⚠️ 以下所有内容总结都来自于 大语言模型的能力,如有错误,仅供参考,谨慎使用
🔴 请注意:千万不要用于严肃的学术场景,只能用于论文阅读前的初筛!
💗 如果您觉得我们的项目对您有帮助 ChatPaperFree ,还请您给我们一些鼓励!⭐️ HuggingFace免费体验
2024-12-19 更新
Light-T2M: A Lightweight and Fast Model for Text-to-motion Generation
Authors:Ling-An Zeng, Guohong Huang, Gaojie Wu, Wei-Shi Zheng
Despite the significant role text-to-motion (T2M) generation plays across various applications, current methods involve a large number of parameters and suffer from slow inference speeds, leading to high usage costs. To address this, we aim to design a lightweight model to reduce usage costs. First, unlike existing works that focus solely on global information modeling, we recognize the importance of local information modeling in the T2M task by reconsidering the intrinsic properties of human motion, leading us to propose a lightweight Local Information Modeling Module. Second, we introduce Mamba to the T2M task, reducing the number of parameters and GPU memory demands, and we have designed a novel Pseudo-bidirectional Scan to replicate the effects of a bidirectional scan without increasing parameter count. Moreover, we propose a novel Adaptive Textual Information Injector that more effectively integrates textual information into the motion during generation. By integrating the aforementioned designs, we propose a lightweight and fast model named Light-T2M. Compared to the state-of-the-art method, MoMask, our Light-T2M model features just 10% of the parameters (4.48M vs 44.85M) and achieves a 16% faster inference time (0.152s vs 0.180s), while surpassing MoMask with an FID of \textbf{0.040} (vs. 0.045) on HumanML3D dataset and 0.161 (vs. 0.228) on KIT-ML dataset. The code is available at https://github.com/qinghuannn/light-t2m.
尽管文本到运动(T2M)生成在各种应用中都扮演着重要角色,但当前的方法涉及大量的参数,并且推理速度慢,导致使用成本高。为了解决这个问题,我们旨在设计一个轻量级的模型来降低使用成本。首先,与现有工作不同,这些工作只专注于全局信息建模,我们认识到局部信息建模在T2M任务中的重要性,通过重新考虑人类运动的内在属性,我们提出了一个轻量级的局部信息建模模块。其次,我们将Mamba引入T2M任务,减少了参数数量和GPU内存需求,并设计了一种新型伪双向扫描,以复制双向扫描的效果而不会增加参数计数。此外,我们提出了一种新型自适应文本信息注入器,更有效地将文本信息集成到生成的运动中。通过整合上述设计,我们提出了一个轻量级且快速的模型,名为Light-T2M。与最新方法MoMask相比,我们的Light-T2M模型仅使用其参数的十分之一(即仅使用了参数量为个位数的状态比非常先进),而其FID分数则在HumanML3D数据集上为领先成绩低于或等于MoMask的FID分数为且推理速度提高了百分之十六(0.152秒对比于MoMask的0.18秒),证明了轻量级T2M模型的优势明显。,能够在较小的计算代价下满足不同的现实需求。我们的代码可以在https://github.com/qinghuannn/light-t2m中找到。
论文及项目相关链接
PDF Accepted to AAAI 2025
摘要
针对文本转运动(T2M)生成技术在各个应用中的重要性,当前方法存在参数多、推理速度慢、使用成本高等问题。为此,本文设计了一个轻量级模型以降低成本。我们认识到局部信息建模在T2M任务中的重要性,并引入了局部信息建模模块。同时,我们将Mamba引入T2M任务,减少参数和GPU内存需求,并提出伪双向扫描来模拟双向扫描的效果而不增加参数计数。此外,我们提出了一种新的自适应文本信息注入器,更有效地将文本信息集成到运动中。通过整合上述设计,我们提出了轻量级、快速的Light-T2M模型。与最新方法MoMask相比,我们的模型参数只有其10%(4.48M vs 44.85M),推理时间快16%(0.152s vs 0.180s),同时在HumanML3D和KIT-ML数据集上的FID得分表现更佳。
关键见解
- 提出设计轻量级模型以降低文本转运动(T2M)技术的高使用成本。
- 认识到局部信息建模在T2M任务中的重要性。
- 引入Mamba来减少T2M任务的参数和GPU内存需求。
- 提出伪双向扫描以模拟双向扫描效果,同时不增加参数计数。
- 引入自适应文本信息注入器,更有效地集成文本信息到运动中。
- 提出的Light-T2M模型与最新方法相比,参数大大减少,推理时间更快,且在多个数据集上的FID得分表现优越。
- 模型代码已公开可用。
点此查看论文截图
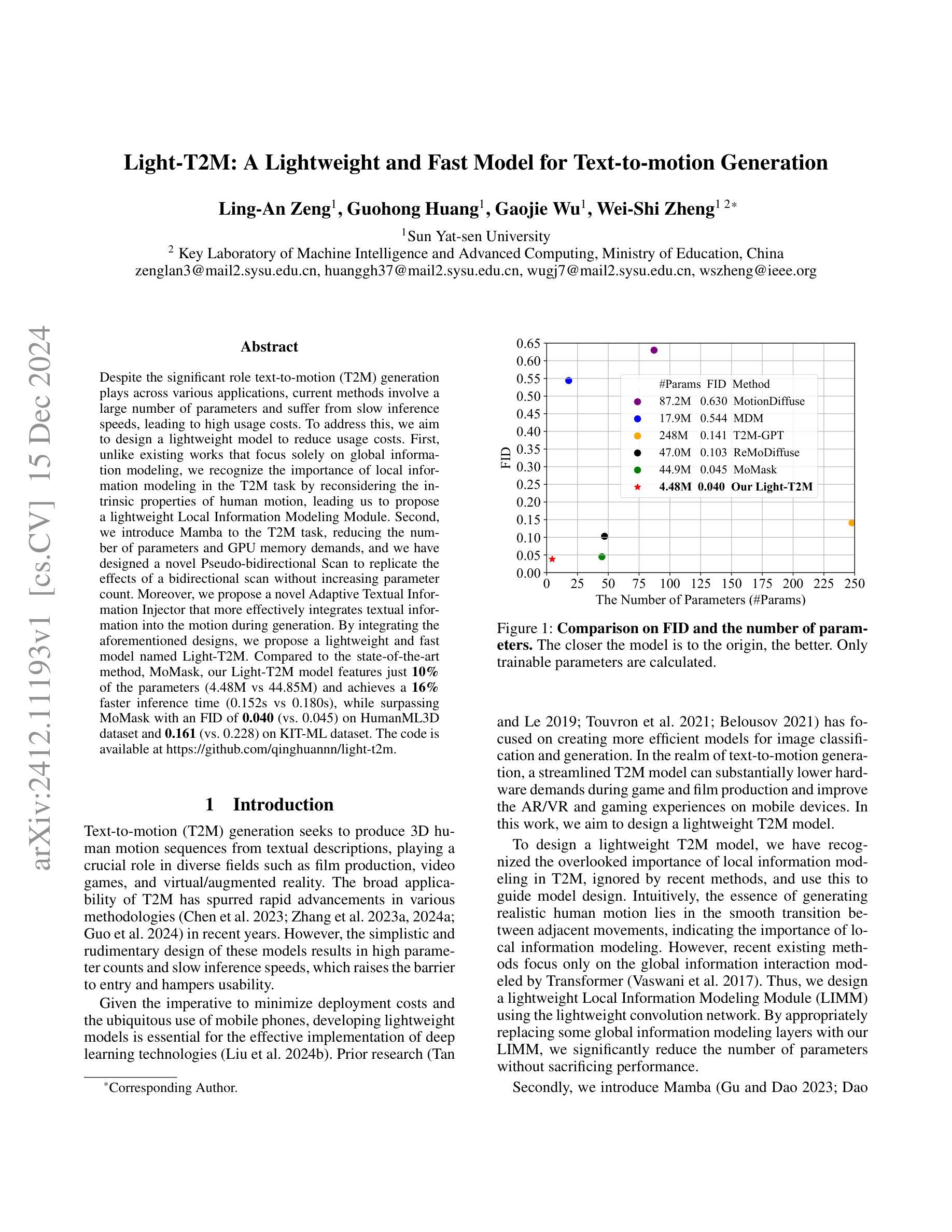
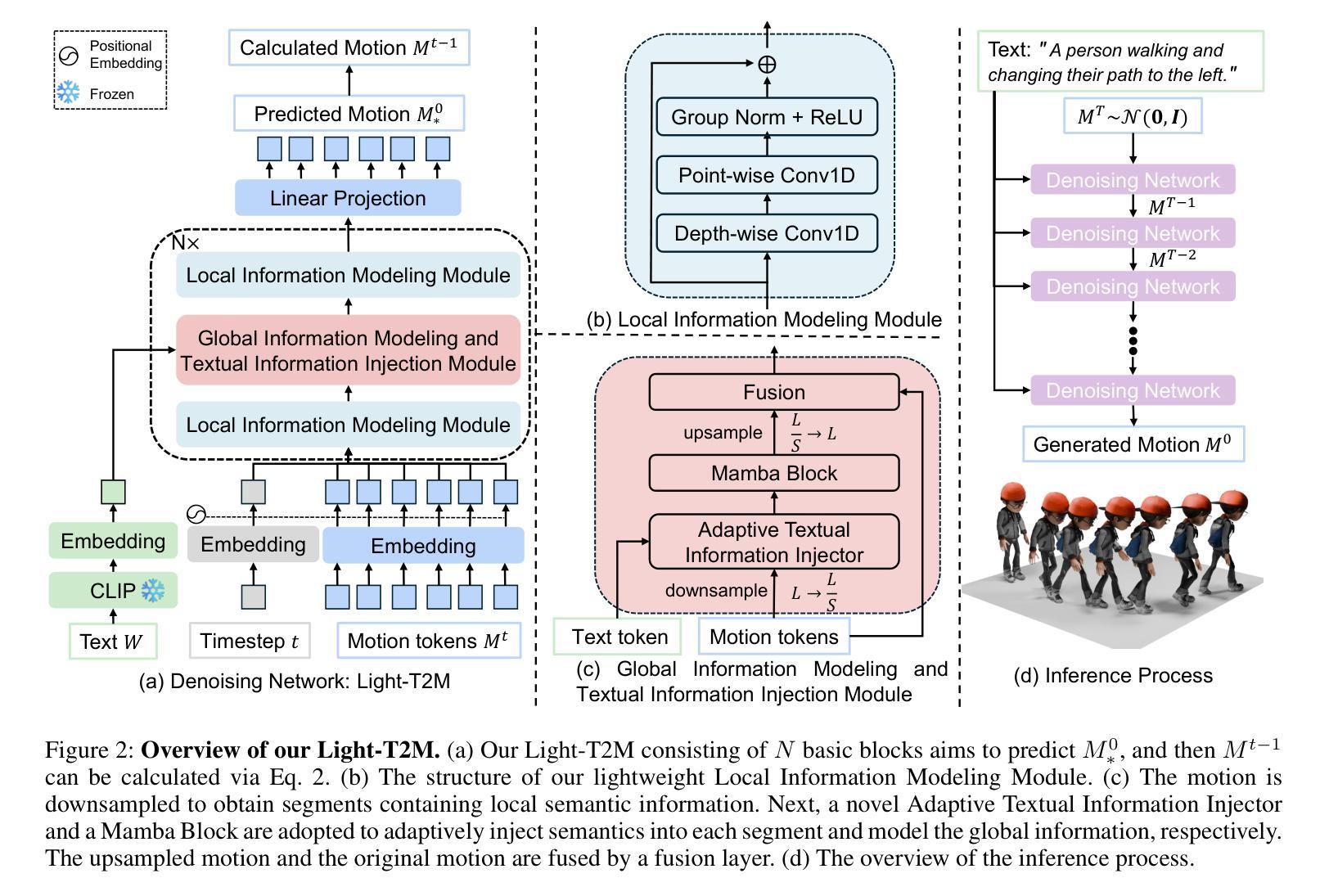
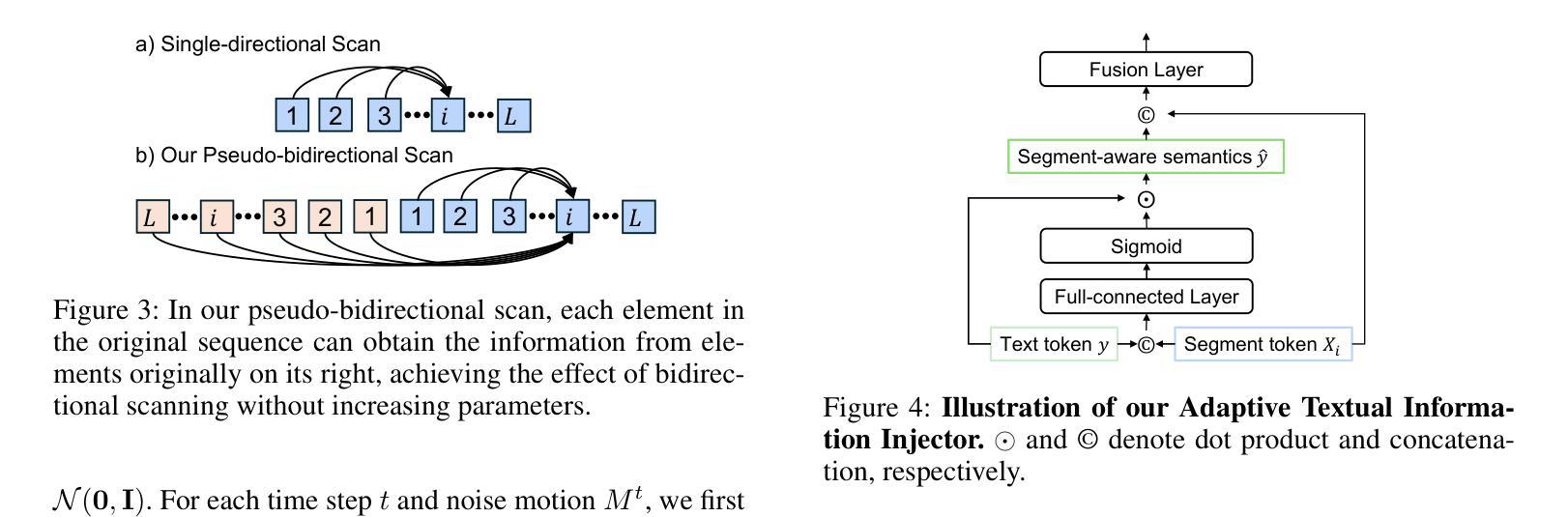
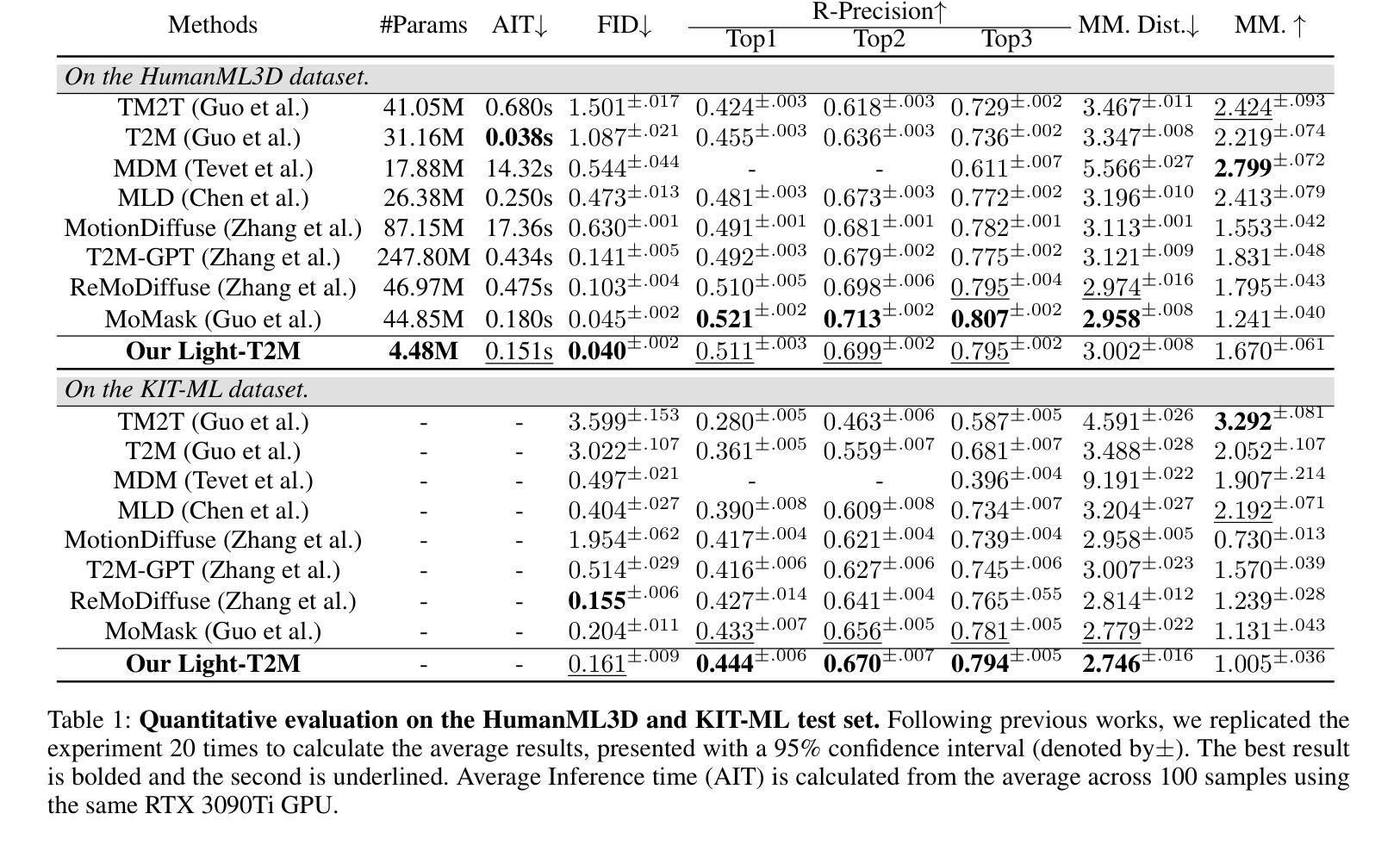
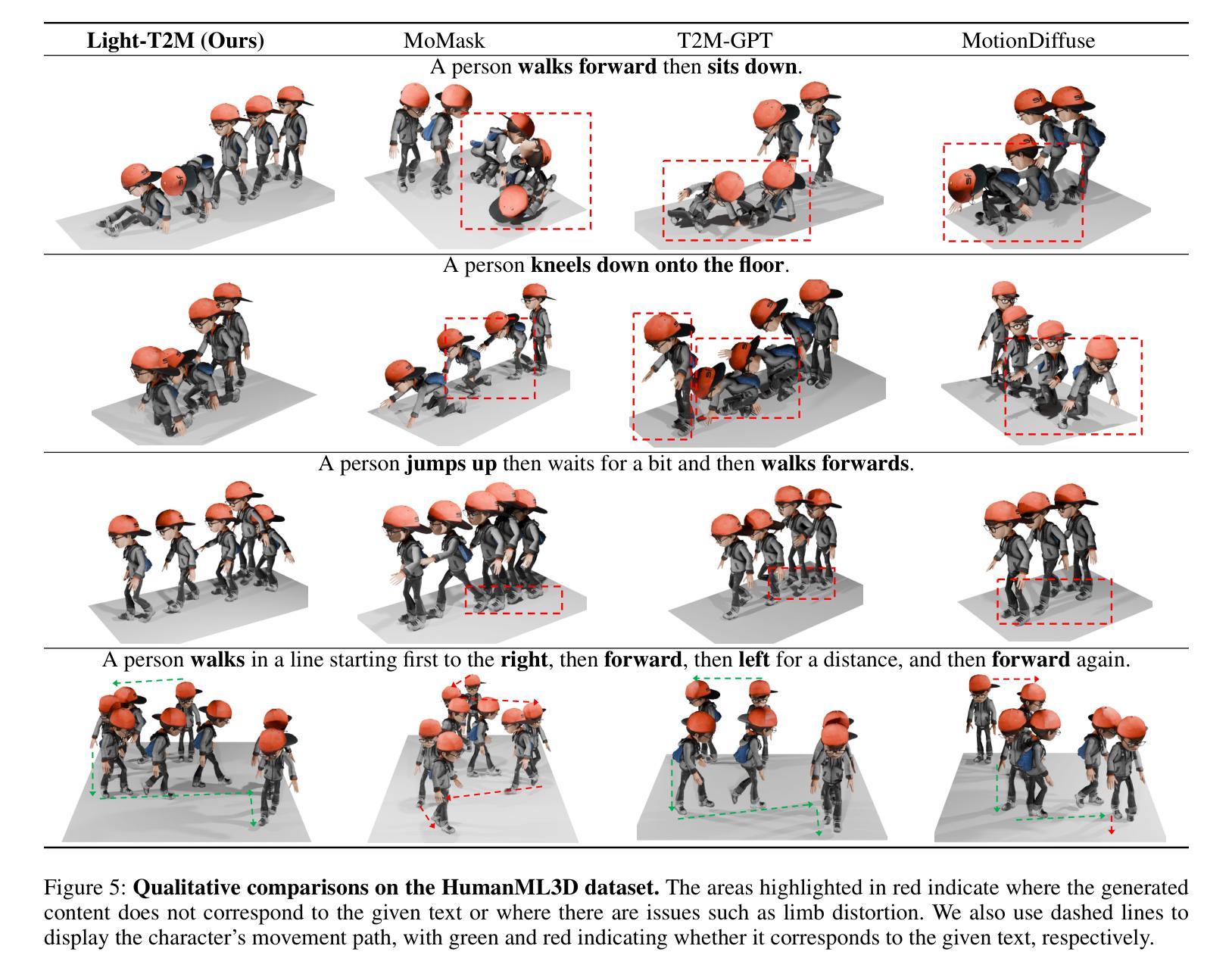