⚠️ 以下所有内容总结都来自于 大语言模型的能力,如有错误,仅供参考,谨慎使用
🔴 请注意:千万不要用于严肃的学术场景,只能用于论文阅读前的初筛!
💗 如果您觉得我们的项目对您有帮助 ChatPaperFree ,还请您给我们一些鼓励!⭐️ HuggingFace免费体验
2024-12-19 更新
GLCF: A Global-Local Multimodal Coherence Analysis Framework for Talking Face Generation Detection
Authors:Xiaocan Chen, Qilin Yin, Jiarui Liu, Wei Lu, Xiangyang Luo, Jiantao Zhou
Talking face generation (TFG) allows for producing lifelike talking videos of any character using only facial images and accompanying text. Abuse of this technology could pose significant risks to society, creating the urgent need for research into corresponding detection methods. However, research in this field has been hindered by the lack of public datasets. In this paper, we construct the first large-scale multi-scenario talking face dataset (MSTF), which contains 22 audio and video forgery techniques, filling the gap of datasets in this field. The dataset covers 11 generation scenarios and more than 20 semantic scenarios, closer to the practical application scenario of TFG. Besides, we also propose a TFG detection framework, which leverages the analysis of both global and local coherence in the multimodal content of TFG videos. Therefore, a region-focused smoothness detection module (RSFDM) and a discrepancy capture-time frame aggregation module (DCTAM) are introduced to evaluate the global temporal coherence of TFG videos, aggregating multi-grained spatial information. Additionally, a visual-audio fusion module (V-AFM) is designed to evaluate audiovisual coherence within a localized temporal perspective. Comprehensive experiments demonstrate the reasonableness and challenges of our datasets, while also indicating the superiority of our proposed method compared to the state-of-the-art deepfake detection approaches.
谈话人脸生成(TFG)技术仅使用面部图像和配套文本就能生成任何角色的逼真谈话视频。这项技术的滥用可能会对社会造成重大风险,因此亟需研究相应的检测方法。然而,这一领域的研究受到了公开数据集缺乏的阻碍。在本文中,我们构建了第一个大规模多场景谈话人脸数据集(MSTF),包含了22种音频和视频伪造技术,填补了该领域数据集的空白。该数据集涵盖了11种生成场景和超过20种语义场景,更接近于TFG的实际应用场景。此外,我们还提出了一个TFG检测框架,该框架利用TFG视频多媒体内容全局和局部一致性的分析。因此,引入了区域重点平滑检测模块(RSFDM)和差异捕获时间帧聚合模块(DCTAM)来评估TFG视频的全局时间一致性,聚合多粒度空间信息。另外,还设计了一个视听融合模块(V-AFM)来评估局部时间视角下的视听一致性。综合实验证明了我们数据集的合理性和挑战性以及我们提出的方法相较于最先进的深度伪造检测方法的优越性。
论文及项目相关链接
Summary:
该文本介绍了说话人脸生成技术(TFG)及其潜在风险,并指出缺乏公开数据集阻碍了相关研究。本研究构建了首个大规模多场景说话人脸数据集(MSTF),包含22种音频和视频伪造技术,填补了该领域的空白。同时,提出了一种TFG检测框架,通过评估TFG视频的全球时间一致性,引入区域重点平滑检测模块(RSFDM)和差异捕获时间帧聚合模块(DCTAM)。此外,还设计了视听融合模块(V-AFM)来评估局部时间内的视听一致性。实验证明了数据集方法的合理性及检测方法的优越性。
Key Takeaways:
- 说话人脸生成技术(TFG)能够产生逼真的人脸视频,但滥用可能会对社会产生风险,需要研究对应的检测方法。
- 缺乏公开数据集限制了该领域的研究进展。
- 研究构建了首个大规模多场景说话人脸数据集(MSTF),包含多种音频和视频伪造技术。
- 数据集覆盖了多种生成场景和语义场景,更接近TFG的实际应用场景。
- 提出了一种TFG检测框架,通过评估全球时间一致性和局部视听一致性来检测伪造视频。
- 引入了区域重点平滑检测模块(RSFDM)、差异捕获时间帧聚合模块(DCTAM)和视听融合模块(V-AFM)来增强检测效果。
点此查看论文截图
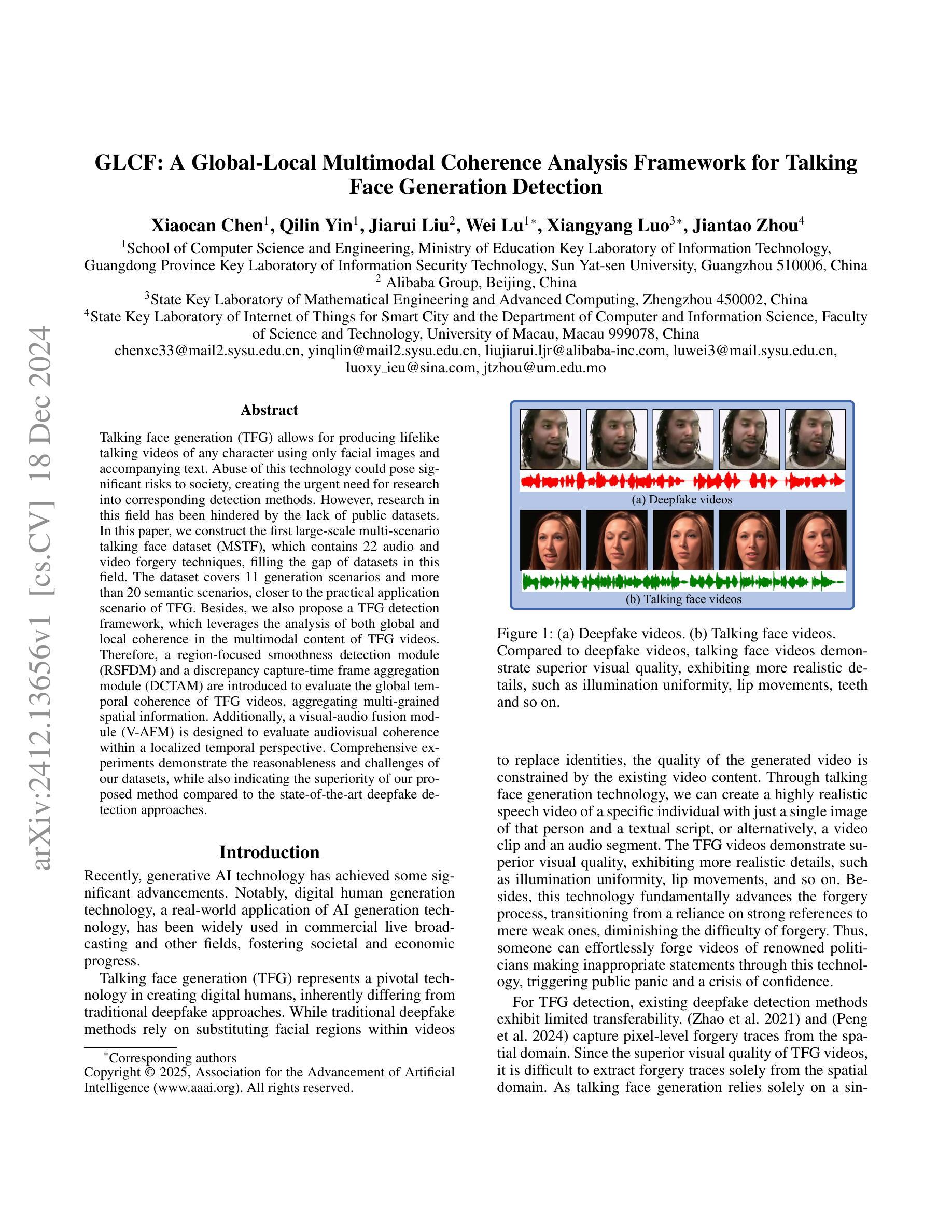
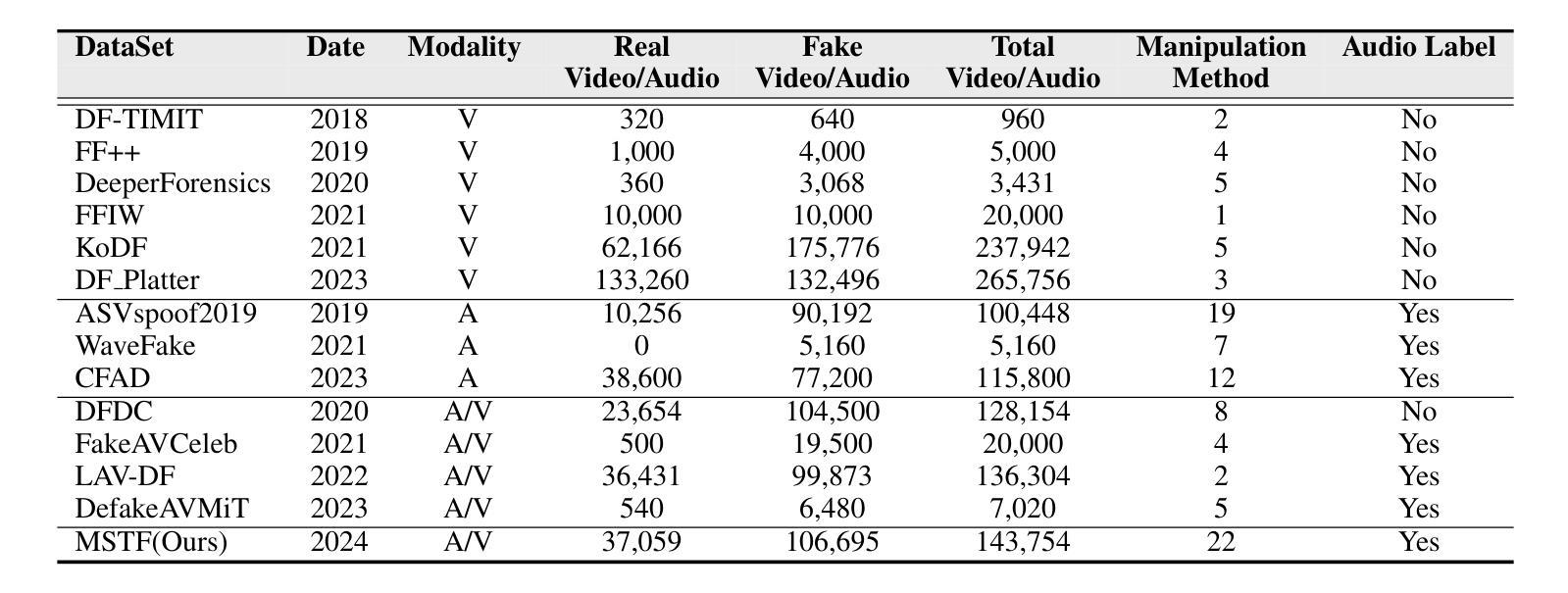
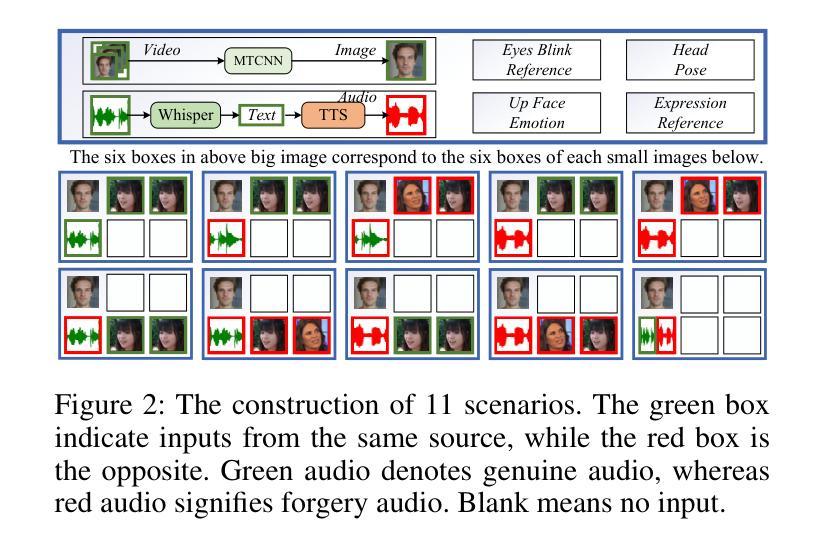
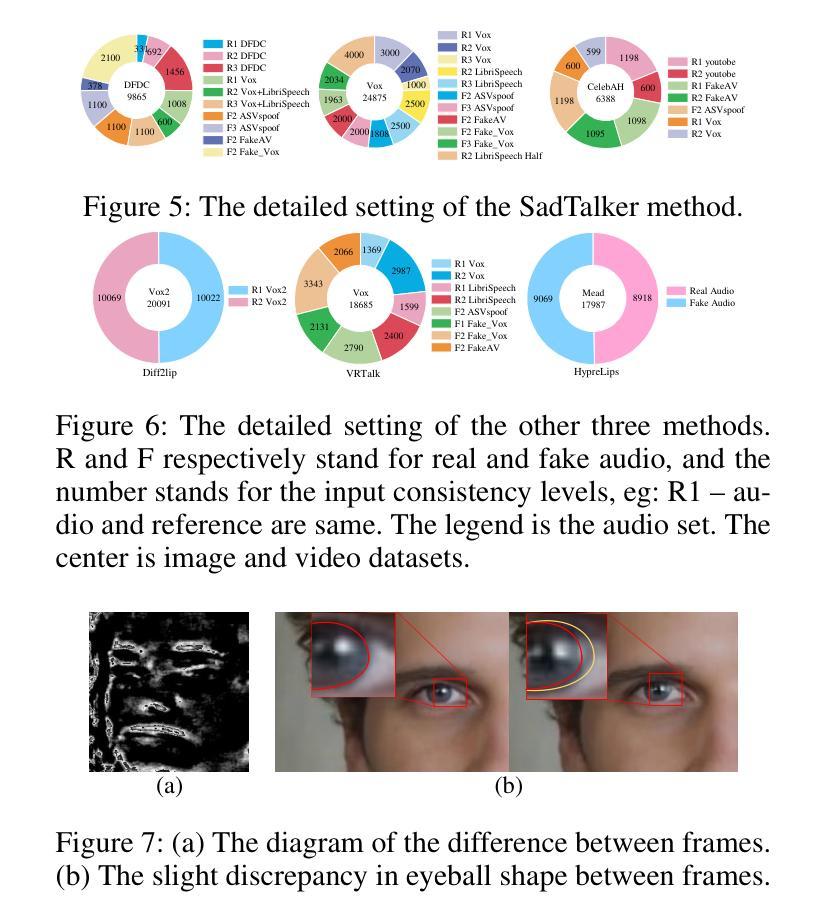
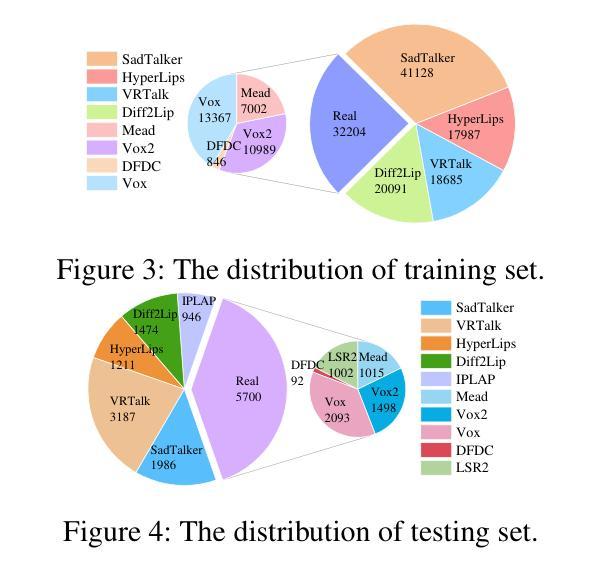
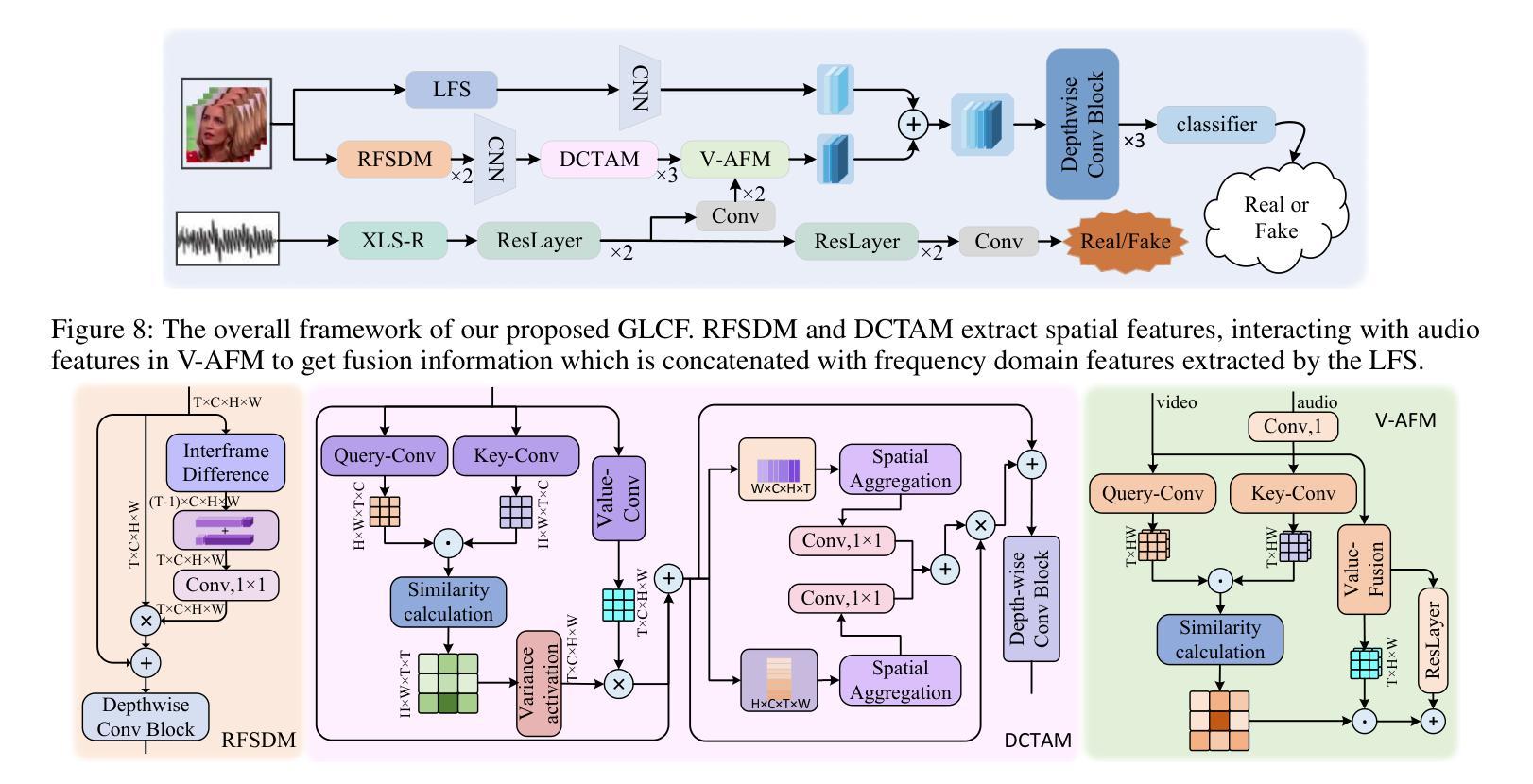