⚠️ 以下所有内容总结都来自于 大语言模型的能力,如有错误,仅供参考,谨慎使用
🔴 请注意:千万不要用于严肃的学术场景,只能用于论文阅读前的初筛!
💗 如果您觉得我们的项目对您有帮助 ChatPaperFree ,还请您给我们一些鼓励!⭐️ HuggingFace免费体验
2024-12-19 更新
Towards a Universal Synthetic Video Detector: From Face or Background Manipulations to Fully AI-Generated Content
Authors:Rohit Kundu, Hao Xiong, Vishal Mohanty, Athula Balachandran, Amit K. Roy-Chowdhury
Existing DeepFake detection techniques primarily focus on facial manipulations, such as face-swapping or lip-syncing. However, advancements in text-to-video (T2V) and image-to-video (I2V) generative models now allow fully AI-generated synthetic content and seamless background alterations, challenging face-centric detection methods and demanding more versatile approaches. To address this, we introduce the \underline{U}niversal \underline{N}etwork for \underline{I}dentifying \underline{T}ampered and synth\underline{E}tic videos (\texttt{UNITE}) model, which, unlike traditional detectors, captures full-frame manipulations. \texttt{UNITE} extends detection capabilities to scenarios without faces, non-human subjects, and complex background modifications. It leverages a transformer-based architecture that processes domain-agnostic features extracted from videos via the SigLIP-So400M foundation model. Given limited datasets encompassing both facial/background alterations and T2V/I2V content, we integrate task-irrelevant data alongside standard DeepFake datasets in training. We further mitigate the model’s tendency to over-focus on faces by incorporating an attention-diversity (AD) loss, which promotes diverse spatial attention across video frames. Combining AD loss with cross-entropy improves detection performance across varied contexts. Comparative evaluations demonstrate that \texttt{UNITE} outperforms state-of-the-art detectors on datasets (in cross-data settings) featuring face/background manipulations and fully synthetic T2V/I2V videos, showcasing its adaptability and generalizable detection capabilities.
现有的深度伪造检测技术主要侧重于面部操作,如换脸或同步嘴唇。然而,文本到视频(T2V)和图像到视频(I2V)生成模型的进步现在允许完全由人工智能生成的合成内容和无缝的背景更改,这对面部中心的检测方法和更通用的方法提出了挑战。针对这一问题,我们引入了“通用网络,用于识别篡改和合成视频(UNITE)模型”,与传统的检测器不同,它可以捕获全帧操作。UNITE将检测能力扩展到没有面部、非人类主体和复杂背景修改的场景。它利用基于变压器的架构,处理通过SigLIP-So400M基础模型从视频中提取的领域无关特征。在包含面部/背景更改以及T2V/I2V内容的有限数据集的情况下,我们将任务不相关的数据与标准深度伪造数据集一起用于训练。我们通过融入注意力多样性(AD)损失来进一步减轻模型过度关注面部的倾向,该损失促进视频帧内空间注意力的多样化。将AD损失与交叉熵结合,提高了不同环境下的检测性能。比较评估表明,UNITE在包含面部/背景操作和完全合成的T2V/I2V视频的数据集上(跨数据设置)超越了最新检测器,展示了其适应性和可泛化的检测能力。
论文及项目相关链接
摘要
随着文本转视频(T2V)和图像转视频(I2V)生成模型的进步,AI生成的内容越来越逼真,背景改动无缝衔接,给传统的以面部为中心的检测方式带来挑战。为解决此问题,我们引入了通用网络识别篡改和合成视频(UNITE)模型。该模型不同于传统检测器,能捕捉全帧操作,扩展了检测能力,适用于无面部、非人类主体和复杂背景修改的场景。UNITE利用基于变压器的架构,处理通过SigLIP-So400M基础模型从视频中提取的领域无关特征。在包含面部/背景修改以及T2V/I2V内容的数据集有限的情况下,我们将任务不相关数据与标准DeepFake数据集相结合进行训练。通过引入注意力多样性(AD)损失,缓解模型过度关注面部的倾向,促进视频帧的空间注意力多样化。结合AD损失和交叉熵可以提高不同环境下的检测性能。对比评估表明,UNITE在包含面部/背景操作和完全合成的T2V/I2V视频的数据集上,优于当前最先进的检测器,展现了其适应性和通用检测能力。
关键见解
- 现有DeepFake检测技术主要关注面部操作,但新型生成模型带来的挑战使其需要更通用的检测方法。
- 引入的UNITE模型能够捕捉全帧操作,适用于多种场景,包括无面部、非人类主体和复杂背景修改。
- UNITE利用基于变压器的架构处理领域无关特征,并通过SigLIP-So400M基础模型从视频中提取这些特征。
- 在训练过程中,UNITE结合了任务不相关数据与标准DeepFake数据集,以应对有限的数据集问题。
- 通过引入注意力多样性(AD)损失,UNITE能够平衡面部与其他视频内容的关注,提高检测性能。
- 结合AD损失和交叉熵损失有助于提高不同环境下的检测性能。
点此查看论文截图
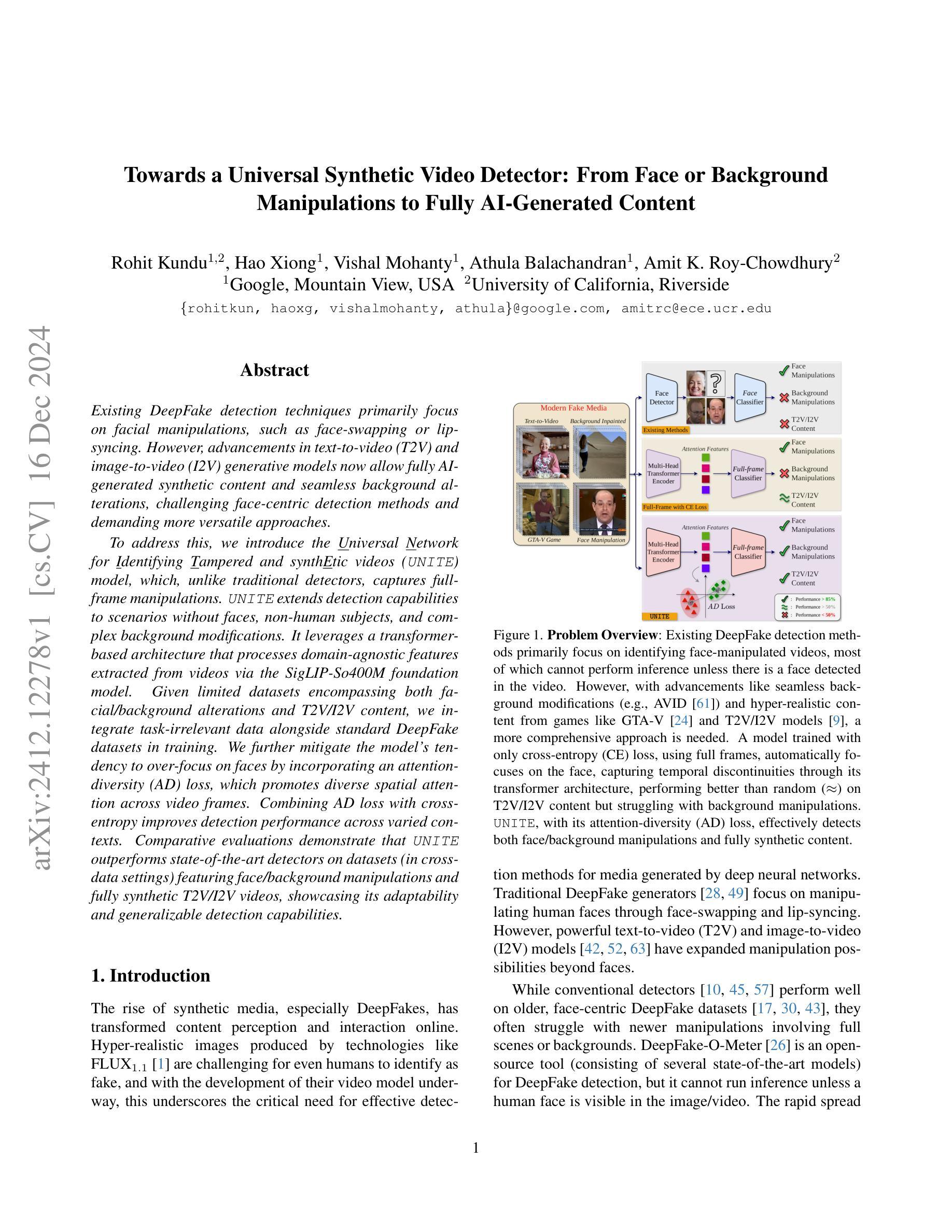
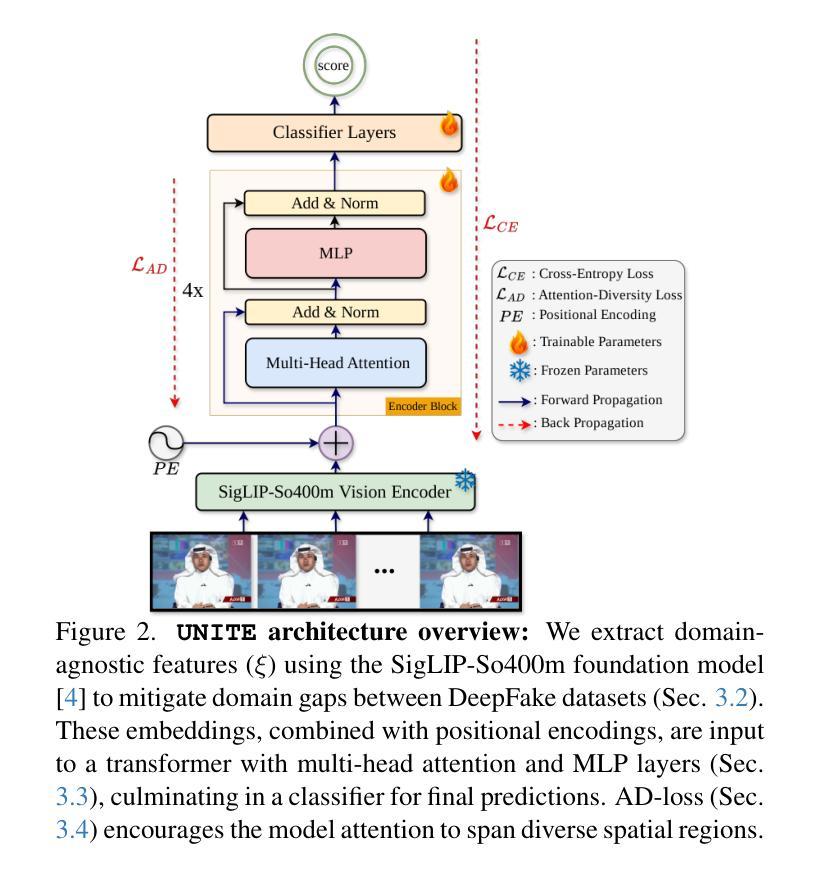
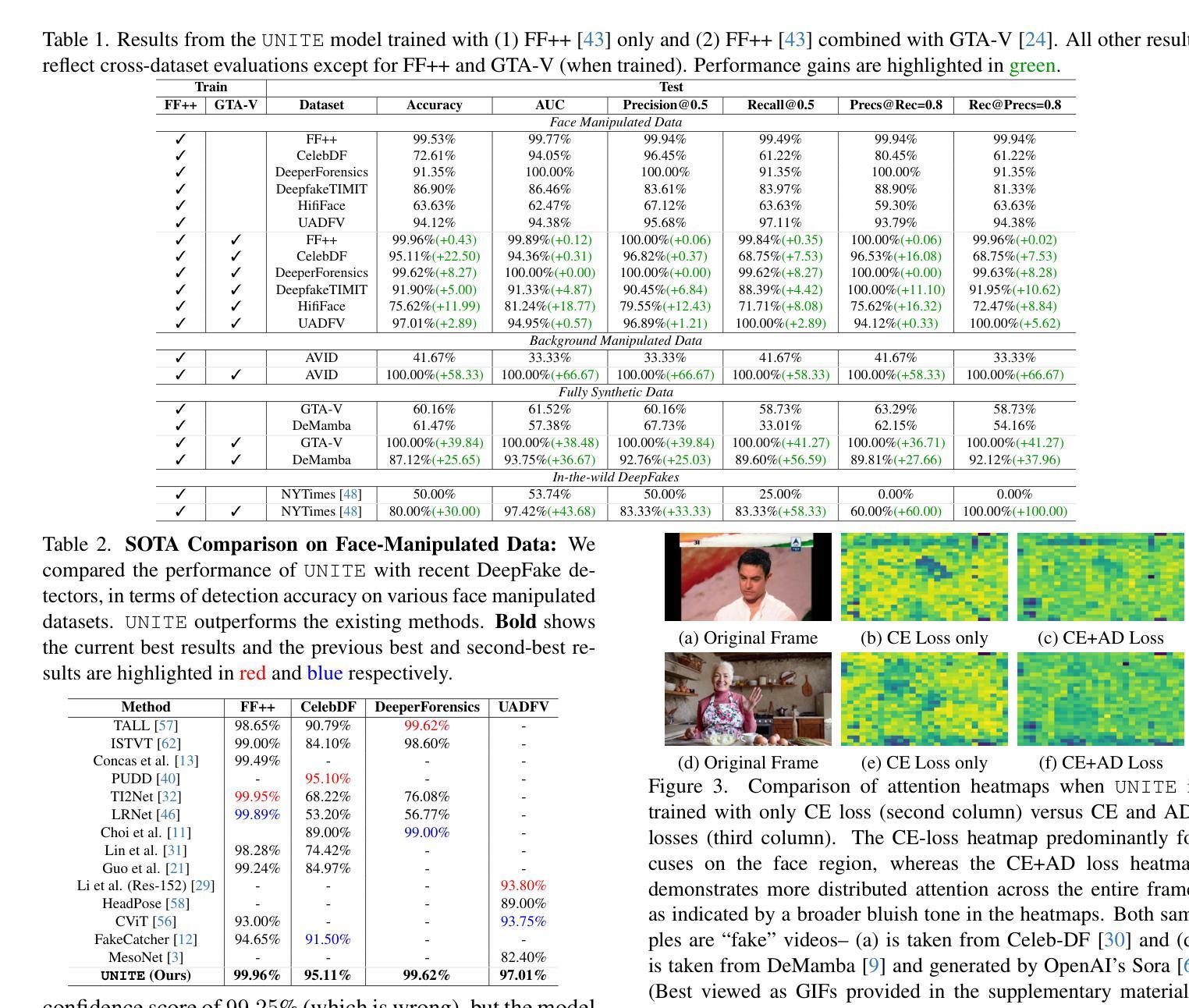
THESAURUS: Contrastive Graph Clustering by Swapping Fused Gromov-Wasserstein Couplings
Authors:Bowen Deng, Tong Wang, Lele Fu, Sheng Huang, Chuan Chen, Tao Zhang
Graph node clustering is a fundamental unsupervised task. Existing methods typically train an encoder through selfsupervised learning and then apply K-means to the encoder output. Some methods use this clustering result directly as the final assignment, while others initialize centroids based on this initial clustering and then finetune both the encoder and these learnable centroids. However, due to their reliance on K-means, these methods inherit its drawbacks when the cluster separability of encoder output is low, facing challenges from the Uniform Effect and Cluster Assimilation. We summarize three reasons for the low cluster separability in existing methods: (1) lack of contextual information prevents discrimination between similar nodes from different clusters; (2) training tasks are not sufficiently aligned with the downstream clustering task; (3) the cluster information in the graph structure is not appropriately exploited. To address these issues, we propose conTrastive grapH clustEring by SwApping fUsed gRomov-wasserstein coUplingS (THESAURUS). Our method introduces semantic prototypes to provide contextual information, and employs a cross-view assignment prediction pretext task that aligns well with the downstream clustering task. Additionally, it utilizes Gromov-Wasserstein Optimal Transport (GW-OT) along with the proposed prototype graph to thoroughly exploit cluster information in the graph structure. To adapt to diverse real-world data, THESAURUS updates the prototype graph and the prototype marginal distribution in OT by using momentum. Extensive experiments demonstrate that THESAURUS achieves higher cluster separability than the prior art, effectively mitigating the Uniform Effect and Cluster Assimilation issues
图节点聚类是一项基本的无监督任务。现有方法通常通过自监督学习训练编码器,然后应用于编码器的输出进行K-means聚类。一些方法直接使用此聚类结果作为最终分配,而其他方法则基于此初始聚类初始化质心,然后微调编码器和这些可学习的质心。然而,由于它们依赖于K-means,这些方法继承了当编码器输出的聚类可分性较低时的缺点,面临着来自统一效应和集群同化等挑战。我们总结了现有方法中低聚类可分性的三个原因:(1)缺乏上下文信息,无法区分来自不同簇的相似节点;(2)训练任务与下游聚类任务不够对齐;(3)图结构中的聚类信息没有得到适当利用。针对这些问题,我们提出了通过交换使用的Gromov-Wasserstein耦合的对比图聚类(THESAURUS)。我们的方法引入了语义原型以提供上下文信息,并采用与下游聚类任务对齐良好的跨视图分配预测文本任务。此外,它利用Gromov-Wasserstein最优传输(GW-OT)和提出的原型图,以充分利用图结构中的聚类信息。为了适应多样化的现实世界数据,THESAURUS通过使用动量来更新原型图和OT中的原型边缘分布。大量实验表明,THESAURUS在聚类可分性方面优于先前技术,有效地缓解了统一效应和集群同化问题。
论文及项目相关链接
PDF Accepted by AAAI 2025
Summary
本文指出图节点聚类是一项基本的无监督任务,现有方法通常通过自监督学习训练编码器,然后应用K-means到编码器输出进行聚类。然而,由于依赖K-means,这些方法在簇分离度较低时面临挑战,如均匀效应和簇同化问题。为解决这些问题,本文提出通过交换使用的Gromov-Wasserstein耦合进行对比图聚类(THESAURUS)。该方法引入语义原型提供上下文信息,采用与下游聚类任务相匹配的跨视图分配预测前导任务,并利用Gromov-Wasserstein最优传输和提出的原型图彻底挖掘图结构中的聚类信息。实验表明,THESAURUS提高了簇分离度,有效缓解了均匀效应和簇同化问题。
Key Takeaways
- 图节点聚类是无监督学习的重要任务之一。
- 现有方法大多通过自监督学习训练编码器并结合K-means进行聚类。
- K-means方法存在簇分离度低的问题,引发均匀效应和簇同化挑战。
- 为解决这些问题,提出THESAURUS方法,引入语义原型提供上下文信息。
- THESAURUS采用跨视图分配预测前导任务,与下游聚类任务相匹配。
- Gromov-Wasserstein最优传输和原型图被用于挖掘图结构中的聚类信息。
点此查看论文截图
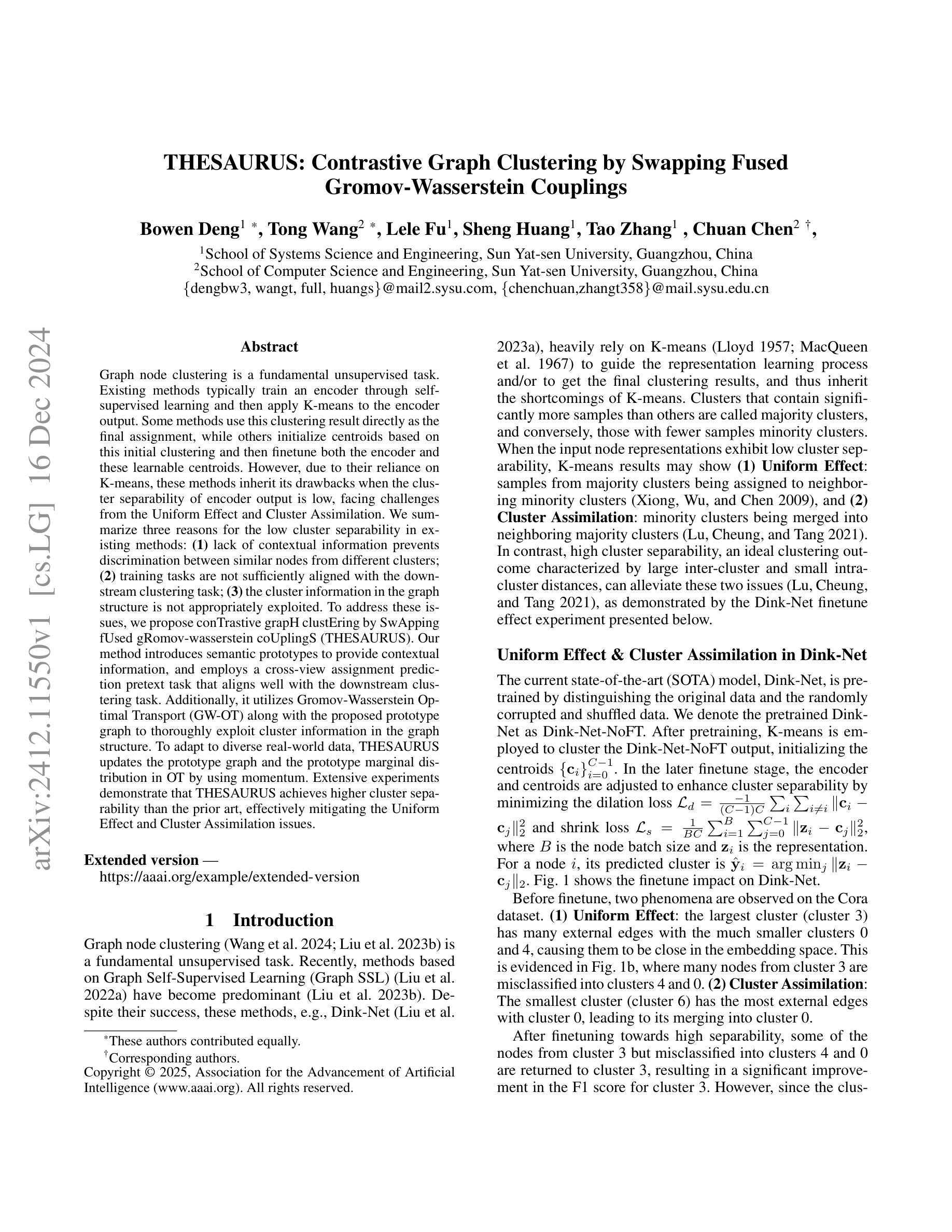
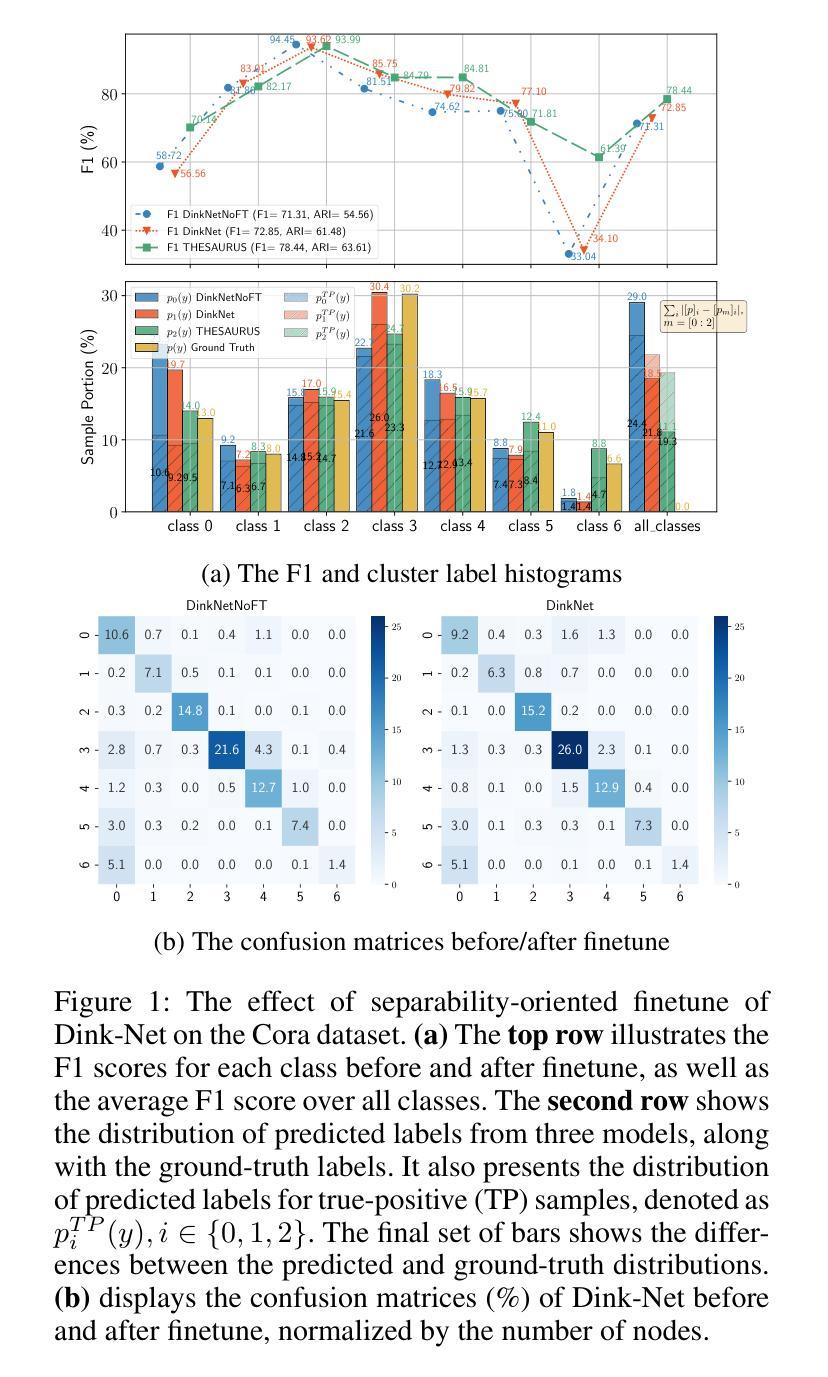
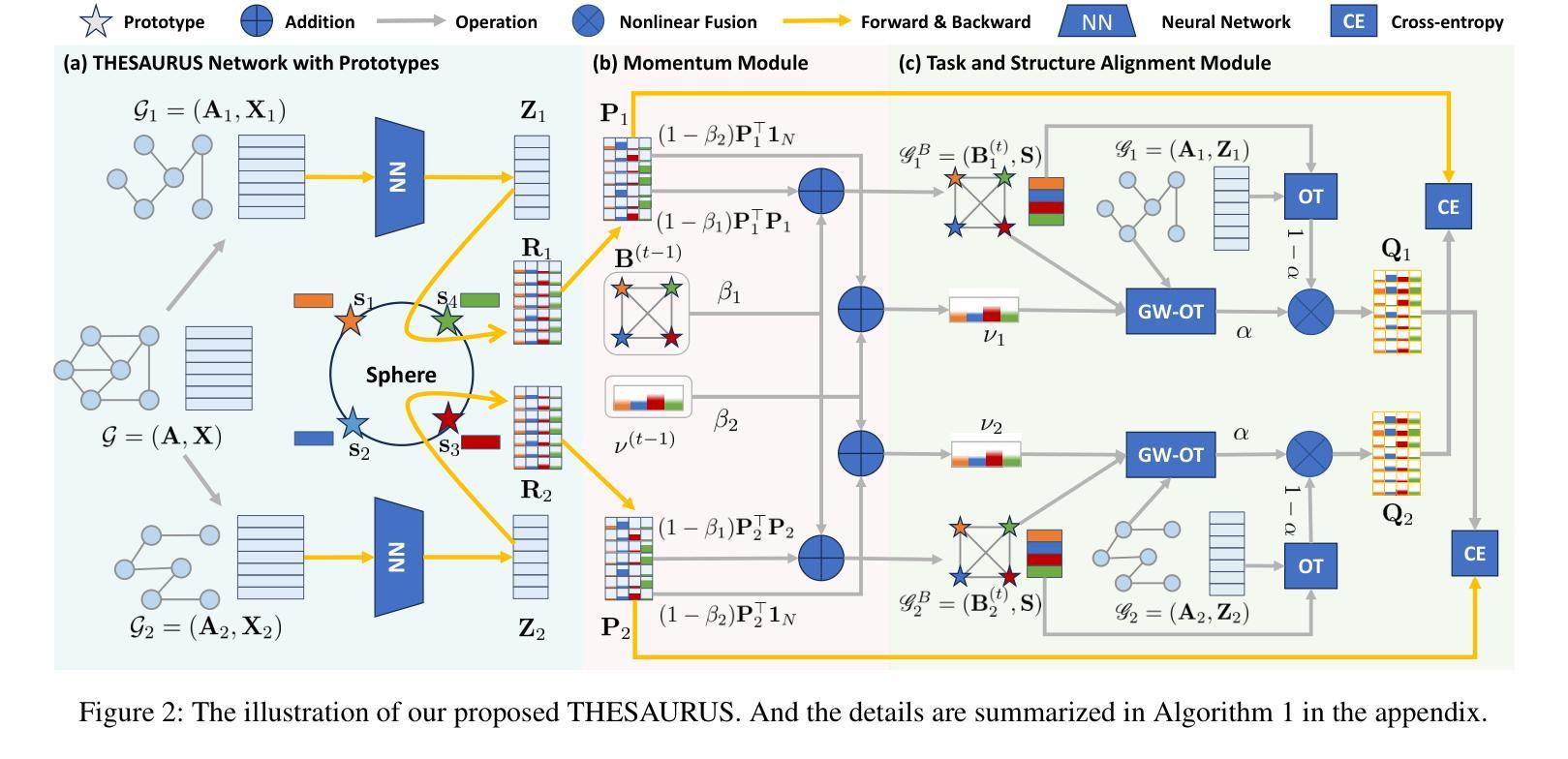
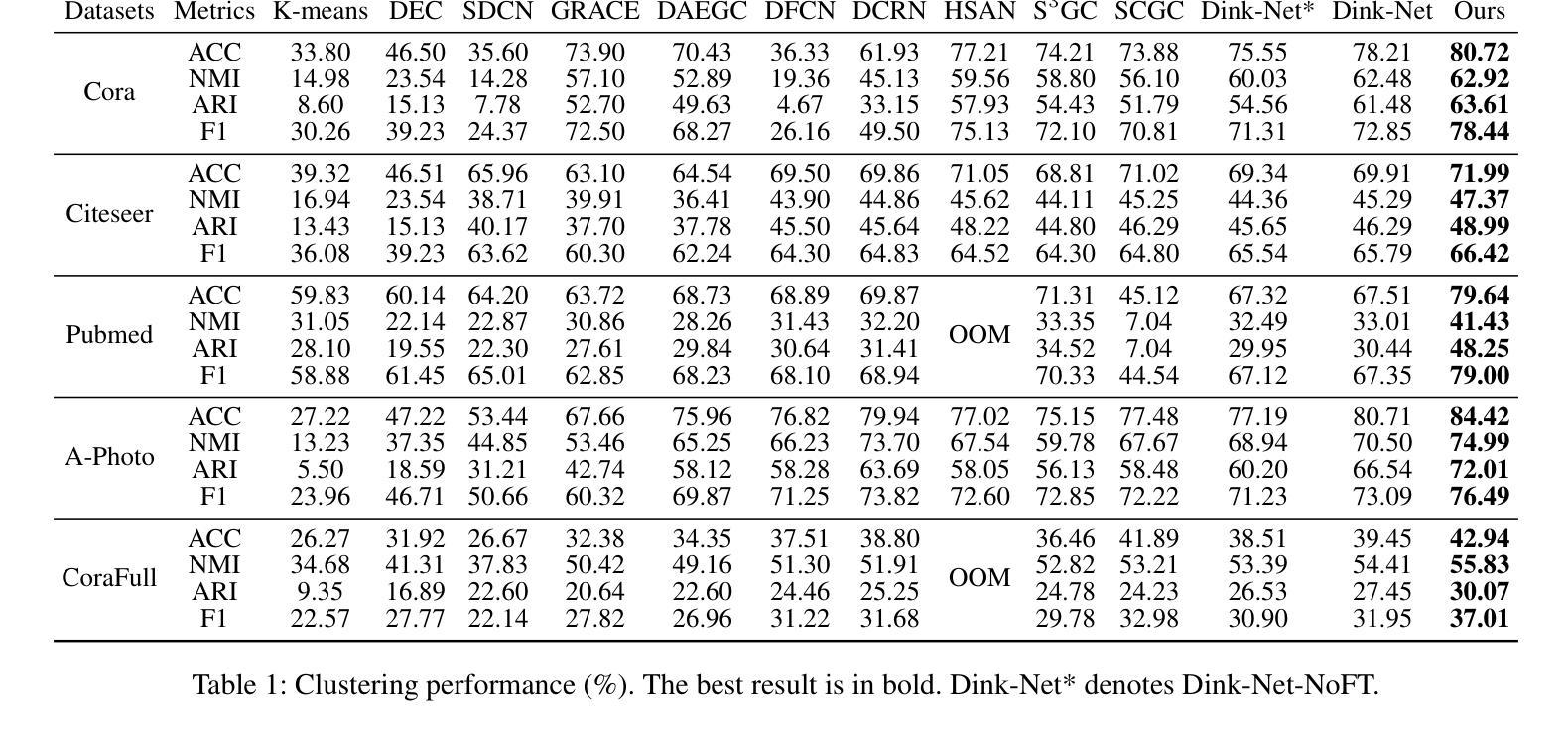
Flash Diffusion: Accelerating Any Conditional Diffusion Model for Few Steps Image Generation
Authors:Clément Chadebec, Onur Tasar, Eyal Benaroche, Benjamin Aubin
In this paper, we propose an efficient, fast, and versatile distillation method to accelerate the generation of pre-trained diffusion models: Flash Diffusion. The method reaches state-of-the-art performances in terms of FID and CLIP-Score for few steps image generation on the COCO2014 and COCO2017 datasets, while requiring only several GPU hours of training and fewer trainable parameters than existing methods. In addition to its efficiency, the versatility of the method is also exposed across several tasks such as text-to-image, inpainting, face-swapping, super-resolution and using different backbones such as UNet-based denoisers (SD1.5, SDXL) or DiT (Pixart-$\alpha$), as well as adapters. In all cases, the method allowed to reduce drastically the number of sampling steps while maintaining very high-quality image generation. The official implementation is available at https://github.com/gojasper/flash-diffusion.
本文提出了一种高效、快速且通用的蒸馏方法,用于加速预训练扩散模型的生成:Flash Diffusion。该方法在COCO2014和COCO2017数据集上的少量步骤图像生成方面达到了最先进的FID和CLIP分数表现,同时只需要几个小时的GPU训练时间,并且相比现有方法需要更少的可训练参数。除了高效性之外,该方法还展现出跨多个任务的通用性,如文本到图像、补全、换脸、超分辨率以及使用不同的主干网络(如基于UNet的去噪器(SD1.5、SDXL)或DiT(Pixart-α))以及适配器。在所有情况下,该方法在保持非常高的图像生成质量的同时,大大降低了采样步骤的数量。官方实现可访问https://github.com/gojasper/flash-diffusion获取。
论文及项目相关链接
PDF Accepted to AAAI 2025
摘要
本文提出了一种高效、快速且通用的蒸馏方法,用于加速预训练扩散模型的生成:Flash Diffusion。该方法在COCO2014和COCO2017数据集上进行少量步骤的图像生成时,在FID和CLIP-Score方面达到了最先进的性能。该方法只需要几个小时的GPU训练时间,而且相比现有方法,需要的可训练参数更少。除了高效性外,该方法还展示了跨多个任务的通用性,如文本到图像、图像修复、面部替换、超分辨率以及使用不同的主干网络(如基于UNet的去噪器SD1.5、SDXL或DiT(Pixart-$\alpha$))或适配器。在所有情况下,该方法在大大减少采样步骤数量的同时,保持了非常高的图像生成质量。官方实现可访问https://github.com/gojasper/flash-diffusion获取。
要点摘要
- 提出了一种名为Flash Diffusion的高效蒸馏方法,用于加速预训练扩散模型的图像生成。
- 在FID和CLIP-Score指标上达到了先进的性能水平,特别是在少量步骤的图像生成方面。
- 训练时间短,仅需要几个小时的GPU时间。
- 与现有方法相比,所需的训练参数大大减少。
- 该方法具有很高的通用性,可应用于多个任务,包括文本到图像、图像修复、面部替换、超分辨率等。
- 支持多种不同的主干网络和适配器,展示了其灵活性和适应性。
点此查看论文截图
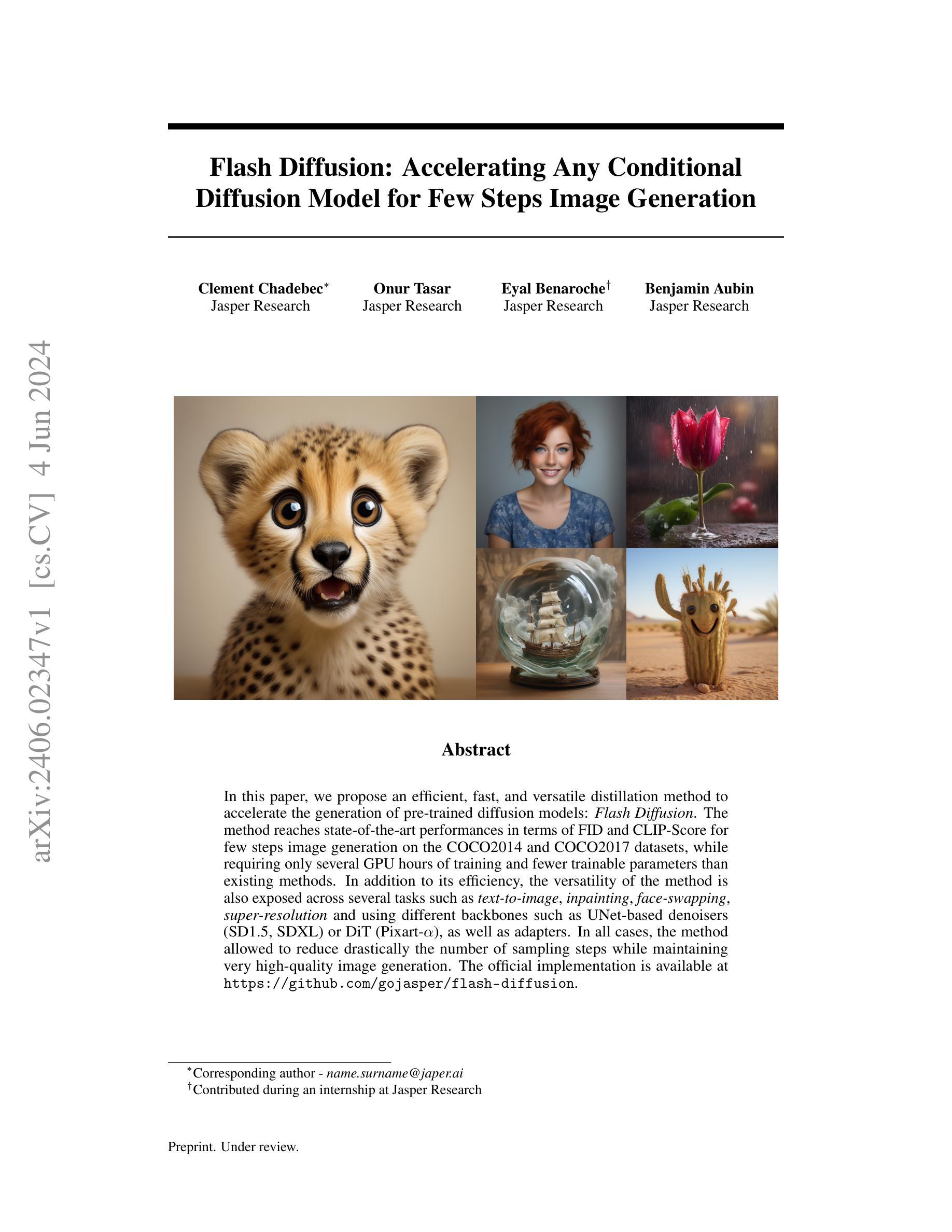
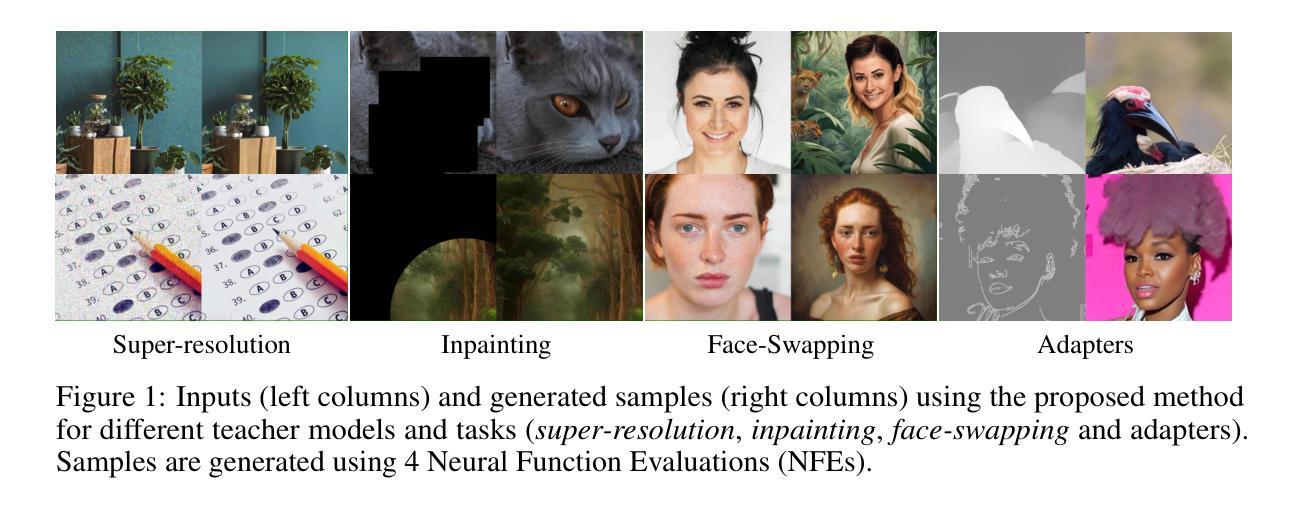
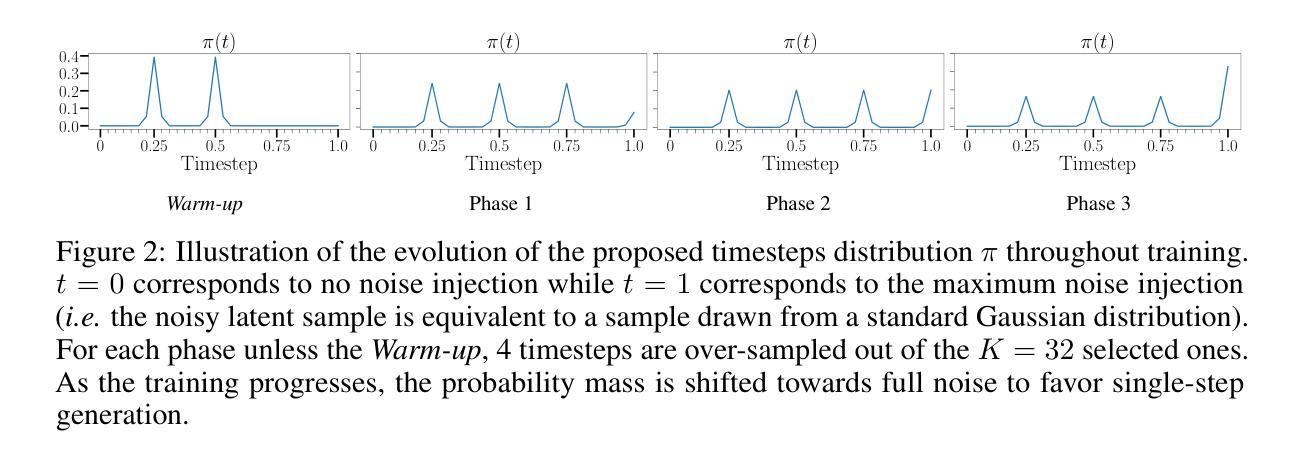