⚠️ 以下所有内容总结都来自于 大语言模型的能力,如有错误,仅供参考,谨慎使用
🔴 请注意:千万不要用于严肃的学术场景,只能用于论文阅读前的初筛!
💗 如果您觉得我们的项目对您有帮助 ChatPaperFree ,还请您给我们一些鼓励!⭐️ HuggingFace免费体验
2024-12-19 更新
GLCF: A Global-Local Multimodal Coherence Analysis Framework for Talking Face Generation Detection
Authors:Xiaocan Chen, Qilin Yin, Jiarui Liu, Wei Lu, Xiangyang Luo, Jiantao Zhou
Talking face generation (TFG) allows for producing lifelike talking videos of any character using only facial images and accompanying text. Abuse of this technology could pose significant risks to society, creating the urgent need for research into corresponding detection methods. However, research in this field has been hindered by the lack of public datasets. In this paper, we construct the first large-scale multi-scenario talking face dataset (MSTF), which contains 22 audio and video forgery techniques, filling the gap of datasets in this field. The dataset covers 11 generation scenarios and more than 20 semantic scenarios, closer to the practical application scenario of TFG. Besides, we also propose a TFG detection framework, which leverages the analysis of both global and local coherence in the multimodal content of TFG videos. Therefore, a region-focused smoothness detection module (RSFDM) and a discrepancy capture-time frame aggregation module (DCTAM) are introduced to evaluate the global temporal coherence of TFG videos, aggregating multi-grained spatial information. Additionally, a visual-audio fusion module (V-AFM) is designed to evaluate audiovisual coherence within a localized temporal perspective. Comprehensive experiments demonstrate the reasonableness and challenges of our datasets, while also indicating the superiority of our proposed method compared to the state-of-the-art deepfake detection approaches.
面部说话生成(TFG)技术仅使用面部图像和配套文本就能生成任何角色的逼真说话视频。该技术的滥用可能会对社会造成重大风险,因此迫切需要研究相应的检测方法。然而,这一领域的研究一直受到公开数据集缺乏的阻碍。在本文中,我们构建了第一个大规模多场景面部说话数据集(MSTF),包含22种音频和视频伪造技术,填补了该领域的数据集空白。该数据集涵盖11种生成场景和超过20种语义场景,更接近于TFG的实际应用场景。此外,我们还提出了一种TFG检测框架,该框架利用对TFG视频多媒体内容全局和局部一致性的分析。因此,引入了区域重点平滑检测模块(RSFDM)和差异捕获时间帧聚合模块(DCTAM),以评估TFG视频的全局时间一致性,聚合多粒度空间信息。此外,还设计了一个视听融合模块(V-AFM),以在局部时间角度内评估视听一致性。综合实验证明了数据集的合理性和挑战性,同时也表明我们提出的方法相较于最新的深度伪造检测方法具有优越性。
论文及项目相关链接
Summary
本文介绍了说话脸生成(TFG)技术,该技术能够仅使用面部图像和文本生成逼真的说话视频。然而,由于缺乏公共数据集,该领域的研究受到了阻碍。本文构建了第一个大规模多场景说话脸数据集(MSTF),包含22种音视频伪造技术,填补了该领域的空白。此外,还提出了一个TFG检测框架,该框架利用对TFG视频的多模态内容的全局和局部一致性的分析,引入了一个重点区域平滑检测模块(RSFDM)和一个差异捕获时间帧聚合模块(DCTAM)来评估TFG视频的全局时间一致性。同时,还设计了一个视觉-音频融合模块(V-AFM)来评估局部时间范围内的视听一致性。实验证明了数据集的合理性及其挑战性以及相较于现有深度伪造检测方法的优越性。
Key Takeaways
- 说话脸生成(TFG)技术能够使用面部图像和文本生成逼真的说话视频。
- 缺乏公共数据集限制了TFG领域的研究进展。
- 构建了第一个大规模多场景说话脸数据集(MSTF),包含多种音视频伪造技术。
- 提出了一个TFG检测框架,利用全局和局部一致性分析TFG视频的多模态内容。
- 通过重点区域平滑检测模块(RSFDM)和差异捕获时间帧聚合模块(DCTAM)评估TFG视频的全局时间一致性。
- 设计了视觉-音频融合模块(V-AFM)以评估局部时间范围内的视听一致性。
点此查看论文截图
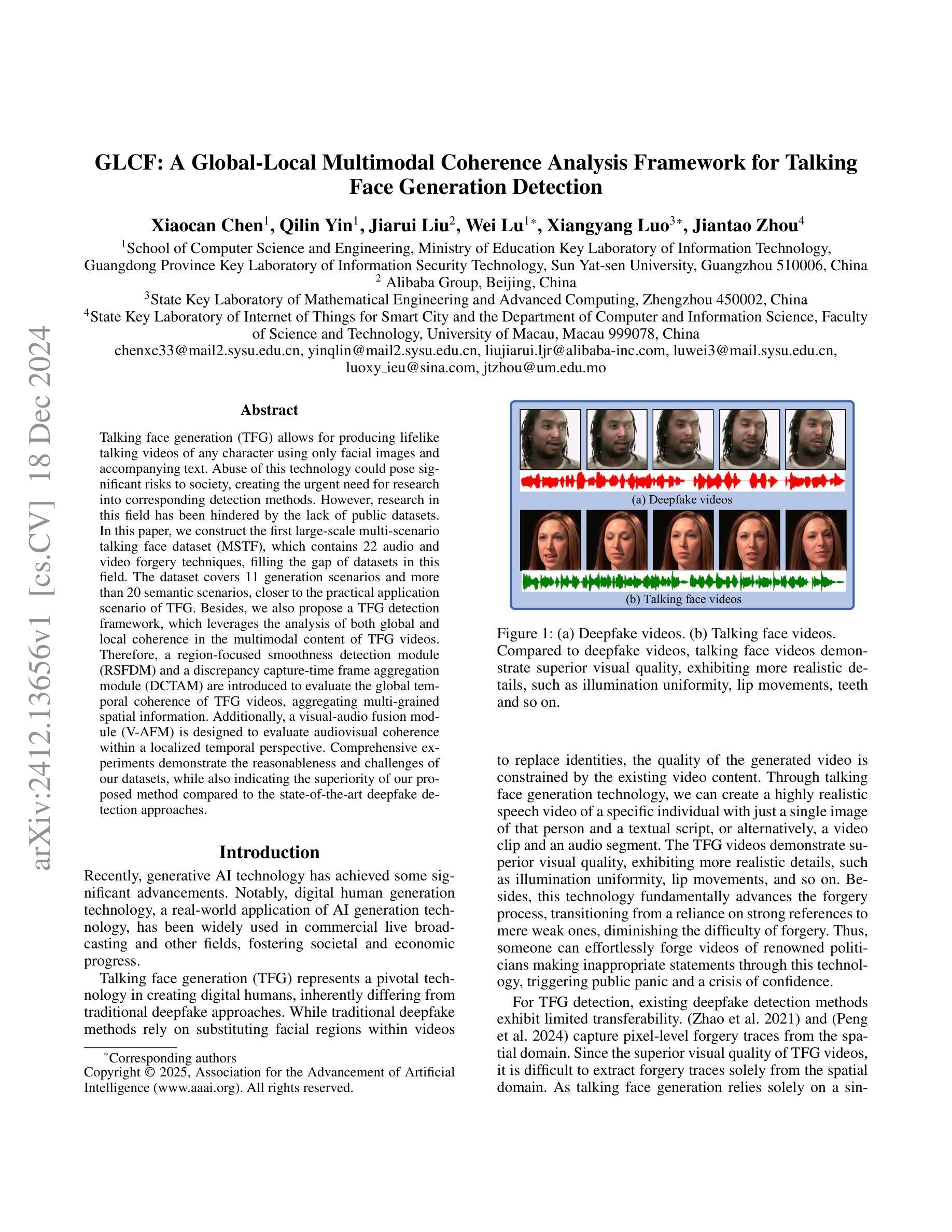
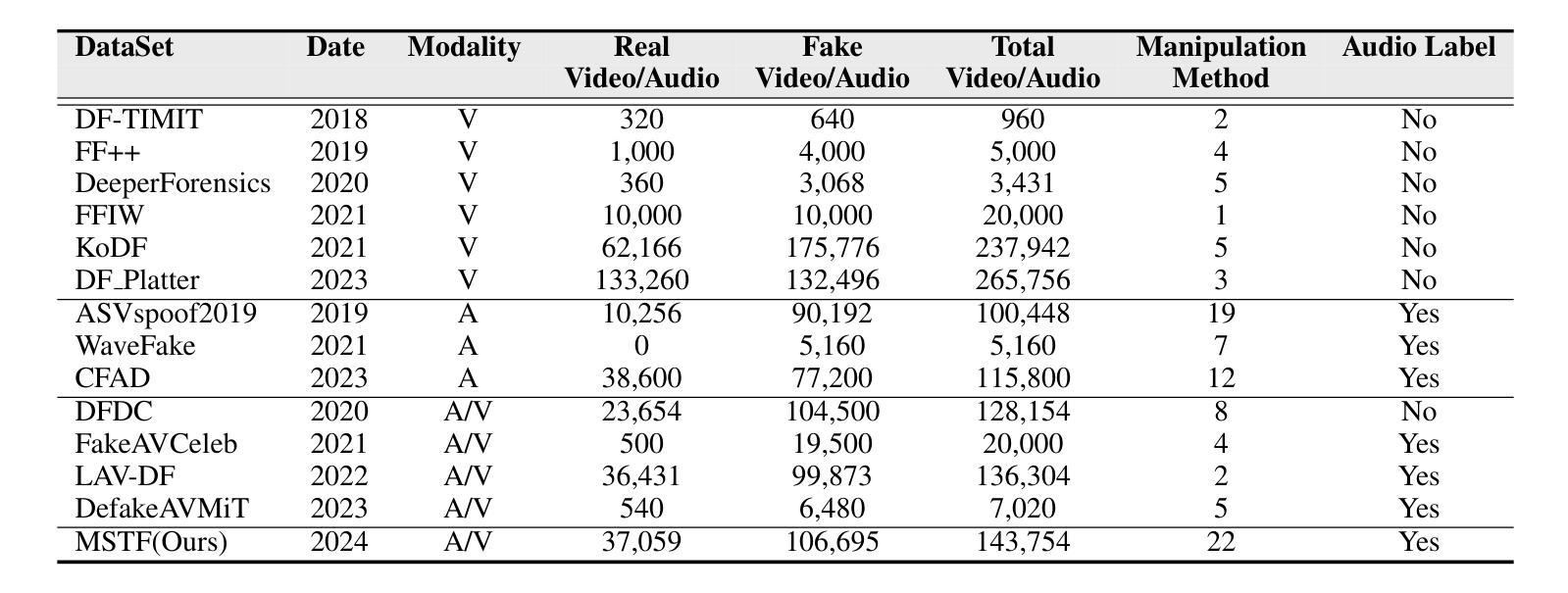
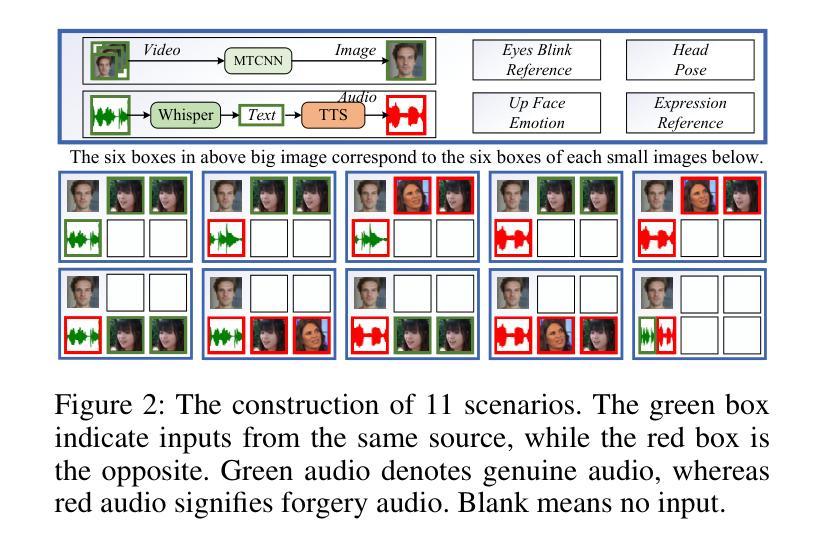
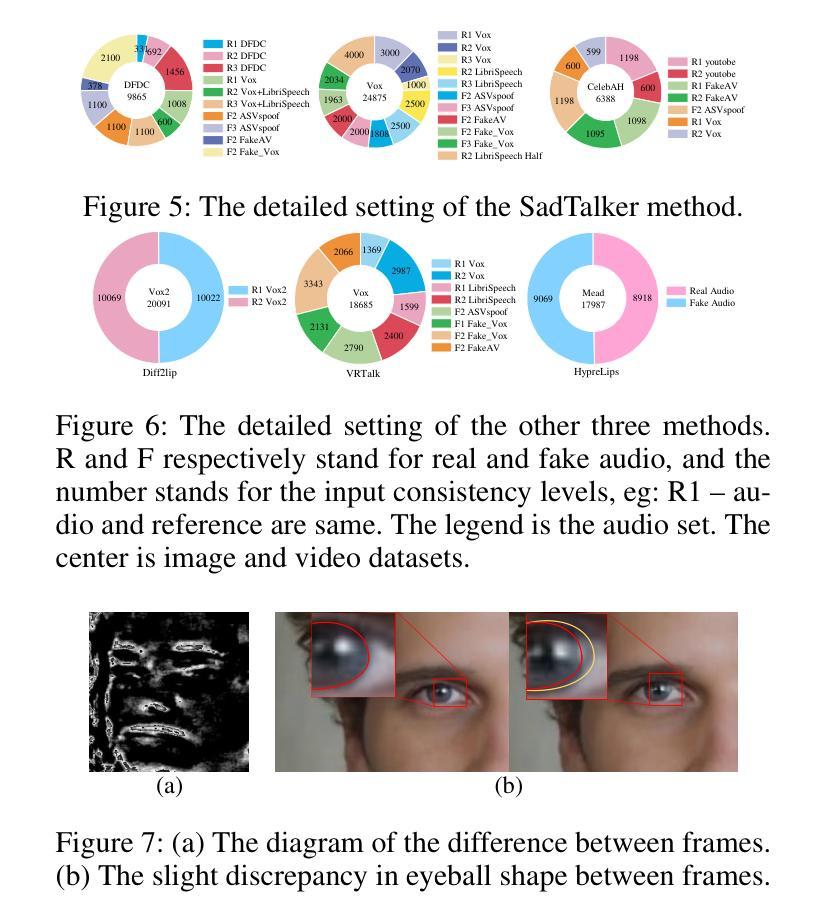
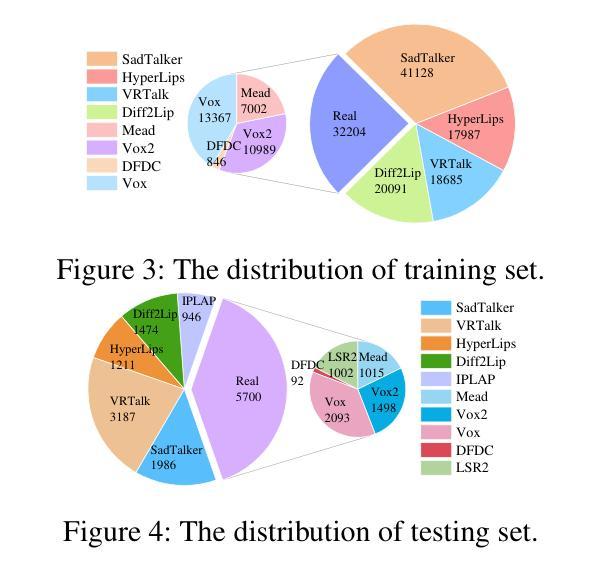
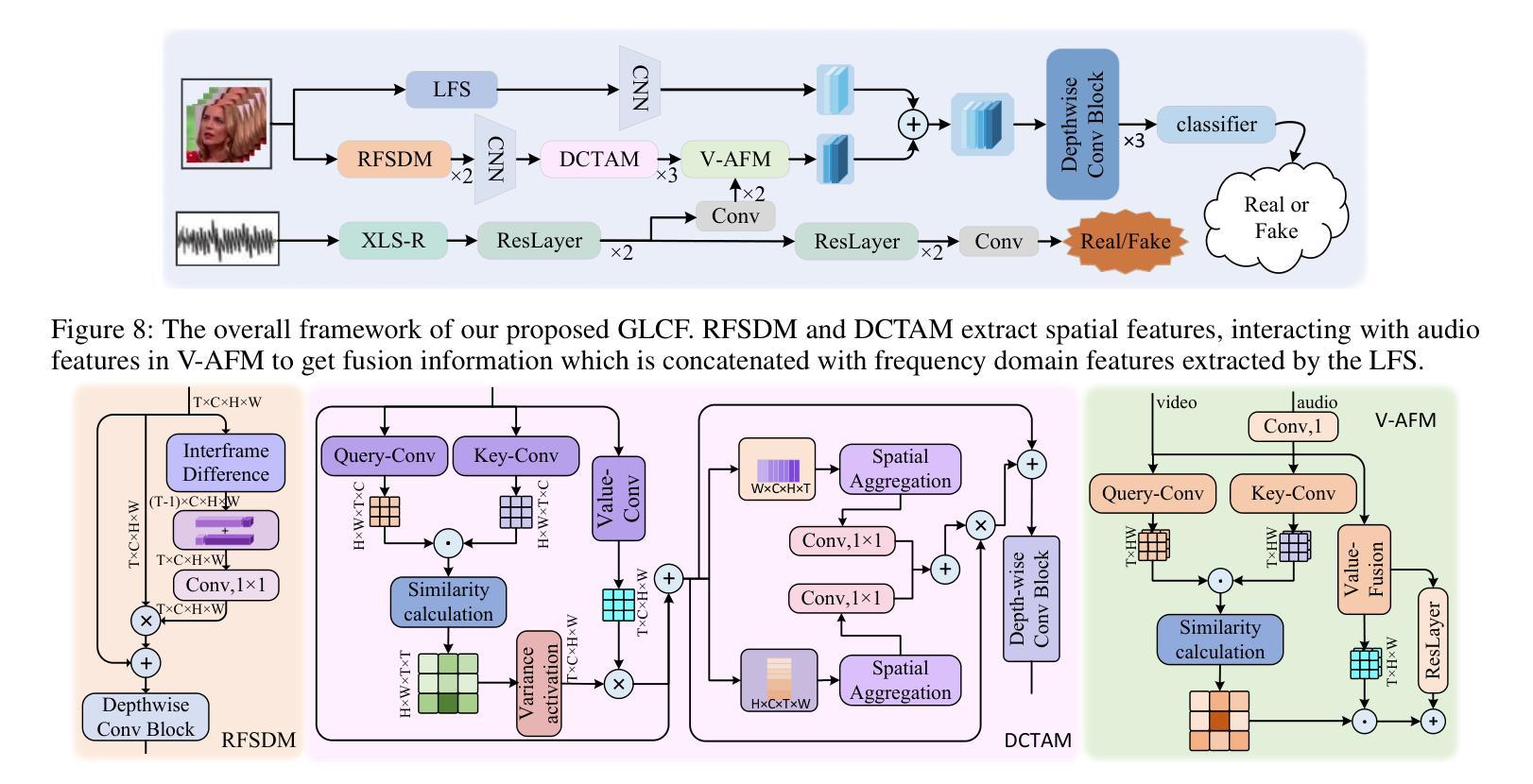
VQTalker: Towards Multilingual Talking Avatars through Facial Motion Tokenization
Authors:Tao Liu, Ziyang Ma, Qi Chen, Feilong Chen, Shuai Fan, Xie Chen, Kai Yu
We present VQTalker, a Vector Quantization-based framework for multilingual talking head generation that addresses the challenges of lip synchronization and natural motion across diverse languages. Our approach is grounded in the phonetic principle that human speech comprises a finite set of distinct sound units (phonemes) and corresponding visual articulations (visemes), which often share commonalities across languages. We introduce a facial motion tokenizer based on Group Residual Finite Scalar Quantization (GRFSQ), which creates a discretized representation of facial features. This method enables comprehensive capture of facial movements while improving generalization to multiple languages, even with limited training data. Building on this quantized representation, we implement a coarse-to-fine motion generation process that progressively refines facial animations. Extensive experiments demonstrate that VQTalker achieves state-of-the-art performance in both video-driven and speech-driven scenarios, particularly in multilingual settings. Notably, our method achieves high-quality results at a resolution of 512*512 pixels while maintaining a lower bitrate of approximately 11 kbps. Our work opens new possibilities for cross-lingual talking face generation. Synthetic results can be viewed at https://x-lance.github.io/VQTalker.
我们推出了VQTalker,这是一个基于向量量化的多语种谈话头生成框架,解决了跨不同语言的嘴唇同步和自然动作挑战。我们的方法基于语音学原理,即人类语音由一组有限的不同声音单元(音素)和相应的视觉发音(可见语音)组成,这些通常在各种语言中都有共性。我们引入了一种基于群组残差有限标量量化(GRFSQ)的面部运动标记器,它创建了一种面部特征的离散表示。这种方法能够全面捕捉面部动作,同时提高了对多种语言的泛化能力,即使训练数据有限。基于这种量化表示,我们实现了一种从粗糙到精细的运动生成过程,逐步优化面部动画。大量实验表明,VQTalker在视频驱动和语音驱动场景中均达到最新技术水平,特别是在多语种环境中。值得注意的是,我们的方法在512*512像素的分辨率下达到高质量结果,同时保持约11kbps的较低比特率。我们的工作为跨语言对话面部生成提供了新的可能性。合成结果可在https://x-lance.github.io/VQTalker查看。
论文及项目相关链接
PDF 14 pages
Summary
VQTalker基于向量量化的多语言说话人头生成框架,解决不同语言间的唇同步和自然动作挑战。此框架基于语音学原理,即人类语音由有限数量的独特声音单元(音素)和相应的视觉表达(面动单元)组成,这些单元在不同语言中常有共性。研究团队引入基于组残差有限标量量化的面部运动标记器(GRFSQ),创建面部特征的离散表示,能全面捕捉面部动作,提高多语言场景下的泛化能力,即使训练数据有限也能实现良好表现。通过此量化表示建立动画粗到细的过程逐步精细调整面部动画。实验证明,VQTalker在视频驱动和语音驱动场景中均达到业界领先水平,尤其适用于多语言环境。值得注意的是,此方法在达到高质量效果的同时维持较低的码率(约每秒上传比特数为千分之十一)。本研究为跨语言说话头生成开辟了新途径。相关成果可访问链接 https://x-lance.github.io/VQTalker 查看。
Key Takeaways
- VQTalker是基于向量量化的多语言说话人头生成框架。
- 它利用语音学原理处理人类语音与视觉表达之间的关系。
- GRFSQ技术用于创建面部特征的离散表示,促进面部动作的全面捕捉。
- 此方法提高在多语言环境下的泛化能力,即使训练数据有限也能良好运作。
- VQTalker在视频和语音驱动场景中表现卓越,特别是在多语言场景中。
- 此技术能够在保持高质量效果的同时维持较低的码率。
点此查看论文截图
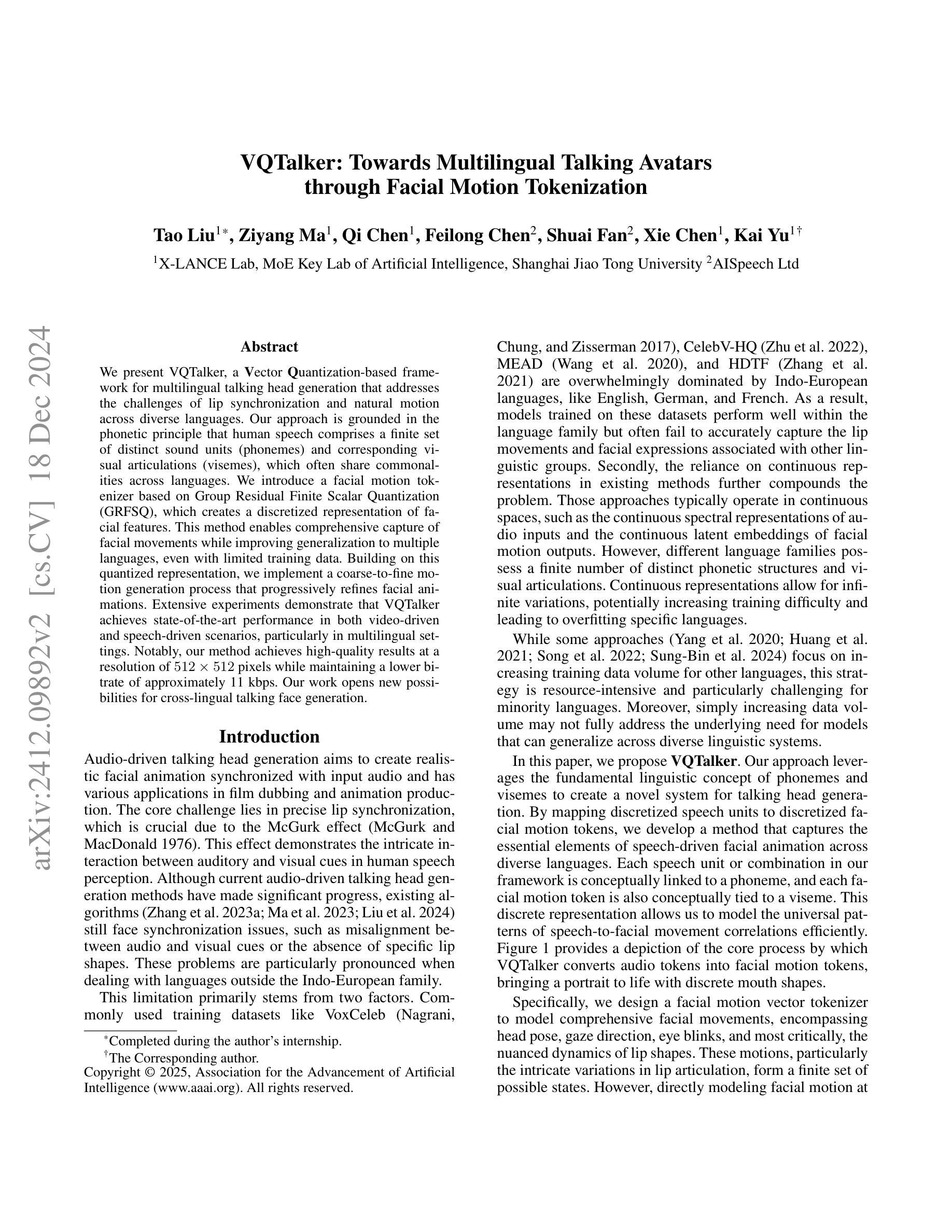
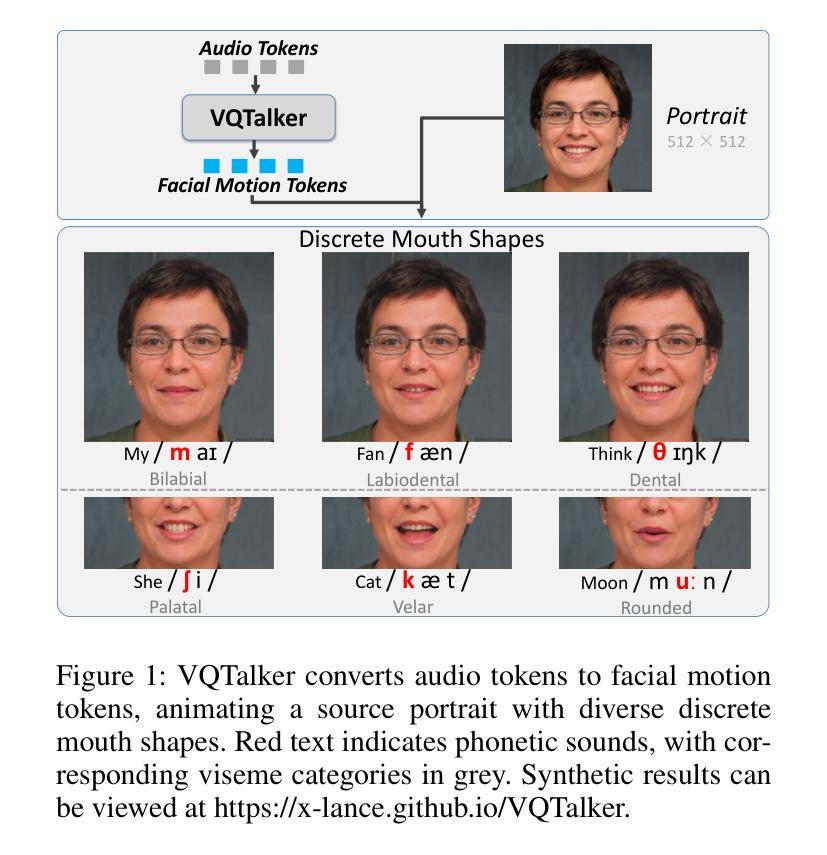
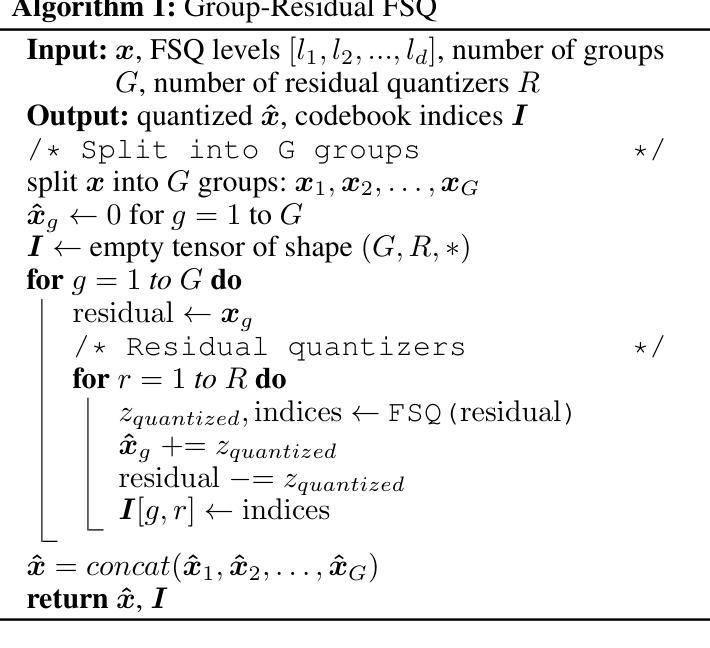
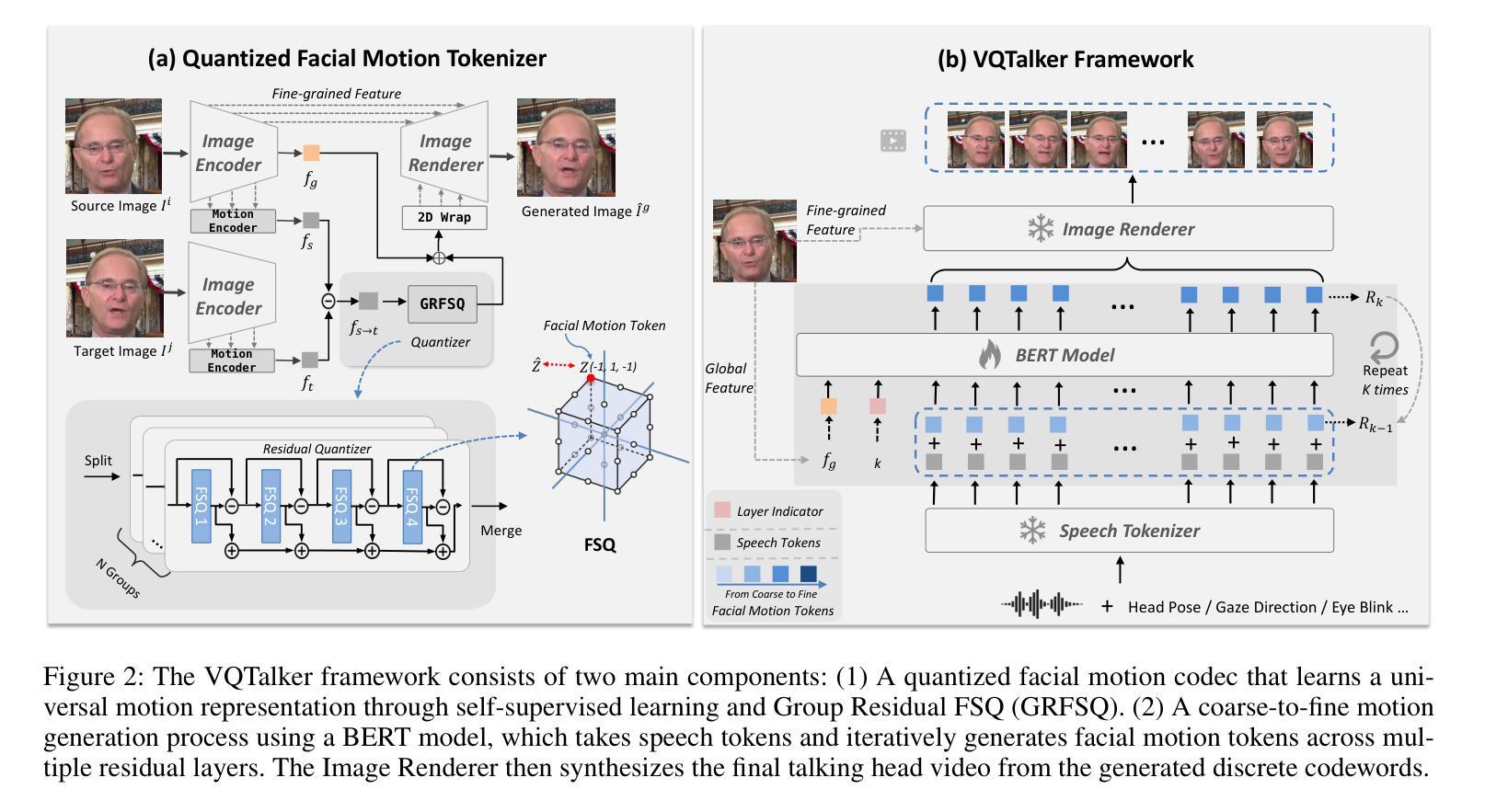
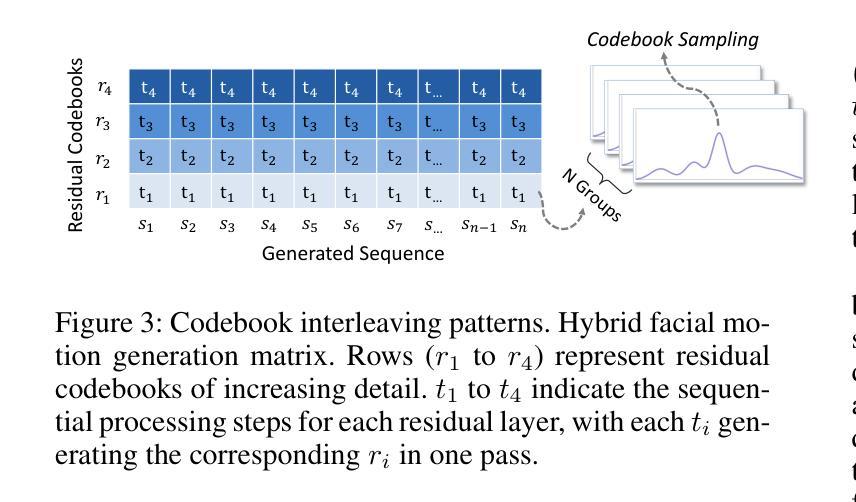
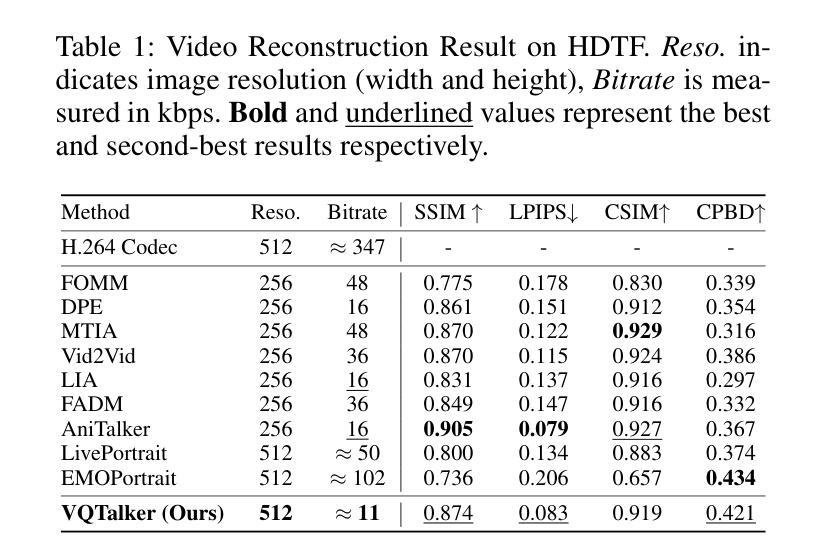
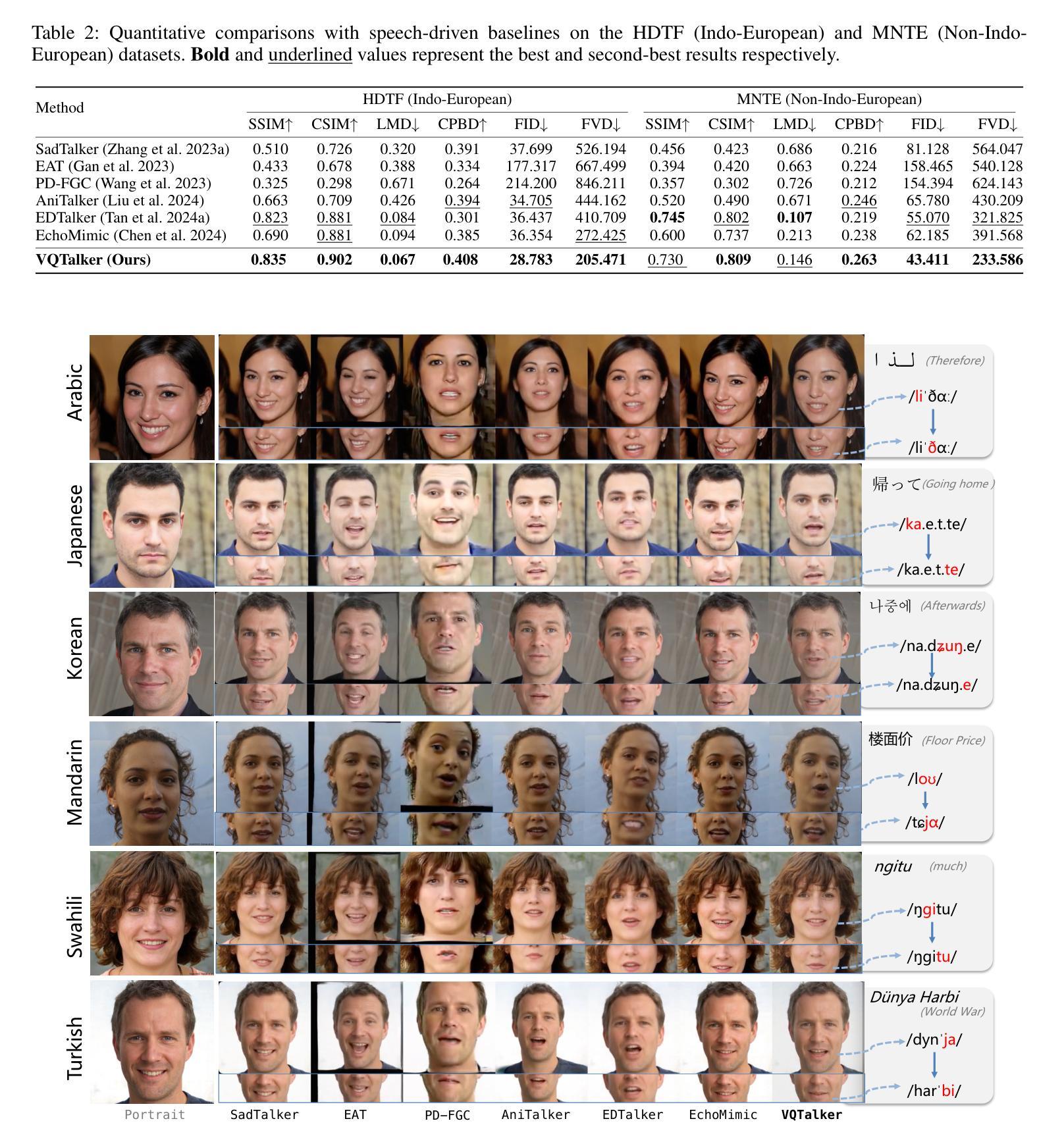
PointTalk: Audio-Driven Dynamic Lip Point Cloud for 3D Gaussian-based Talking Head Synthesis
Authors:Yifan Xie, Tao Feng, Xin Zhang, Xiangyang Luo, Zixuan Guo, Weijiang Yu, Heng Chang, Fei Ma, Fei Richard Yu
Talking head synthesis with arbitrary speech audio is a crucial challenge in the field of digital humans. Recently, methods based on radiance fields have received increasing attention due to their ability to synthesize high-fidelity and identity-consistent talking heads from just a few minutes of training video. However, due to the limited scale of the training data, these methods often exhibit poor performance in audio-lip synchronization and visual quality. In this paper, we propose a novel 3D Gaussian-based method called PointTalk, which constructs a static 3D Gaussian field of the head and deforms it in sync with the audio. It also incorporates an audio-driven dynamic lip point cloud as a critical component of the conditional information, thereby facilitating the effective synthesis of talking heads. Specifically, the initial step involves generating the corresponding lip point cloud from the audio signal and capturing its topological structure. The design of the dynamic difference encoder aims to capture the subtle nuances inherent in dynamic lip movements more effectively. Furthermore, we integrate the audio-point enhancement module, which not only ensures the synchronization of the audio signal with the corresponding lip point cloud within the feature space, but also facilitates a deeper understanding of the interrelations among cross-modal conditional features. Extensive experiments demonstrate that our method achieves superior high-fidelity and audio-lip synchronization in talking head synthesis compared to previous methods.
头部说话人合成与任意语音音频是数字人类领域的一个关键挑战。最近,基于辐射场的方法由于其仅从几分钟的训练视频就能合成高保真和身份一致的说话人头部的能力而备受关注。然而,由于训练数据的规模有限,这些方法在音频-唇部同步和视觉质量方面往往表现不佳。在本文中,我们提出了一种基于3D高斯的新型方法PointTalk,该方法构建了静态的3D高斯头部场,并与其音频同步进行变形。它还纳入了音频驱动的动态唇部点云作为条件信息的重要组成部分,从而促进了有效合成说话人的头部。具体来说,最初的步骤是从音频信号生成相应的唇部点云并捕获其拓扑结构。动态差异编码器的设计旨在更有效地捕捉动态唇部运动中所固有的细微差别。此外,我们集成了音频点增强模块,这不仅确保了音频信号与特征空间内相应唇部点云的同步,还促进了跨模态条件特征之间关系的深入理解。大量实验表明,我们的方法在说话人头部合成方面实现了高保真和优越的音频-唇部同步,相较于之前的方法具有显著优势。
论文及项目相关链接
PDF 9 pages, accepted by AAAI 2025
Summary
基于辐射场的方法在数字人类合成说话头部时面临音频唇同步及视觉质量方面的挑战。本文提出了一种新颖的基于3D高斯场的PointTalk方法,构建静态头部3D高斯场并根据音频进行变形。通过引入音频驱动的动态唇部点云作为条件信息的关键部分,实现了高保真和音频唇同步的说话头部合成。
Key Takeaways
- 说话头合成是一项挑战,特别是与任意语音音频的合成。
- 基于辐射场的方法能合成高保真和身份一致的说话头部,但面临音频唇同步和视觉质量的挑战。
- 提出的PointTalk方法使用3D高斯场构建头部模型,并依音频进行变形。
- 动态唇部点云作为关键条件信息,增强了唇部运动的细微表现。
- 设计动态差异编码器以更有效地捕捉动态唇部运动的细微变化。
- 引入音频点增强模块,确保音频信号与唇部点云的同步,并深化跨模态条件特征之间的关联理解。
点此查看论文截图
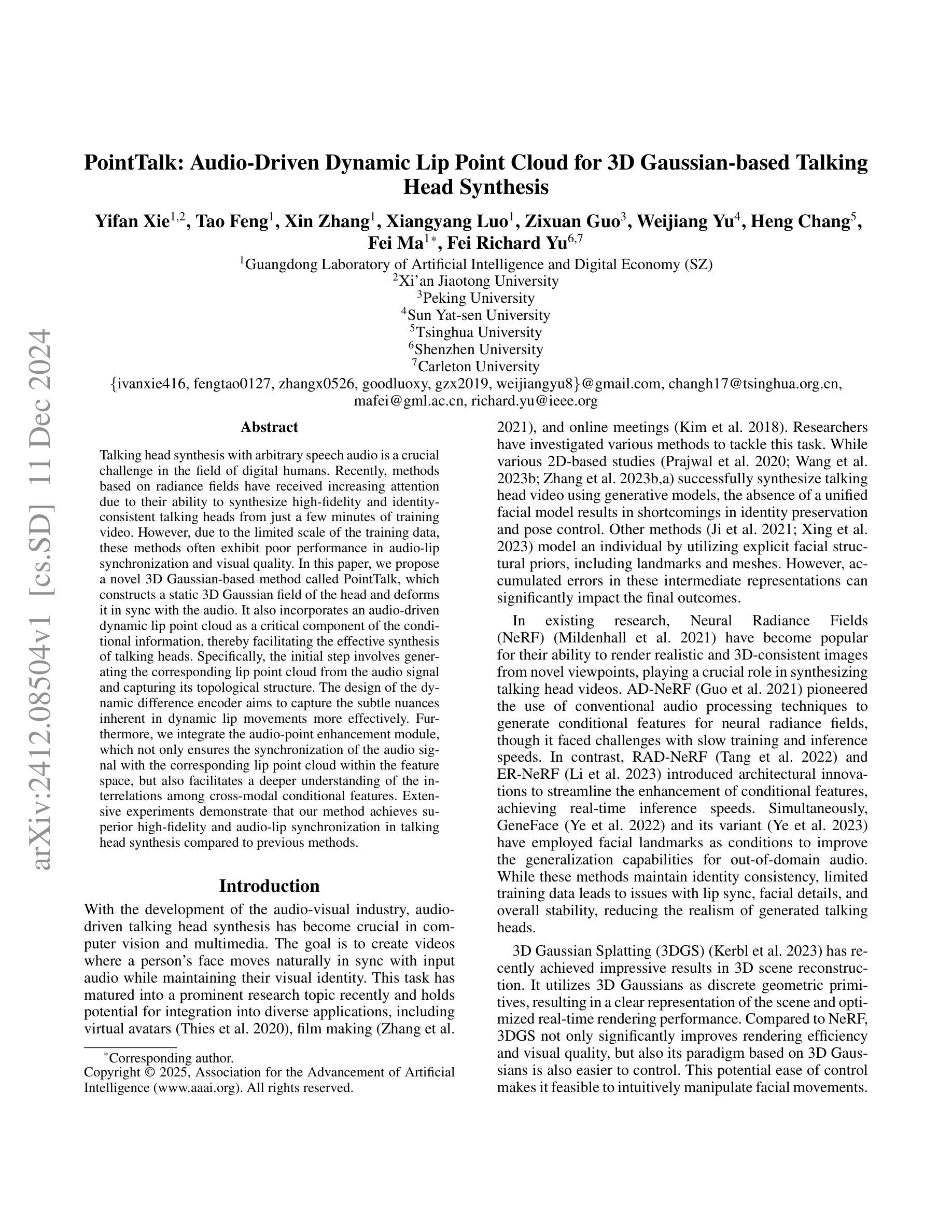
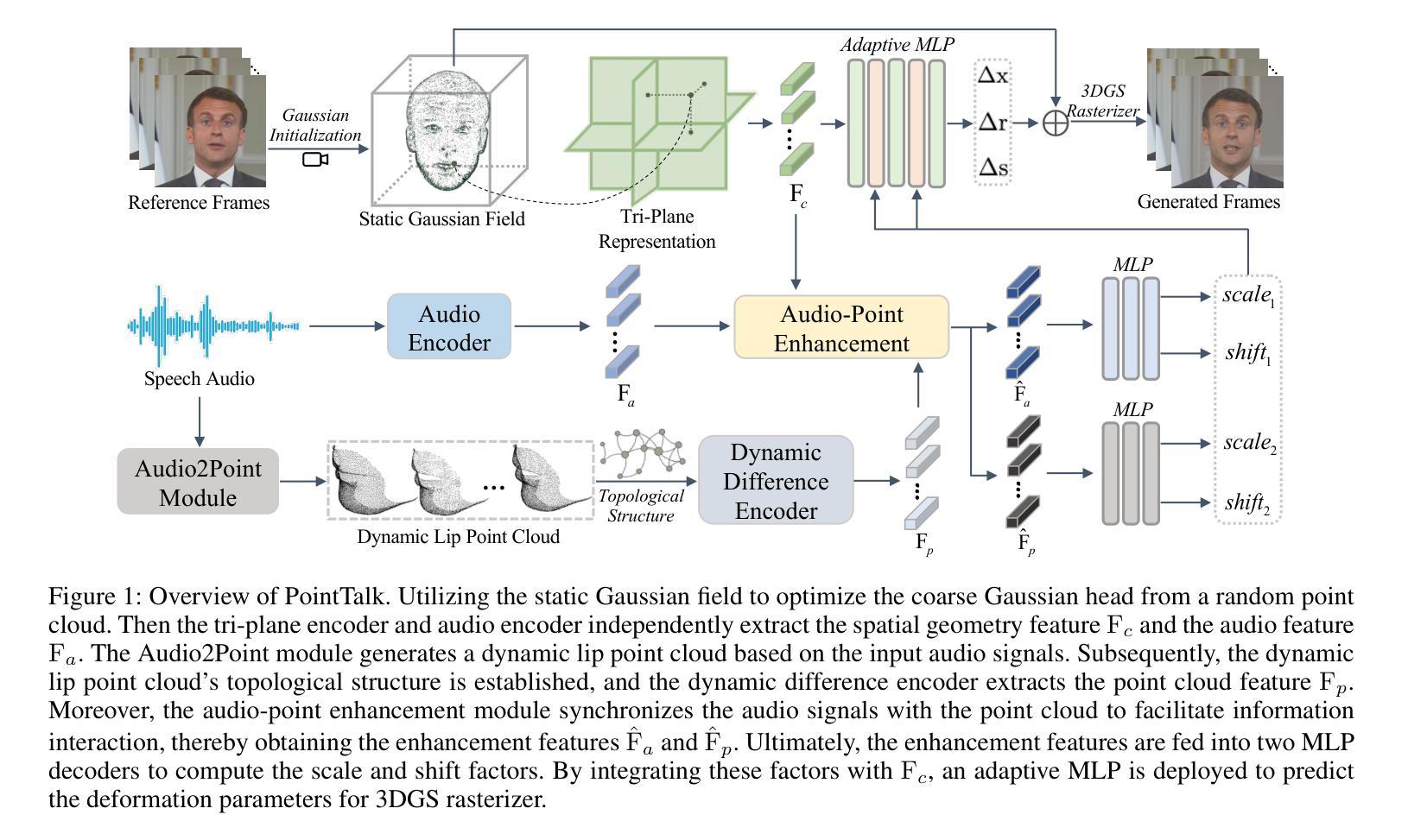
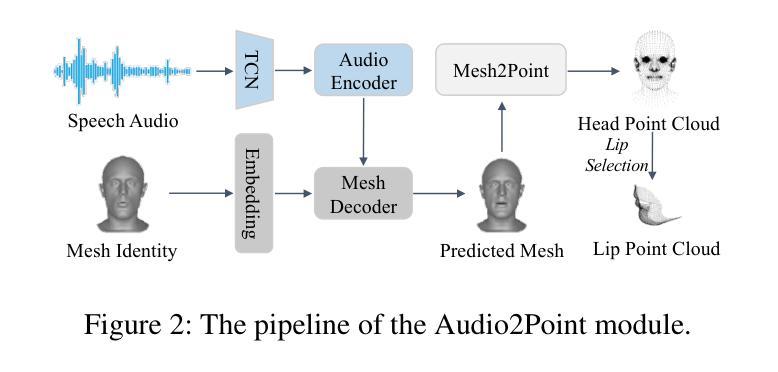
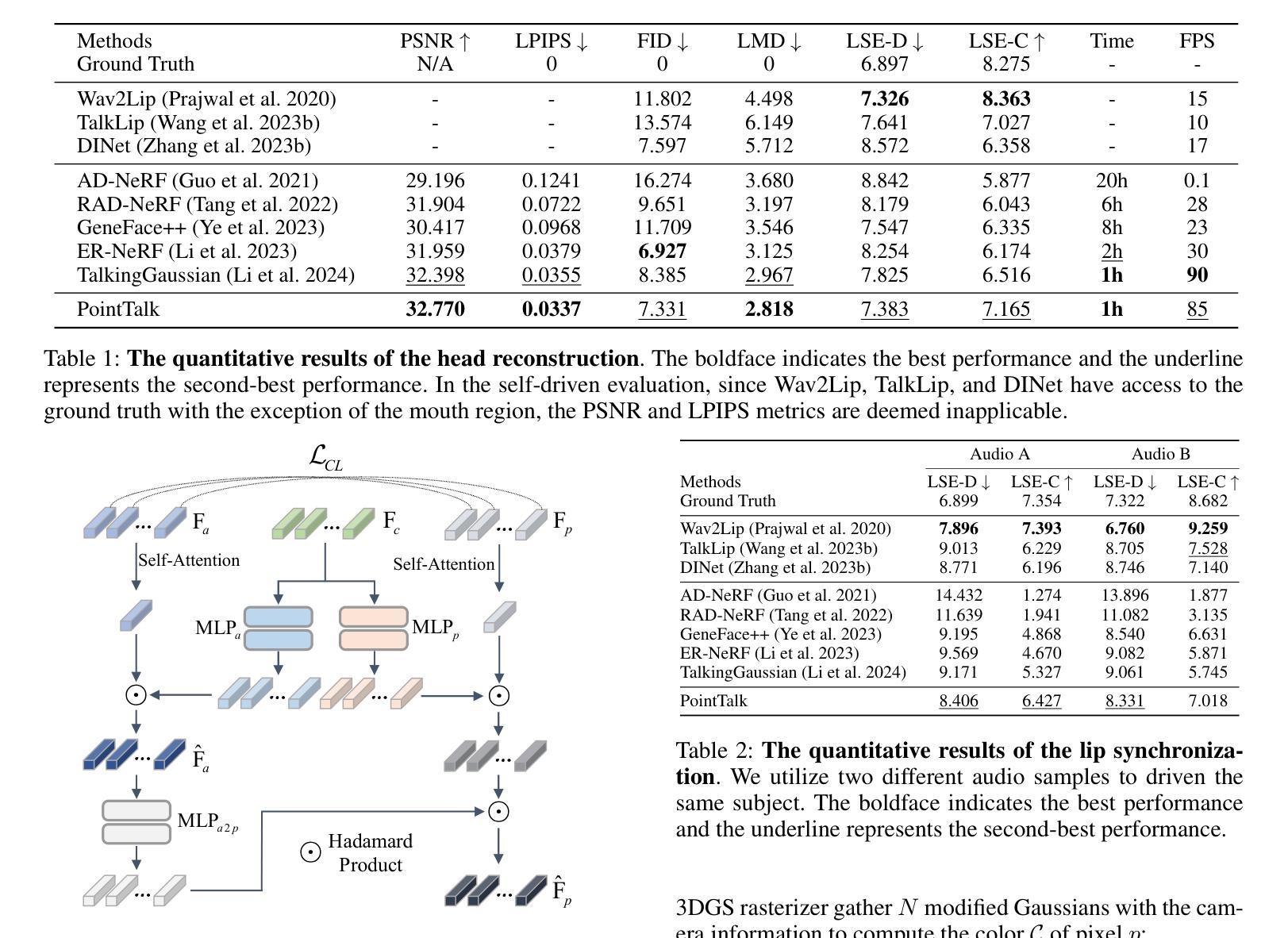
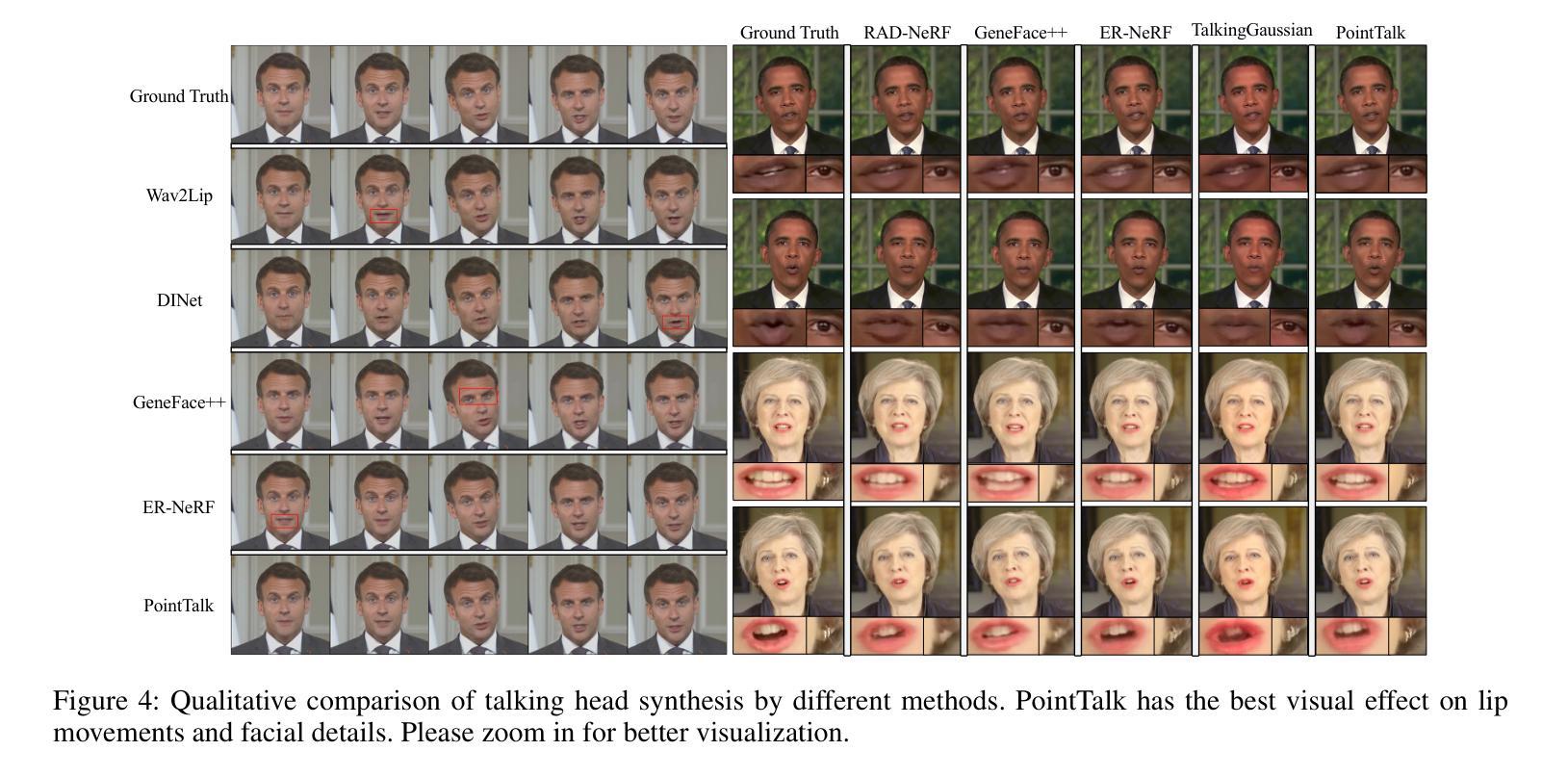
COSMo: CLIP Talks on Open-Set Multi-Target Domain Adaptation
Authors:Munish Monga, Sachin Kumar Giroh, Ankit Jha, Mainak Singha, Biplab Banerjee, Jocelyn Chanussot
Multi-Target Domain Adaptation (MTDA) entails learning domain-invariant information from a single source domain and applying it to multiple unlabeled target domains. Yet, existing MTDA methods predominantly focus on addressing domain shifts within visual features, often overlooking semantic features and struggling to handle unknown classes, resulting in what is known as Open-Set (OS) MTDA. While large-scale vision-language foundation models like CLIP show promise, their potential for MTDA remains largely unexplored. This paper introduces COSMo, a novel method that learns domain-agnostic prompts through source domain-guided prompt learning to tackle the MTDA problem in the prompt space. By leveraging a domain-specific bias network and separate prompts for known and unknown classes, COSMo effectively adapts across domain and class shifts. To the best of our knowledge, COSMo is the first method to address Open-Set Multi-Target DA (OSMTDA), offering a more realistic representation of real-world scenarios and addressing the challenges of both open-set and multi-target DA. COSMo demonstrates an average improvement of $5.1%$ across three challenging datasets: Mini-DomainNet, Office-31, and Office-Home, compared to other related DA methods adapted to operate within the OSMTDA setting. Code is available at: https://github.com/munish30monga/COSMo
多目标域适应(MTDA)涉及从单个源域学习域不变信息,并将其应用于多个无标签的目标域。然而,现有的MTDA方法主要集中在解决视觉特征中的域偏移问题,往往忽略了语义特征,并且在处理未知类别时遇到困难,这就导致了被称为开放集(OS)MTDA的情况。虽然像CLIP这样的大规模视觉语言基础模型显示出了一定的潜力,但它们在MTDA方面的潜力仍待探索。本文介绍了一种新的方法COSMo,它通过源域引导提示学习,学习域无关的提示来解决提示空间中的MTDA问题。通过利用域特定偏置网络和为已知和未知类别分别设置的提示,COSMo可以有效地适应域和类别的变化。据我们所知,COSMo是第一个解决开放集多目标DA(OSMTDA)的方法,它提供了现实世界场景的更现实表示,并解决了开放集和多目标DA的挑战。在Mini-DomainNet、Office-31和Office-Home三个具有挑战性的数据集上,COSMo与其他适应OSMTDA设置的DA方法相比,平均改进了5.1%。代码可用在:https://github.com/munish30monga/COSMo
论文及项目相关链接
PDF Accepted in BMVC 2024
Summary
多目标域自适应(MTDA)方法主要关注视觉特征的域迁移问题,却忽略了语义特征,并且在处理未知类别时表现不佳。针对此问题,本文提出了名为COSMo的新方法,通过源域指导的提示学习来解决MTDA问题。COSMo利用域特定偏差网络和已知与未知类别的独立提示,有效适应跨域和类别转移。它是首个解决开放集多目标域自适应(OSMTDA)的方法,更真实地反映了现实世界的场景,并解决了开放集和多目标域适应的挑战。在三个具有挑战性的数据集上,COSMo与其他相关DA方法相比,在OSMTDA设置下的平均改进率为5.1%。
Key Takeaways
- MTDA主要关注视觉特征的域迁移,但忽略了语义特征。
- 处理未知类别时,现有MTDA方法表现不佳。
- COSMo是一种新的MTDA解决方法,通过源域指导的提示学习来适应跨域和类别转移。
- COSMo是首个解决开放集多目标域自适应(OSMTDA)的方法。
- COSMo在三个具有挑战性的数据集上的表现优于其他相关DA方法,平均改进率为5.1%。
- COSMo利用域特定偏差网络来处理域迁移问题。
点此查看论文截图
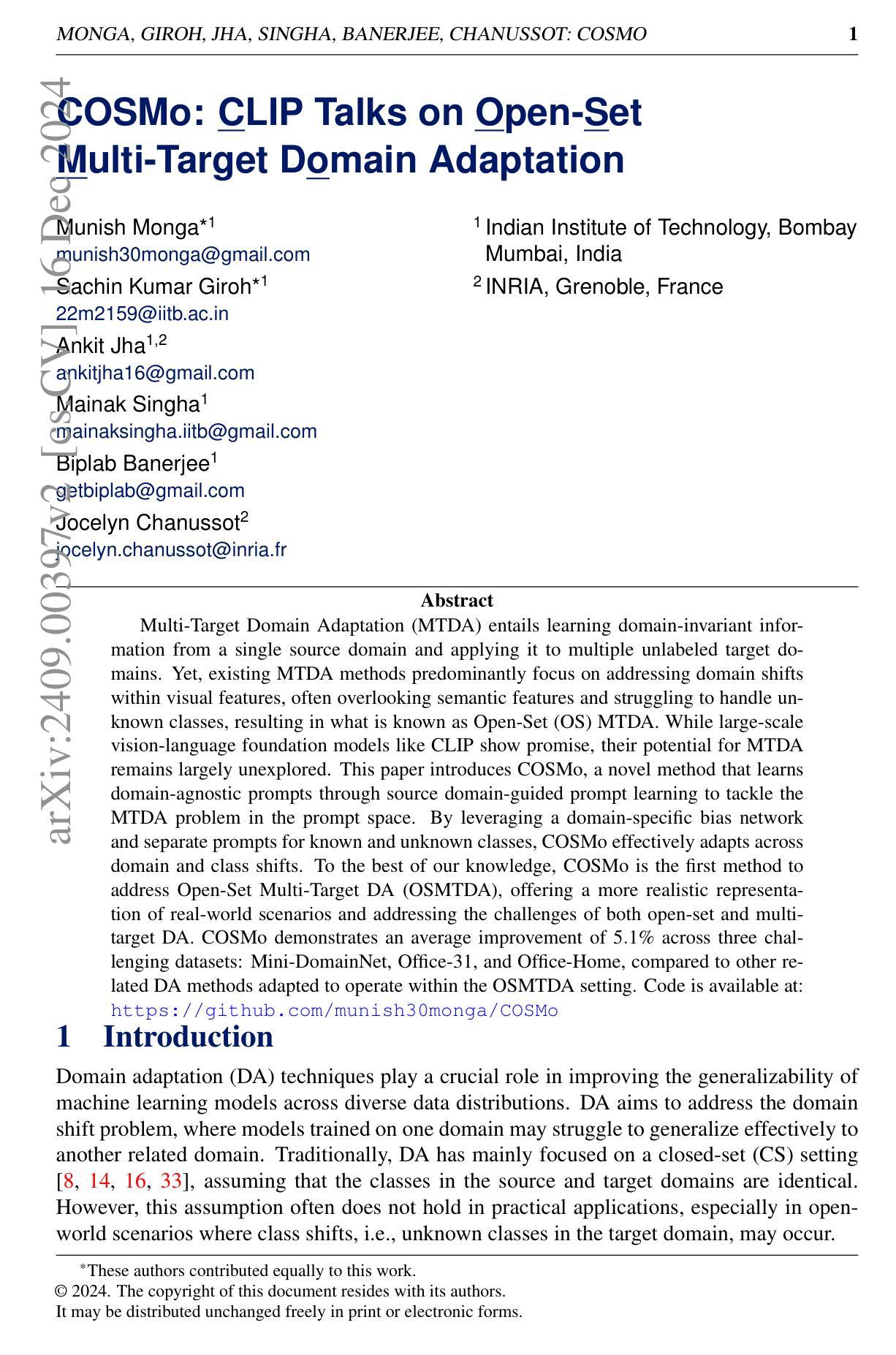
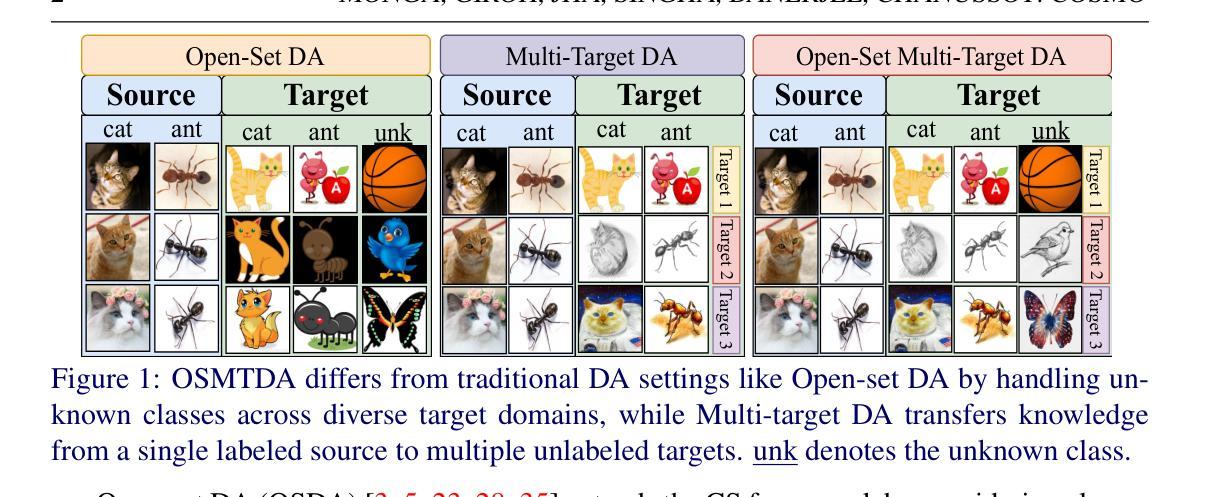
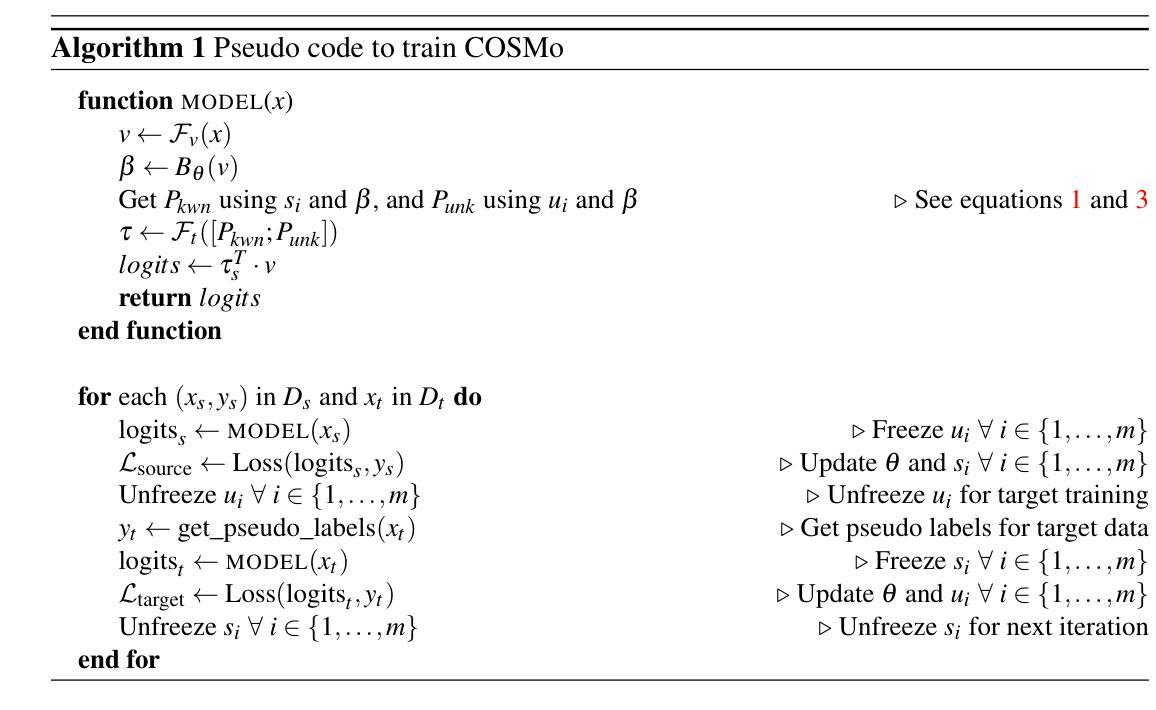