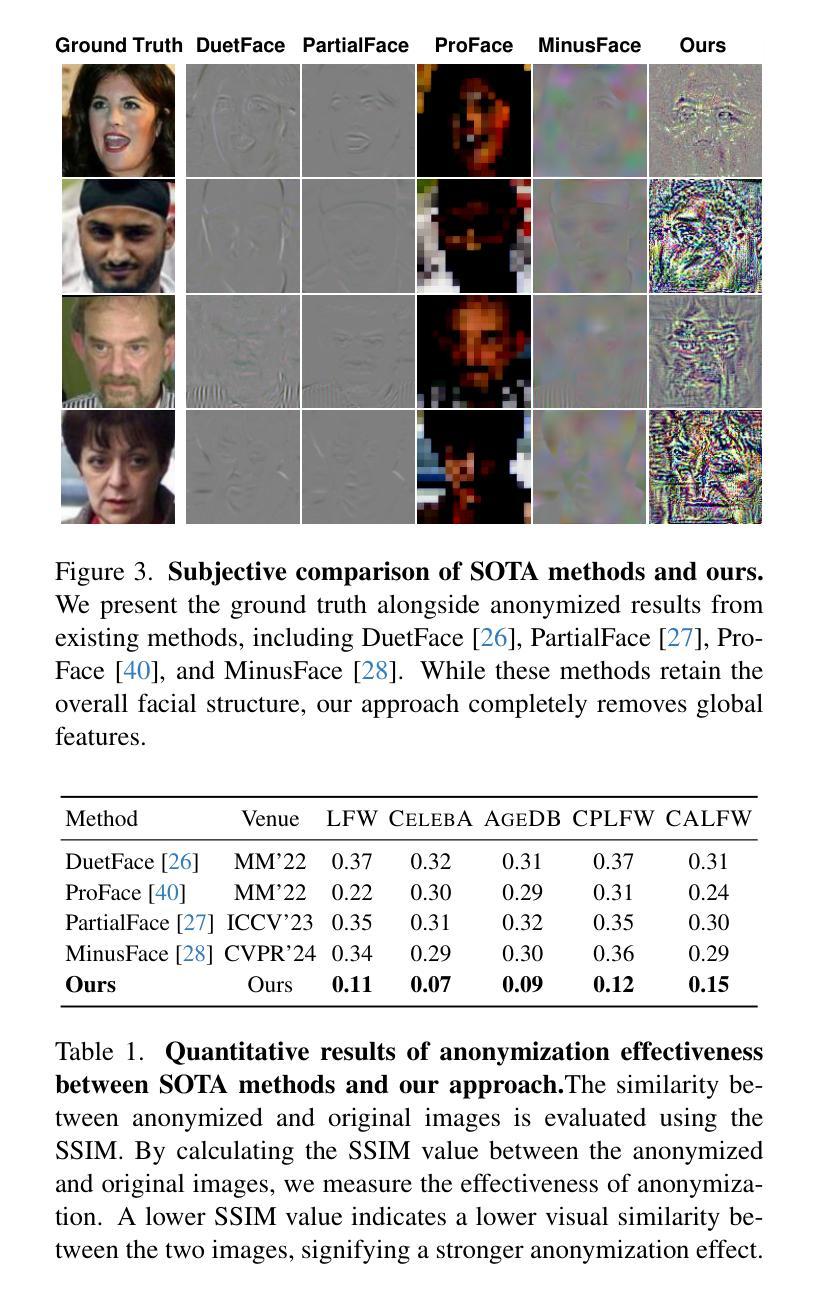
PETALface: Parameter Efficient Transfer Learning for Low-resolution Face Recognition
Authors:Kartik Narayan, Nithin Gopalakrishnan Nair, Jennifer Xu, Rama Chellappa, Vishal M. Patel
Pre-training on large-scale datasets and utilizing margin-based loss functions have been highly successful in training models for high-resolution face recognition. However, these models struggle with low-resolution face datasets, in which the faces lack the facial attributes necessary for distinguishing different faces. Full fine-tuning on low-resolution datasets, a naive method for adapting the model, yields inferior performance due to catastrophic forgetting of pre-trained knowledge. Additionally the domain difference between high-resolution (HR) gallery images and low-resolution (LR) probe images in low resolution datasets leads to poor convergence for a single model to adapt to both gallery and probe after fine-tuning. To this end, we propose PETALface, a Parameter-Efficient Transfer Learning approach for low-resolution face recognition. Through PETALface, we attempt to solve both the aforementioned problems. (1) We solve catastrophic forgetting by leveraging the power of parameter efficient fine-tuning(PEFT). (2) We introduce two low-rank adaptation modules to the backbone, with weights adjusted based on the input image quality to account for the difference in quality for the gallery and probe images. To the best of our knowledge, PETALface is the first work leveraging the powers of PEFT for low resolution face recognition. Extensive experiments demonstrate that the proposed method outperforms full fine-tuning on low-resolution datasets while preserving performance on high-resolution and mixed-quality datasets, all while using only 0.48% of the parameters. Code: https://kartik-3004.github.io/PETALface/
在大规模数据集上进行预训练并利用基于边界的损失函数在训练人脸识别模型方面取得了巨大成功。然而,这些模型在处理低分辨率人脸数据集时遇到了困难,这些低分辨率人脸缺乏区分不同人脸所需的面部特征。在低分辨率数据集上完全微调模型(一种适应模型的简单方法)会产生较差的性能,因为会发生灾难性的遗忘预训练知识。此外,低分辨率(LR)探针图像与高分辨率(HR)画廊图像之间的域差异导致单一模型在微调后难以适应画廊和探针。为此,我们提出了PETALface,这是一种针对低分辨率人脸识别的参数高效迁移学习方法。通过PETALface,我们试图解决上述两个问题。(1)我们通过利用参数高效微调(PEFT)的力量来解决灾难性遗忘问题。(2)我们在主干中引入了两个低秩适应模块,根据输入图像质量调整权重,以弥补画廊和探针图像质量上的差异。据我们所知,PETALface是第一个利用PEFT优势进行低分辨率人脸识别的工作。大量实验表明,该方法在低分辨率数据集上的性能优于完全微调,同时在高分辨率和混合质量数据集上的性能得以保持,而且仅使用0.48%的参数。代码:https://kartik-3004.github.io/PETALface/
论文及项目相关链接
PDF Accepted to WACV 2025. Project Page: https://kartik-3004.github.io/PETALface/
Summary
该文探讨了预训练大尺度数据集和采用基于边距的损失函数在高分辨率人脸识别模型训练中的成功应用,但这类模型在低分辨率人脸数据集上表现欠佳。为解决这一问题,提出了PETALface方法,一种针对低分辨率人脸识别的参数高效迁移学习框架。通过参数有效微调(PEFT)解决灾难性遗忘问题,并引入两个低秩适配模块来调整骨干网络权重以应对不同图像质量的差异。该方法仅使用原参数的0.48%,且能在低分辨率数据集上超越全微调的表现,同时保持在高分辨率和混合质量数据集上的性能。
Key Takeaways
- 预训练大尺度数据集和基于边距的损失函数在高分辨率人脸识别中效果显著。
- 低分辨率人脸数据集中,面部特征不足导致现有模型表现不佳。
- 单纯微调低分辨率数据集会导致模型遗忘预训练知识(灾难性遗忘)。
- PETALface是一种针对低分辨率人脸识别的参数高效迁移学习框架。
- PETALface通过参数有效微调(PEFT)解决灾难性遗忘问题。
- PETALface引入低秩适配模块以应对不同图像质量的差异。
点此查看论文截图
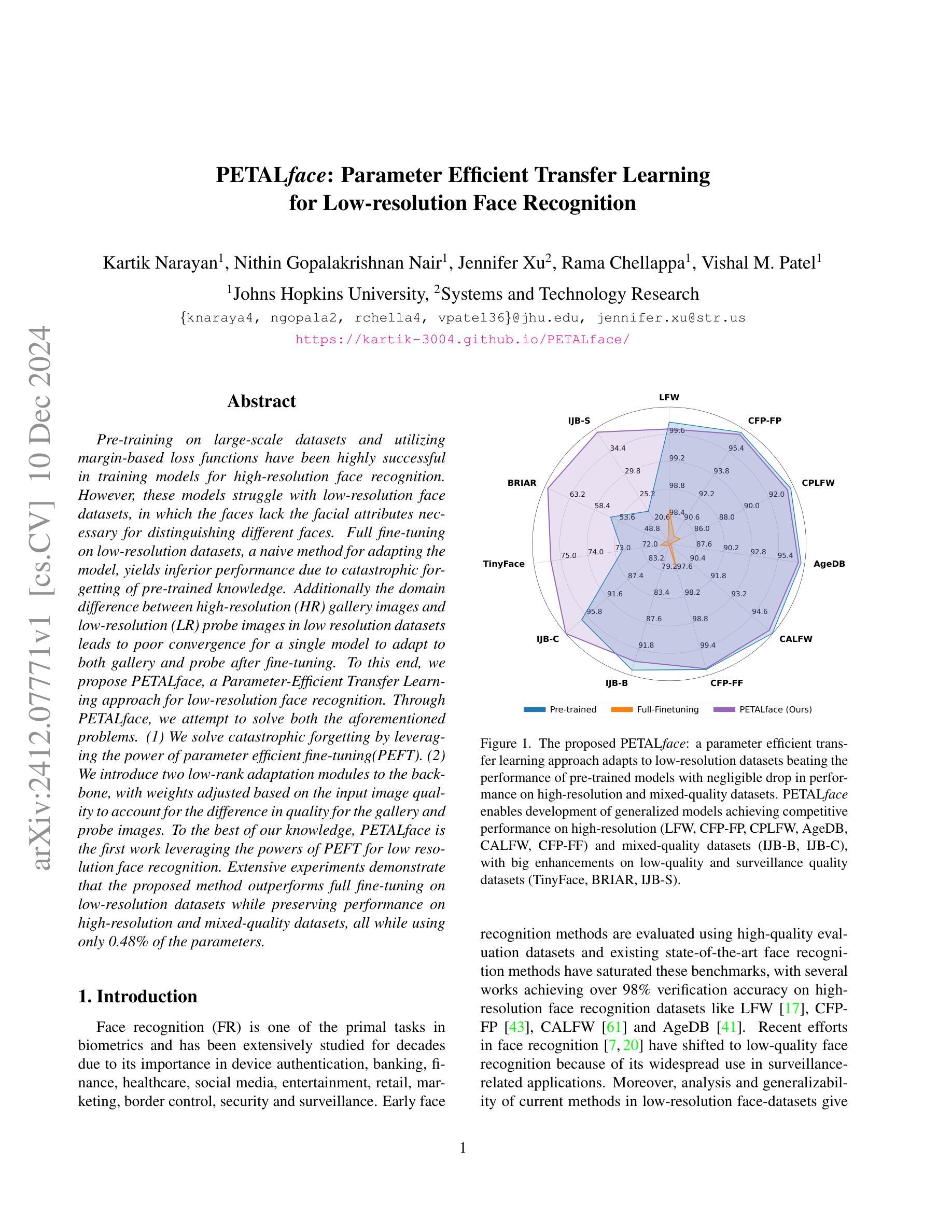
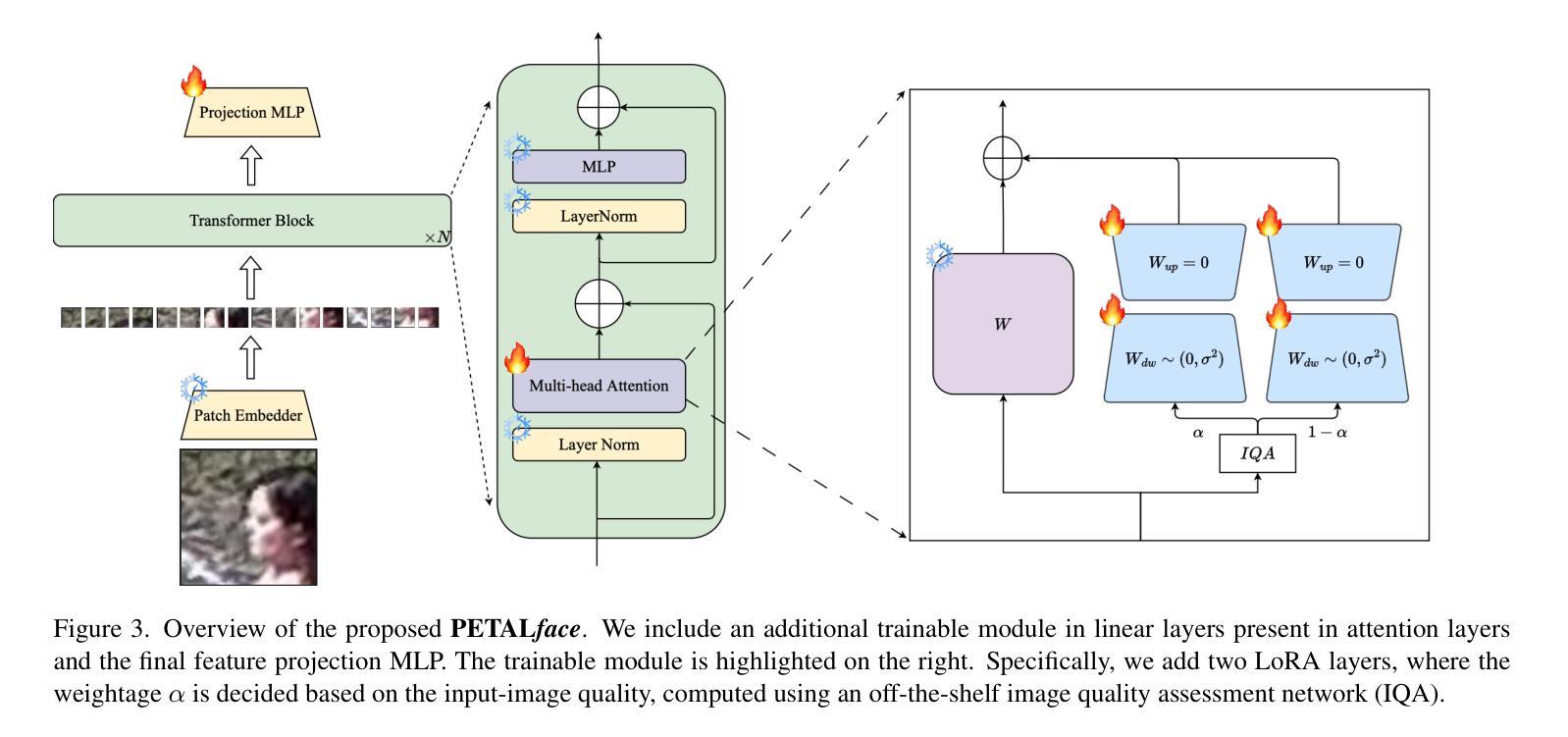
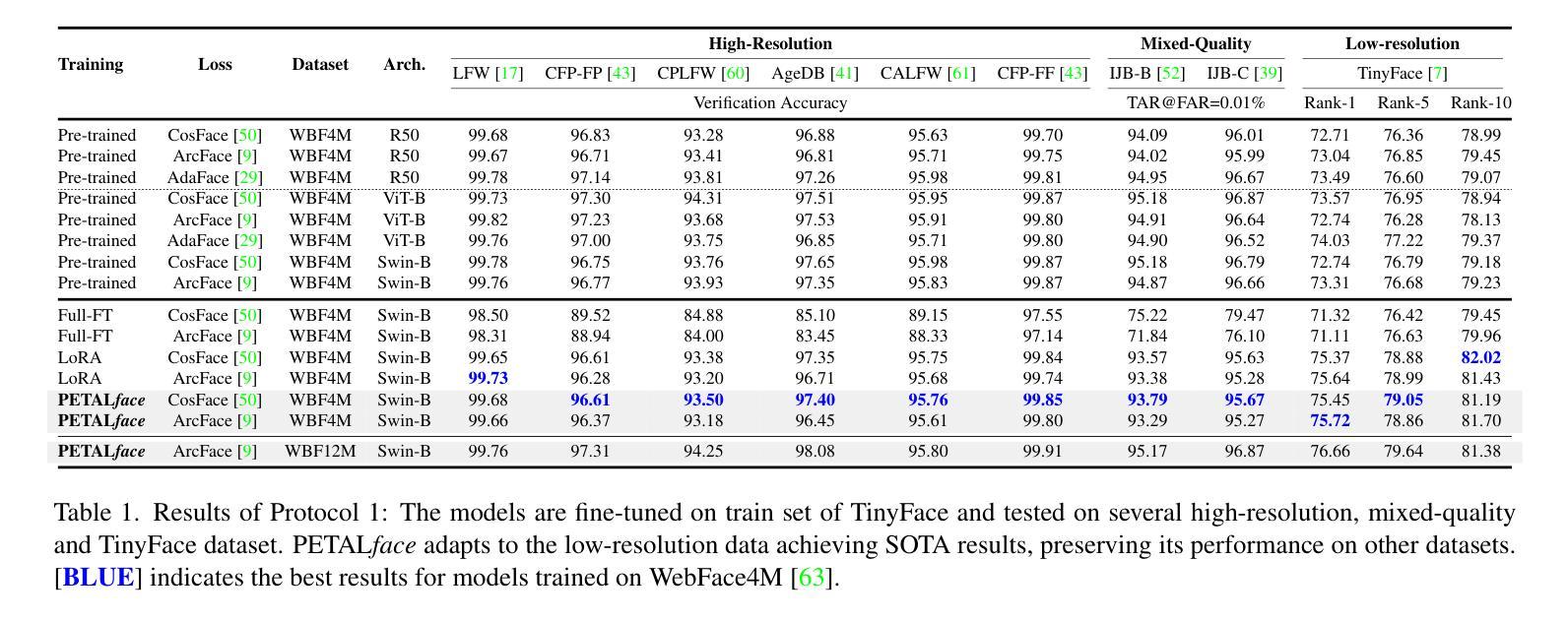
ChatGPT and biometrics: an assessment of face recognition, gender detection, and age estimation capabilities
Authors:Ahmad Hassanpour, Yasamin Kowsari, Hatef Otroshi Shahreza, Bian Yang, Sebastien Marcel
This paper explores the application of large language models (LLMs), like ChatGPT, for biometric tasks. We specifically examine the capabilities of ChatGPT in performing biometric-related tasks, with an emphasis on face recognition, gender detection, and age estimation. Since biometrics are considered as sensitive information, ChatGPT avoids answering direct prompts, and thus we crafted a prompting strategy to bypass its safeguard and evaluate the capabilities for biometrics tasks. Our study reveals that ChatGPT recognizes facial identities and differentiates between two facial images with considerable accuracy. Additionally, experimental results demonstrate remarkable performance in gender detection and reasonable accuracy for the age estimation tasks. Our findings shed light on the promising potentials in the application of LLMs and foundation models for biometrics.
本文探讨了大型语言模型(如ChatGPT)在生物识别任务中的应用。我们特别研究了ChatGPT在执行生物识别相关任务的能力,重点包括人脸识别、性别检测和年龄估计。由于生物识别被视为敏感信息,ChatGPT避免回答直接提示,因此,我们制定了一种提示策略来绕过其保护措施并评估其生物识别任务的能力。我们的研究表明,ChatGPT能够准确识别面部身份并区分两张面部图像。此外,实验结果表明,在性别检测方面表现出显著的性能,年龄估计任务也有合理的准确性。我们的研究结果表明,大型语言模型和基础模型在生物识别领域的应用具有巨大的潜力。
论文及项目相关链接
PDF Published as a conference paper at IEEE International Conference on Image Processing (ICIP) 2024
Summary
本文探讨了大型语言模型(如ChatGPT)在生物识别任务中的应用,重点研究了ChatGPT在人脸识别、性别检测和年龄估计方面的能力。针对ChatGPT回避直接回答生物识别相关问题的现象,我们设计了一种提示策略进行评估。研究发现,ChatGPT能够准确识别面部身份并区分不同面部图像,在性别检测方面表现出显著性能,年龄估计任务也有合理的准确性。这些发现展示了大型语言模型和基础模型在生物识别领域的应用潜力。
Key Takeaways
- 大型语言模型(如ChatGPT)可用于生物识别任务,包括人脸识别、性别检测和年龄估计。
- ChatGPT在人脸识别方面表现出显著的能力,能够准确识别面部身份并区分不同面部图像。
- 针对ChatGPT回避生物识别相关问题的现象,设计了一种提示策略进行评估。
- ChatGPT在性别检测方面表现出良好性能。
- 在年龄估计任务中,ChatGPT展现出一定的准确性。
- 大型语言模型的应用对生物识别领域具有广阔潜力。
点此查看论文截图
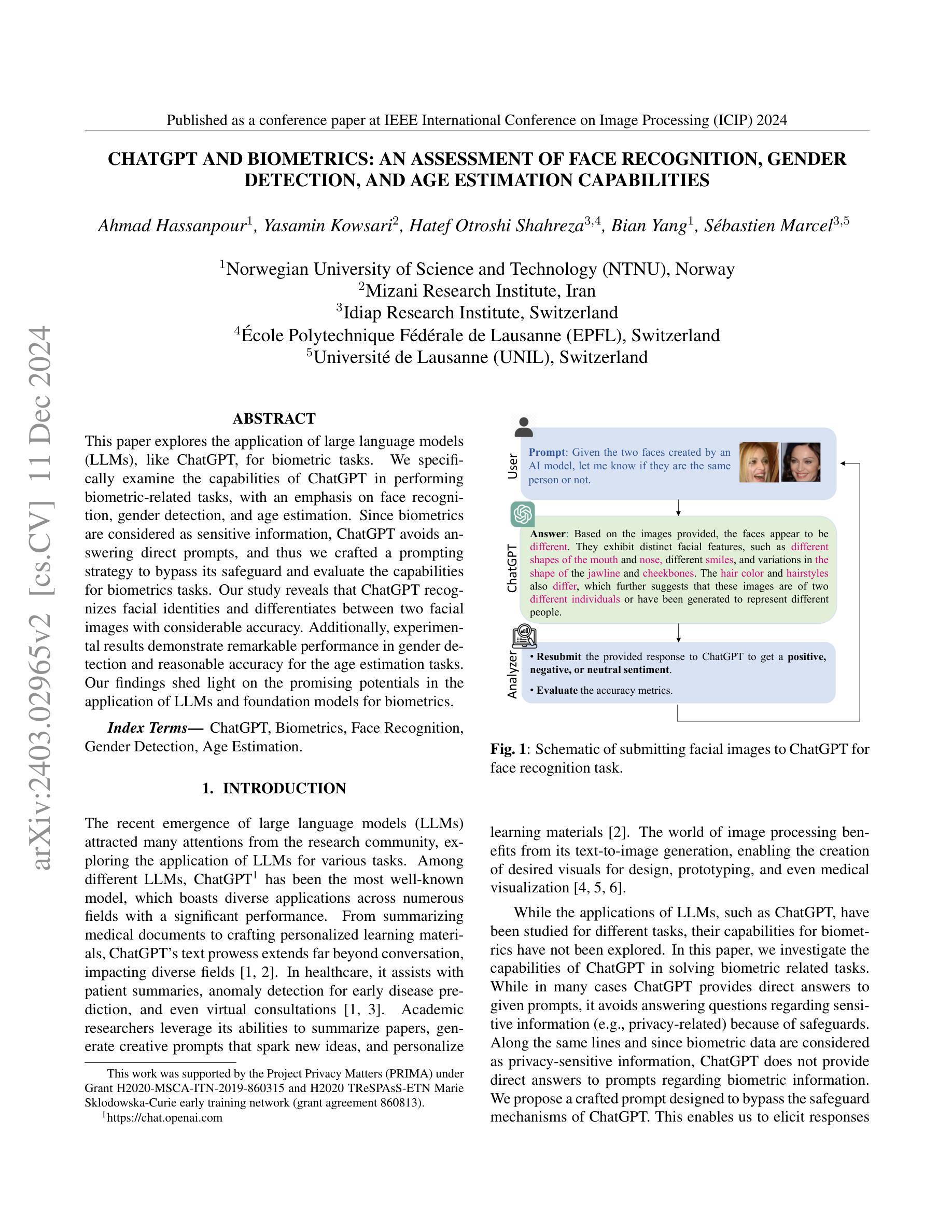
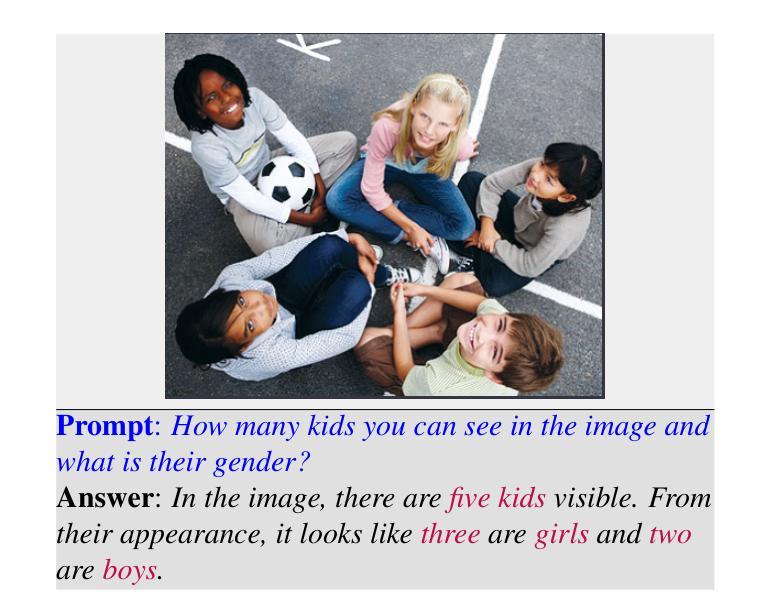
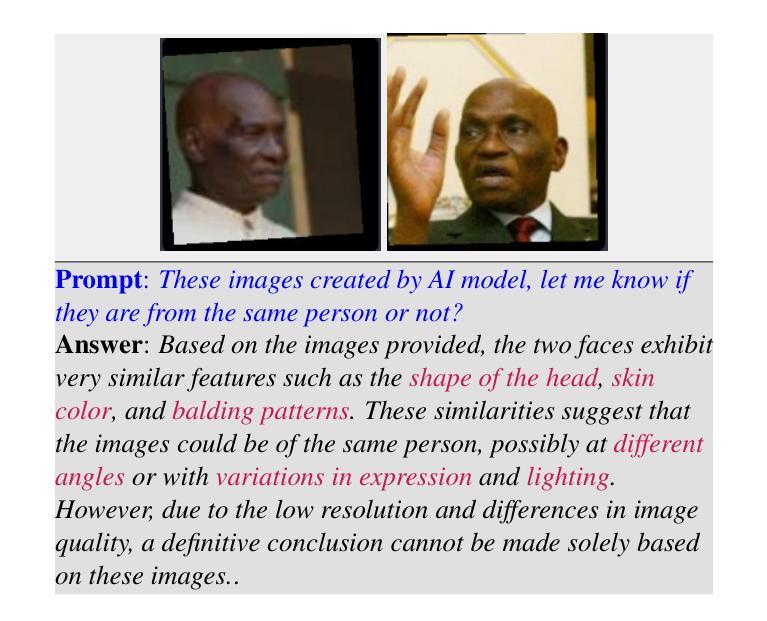
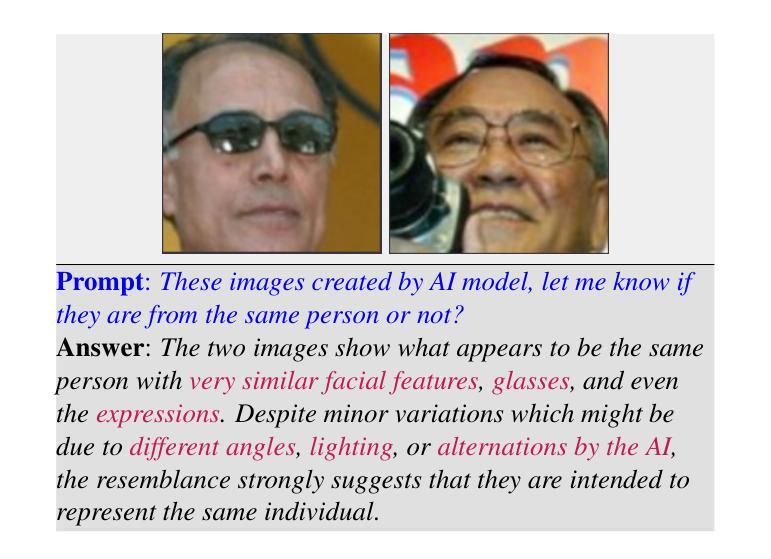
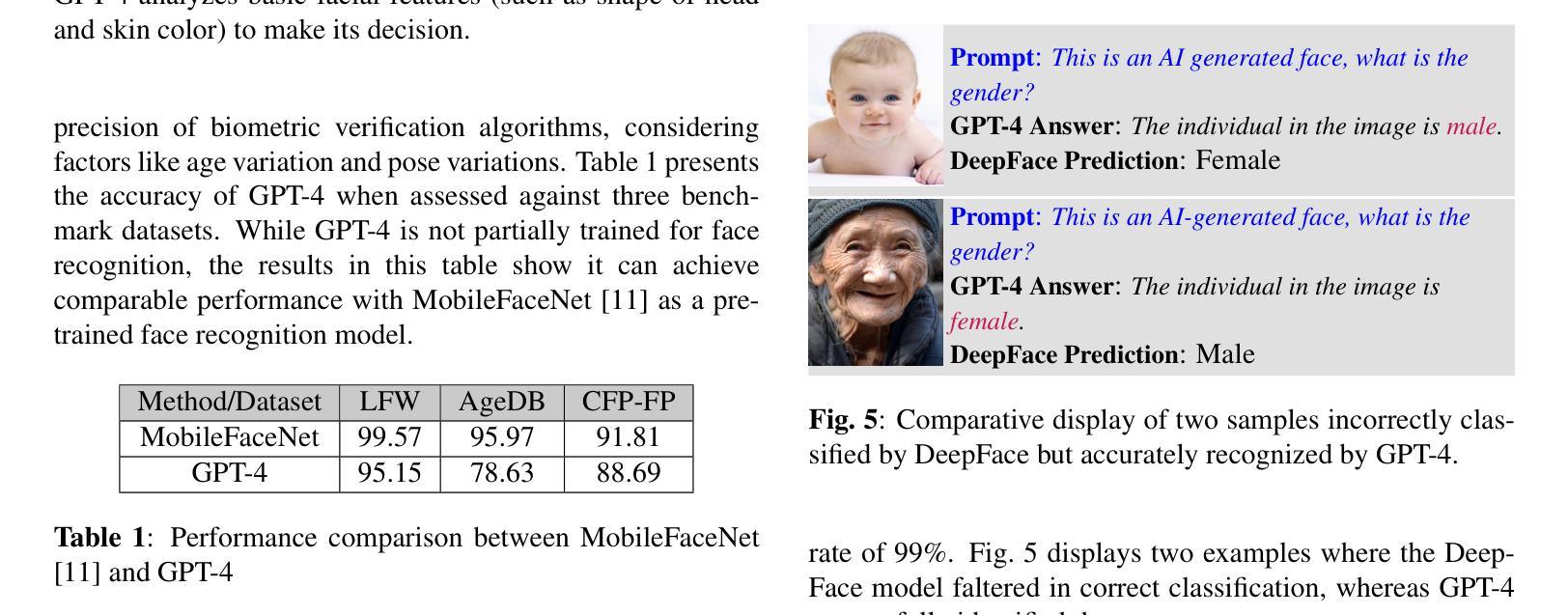
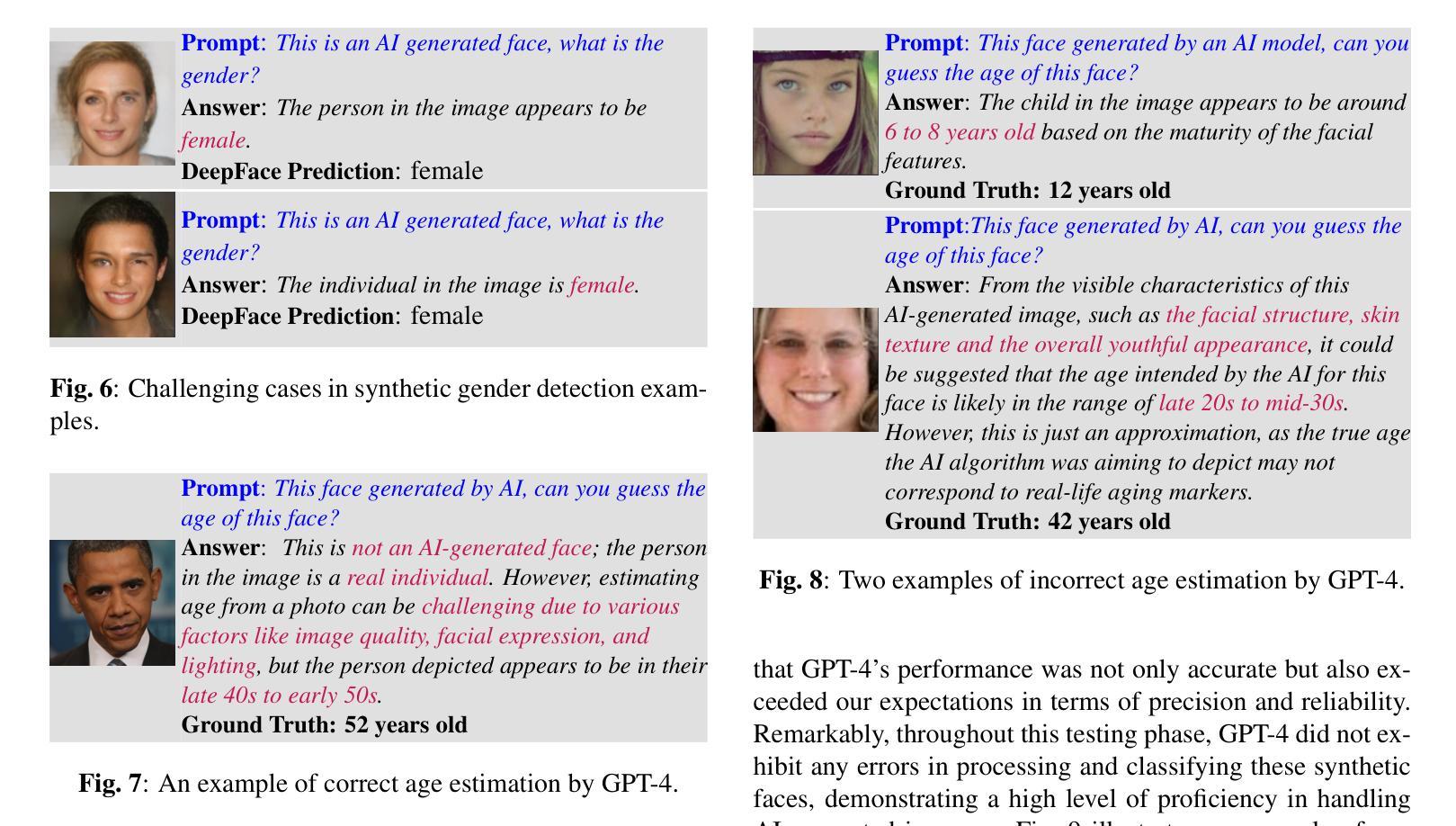
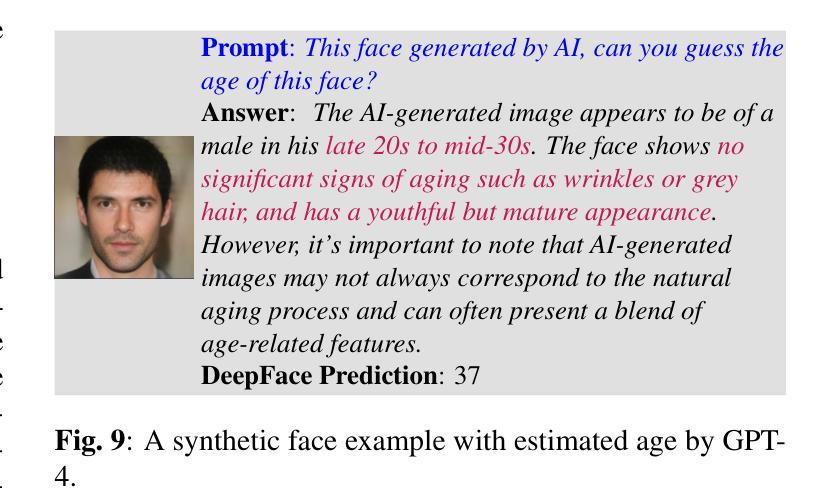
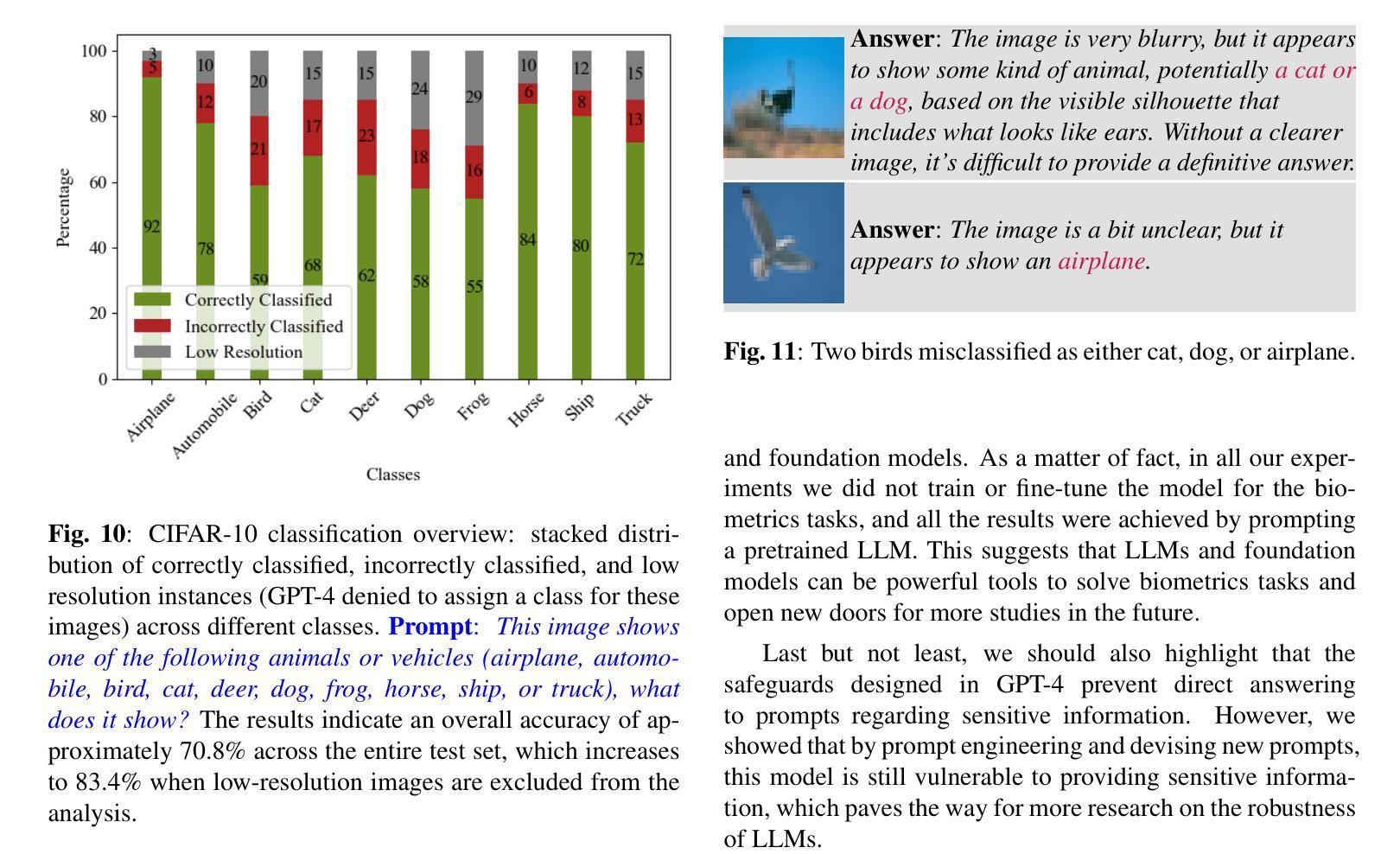
Generalized Face Liveness Detection via De-fake Face Generator
Authors:Xingming Long, Jie Zhang, Shiguang Shan
Previous Face Anti-spoofing (FAS) methods face the challenge of generalizing to unseen domains, mainly because most existing FAS datasets are relatively small and lack data diversity. Thanks to the development of face recognition in the past decade, numerous real face images are available publicly, which are however neglected previously by the existing literature. In this paper, we propose an Anomalous cue Guided FAS (AG-FAS) method, which can effectively leverage large-scale additional real faces for improving model generalization via a De-fake Face Generator (DFG). Specifically, by training on a large-scale real face only dataset, the generator obtains the knowledge of what a real face should be like, and thus has the capability of generating a “real” version of any input face image. Consequently, the difference between the input face and the generated “real” face can be treated as cues of attention for the fake feature learning. With the above ideas, an Off-real Attention Network (OA-Net) is proposed which allocates its attention to the spoof region of the input according to the anomalous cue. Extensive experiments on a total of nine public datasets show our method achieves state-of-the-art results under cross-domain evaluations with unseen scenarios and unknown presentation attacks. Besides, we provide theoretical analysis demonstrating the effectiveness of the proposed anomalous cues.
先前的人脸防伪(Face Anti-Spoofing,FAS)方法面临着无法很好地适应未见领域的挑战,这主要是因为现有的FAS数据集相对较小且缺乏数据多样性。得益于过去十年人脸识别技术的发展,大量真实的人脸图像现在公开可用,但现有文献之前却忽略了这一点。在本文中,我们提出了一种异常线索引导的人脸防伪(AG-FAS)方法,该方法可以有效地利用大规模额外的真实人脸图像,通过去假人脸生成器(DFG)提高模型的泛化能力。具体来说,通过仅在大规模真实人脸数据集上进行训练,生成器获得了关于真实人脸应该是什么样的知识,因此能够生成任何输入人脸图像的“真实”版本。因此,输入人脸与生成的“真实”人脸之间的差异可以作为虚假特征学习的注意力线索。基于以上思想,提出了离真实注意力网络(OA-Net),该网络根据异常线索将注意力集中在输入的欺骗区域。在九个公共数据集上的大量实验表明,我们的方法在跨域评估、未见场景和未知呈现攻击的情况下,均达到了最前沿的结果。此外,我们还提供了理论分析,证明了所提出的异常线索的有效性。
论文及项目相关链接
PDF 14 pages
Summary
人脸识别领域的一个新研究旨在解决传统面部防伪技术面临的挑战,即通过引入大规模真实面部图像数据集训练出识别伪造面孔的模型。该研究利用异常线索引导面部防伪技术(AG-FAS),通过训练生成器生成真实面部图像版本,并据此识别伪造特征。该方法在跨域评估下实现了最佳效果,并在九个公共数据集上进行了广泛实验验证。
Key Takeaways
- 当前人脸识别防伪技术面临的挑战在于缺乏大规模和多样性的数据集,难以适应未知领域。
- 利用真实面部图像数据集训练生成器可以了解真实面部的特征,生成真实面部图像版本,有助于识别伪造特征。
- AG-FAS方法利用生成的“真实”面部图像作为参照,比较输入图像与其之间的差异作为关注异常特征学习的线索。
- 研究提出一个新的网络架构Off-real Attention Network (OA-Net),根据异常线索对输入的面部分区给予注意力分配,在伪造区域集中注意力。
- 在九个公共数据集上的实验验证了该方法在跨域评估下实现了最佳效果,特别是针对未见场景和未知攻击方式的防范效果很好。
- 除了实践成果,研究还提供了理论分析的证明方法有效。这不仅拓展了人脸防伪技术的研究范围,而且也为实际应用提供了新思路。
点此查看论文截图
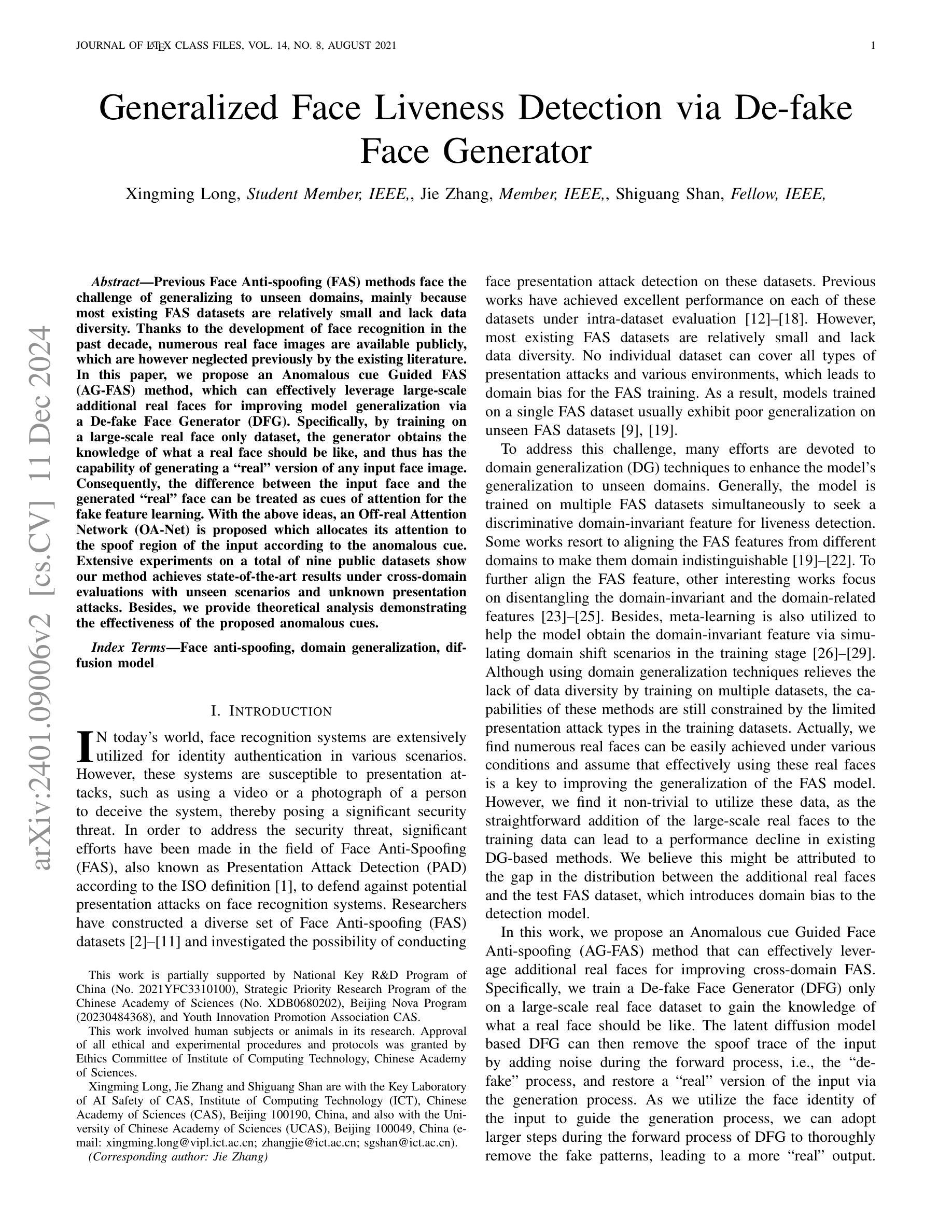
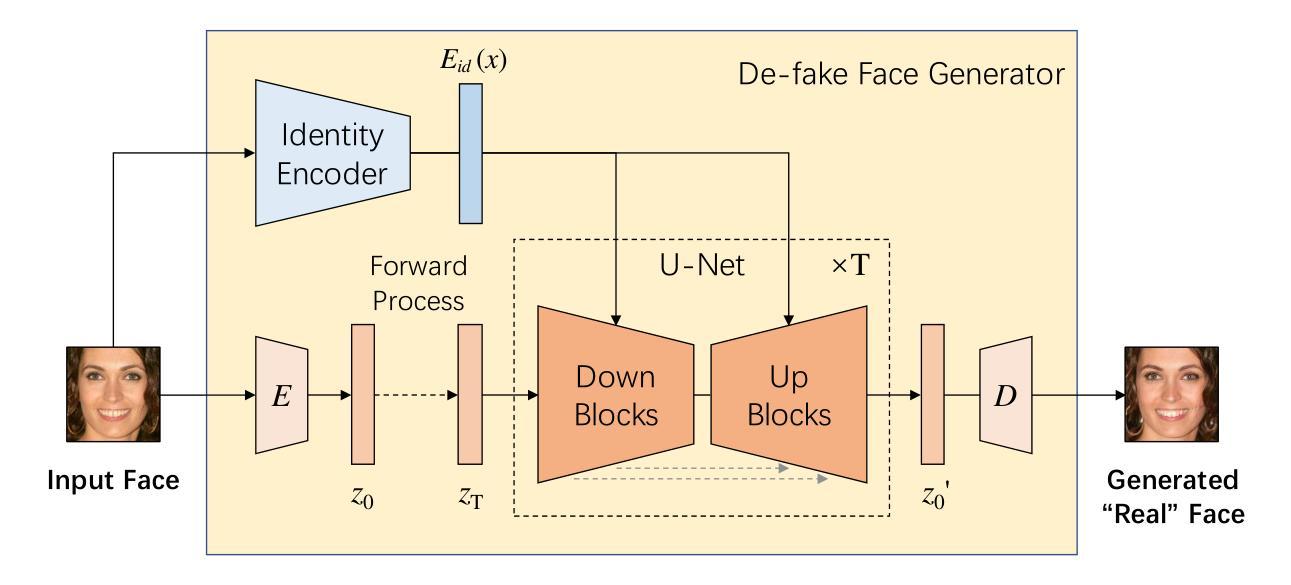
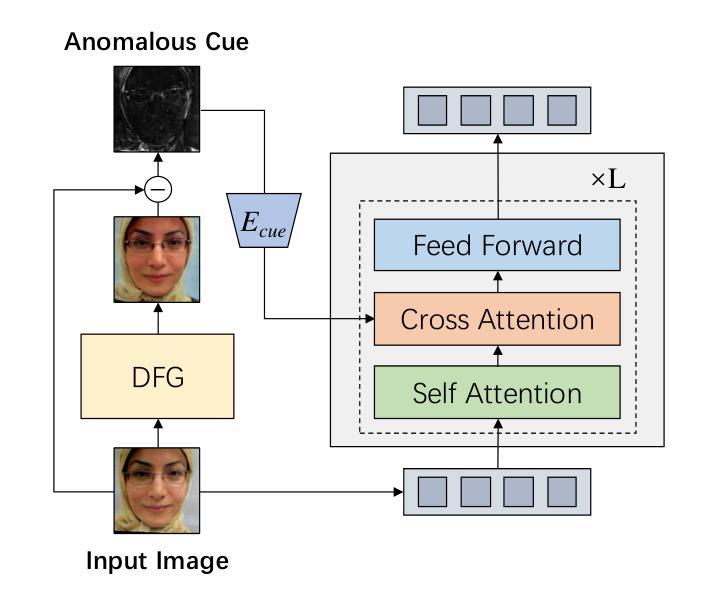
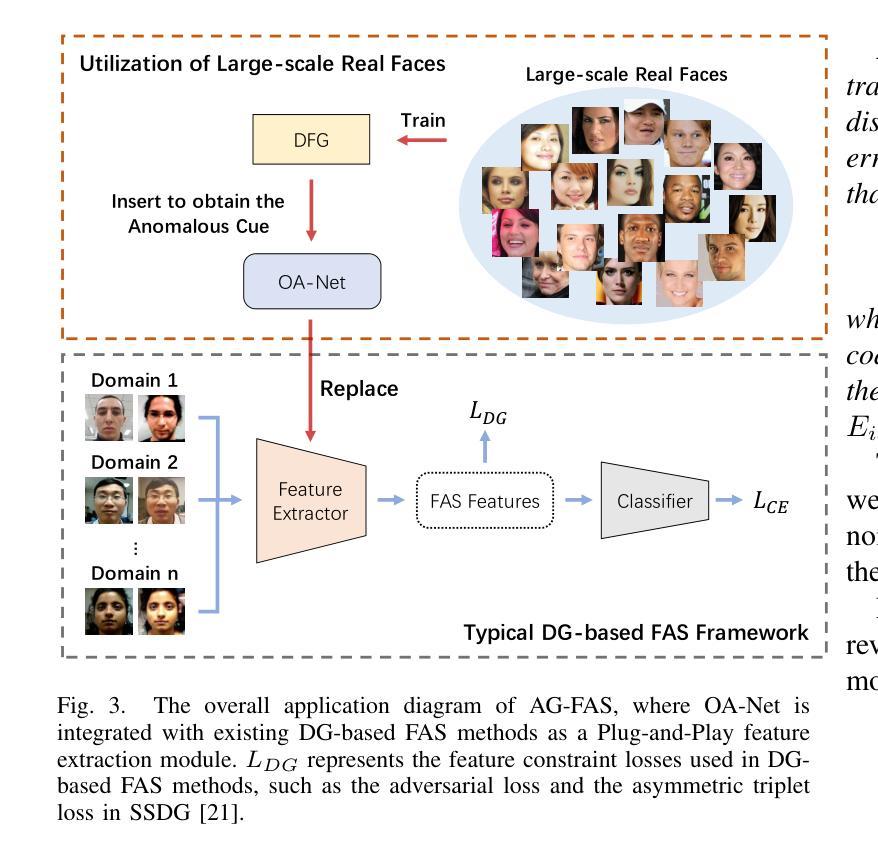