⚠️ 以下所有内容总结都来自于 大语言模型的能力,如有错误,仅供参考,谨慎使用
🔴 请注意:千万不要用于严肃的学术场景,只能用于论文阅读前的初筛!
💗 如果您觉得我们的项目对您有帮助 ChatPaperFree ,还请您给我们一些鼓励!⭐️ HuggingFace免费体验
2024-12-12 更新
Exploring What Why and How: A Multifaceted Benchmark for Causation Understanding of Video Anomaly
Authors:Hang Du, Guoshun Nan, Jiawen Qian, Wangchenhui Wu, Wendi Deng, Hanqing Mu, Zhenyan Chen, Pengxuan Mao, Xiaofeng Tao, Jun Liu
Recent advancements in video anomaly understanding (VAU) have opened the door to groundbreaking applications in various fields, such as traffic monitoring and industrial automation. While the current benchmarks in VAU predominantly emphasize the detection and localization of anomalies. Here, we endeavor to delve deeper into the practical aspects of VAU by addressing the essential questions: “what anomaly occurred?”, “why did it happen?”, and “how severe is this abnormal event?”. In pursuit of these answers, we introduce a comprehensive benchmark for Exploring the Causation of Video Anomalies (ECVA). Our benchmark is meticulously designed, with each video accompanied by detailed human annotations. Specifically, each instance of our ECVA involves three sets of human annotations to indicate “what”, “why” and “how” of an anomaly, including 1) anomaly type, start and end times, and event descriptions, 2) natural language explanations for the cause of an anomaly, and 3) free text reflecting the effect of the abnormality. Building upon this foundation, we propose a novel prompt-based methodology that serves as a baseline for tackling the intricate challenges posed by ECVA. We utilize “hard prompt” to guide the model to focus on the critical parts related to video anomaly segments, and “soft prompt” to establish temporal and spatial relationships within these anomaly segments. Furthermore, we propose AnomEval, a specialized evaluation metric crafted to align closely with human judgment criteria for ECVA. This metric leverages the unique features of the ECVA dataset to provide a more comprehensive and reliable assessment of various video large language models. We demonstrate the efficacy of our approach through rigorous experimental analysis and delineate possible avenues for further investigation into the comprehension of video anomaly causation.
近期视频异常理解(VAU)的进展为各个领域的应用打开了突破性的大门,如交通监控和工业自动化。虽然当前的VAU基准测试主要强调异常的检测和定位,但在这里,我们努力深入探索VAU的实际方面,通过解决本质问题:“发生了什么异常?”、“为什么发生?”以及“这个异常事件的严重性如何?”来介绍一个用于探索视频异常因果关系的全面基准测试(ECVA)。我们的基准测试经过精心设计,每段视频都附有详细的人类注释。具体来说,我们的ECVA的每个实例都包含三组人类注释,以指示异常的“是什么”、“为什么”和“如何”,包括1)异常类型、开始和结束时间以及事件描述;2)异常原因的自然语言解释;3)反映异常影响的自由文本。在此基础上,我们提出了一种新的基于提示的方法,作为解决ECVA所带来复杂挑战的基础。我们使用“硬提示”来指导模型关注与视频异常片段相关的关键部分,并使用“软提示”在这些异常片段内建立时间和空间关系。此外,我们提出了AnomEval,这是一个专门设计的评估指标,与人类对ECVA的评判标准紧密对齐。该指标利用ECVA数据集的独特功能,为各种视频大型语言模型提供更全面和可靠的评估。我们通过严格的实验分析证明了我们的方法的有效性,并概述了进一步探索视频异常因果理解的可能途径。
论文及项目相关链接
PDF Submitted to IEEE Transactions on Pattern Analysis and Machine Intelligence. arXiv admin note: substantial text overlap with arXiv:2405.00181
Summary:
视频异常理解(VAU)领域最新进展为交通监测、工业自动化等跨领域应用带来了突破性机会。当前基准测试主要关注异常的检测和定位,而本研究致力于挖掘视频异常事件的因果关系的基准测试(ECVA)。每个视频都伴随详细的人类标注,包括异常类型、时间、事件描述、异常原因的自然语言解释以及异常影响的自由文本。本研究提出了基于提示的方法作为解决ECVA挑战的基础,并利用硬提示和软提示建立异常段的时间和空间关系。此外,还提出了与ECVA数据集的人类判断标准紧密对齐的AnomEval评估指标,为视频大语言模型提供更全面可靠的评估。
Key Takeaways:
- 视频异常理解(VAU)领域正快速发展,应用领域广泛,如交通监测和工业自动化。
- 当前主要关注异常的检测和定位,但深入理解异常的因果关系至关重要。
- 引入了一个全面的基准测试(ECVA),包含详细的人类标注,涵盖异常的“是什么”,“为什么”和“严重性”。
- 提出了一种基于提示的方法来处理视频异常的复杂挑战。
- 利用硬提示和软提示指导模型关注关键部分并建立异常段的时间和空间关系。
- 提出了一种新的评估指标AnomEval,与ECVA数据集的人类判断标准紧密对齐。
点此查看论文截图
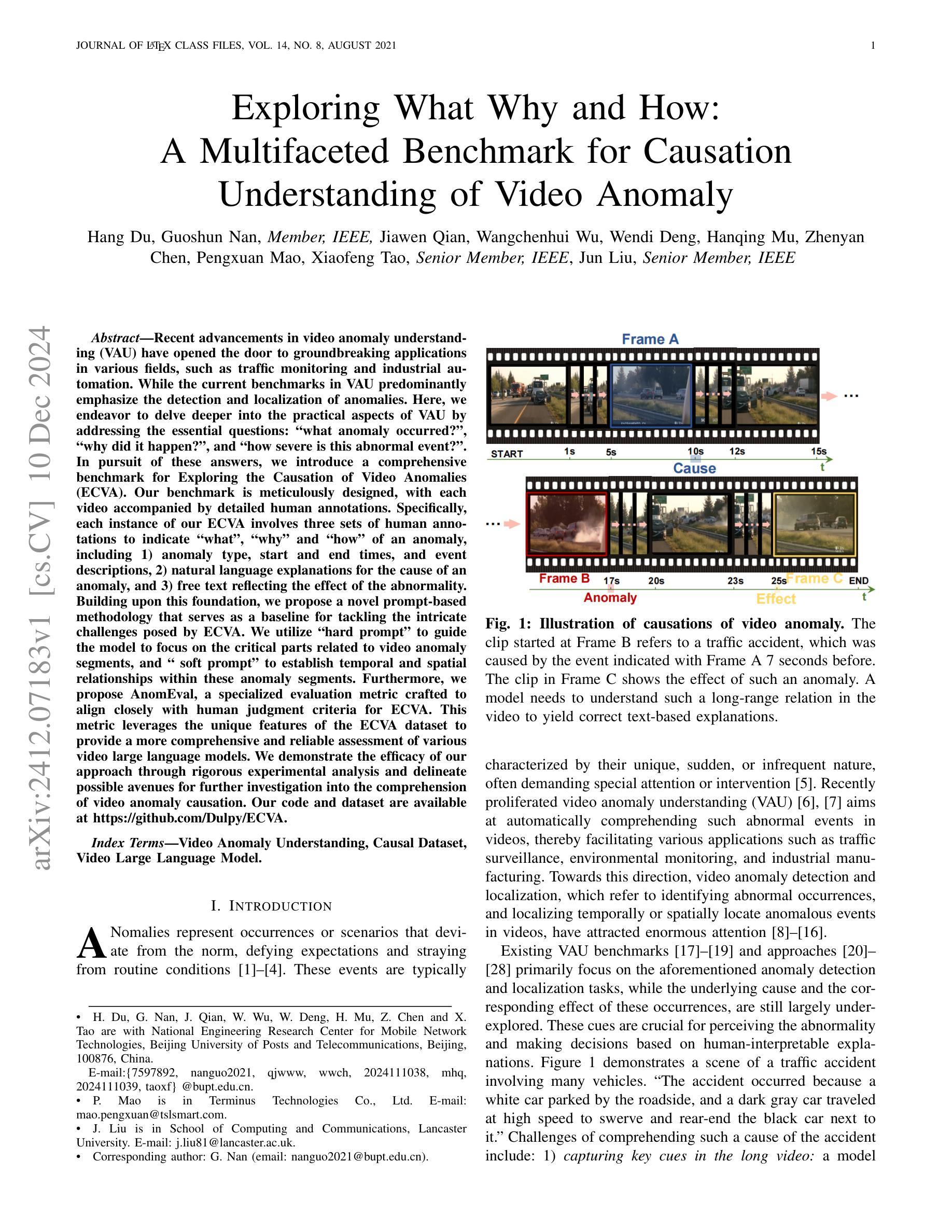
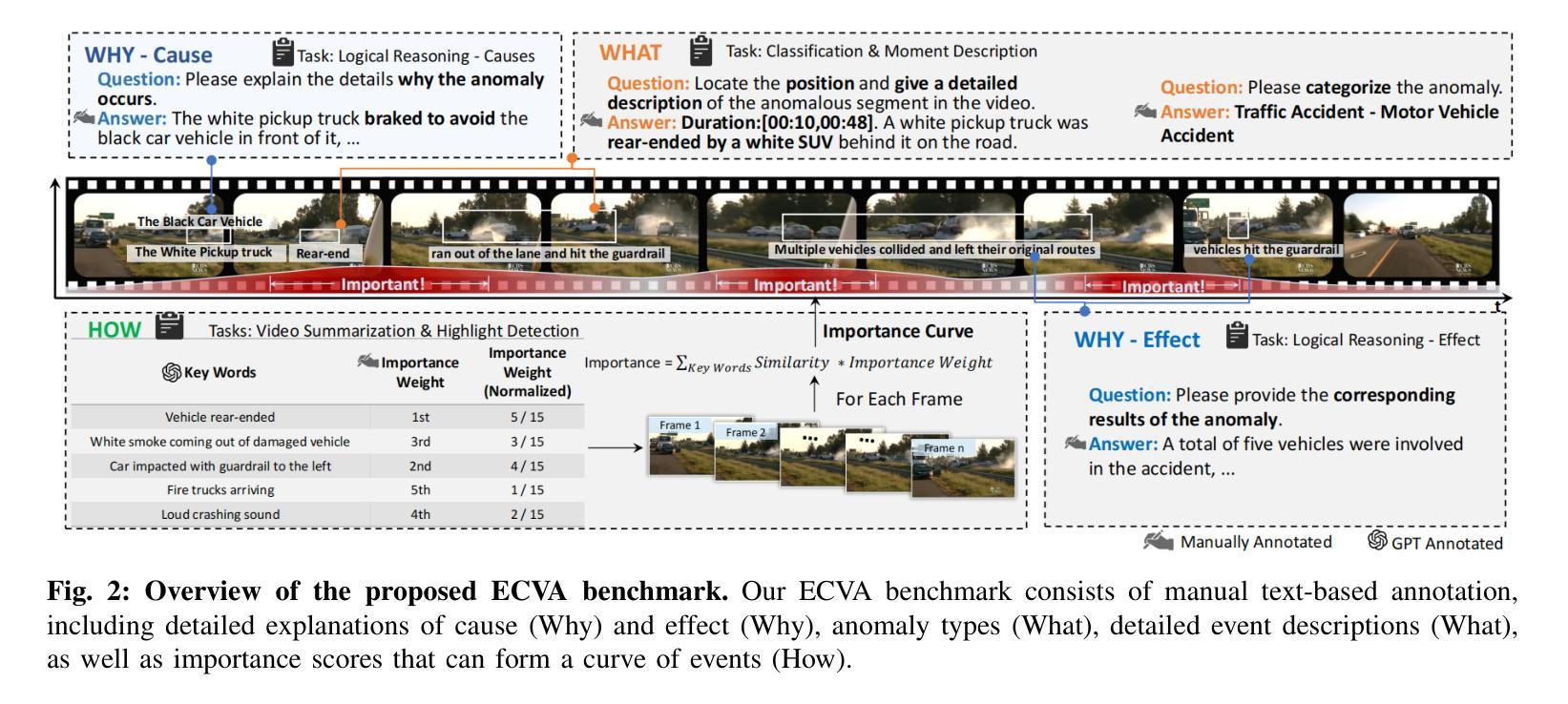
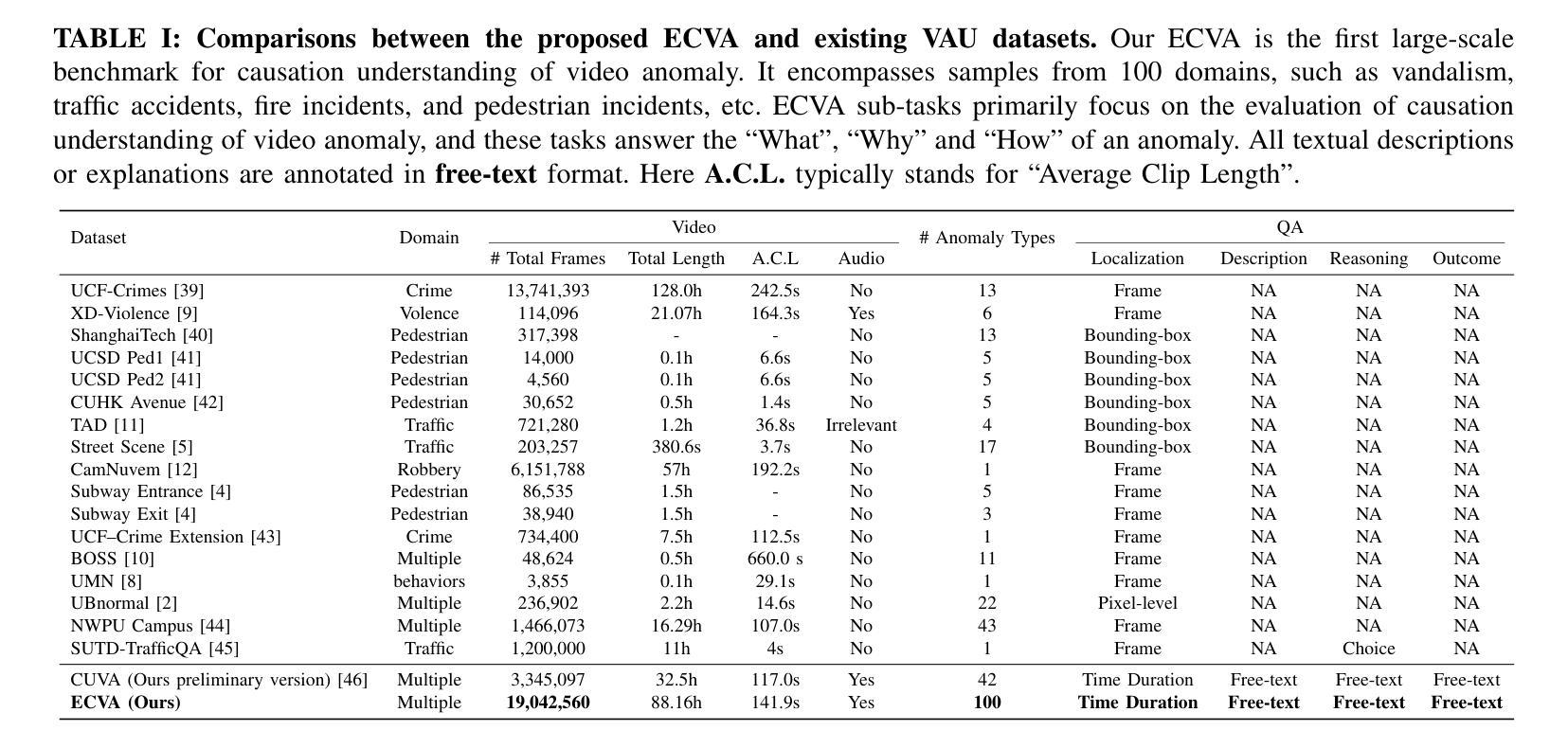
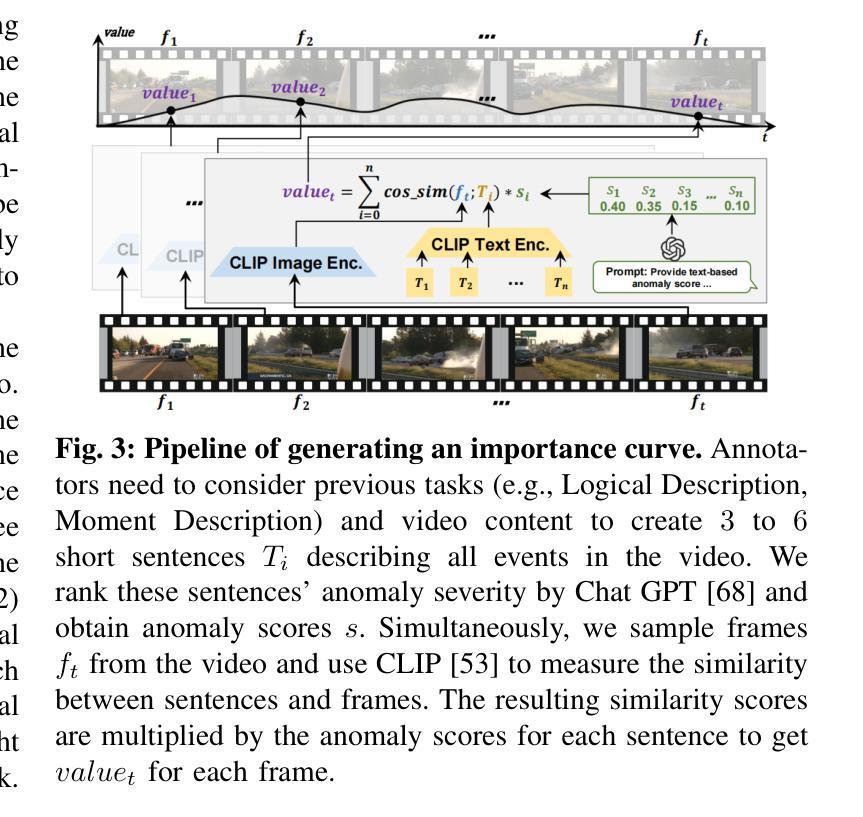
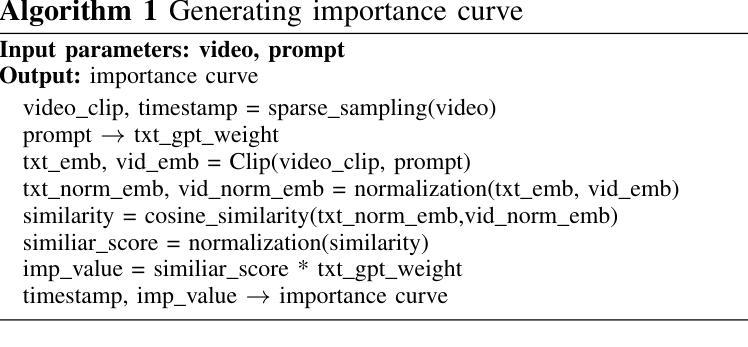
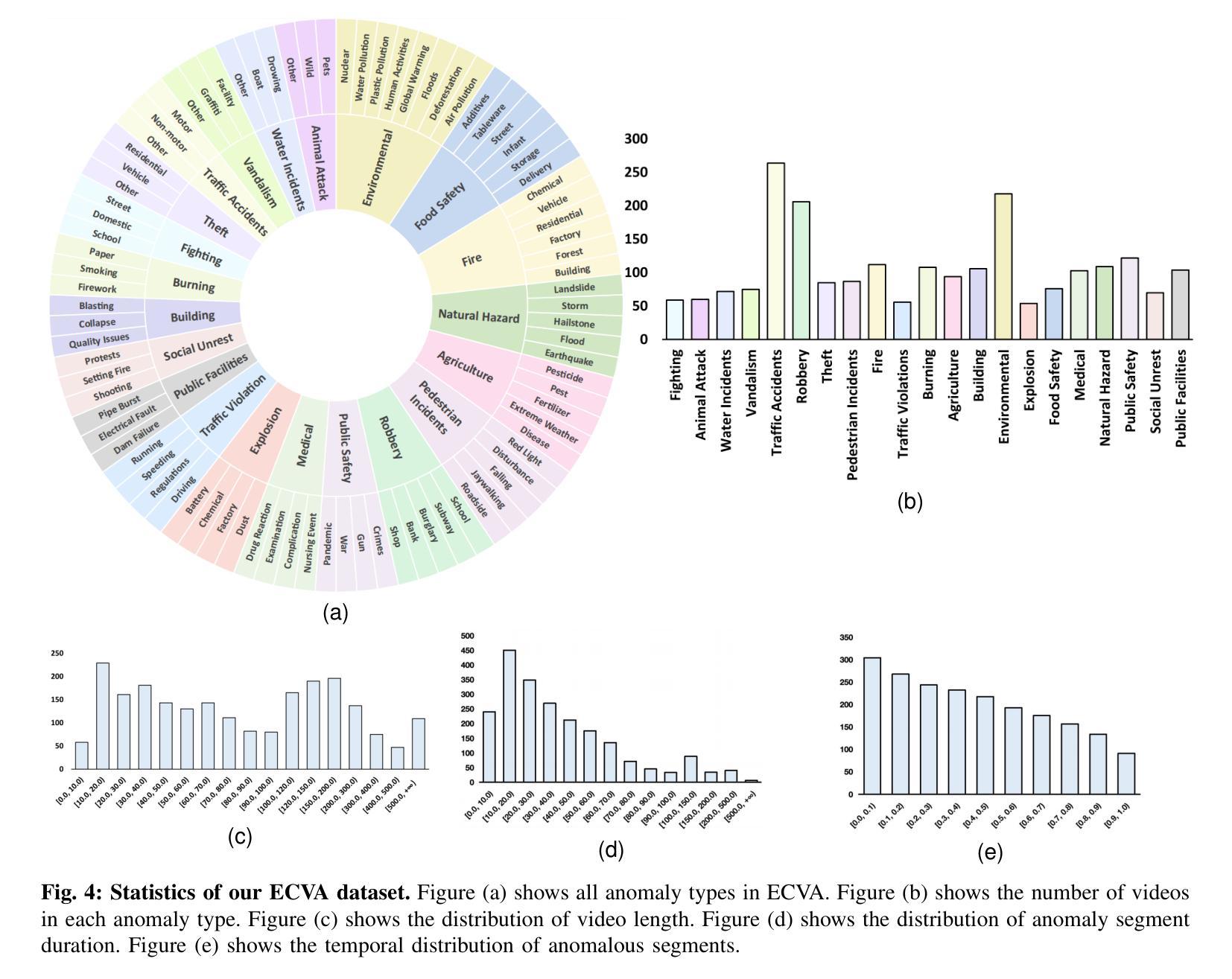
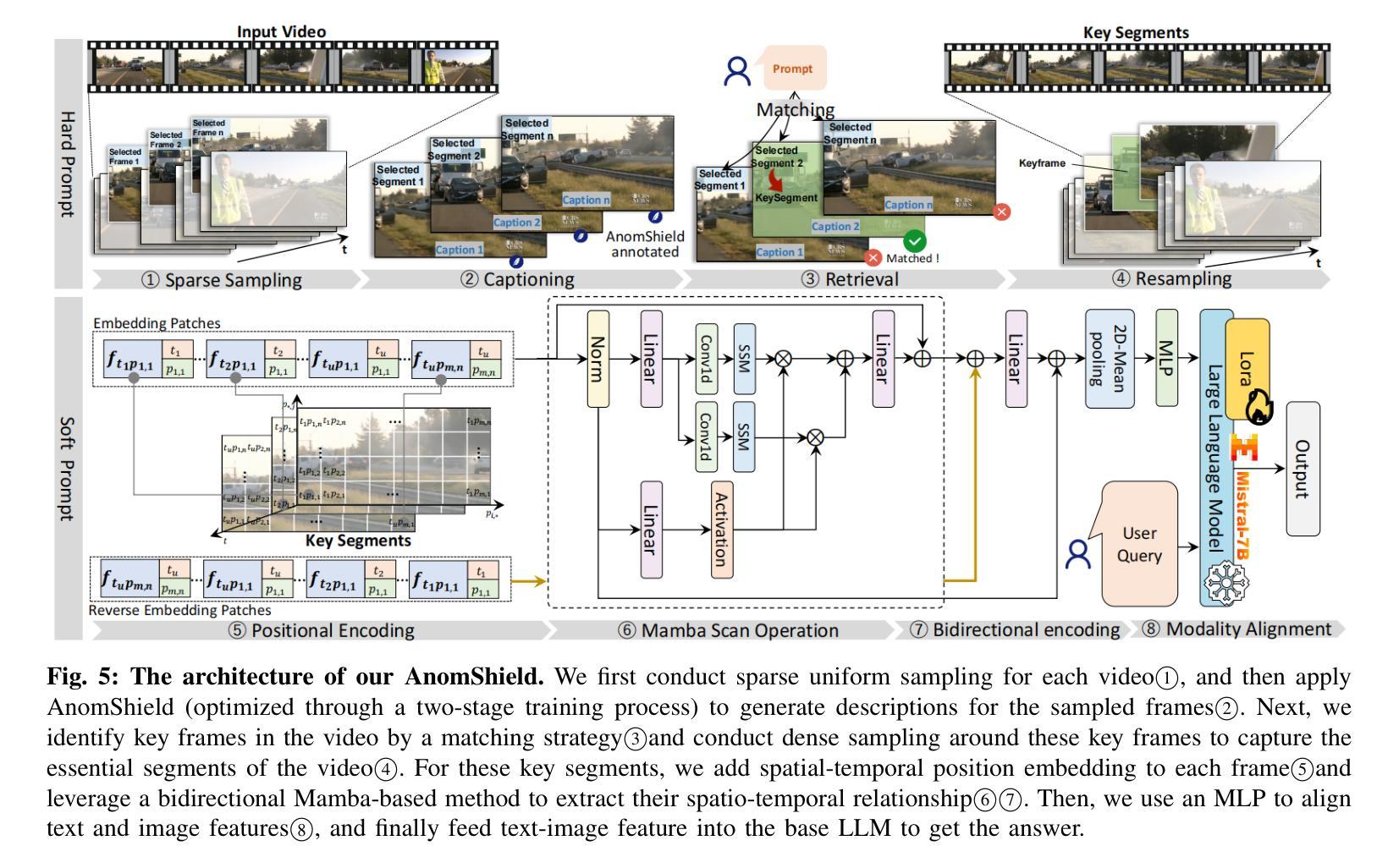
Towards Long Video Understanding via Fine-detailed Video Story Generation
Authors:Zeng You, Zhiquan Wen, Yaofo Chen, Xin Li, Runhao Zeng, Yaowei Wang, Mingkui Tan
Long video understanding has become a critical task in computer vision, driving advancements across numerous applications from surveillance to content retrieval. Existing video understanding methods suffer from two challenges when dealing with long video understanding: intricate long-context relationship modeling and interference from redundancy. To tackle these challenges, we introduce Fine-Detailed Video Story generation (FDVS), which interprets long videos into detailed textual representations. Specifically, to achieve fine-grained modeling of long-temporal content, we propose a Bottom-up Video Interpretation Mechanism that progressively interprets video content from clips to video. To avoid interference from redundant information in videos, we introduce a Semantic Redundancy Reduction mechanism that removes redundancy at both the visual and textual levels. Our method transforms long videos into hierarchical textual representations that contain multi-granularity information of the video. With these representations, FDVS is applicable to various tasks without any fine-tuning. We evaluate the proposed method across eight datasets spanning three tasks. The performance demonstrates the effectiveness and versatility of our method.
长视频理解已成为计算机视觉中的一项关键任务,推动了从监控到内容检索等多个应用的发展。现有的视频理解方法在处理长视频理解时面临两个挑战:复杂的长上下文关系建模和冗余信息的干扰。为了解决这些挑战,我们引入了精细详细视频故事生成(FDVS)方法,它将长视频解释为详细的文本表示。具体来说,为了实现长时内容的精细建模,我们提出了自下而上的视频解释机制,该机制从片段到视频逐步解释视频内容。为了避免视频中冗余信息的干扰,我们引入了语义冗余减少机制,该机制在视觉和文本层面消除了冗余。我们的方法将长视频转换为层次化的文本表示形式,包含视频的多粒度信息。利用这些表示形式,FDVS可广泛应用于各种任务,无需进行微调。我们在三个任务的八个数据集上评估了所提出的方法。性能表现证明了我们的方法的有效性和通用性。
论文及项目相关链接
Summary
长视频理解已成为计算机视觉中的关键任务,推动了从监控到内容检索等多个应用的发展。针对长视频理解,现有方法面临复杂的长上下文关系建模和冗余信息的干扰两大挑战。为解决这些问题,我们提出了精细详细视频故事生成(FDVS)方法,将长视频转化为详细的文本表示。通过自下而上的视频解释机制和语义冗余减少机制,实现了对长视频内容的精细颗粒度建模和冗余信息的避免。该方法将长视频转换为层次化的文本表示,包含视频的多粒度信息,可广泛应用于各种任务而无需微调。实验结果表明,该方法的有效性和通用性。
Key Takeaways
- 长视频理解已成为计算机视觉的关键任务,推动多个应用领域的发展。
- 现有视频理解方法面临复杂长上下文关系建模和冗余信息干扰的挑战。
- 提出的Fine-Detailed Video Story generation(FDVS)方法将长视频转化为详细的文本表示。
- 通过自下而上的视频解释机制实现长视频内容的精细颗粒度建模。
- 语义冗余减少机制避免了冗余信息的干扰。
- FDVS方法将长视频转换为层次化的文本表示,包含视频的多粒度信息。
点此查看论文截图
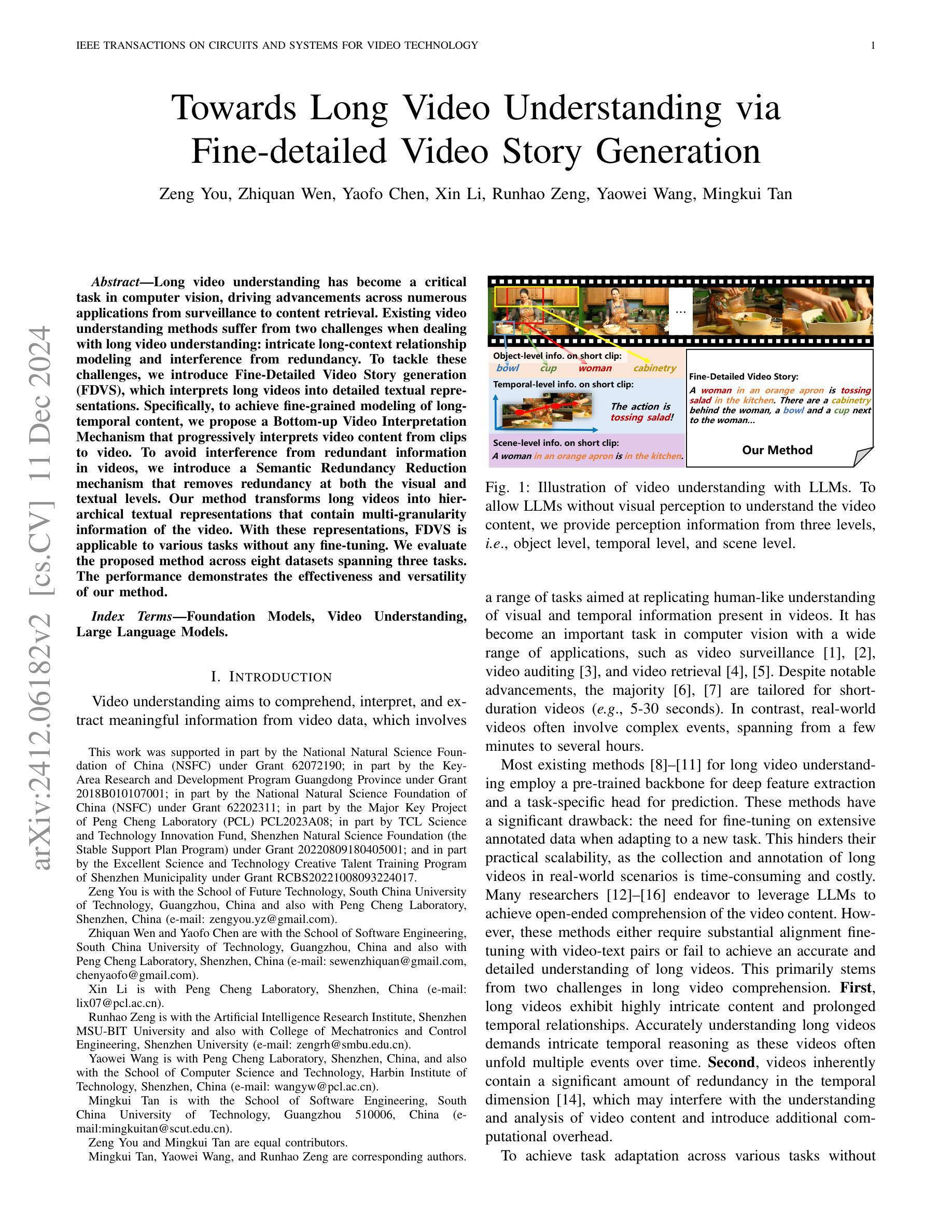
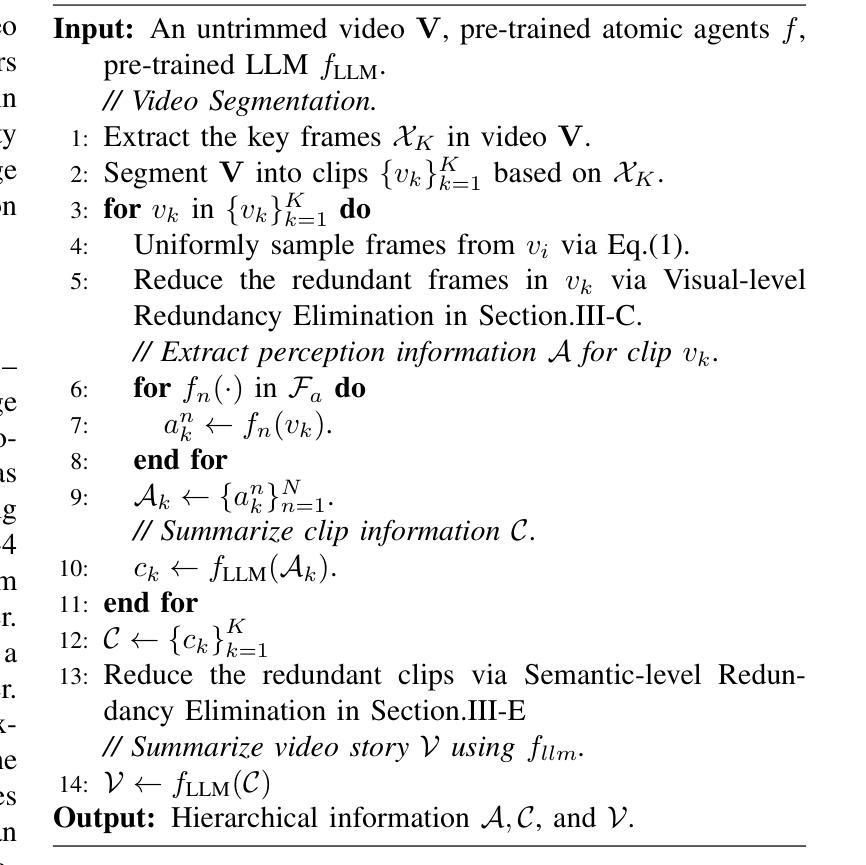
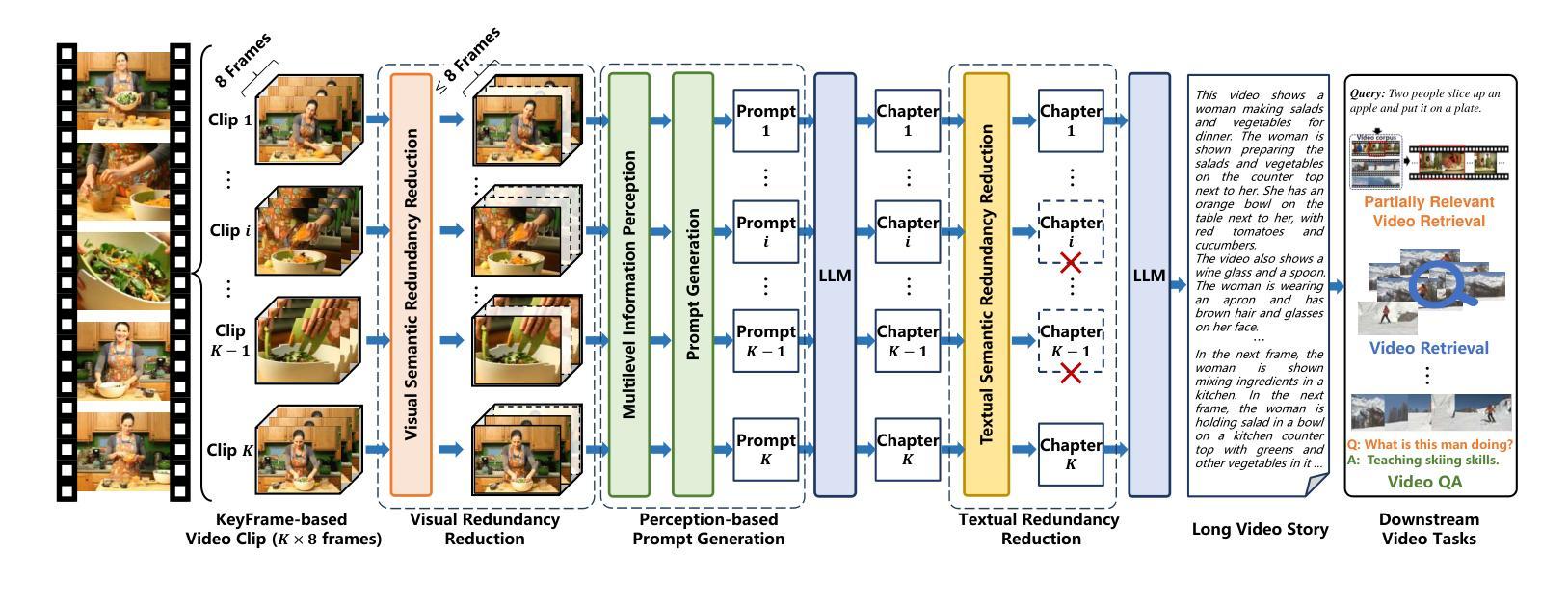
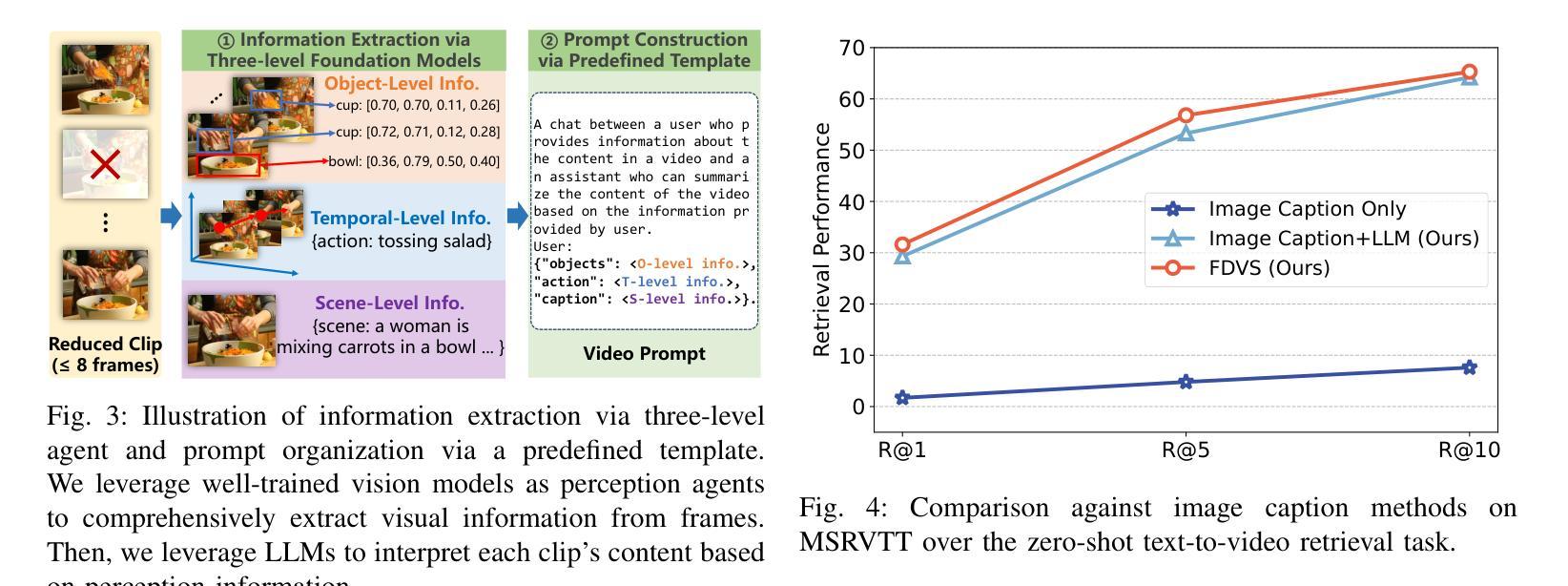
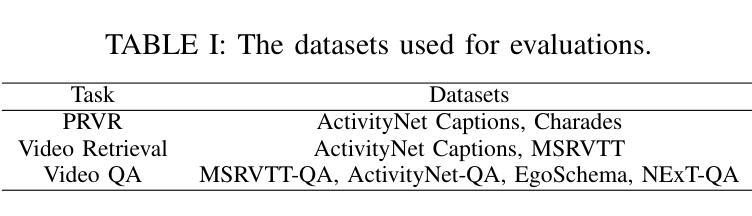
LinVT: Empower Your Image-level Large Language Model to Understand Videos
Authors:Lishuai Gao, Yujie Zhong, Yingsen Zeng, Haoxian Tan, Dengjie Li, Zheng Zhao
Large Language Models (LLMs) have been widely used in various tasks, motivating us to develop an LLM-based assistant for videos. Instead of training from scratch, we propose a module to transform arbitrary well-trained image-based LLMs into video-LLMs (after being trained on video data). To better adapt image-LLMs for processing videos, we introduce two design principles: linear transformation to preserve the original visual-language alignment and representative information condensation from redundant video content. Guided by these principles, we propose a plug-and-play Linear Video Tokenizer(LinVT), which enables existing image-LLMs to understand videos. We benchmark LinVT with six recent visual LLMs: Aquila, Blip-3, InternVL2, Mipha, Molmo and Qwen2-VL, showcasing the high compatibility of LinVT. LinVT-based LLMs achieve state-of-the-art performance across various video benchmarks, illustrating the effectiveness of LinVT in multi-modal video understanding.
大规模语言模型(LLMs)已在各种任务中得到了广泛应用,这促使我们开发基于LLM的视频助理。我们不是从头开始训练,而是提出一个模块,将任意的、基于图像的良好训练的LLMs转化为在视频数据训练后的视频LLMs。为了更好地适应图像LLMs处理视频,我们引入了两个设计原则:线性变换以保留原始视觉语言对齐和从冗余视频内容中凝练代表性信息。在这些原则的指导下,我们提出了即插即用的线性视频令牌化器(LinVT),使现有的图像LLMs能够理解视频。我们以LinVT与最近的六个视觉LLMs进行基准测试:Aquila、Blip-3、InternVL2、Mipha、Molmo和Qwen2-VL,展示了LinVT的高度兼容性。基于LinVT的LLMs在各种视频基准测试中达到了最先进的性能,证明了LinVT在多模态视频理解中的有效性。
论文及项目相关链接
Summary
基于大型语言模型(LLM)在多个任务中的广泛应用,我们提出了一种基于LLM的视频助理。为解决从零开始训练视频LLM的难题,我们提出了一种将预训练的图像LLM转换为视频LLM的模块(该模块经过视频数据训练)。为更好地适应视频处理,我们提出了两个设计原则:保持原始视觉语言对齐的线性转换和从冗余视频内容中提取代表性信息。基于这些原则,我们提出了一种即插即用的线性视频令牌器(LinVT),使现有图像LLM能够理解视频。我们对LinVT进行了基准测试,涵盖了六种最新的视觉LLM,展示了LinVT的高度兼容性。基于LinVT的LLM在多个视频基准测试中达到了最先进的性能,证明了LinVT在多模态视频理解中的有效性。
Key Takeaways
- 提出了将预训练的图像LLM转换为视频LLM的模块。
- 采用线性转换和代表性信息冷凝两个设计原则,以适应视频处理。
- 提出了一种即插即用的线性视频令牌器(LinVT),增强了现有图像LLM的视频理解能力。
- LinVT与六种最新的视觉LLM兼容。
- 基于LinVT的LLM在多个视频基准测试中表现优异。
- LinVT有助于实现多模态视频理解。
- 该方法通过引入线性转换和冗余信息冷凝技术,有效提高了视频理解的准确性和效率。
点此查看论文截图
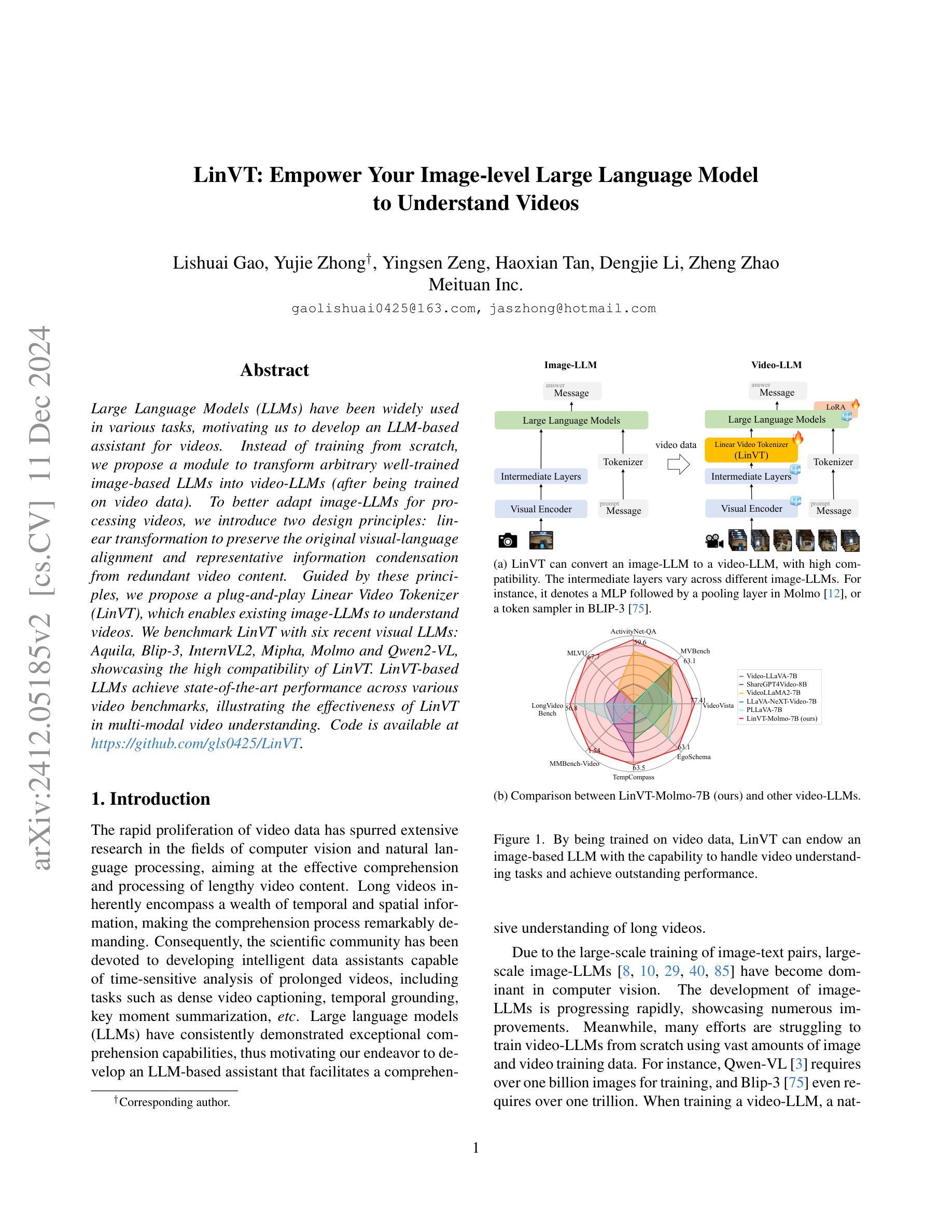
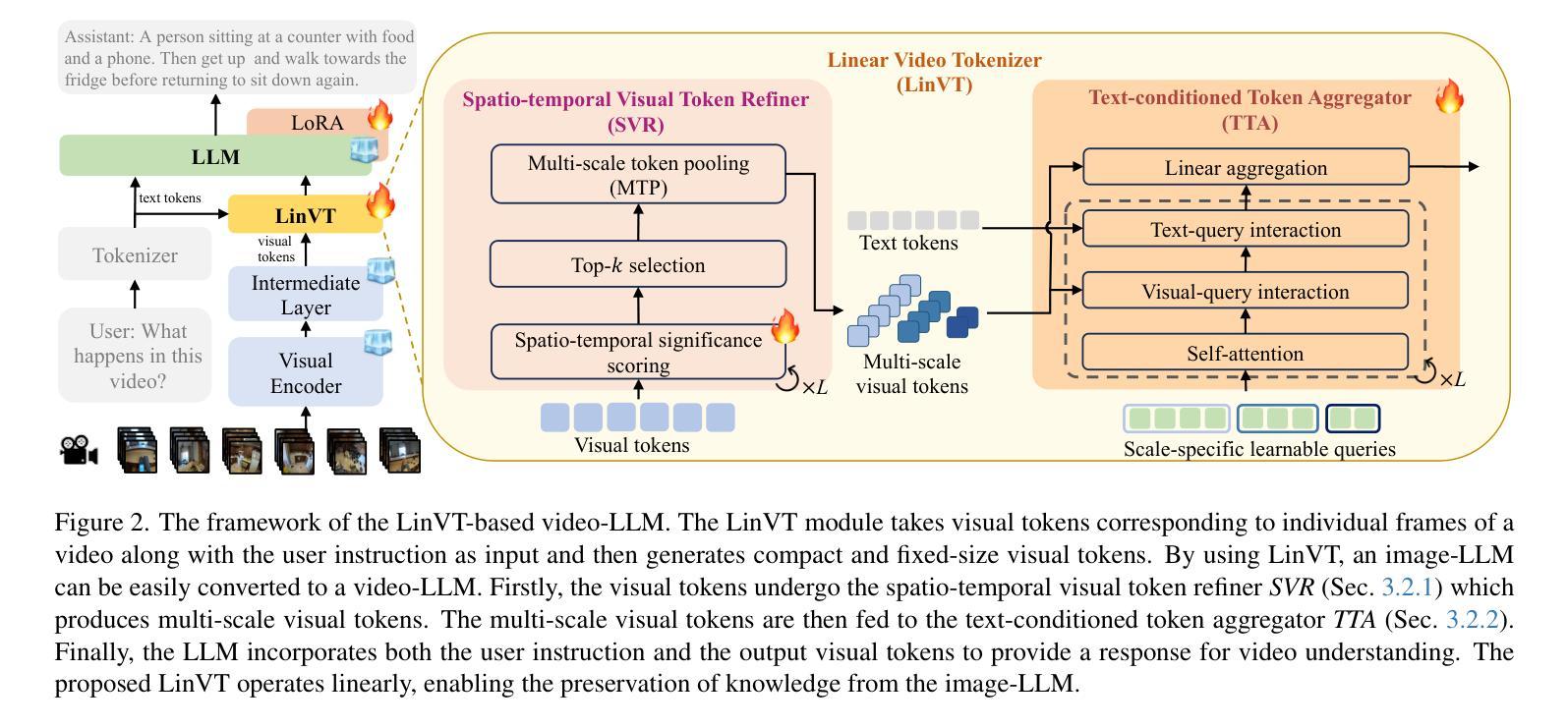
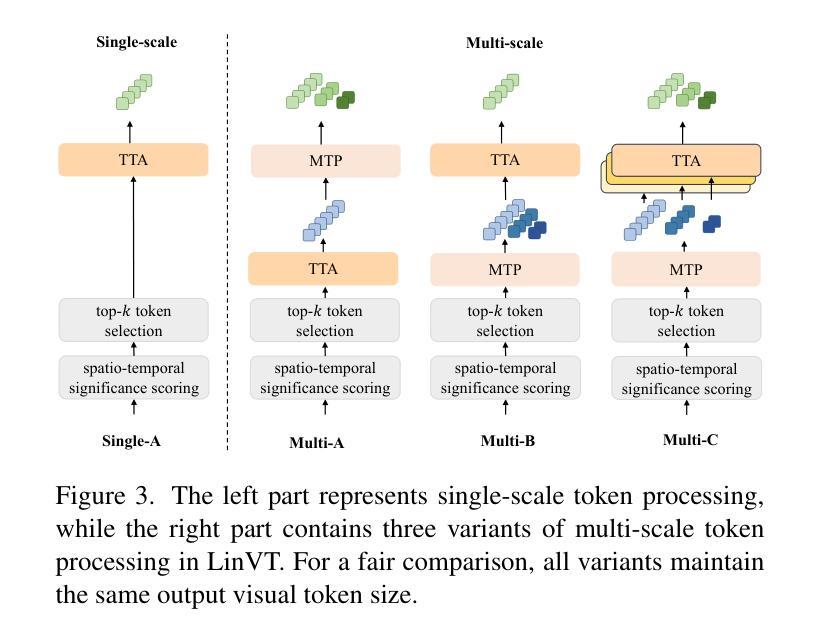
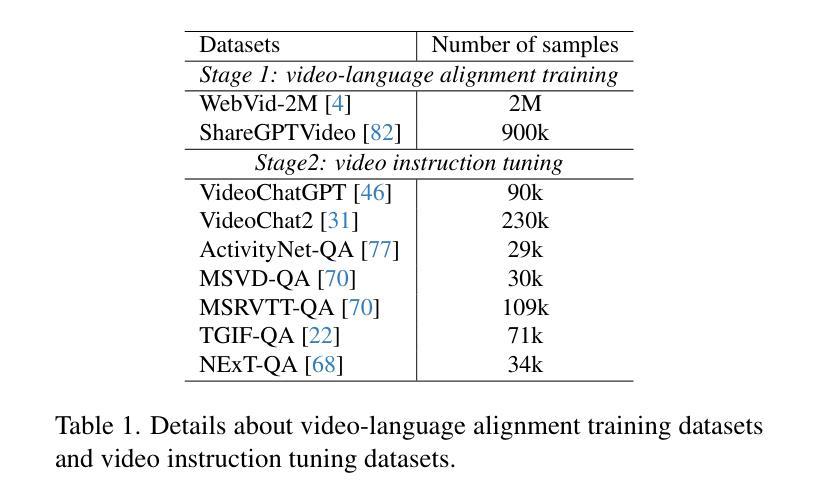
SAVEn-Vid: Synergistic Audio-Visual Integration for Enhanced Understanding in Long Video Context
Authors:Jungang Li, Sicheng Tao, Yibo Yan, Xiaojie Gu, Haodong Xu, Xu Zheng, Yuanhuiyi Lyu, Linfeng Zhang, Xuming Hu
Endeavors have been made to explore Large Language Models for video analysis (Video-LLMs), particularly in understanding and interpreting long videos. However, existing Video-LLMs still face challenges in effectively integrating the rich and diverse audio-visual information inherent in long videos, which is crucial for comprehensive understanding. This raises the question: how can we leverage embedded audio-visual information to enhance long video understanding? Therefore, (i) we introduce SAVEn-Vid, the first-ever long audio-visual video dataset comprising over 58k audio-visual instructions. (ii) From the model perspective, we propose a time-aware Audio-Visual Large Language Model (AV-LLM), SAVEnVideo, fine-tuned on SAVEn-Vid. (iii) Besides, we present AVBench, a benchmark containing 2,500 QAs designed to evaluate models on enhanced audio-visual comprehension tasks within long video, challenging their ability to handle intricate audio-visual interactions. Experiments on AVBench reveal the limitations of current AV-LLMs. Experiments also demonstrate that SAVEnVideo outperforms the best Video-LLM by 3.61% on the zero-shot long video task (Video-MME) and surpasses the leading audio-visual LLM by 1.29% on the zero-shot audio-visual task (Music-AVQA). Consequently, at the 7B parameter scale, SAVEnVideo can achieve state-of-the-art performance. Our dataset and code will be released at https://ljungang.github.io/SAVEn-Vid/ upon acceptance.
在视频分析(Video-LLMs)方面,特别是在理解和解释长视频方面,已经付出了很大的努力来探索大型语言模型的应用。然而,现有的Video-LLMs仍然面临着有效整合长视频中丰富多样的视听信息的挑战,这对于全面理解来说是至关重要的。这就提出了一个问题:我们如何利用嵌入的视听信息来提高对长视频的理解能力?因此,(i)我们推出了SAVEn-Vid,这是首个包含超过58,000条视听指令的视听长视频数据集。(ii)从模型的角度来看,我们提出了时间感知视听大型语言模型(AV-LLM),名为SAVEnVideo,在SAVEn-Vid上进行了微调。(iii)此外,我们还推出了AVBench,这是一个包含2500个问答的基准测试,旨在评估模型在处理长视频中的增强视听理解任务的能力,挑战其处理复杂视听交互的能力。在AVBench上的实验揭示了当前AV-LLM的局限性。实验还表明,在零样本长视频任务(Video-MME)上,SAVEnVideo比最佳Video-LLM高出3.61%,在零样本视听任务(Music-AVQA)上,SAVEnVideo超过领先的视听LLM 1.29%。因此,在7B参数规模下,SAVEnVideo可以达到最先进的性能。我们的数据集和代码将在https://ljungang.github.io/SAVEn-Vid/上发布,待审核通过后即可使用。
论文及项目相关链接
PDF The publication has some processing errors (language short-cuts in synthetic data are not avoided) that invalidate some of the conclusions
Summary
为视频分析引入大型语言模型(Video-LLMs)时面临挑战,尤其是理解和解释长视频时。研究团队构建了首个长音频视觉视频数据集SAVEn-Vid,并据此提出了时间感知的音频视觉大型语言模型(AV-LLM)SAVEnVideo。此外,还推出了评估模型在长时间音频视觉理解任务上的表现的基准测试AVBench。实验表明,SAVEnVideo在零样本长视频任务上优于最佳Video-LLM模型,并在音频视觉任务上达到领先水平。
Key Takeaways
- 大型语言模型在视频分析中的应用仍面临挑战,尤其是在处理和解释长视频时遇到的困难主要是整合丰富多样的音视频信息的问题。
- SAVEn-Vid是首个长音频视觉视频数据集,包含超过58k的音视频指令。
- SAVEnVideo是一种时间感知的音频视觉大型语言模型(AV-LLM),经过SAVEn-Vid数据集微调。
- AVBench是一个基准测试平台,旨在评估模型在复杂的音视频交互处理方面的性能。
- 实验结果显示,SAVEnVideo在零样本长视频任务和音频视觉任务上的表现优于其他模型。
- SAVEnVideo在参数规模为7B时达到了前沿水平的表现。
点此查看论文截图
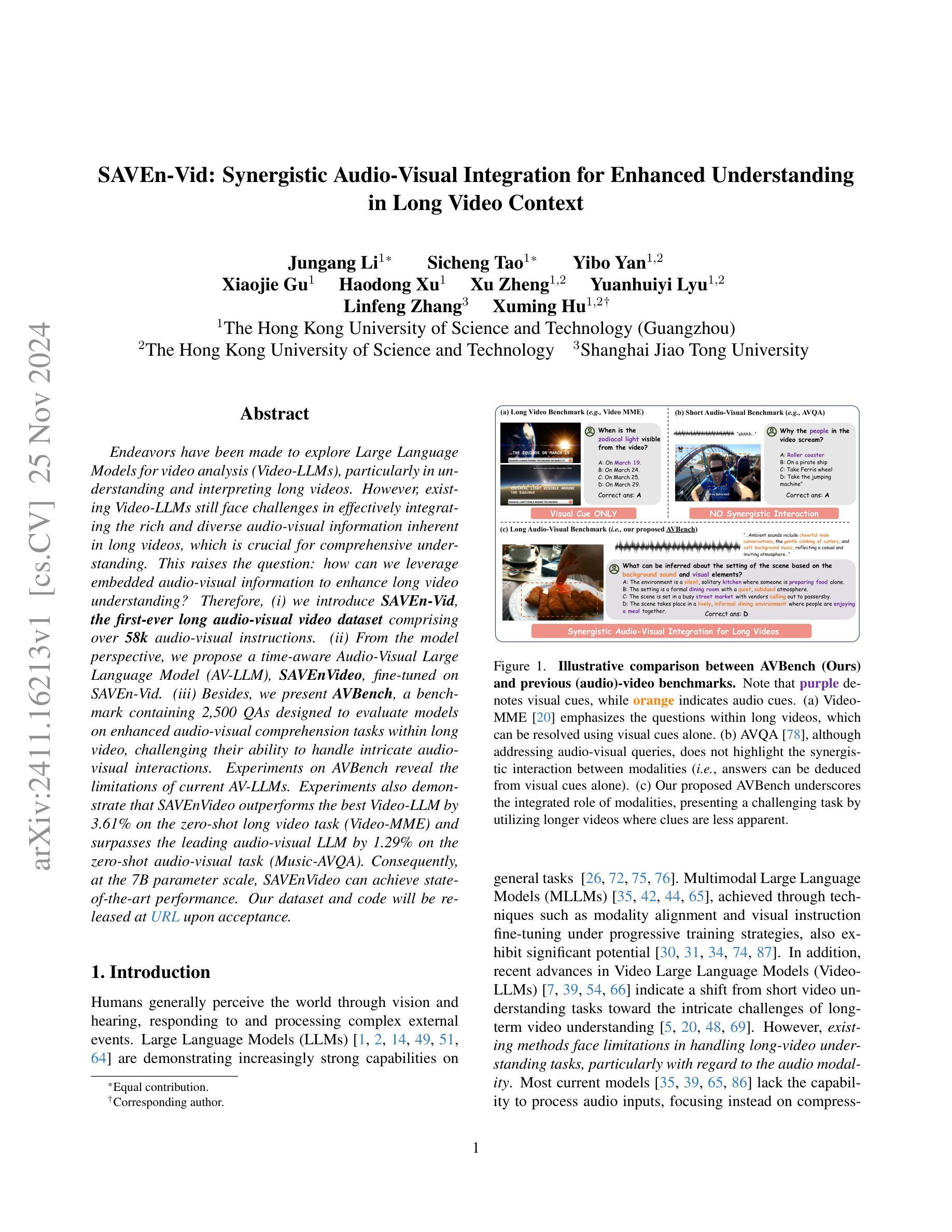
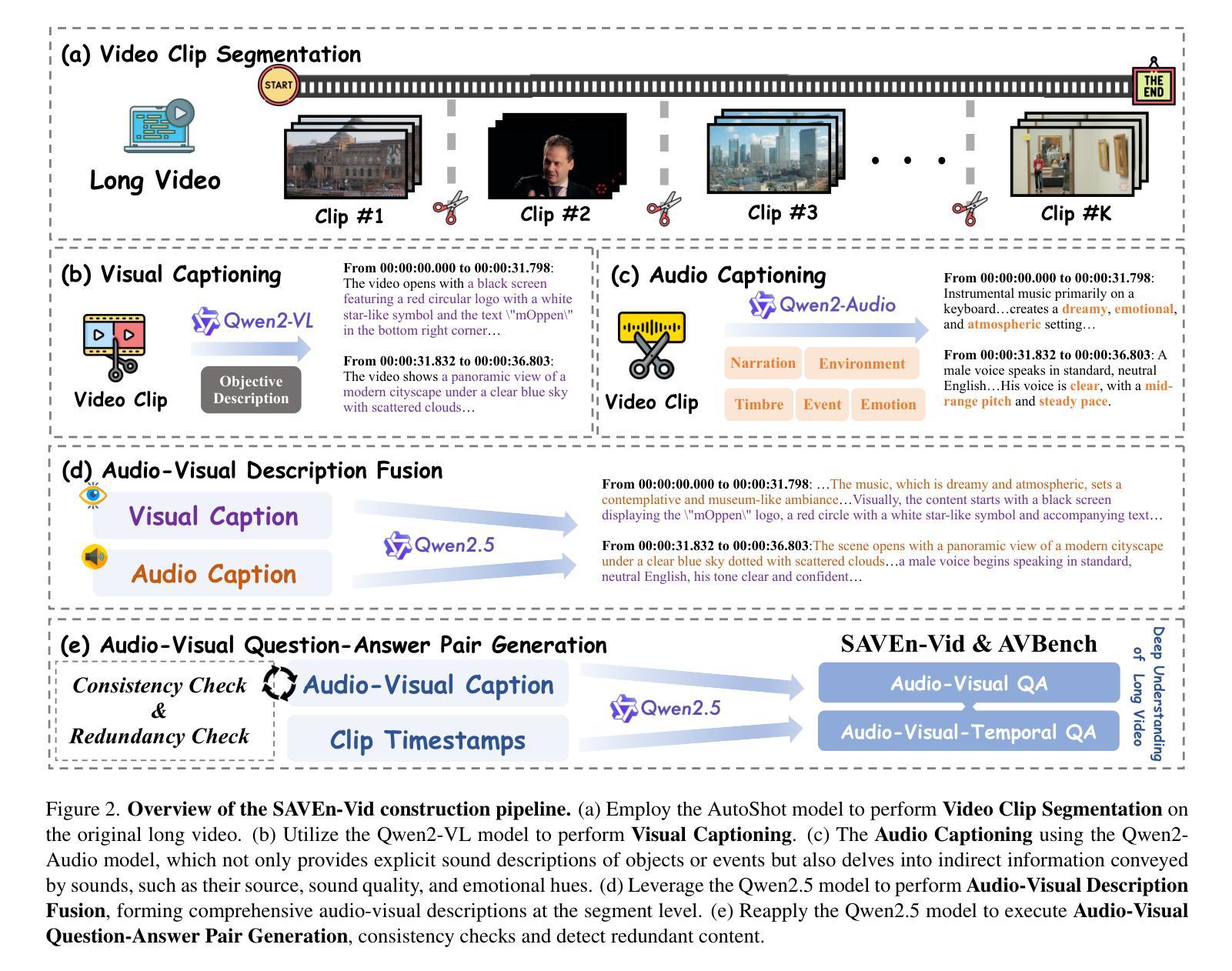
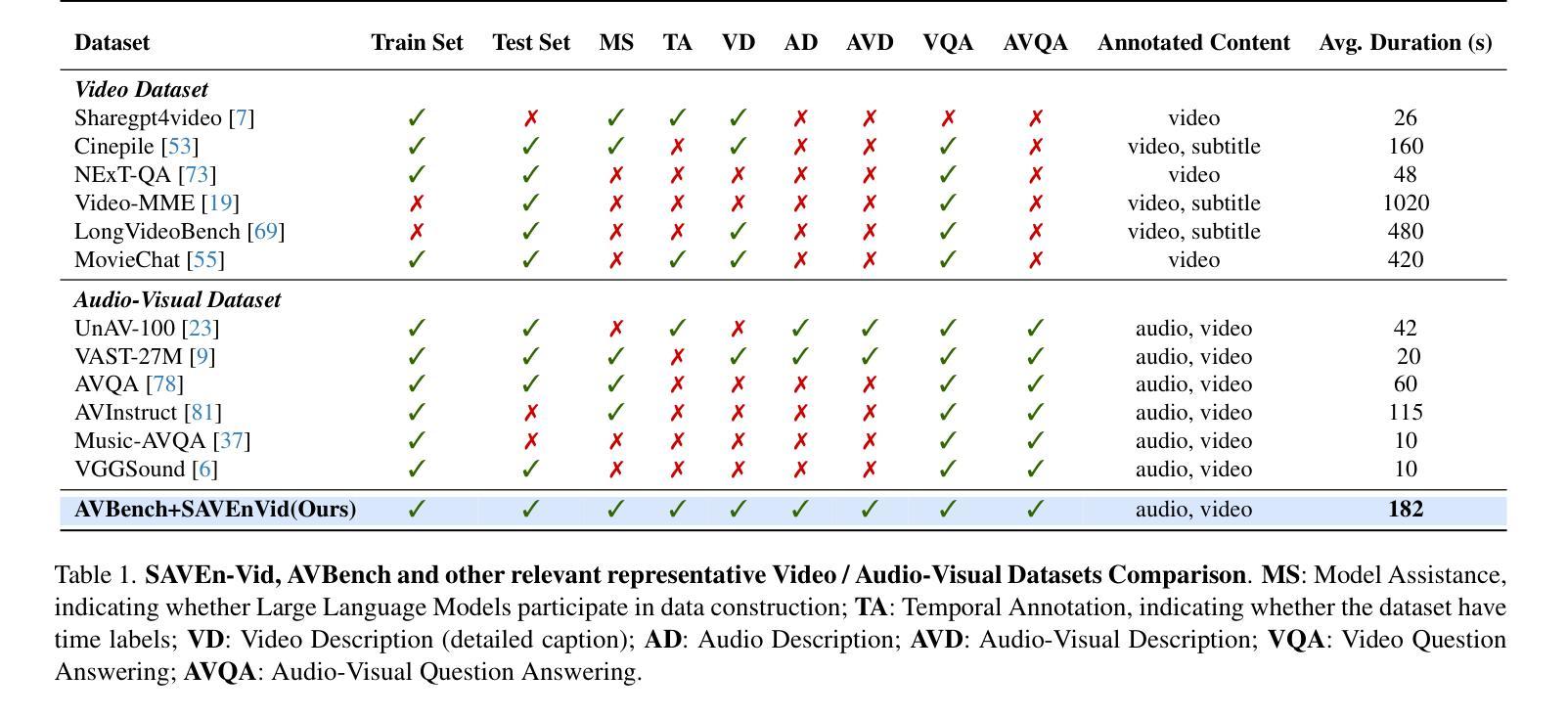
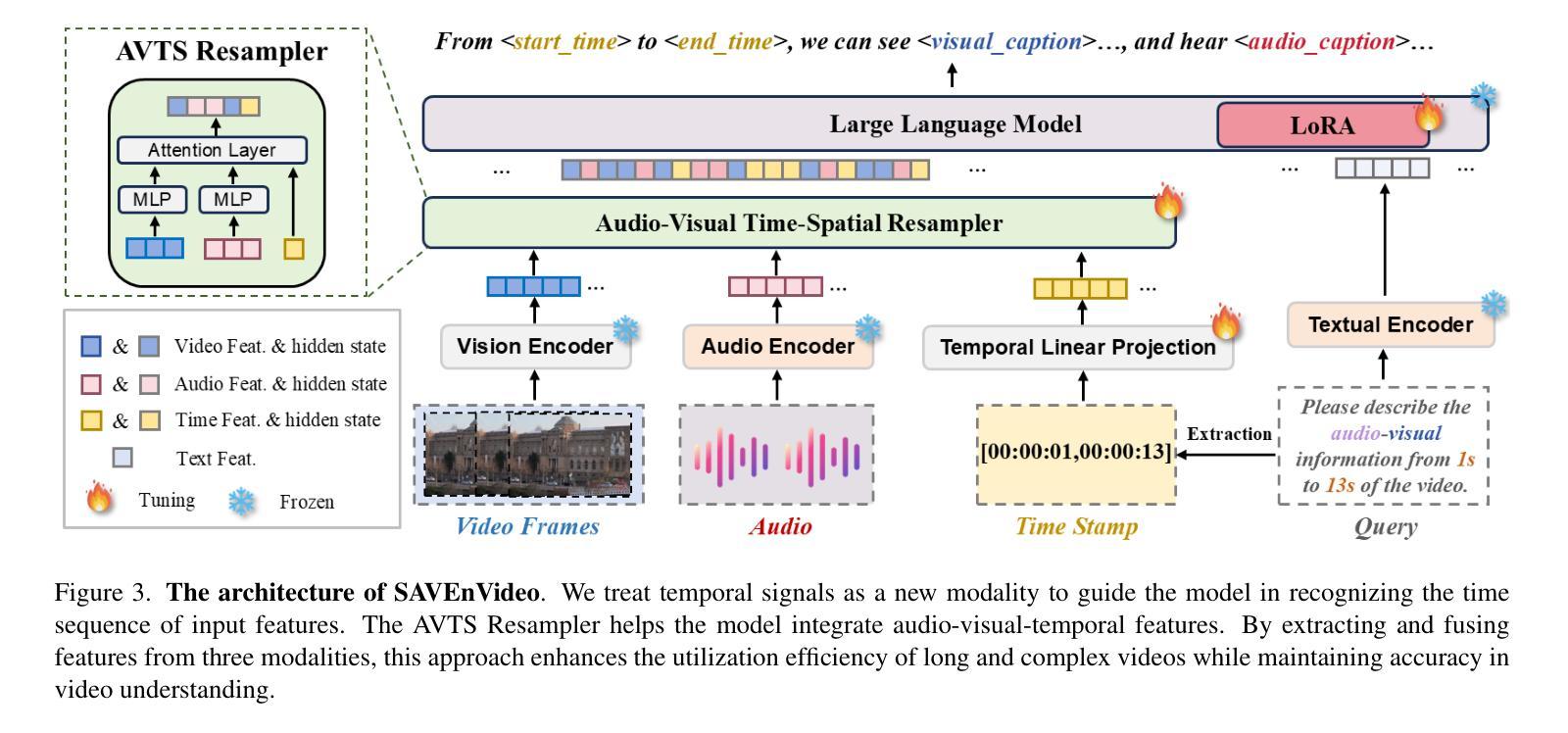
Video-XL: Extra-Long Vision Language Model for Hour-Scale Video Understanding
Authors:Yan Shu, Zheng Liu, Peitian Zhang, Minghao Qin, Junjie Zhou, Zhengyang Liang, Tiejun Huang, Bo Zhao
Long video understanding poses a significant challenge for current Multi-modal Large Language Models (MLLMs). Notably, the MLLMs are constrained by their limited context lengths and the substantial costs while processing long videos. Although several existing methods attempt to reduce visual tokens, their strategies encounter severe bottleneck, restricting MLLMs’ ability to perceive fine-grained visual details. In this work, we propose Video-XL, a novel approach that leverages MLLMs’ inherent key-value (KV) sparsification capacity to condense the visual input. Specifically, we introduce a new special token, the Visual Summarization Token (VST), for each interval of the video, which summarizes the visual information within the interval as its associated KV. The VST module is trained by instruction fine-tuning, where two optimizing strategies are offered. 1.Curriculum learning, where VST learns to make small (easy) and large compression (hard) progressively. 2. Composite data curation, which integrates single-image, multi-image, and synthetic data to overcome the scarcity of long-video instruction data. The compression quality is further improved by dynamic compression, which customizes compression granularity based on the information density of different video intervals. Video-XL’s effectiveness is verified from three aspects. First, it achieves a superior long-video understanding capability, outperforming state-of-the-art models of comparable sizes across multiple popular benchmarks. Second, it effectively preserves video information, with minimal compression loss even at 16x compression ratio. Third, it realizes outstanding cost-effectiveness, enabling high-quality processing of thousands of frames on a single A100 GPU.
长视频理解对当前的多模态大型语言模型(MLLMs)构成重大挑战。特别是,MLLMs在处理长视频时受到其有限上下文长度和巨大成本的限制。尽管有几种现有方法试图减少视觉标记,但它们的策略遇到了严重瓶颈,限制了MLLMs感知精细粒度视觉细节的能力。在这项工作中,我们提出了Video-XL,这是一种利用MLLMs固有的键值(KV)稀疏化能力来精简视觉输入的新方法。具体来说,我们为每个视频间隔引入了一个新的特殊标记,即视觉摘要标记(VST),该标记汇总间隔内的视觉信息作为其关联的KV。VST模块通过指令微调进行训练,其中提供了两种优化策略。1. 课程内容学习,VST学习逐步进行小(容易)和大压缩(困难)。2. 综合数据收集,整合单图像、多图像和合成数据,以克服长视频指令数据的稀缺性。压缩质量通过动态压缩进一步改进,动态压缩根据不同视频间隔的信息密度定制压缩粒度。Video-XL的有效性从三个方面得到了验证。首先,它实现了卓越的长视频理解能力,在多个流行基准测试中超越了同类先进模型。其次,它有效地保存了视频信息,即使在16倍压缩比下也几乎没有压缩损失。第三,它实现了出色的成本效益,能够在单个A100 GPU上实现高质量处理数千帧。
论文及项目相关链接
Summary
视频理解中,长视频对现有的多模态大型语言模型(MLLMs)构成挑战。针对MLLMs在处理长视频时的局限性,如上下文长度有限和成本高昂,本文提出了一种新的方法Video-XL。该方法利用MLLMs的固有键值(KV)稀疏化能力来精简视觉输入。具体来说,我们为每个视频间隔引入了一个新的特殊令牌——视觉摘要令牌(VST),该令牌将间隔内的视觉信息概括为其关联的KV。通过指令微调训练VST模块,提供了两种优化策略:1. 渐进式学习,使VST逐步学习进行大小不同的压缩;2. 整合单帧、多帧和合成数据以克服长视频指令数据的稀缺性的复合数据整理。Video-XL的有效性从三个方面得到了验证:一是其卓越的长视频理解能力,在多个流行基准测试中优于同类模型;二是信息保存效果好,即使在16倍压缩比下也几乎没有压缩损失;三是成本效益高,可在单个A100 GPU上实现高质量处理数千帧。
Key Takeaways
- 长视频理解对于现有Multi-modal Large Language Models(MLLMs)构成挑战,需要处理上下文长度有限和成本高昂的问题。
- Video-XL利用MLLMs的键值(KV)稀疏化能力精简视觉输入,以提高处理效率。
- Video-XL引入视觉摘要令牌(VST),为每个视频间隔提供视觉信息的摘要。
- VST模块通过指令微调进行训练,采用渐进式学习和复合数据整理两种优化策略。
- Video-XL在多个基准测试中表现出卓越的长视频理解能力。
- Video-XL能有效保存视频信息,即使在较高的压缩比下也几乎没有信息损失。
点此查看论文截图
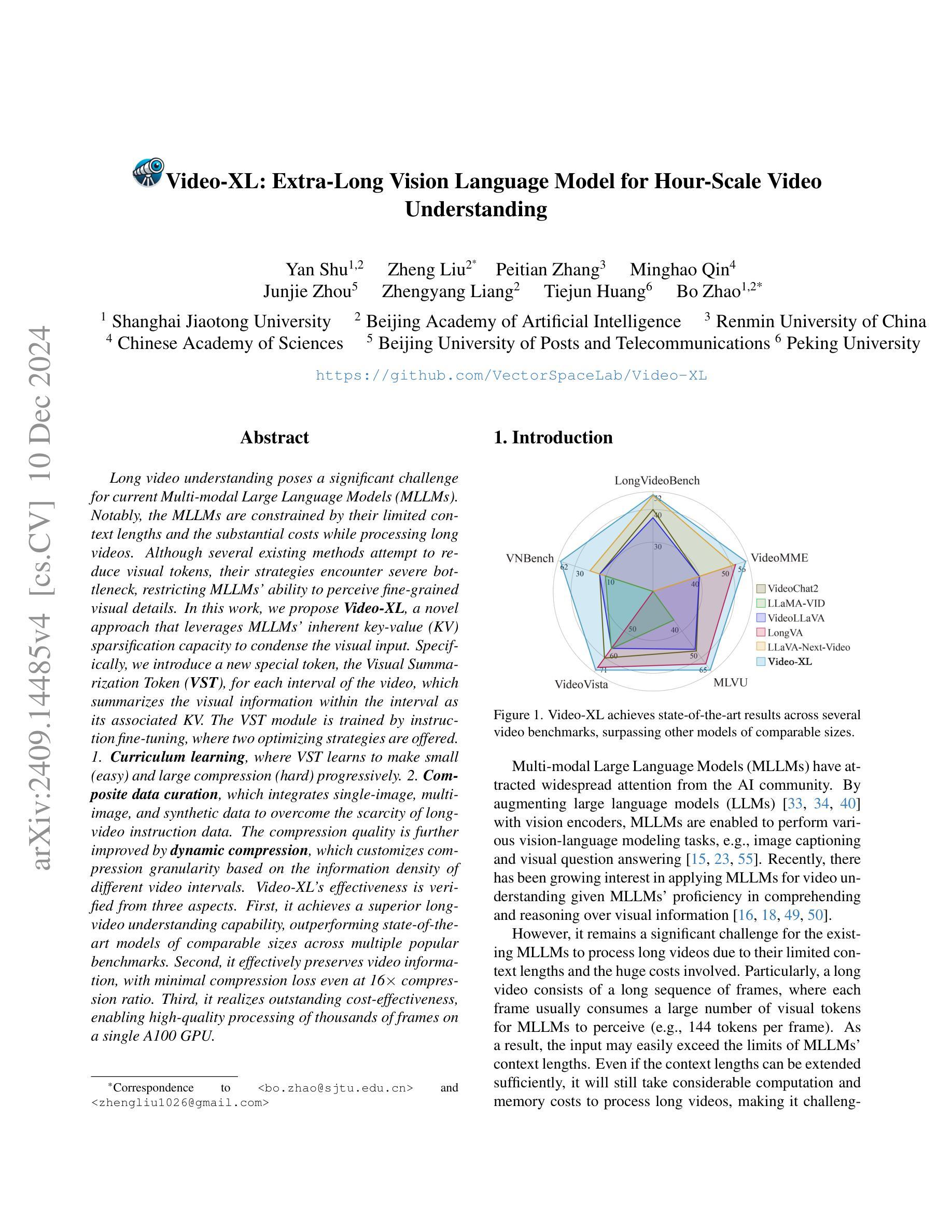
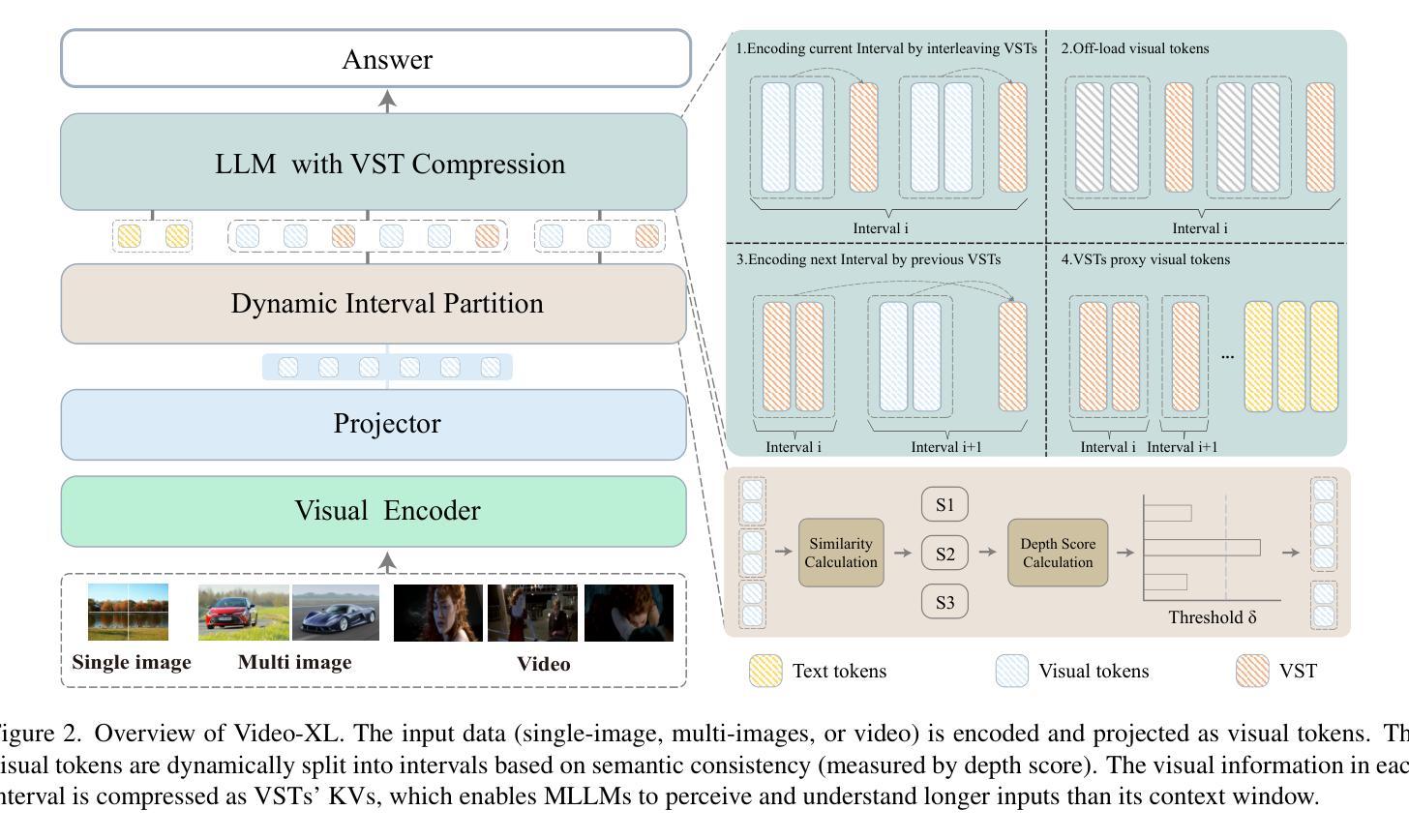
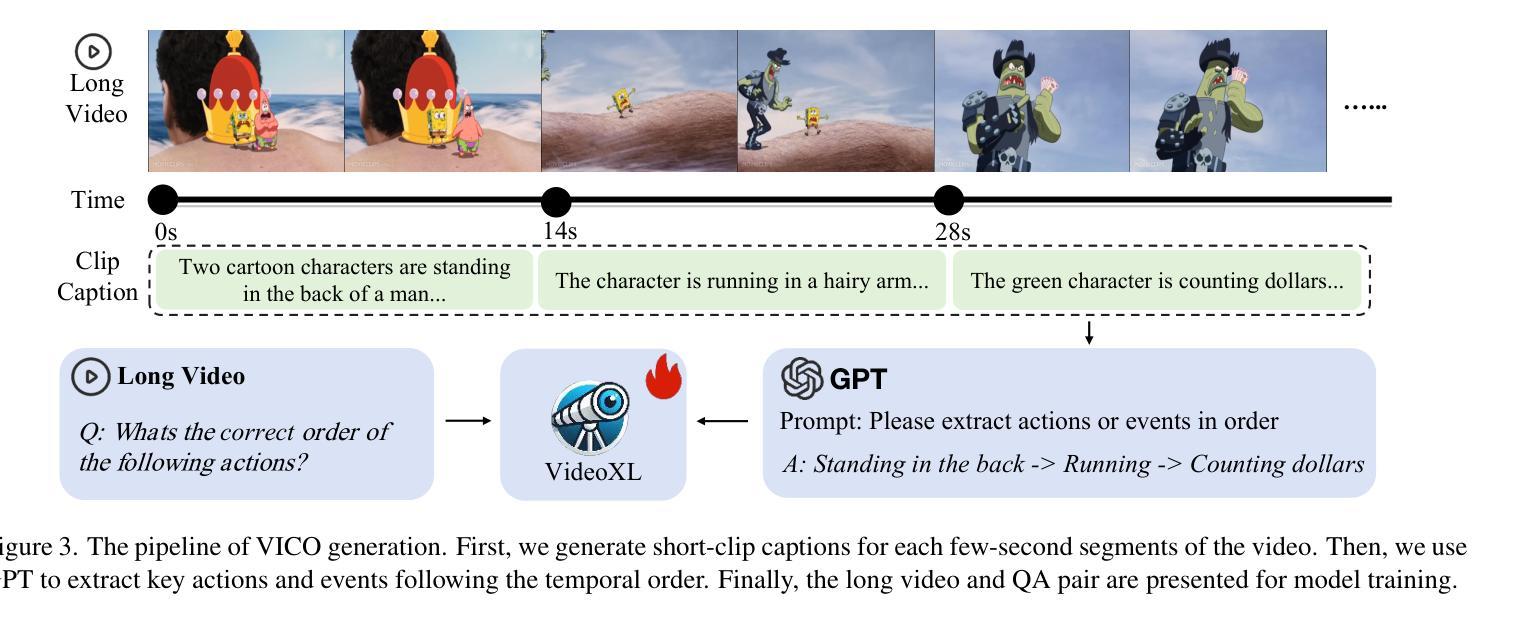
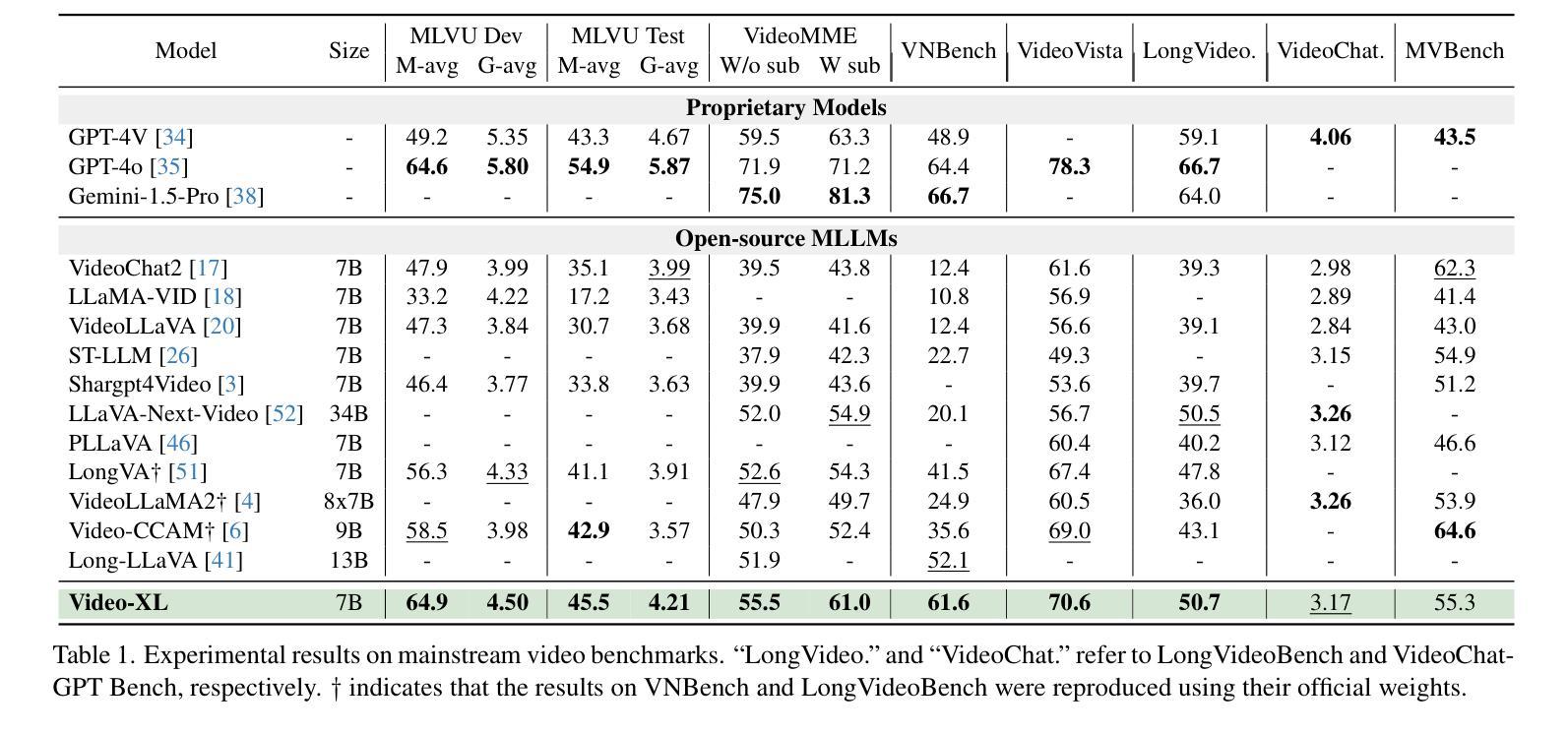
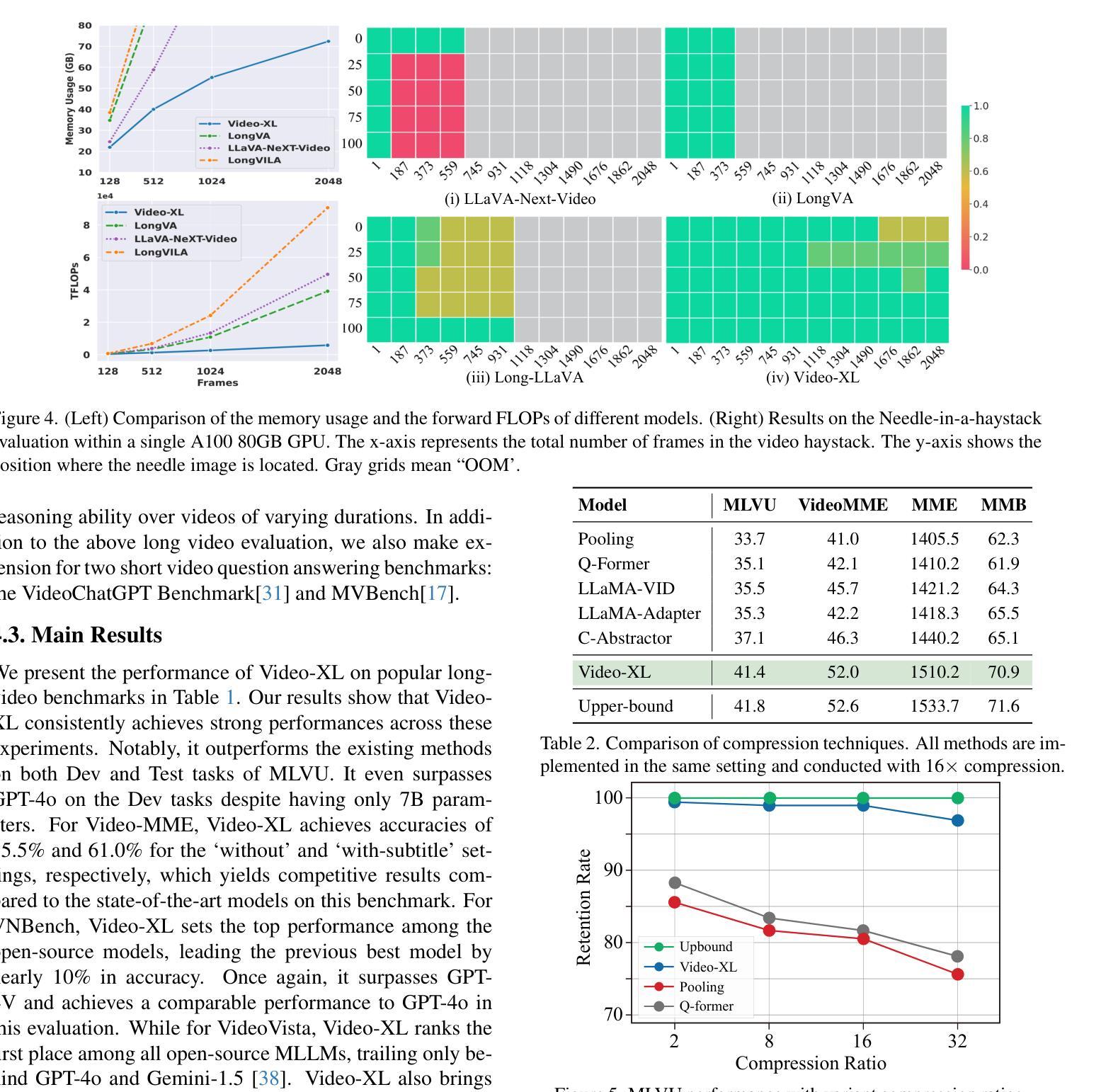