⚠️ 以下所有内容总结都来自于 大语言模型的能力,如有错误,仅供参考,谨慎使用
🔴 请注意:千万不要用于严肃的学术场景,只能用于论文阅读前的初筛!
💗 如果您觉得我们的项目对您有帮助 ChatPaperFree ,还请您给我们一些鼓励!⭐️ HuggingFace免费体验
2024-12-12 更新
PortraitTalk: Towards Customizable One-Shot Audio-to-Talking Face Generation
Authors:Fatemeh Nazarieh, Zhenhua Feng, Diptesh Kanojia, Muhammad Awais, Josef Kittler
Audio-driven talking face generation is a challenging task in digital communication. Despite significant progress in the area, most existing methods concentrate on audio-lip synchronization, often overlooking aspects such as visual quality, customization, and generalization that are crucial to producing realistic talking faces. To address these limitations, we introduce a novel, customizable one-shot audio-driven talking face generation framework, named PortraitTalk. Our proposed method utilizes a latent diffusion framework consisting of two main components: IdentityNet and AnimateNet. IdentityNet is designed to preserve identity features consistently across the generated video frames, while AnimateNet aims to enhance temporal coherence and motion consistency. This framework also integrates an audio input with the reference images, thereby reducing the reliance on reference-style videos prevalent in existing approaches. A key innovation of PortraitTalk is the incorporation of text prompts through decoupled cross-attention mechanisms, which significantly expands creative control over the generated videos. Through extensive experiments, including a newly developed evaluation metric, our model demonstrates superior performance over the state-of-the-art methods, setting a new standard for the generation of customizable realistic talking faces suitable for real-world applications.
音频驱动的对话面部生成是数字通信中的一个具有挑战性的任务。尽管该领域已经取得了重大进展,但大多数现有方法主要集中在音频与嘴唇的同步上,往往忽视了视觉质量、定制和通用性等方面,而这些方面对于生成逼真的对话面部至关重要。为了解决这些局限性,我们引入了一种可定制的一次性音频驱动对话面部生成框架,名为PortraitTalk。我们提出的方法利用了一个潜在扩散框架,主要包括两个组件:IdentityNet和AnimateNet。IdentityNet旨在在生成的视频帧中保持身份特征的连续性,而AnimateNet旨在提高时间连贯性和运动一致性。该框架还将音频输入与参考图像相结合,从而减少了现有方法中对参考风格视频的依赖。PortraitTalk的一个关键创新之处在于通过解耦交叉注意力机制融入了文本提示,这极大地增强了生成视频的创意控制。通过大量实验,包括新开发的评估指标,我们的模型在最新技术方法上表现出了卓越的性能,为定制现实对话面部的生成设定了新的标准,适用于现实世界应用。
论文及项目相关链接
Summary
本文介绍了一种名为PortraitTalk的新型音频驱动说话面部生成框架。该框架利用潜在扩散模型,包括IdentityNet和AnimateNet两个主要组件,旨在解决现有方法忽略的视觉质量、定制性和泛化能力等问题。IdentityNet可保持身份特征在生成视频帧中的一致性,而AnimateNet则提高时间连贯性和运动一致性。此外,该框架结合音频输入和参考图像,降低对参考样式视频的依赖。PortraitTalk的一大创新在于通过解耦交叉注意力机制融入文本提示,大大增强了生成视频的创作控制力。实验证明,与现有方法相比,该模型表现出卓越性能,为定制现实说话面部生成树立了新标准,适用于实际应用。
Key Takeaways
- PortraitTalk是一个新型的音频驱动说话面部生成框架,旨在解决现有方法的局限性。
- 该框架利用潜在扩散模型,包括IdentityNet和AnimateNet两个组件。
- IdentityNet保持身份特征的一致性,而AnimateNet增强时间连贯性和运动一致性。
- 框架结合音频输入和参考图像,降低对参考样式视频的依赖。
- PortraitTalk通过解耦交叉注意力机制融入文本提示,增强生成视频的创作控制力。
- 与现有方法相比,该模型在实验中表现出卓越性能。
点此查看论文截图
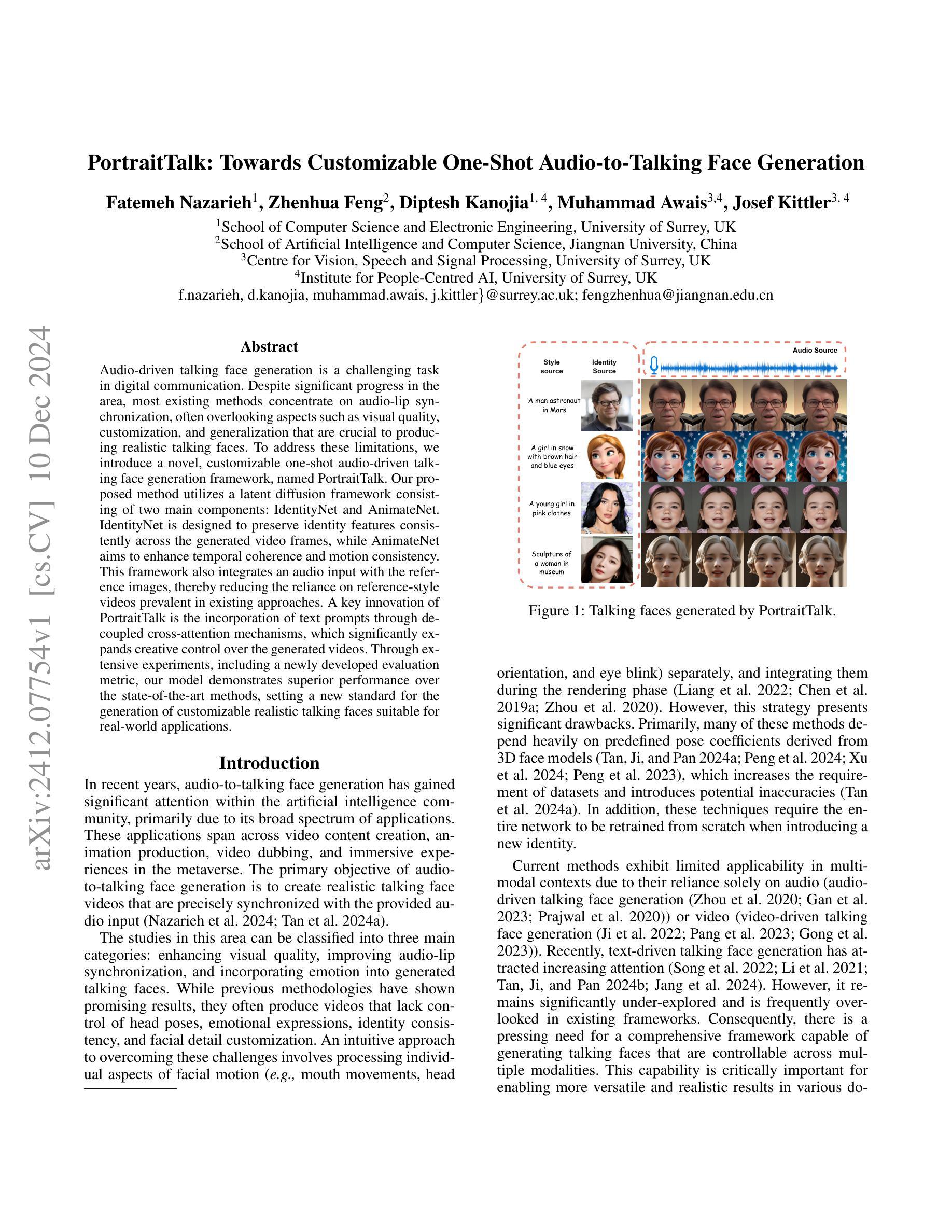
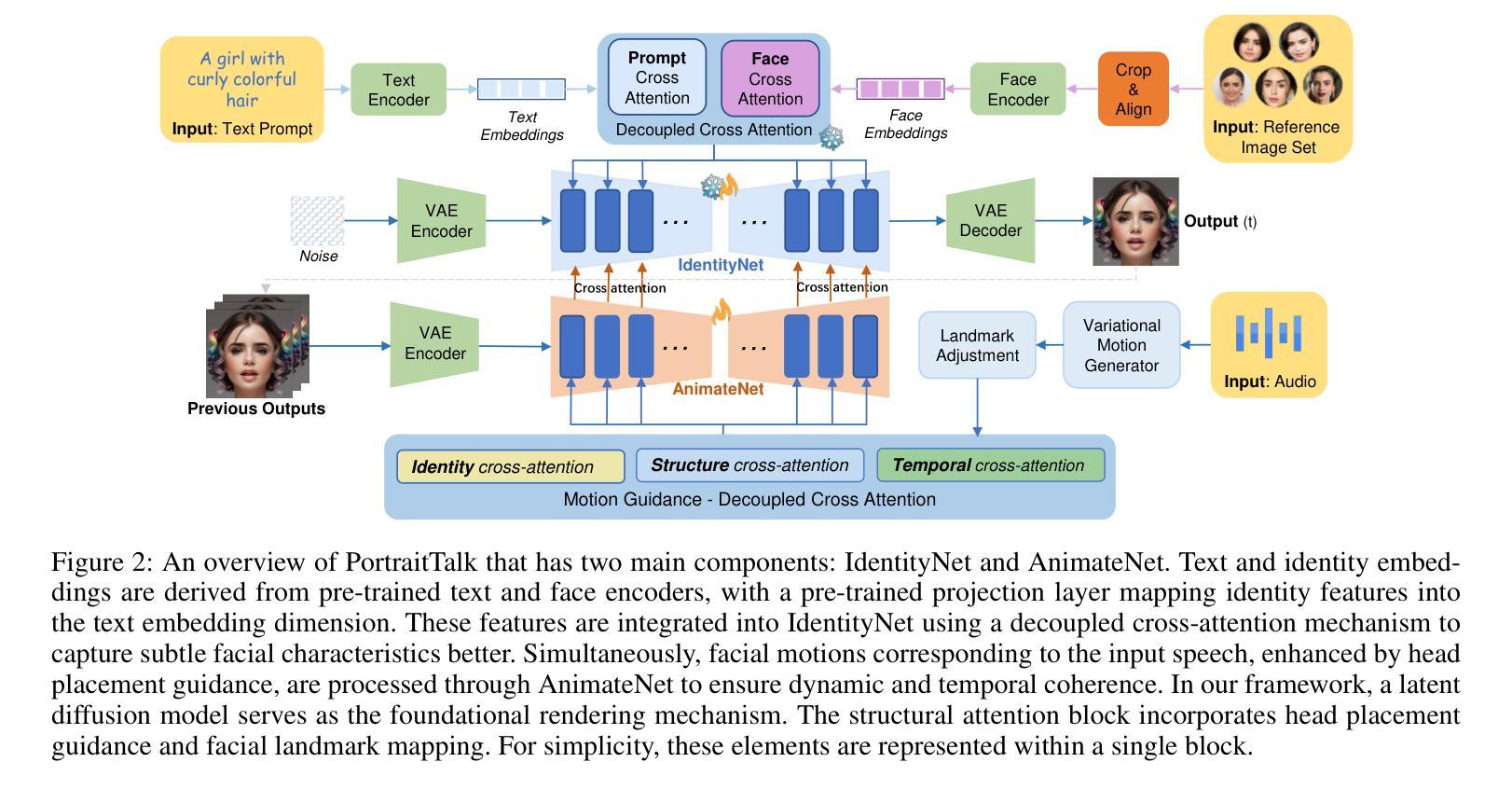
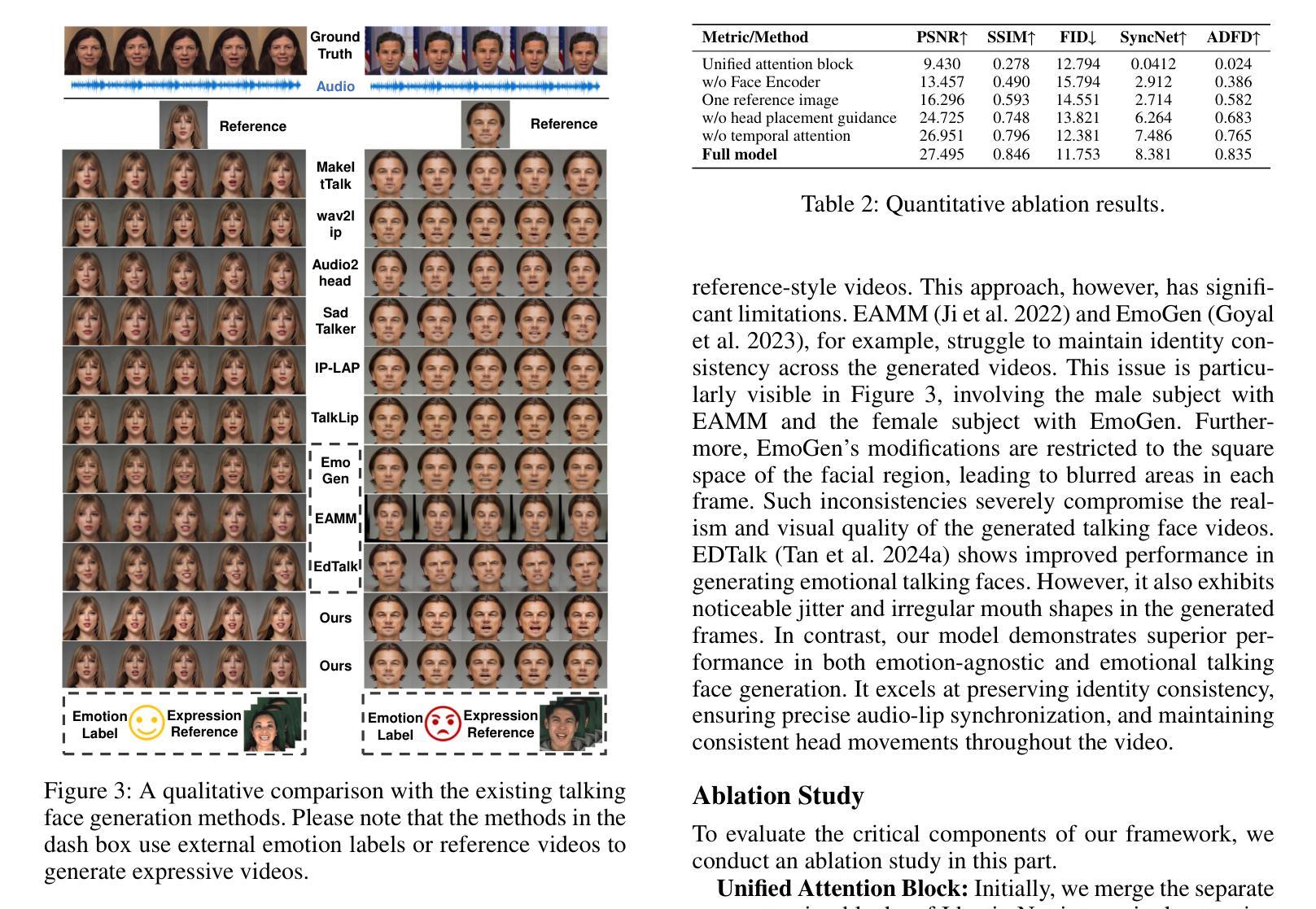
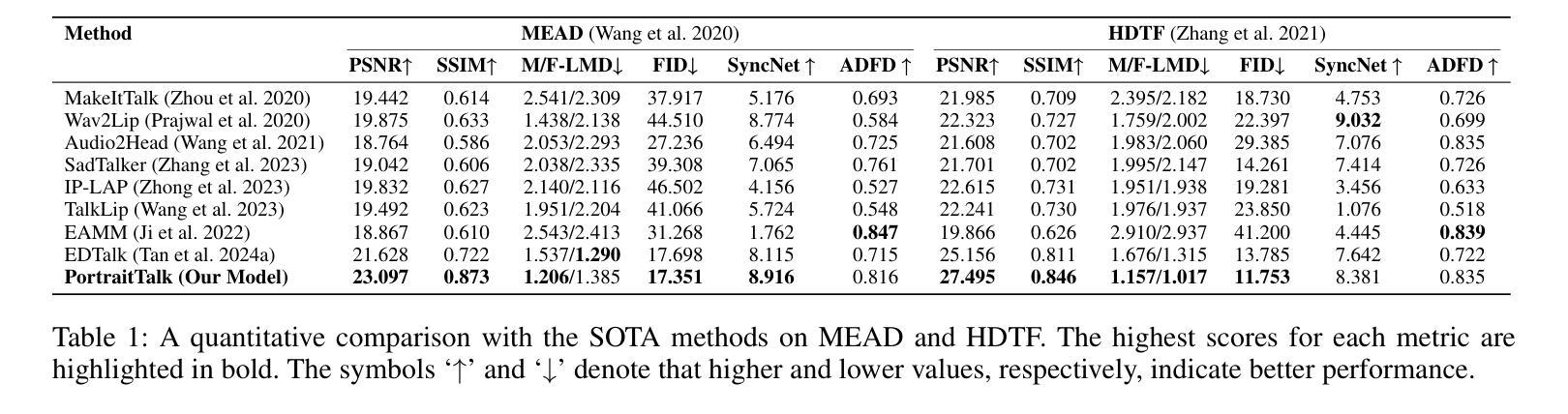
INFP: Audio-Driven Interactive Head Generation in Dyadic Conversations
Authors:Yongming Zhu, Longhao Zhang, Zhengkun Rong, Tianshu Hu, Shuang Liang, Zhipeng Ge
Imagine having a conversation with a socially intelligent agent. It can attentively listen to your words and offer visual and linguistic feedback promptly. This seamless interaction allows for multiple rounds of conversation to flow smoothly and naturally. In pursuit of actualizing it, we propose INFP, a novel audio-driven head generation framework for dyadic interaction. Unlike previous head generation works that only focus on single-sided communication, or require manual role assignment and explicit role switching, our model drives the agent portrait dynamically alternates between speaking and listening state, guided by the input dyadic audio. Specifically, INFP comprises a Motion-Based Head Imitation stage and an Audio-Guided Motion Generation stage. The first stage learns to project facial communicative behaviors from real-life conversation videos into a low-dimensional motion latent space, and use the motion latent codes to animate a static image. The second stage learns the mapping from the input dyadic audio to motion latent codes through denoising, leading to the audio-driven head generation in interactive scenarios. To facilitate this line of research, we introduce DyConv, a large scale dataset of rich dyadic conversations collected from the Internet. Extensive experiments and visualizations demonstrate superior performance and effectiveness of our method. Project Page: https://grisoon.github.io/INFP/.
想象一下与一个社会智能代理进行对话的场景。它能够专注地倾听你的话语,并提供及时的视觉和语言反馈。这种无缝互动允许多轮对话流畅、自然地展开。为了实现实景交互,我们提出了INFP,这是一个新型音频驱动的头部生成框架,用于双人交互。与以前只关注单方面的头部生成作品,或需要手动分配角色和明确的角色切换不同,我们的模型通过输入的双人音频来指导代理肖像动态地在说话和倾听状态之间切换。具体来说,INFP包括基于运动的头部模仿阶段和音频引导的运动生成阶段。第一阶段学习将来自真实对话视频的面部交流行为投影到一个低维运动潜在空间,并使用运动潜在代码来驱动静态图像。第二阶段通过去噪学习将输入的双人音频映射到运动潜在代码,从而在交互场景中实现音频驱动的头部生成。为了促进这一领域的研究,我们引入了DyConv,这是一个从互联网收集的大规模丰富的双人对话数据集。大量的实验和可视化展示了我们方法优越的性能和效果。项目页面:https://grisoon.github.io/INFP/。
论文及项目相关链接
Summary
本文描述了一种具有社交智能的交互代理,该代理能够与用户进行无缝交流,并通过音频驱动头部生成框架INFP实现动态角色切换。INFP包括基于运动的头部模仿阶段和音频引导的运动生成阶段,能够从真实对话视频中学习面部沟通行为,并通过去噪技术实现音频驱动的头部生成。同时,引入DyConv数据集推动这一领域的研究。
Key Takeaways
- 介绍了社交智能交互代理的概念,其能进行无缝交流并实现动态角色切换。
- 提出了INFP框架,包括基于运动的头部模仿和音频引导的运动生成两个阶段。
- INFP能够从真实对话视频中学习面部沟通行为,并使用运动潜在代码来驱动静态图像。
- 通过去噪技术实现音频驱动的头部生成,适应交互场景。
- 引入了DyConv数据集,用于推动此领域的研究。
- 实验和可视化证明INFP方法的优越性能和有效性。
点此查看论文截图
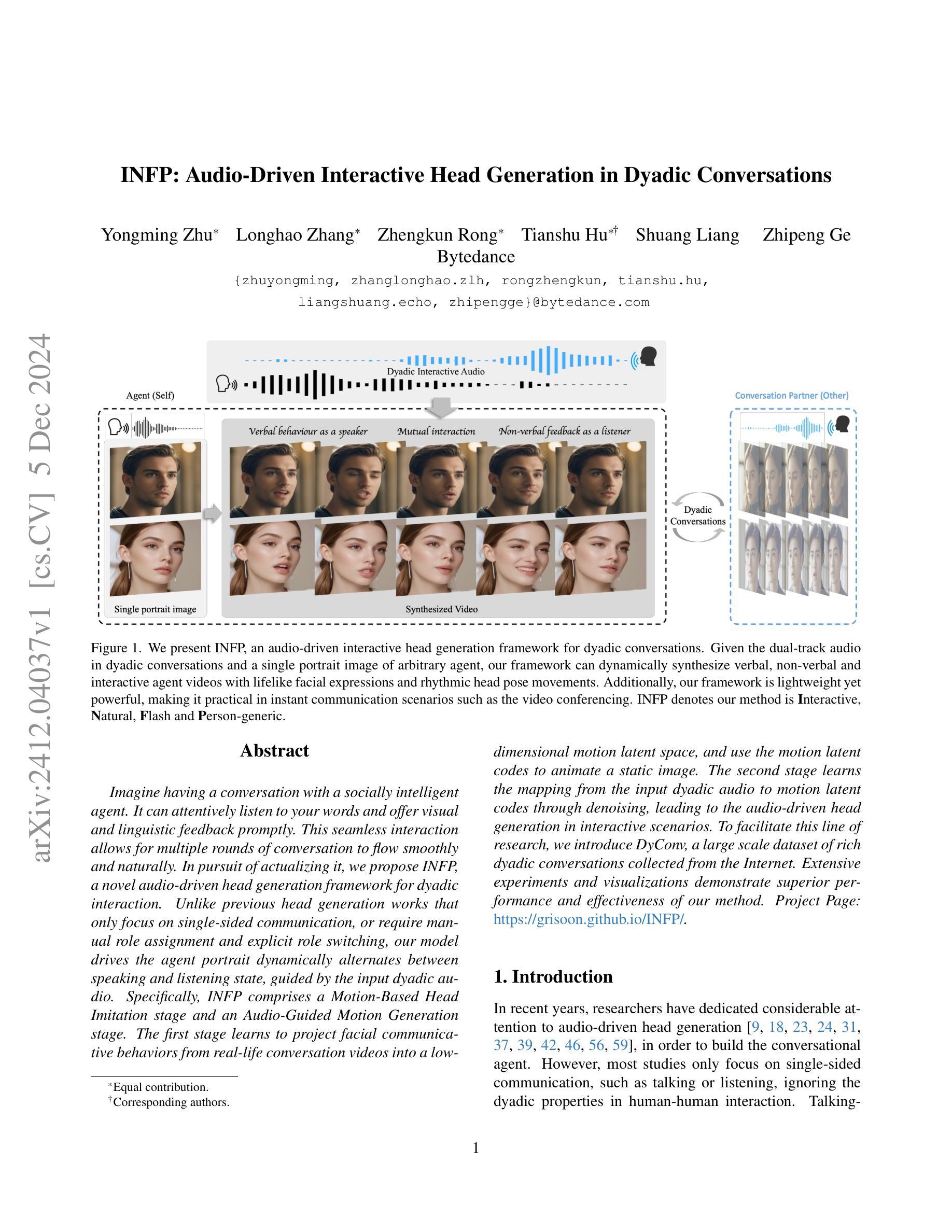
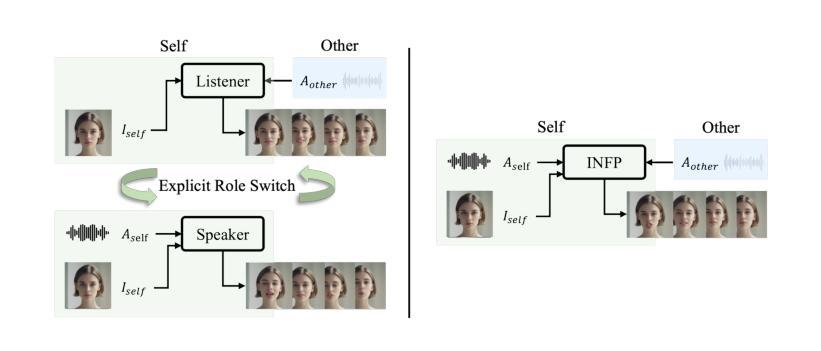
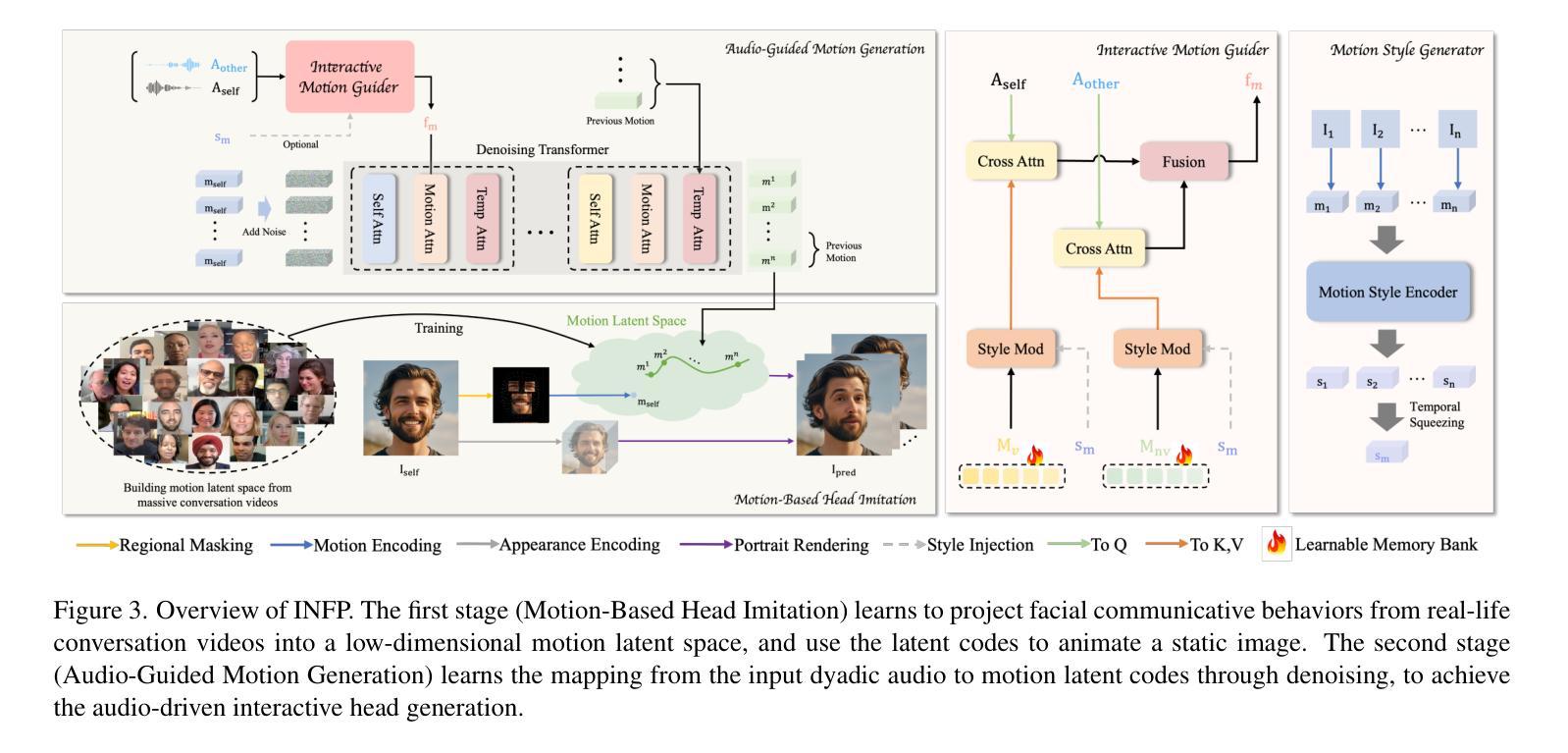
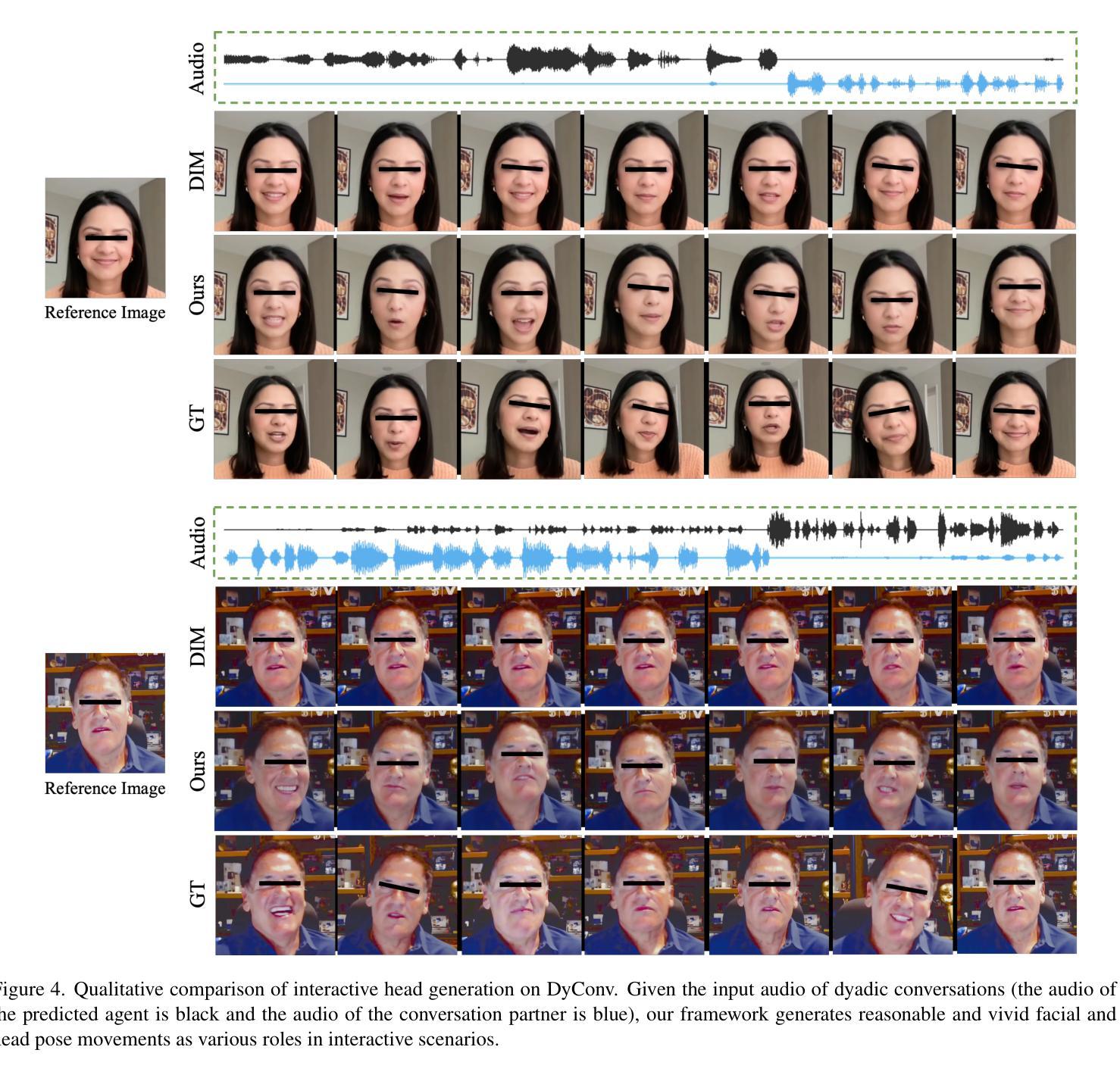
IF-MDM: Implicit Face Motion Diffusion Model for High-Fidelity Realtime Talking Head Generation
Authors:Sejong Yang, Seoung Wug Oh, Yang Zhou, Seon Joo Kim
We introduce a novel approach for high-resolution talking head generation from a single image and audio input. Prior methods using explicit face models, like 3D morphable models (3DMM) and facial landmarks, often fall short in generating high-fidelity videos due to their lack of appearance-aware motion representation. While generative approaches such as video diffusion models achieve high video quality, their slow processing speeds limit practical application. Our proposed model, Implicit Face Motion Diffusion Model (IF-MDM), employs implicit motion to encode human faces into appearance-aware compressed facial latents, enhancing video generation. Although implicit motion lacks the spatial disentanglement of explicit models, which complicates alignment with subtle lip movements, we introduce motion statistics to help capture fine-grained motion information. Additionally, our model provides motion controllability to optimize the trade-off between motion intensity and visual quality during inference. IF-MDM supports real-time generation of 512x512 resolution videos at up to 45 frames per second (fps). Extensive evaluations demonstrate its superior performance over existing diffusion and explicit face models. The code will be released publicly, available alongside supplementary materials. The video results can be found on https://bit.ly/ifmdm_supplementary.
我们提出了一种新型的高分辨率谈唱人头生成方法,仅使用单张图像和音频输入。之前使用明确面部模型的方法,如3D可变形模型(3DMM)和面部特征点,常常因缺乏外观感知运动表示而无法生成高质量的视频。虽然生成式方法(如视频扩散模型)可以达到高质量的视频效果,但其较慢的处理速度限制了实际应用。我们提出的隐式面部运动扩散模型(IF-MDM)采用隐式运动来将人脸编码为具有外观感知的压缩面部潜在空间,从而提高视频生成质量。尽管隐式运动缺乏显式模型的空间分离性,这使得与细微的唇部运动对齐变得复杂,但我们引入了运动统计信息来帮助捕捉精细的运动信息。此外,我们的模型提供运动可控性,在推理过程中优化运动强度与视觉质量之间的权衡。IF-MDM支持实时生成分辨率为512x512的视频,最高可达每秒45帧(fps)。广泛的评估表明其在扩散和显式面部模型中的卓越性能。代码将与补充材料一同公开发布。视频结果可通过链接https://bit.ly/ifmdm_supplementary查看。
论文及项目相关链接
PDF Underreview
Summary
基于单张图像和音频输入,我们提出了一种用于高分辨率说话人头部生成的新型方法。传统方法使用明确的面部模型,如三维可变形模型(3DMM)和面部特征点,但由于缺乏外观感知的运动表示,往往在生成高质量视频时表现不足。我们的提出的模型——隐式面部运动扩散模型(IF-MDM)采用隐式运动将人脸编码为具有外观感知的压缩面部潜在空间,提高了视频生成质量。尽管隐式运动缺乏明确模型的空间分解,这使得与细微的嘴唇运动对齐变得复杂,但我们引入了运动统计来帮助捕捉精细的运动信息。此外,我们的模型提供了运动可控性,在推理过程中优化了运动强度与视觉质量之间的权衡。IF-MDM支持以每秒45帧的速度实时生成分辨率为512x512的视频。全面的评估证明了其在扩散和明确面部模型上的优越性。结果视频可以在https://bit.ly/ifmdm_supplementary找到。
Key Takeaways
- 介绍了一种基于单张图像和音频输入的高分辨率说话头部生成新方法。
- 提出了隐式面部运动扩散模型(IF-MDM),利用隐式运动编码人脸,提高视频生成质量。
- 引入运动统计以捕捉精细的运动信息,解决隐式运动中嘴唇运动对齐的复杂性。
- 模型提供运动可控性,优化运动强度与视觉质量的权衡。
- IF-MDM支持实时生成高分辨率视频,达到每秒45帧的速度。
点此查看论文截图
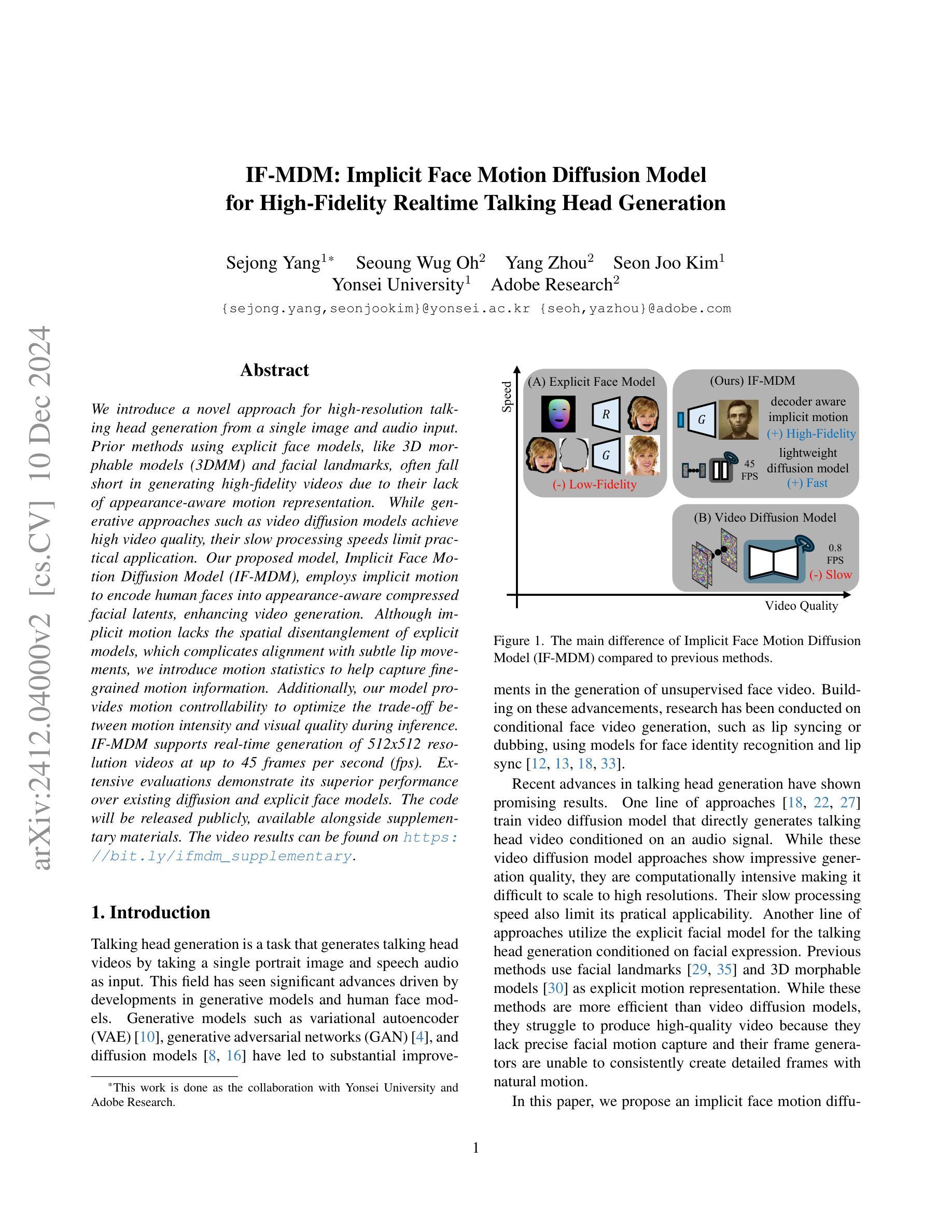
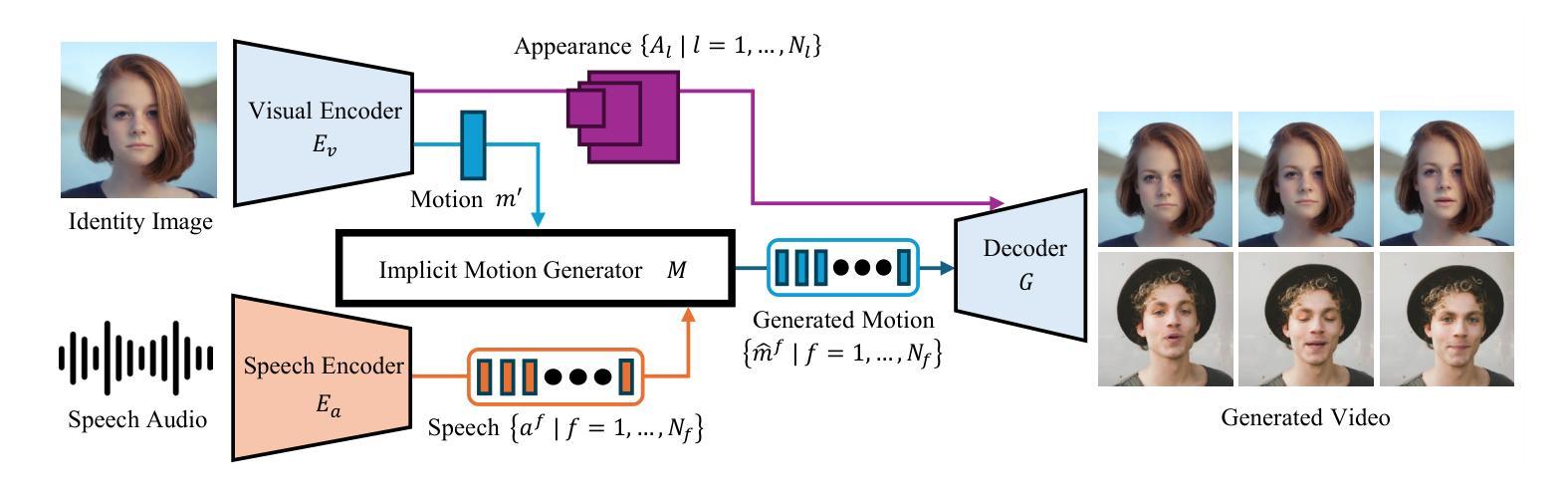
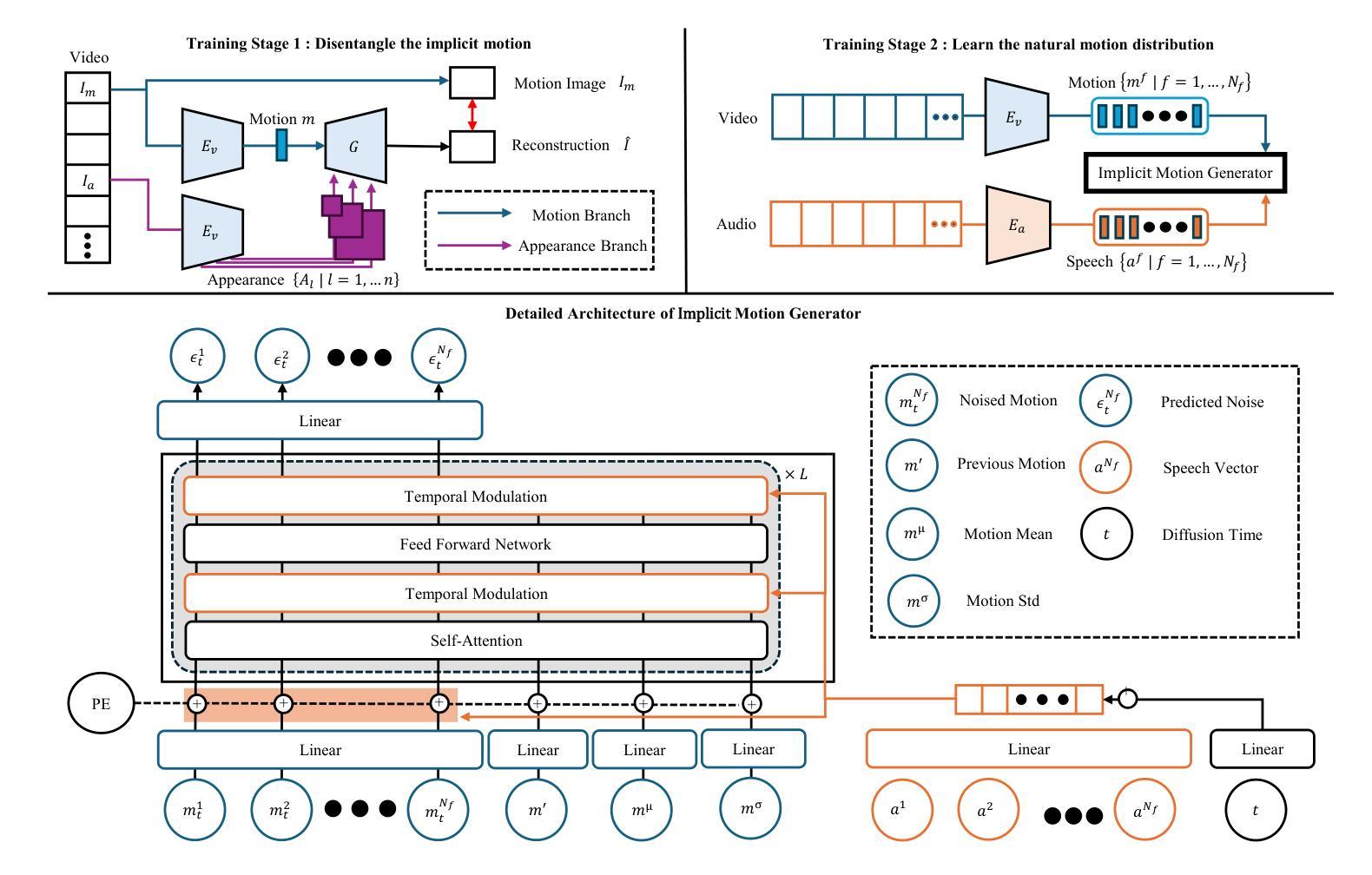
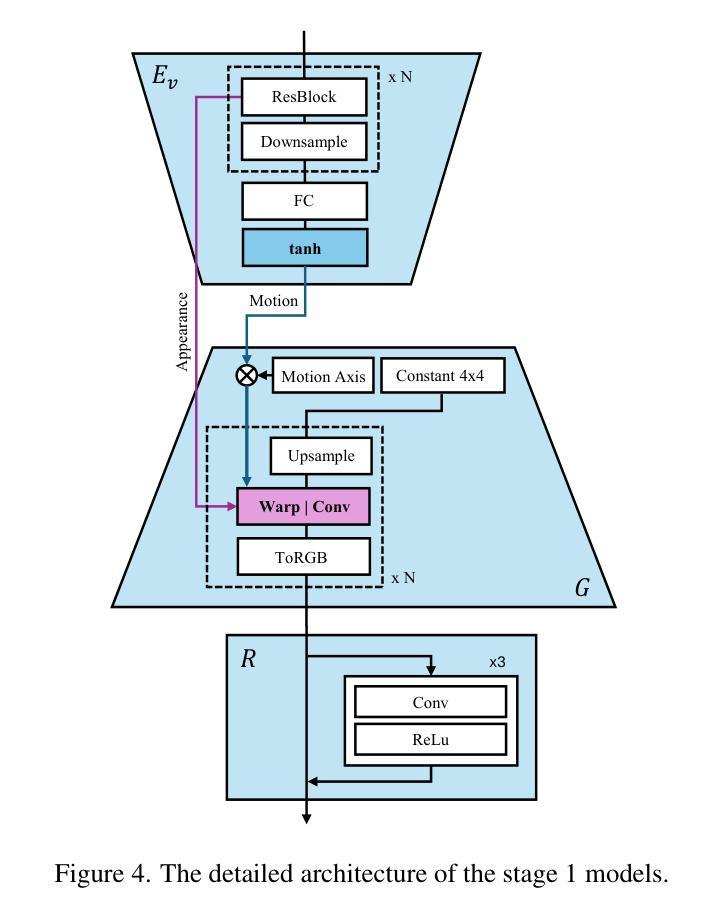
DEEPTalk: Dynamic Emotion Embedding for Probabilistic Speech-Driven 3D Face Animation
Authors:Jisoo Kim, Jungbin Cho, Joonho Park, Soonmin Hwang, Da Eun Kim, Geon Kim, Youngjae Yu
Speech-driven 3D facial animation has garnered lots of attention thanks to its broad range of applications. Despite recent advancements in achieving realistic lip motion, current methods fail to capture the nuanced emotional undertones conveyed through speech and produce monotonous facial motion. These limitations result in blunt and repetitive facial animations, reducing user engagement and hindering their applicability. To address these challenges, we introduce DEEPTalk, a novel approach that generates diverse and emotionally rich 3D facial expressions directly from speech inputs. To achieve this, we first train DEE (Dynamic Emotion Embedding), which employs probabilistic contrastive learning to forge a joint emotion embedding space for both speech and facial motion. This probabilistic framework captures the uncertainty in interpreting emotions from speech and facial motion, enabling the derivation of emotion vectors from its multifaceted space. Moreover, to generate dynamic facial motion, we design TH-VQVAE (Temporally Hierarchical VQ-VAE) as an expressive and robust motion prior overcoming limitations of VAEs and VQ-VAEs. Utilizing these strong priors, we develop DEEPTalk, A talking head generator that non-autoregressively predicts codebook indices to create dynamic facial motion, incorporating a novel emotion consistency loss. Extensive experiments on various datasets demonstrate the effectiveness of our approach in creating diverse, emotionally expressive talking faces that maintain accurate lip-sync. Source code will be made publicly available soon.
语音驱动的3D面部动画因其广泛的应用领域而备受关注。尽管最近在实现逼真的唇部运动方面取得了进展,但当前的方法无法捕捉通过语音传达的微妙情绪基调,并产生单调的面部运动。这些限制导致面部动画生硬且重复,降低了用户参与度并阻碍了其适用性。为了应对这些挑战,我们引入了DEEPTalk,这是一种从语音输入直接生成多样且情感丰富的3D面部表情的新方法。为实现这一点,我们首先训练DEE(动态情绪嵌入),它采用概率对比学习来锻造用于语音和面部运动的联合情绪嵌入空间。这个概率框架捕捉了从语音和面部运动中解释情绪的的不确定性,从而能够从其多角度空间中推导出情绪向量。此外,为了生成动态面部运动,我们设计了TH-VQVAE(时序分层VQ-VAE)作为表现力强且稳健的运动先验,克服了VAE和VQ-VAE的局限性。利用这些强大的先验条件,我们开发了DEEPTalk,这是一款非自回归地预测代码本索引来创建动态面部运动的说话人头生成器,并结合了一种新型的情绪一致性损失。在多个数据集上的大量实验表明,我们的方法在创建多样且情感丰富的说话面部时非常有效,同时保持了准确的唇同步。源代码将很快公开发布。
论文及项目相关链接
PDF First two authors contributed equally. This is a revised version of the original submission, which has been accepted for publication at AAAI 2025
Summary
本文提出了一种新的方法DEEPTalk,用于从语音输入生成丰富情感的3D面部表情。通过训练动态情绪嵌入(DEE)和采用时序层次VQ-VAE技术,该方法能够捕捉语音中的情绪不确定性,生成动态面部表情,并维持准确的唇形同步。实验证明,该方法能有效创建多样且富有情感的说话人脸。
Key Takeaways
- DEEPTalk是一种新的方法,旨在解决现有面部动画方法在捕捉情绪方面的不足,提升动画的真实性和情感丰富度。
- DEEPTalk通过使用动态情绪嵌入(DEE)技术,结合概率对比学习,创建了一个联合的情绪嵌入空间,用于处理语音和面部运动的情感信息。
- 该方法采用时序层次VQ-VAE技术,生成动态面部表情,并设计了一种非自回归的预测编码本索引的说话头生成器。
- DEEPTalk能够捕捉语音中的情绪不确定性,使生成的面部表情更加自然和多样。
- 通过广泛实验验证,DEEPTalk在多种数据集上表现出良好的效果,能够创建准确唇形同步的、富有情感的说话人脸。
- DEEPTalk将公开源代码,以供研究使用。
点此查看论文截图
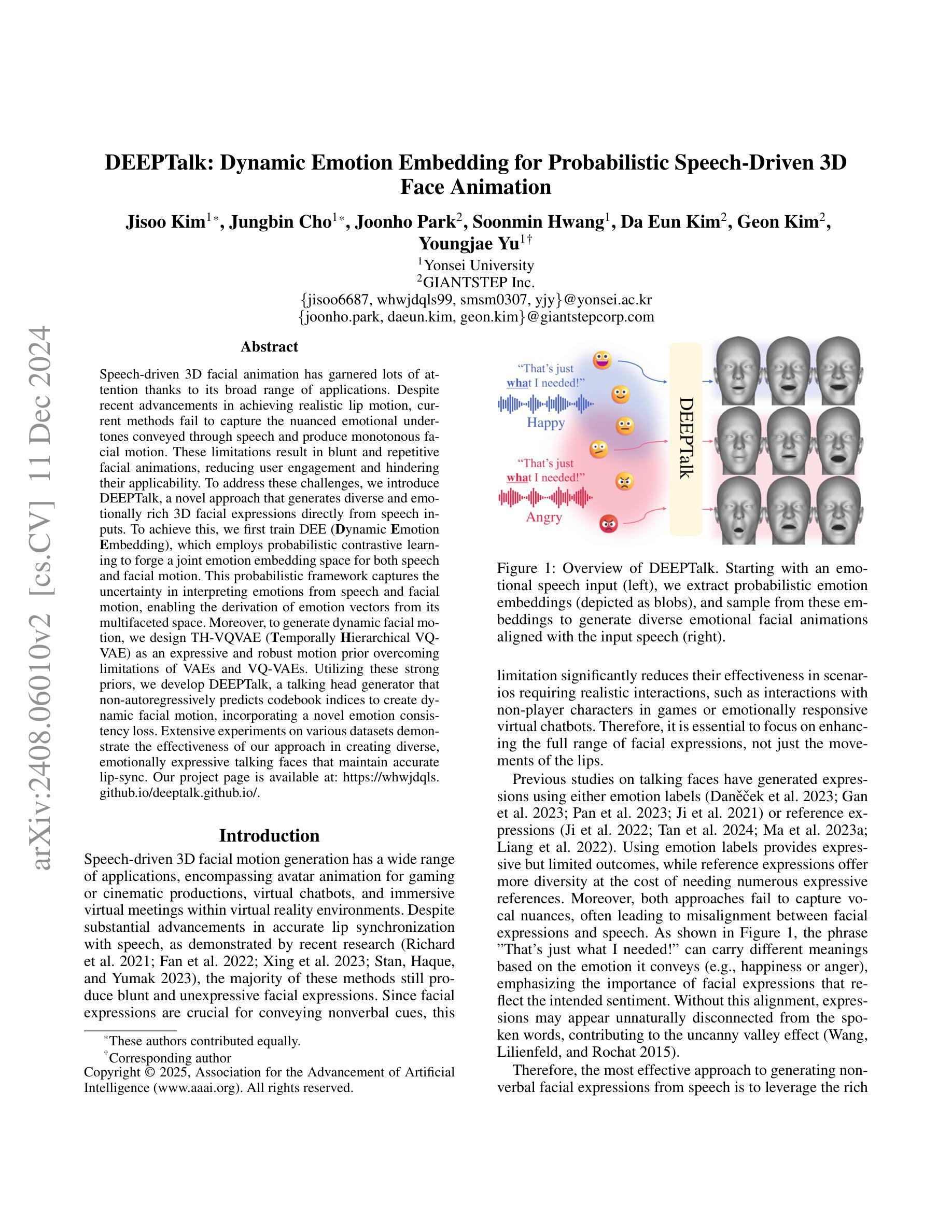
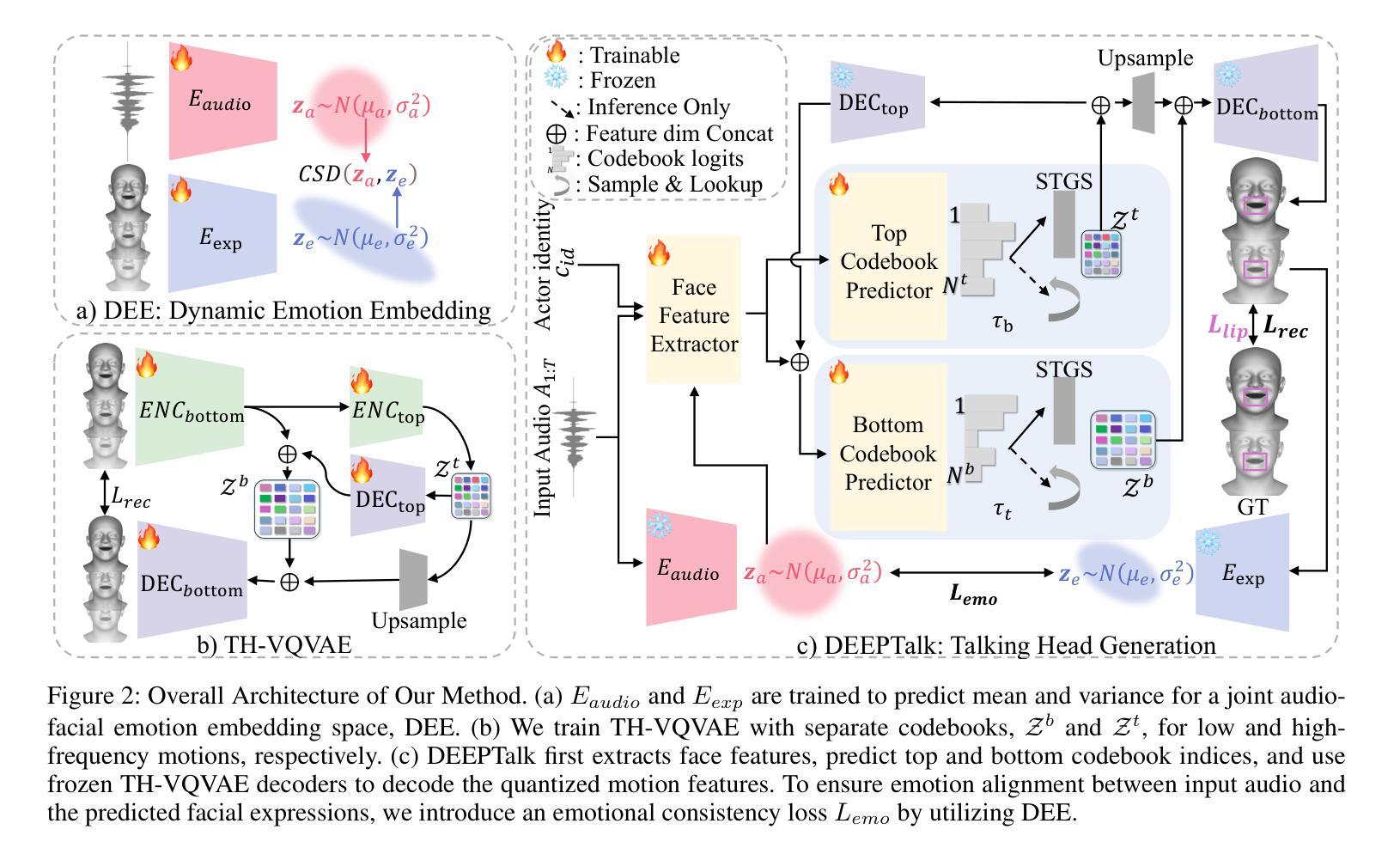
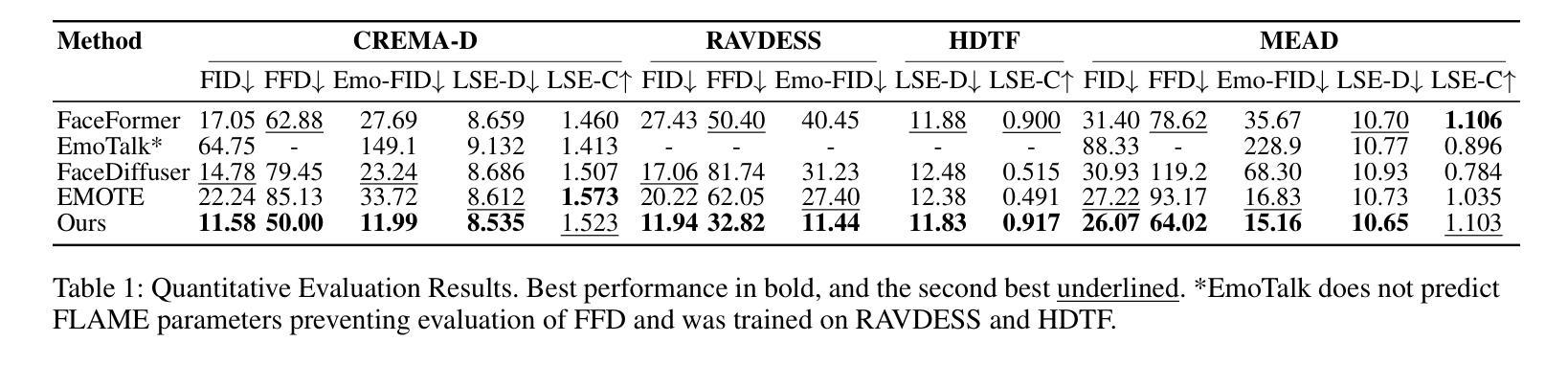
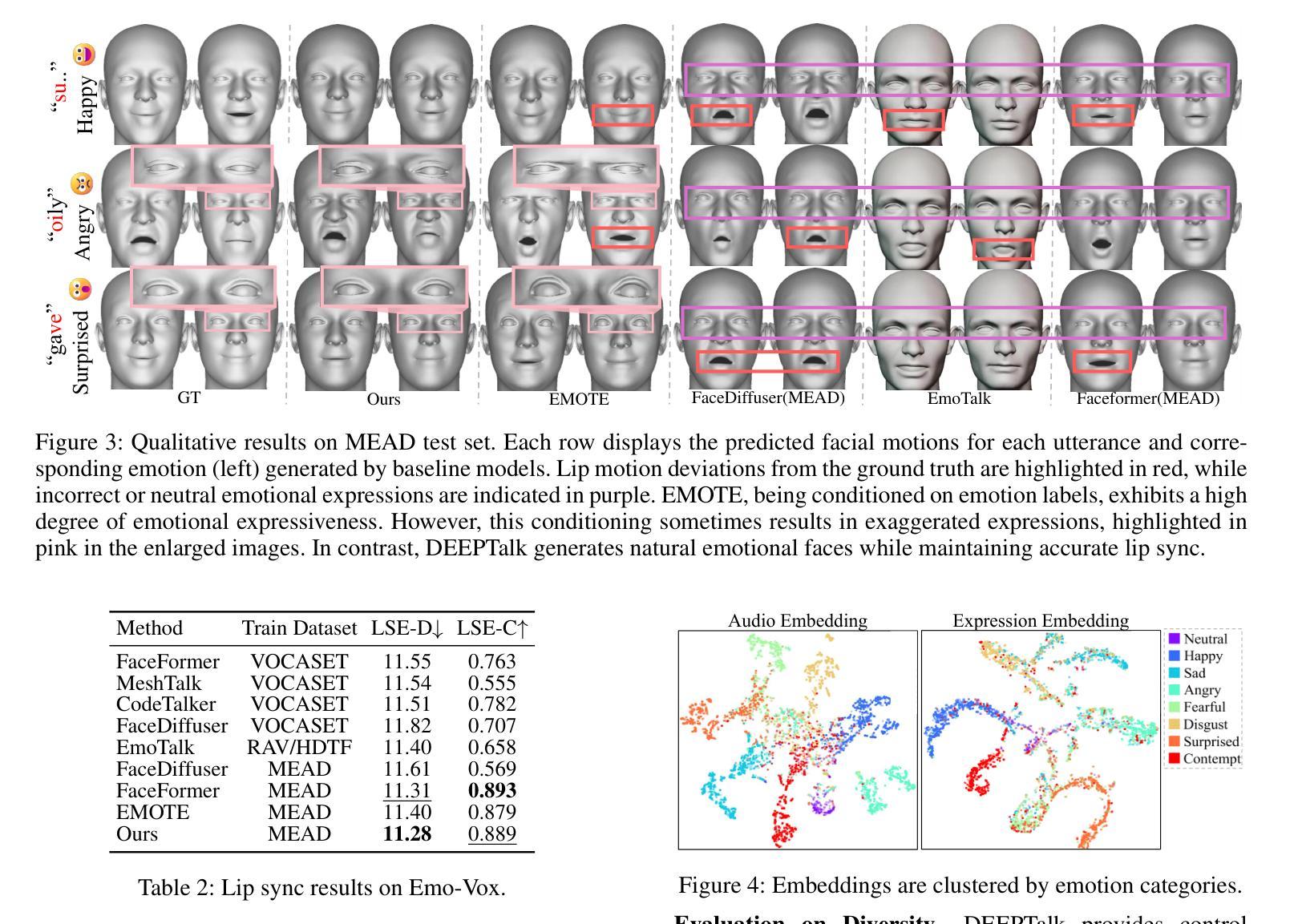
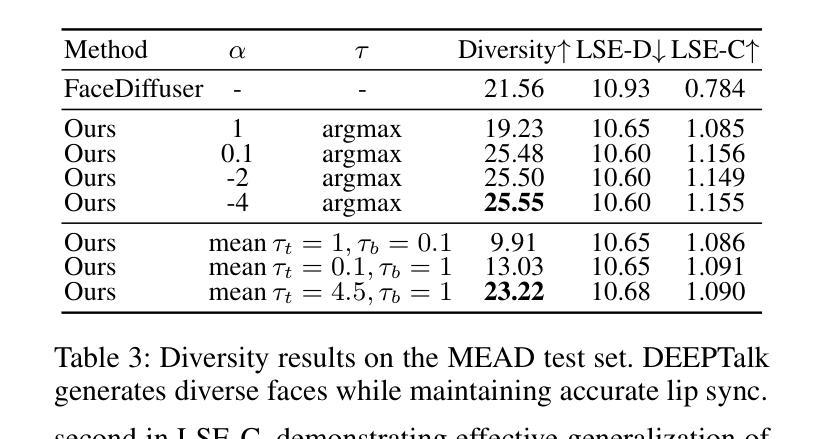