⚠️ 以下所有内容总结都来自于 大语言模型的能力,如有错误,仅供参考,谨慎使用
🔴 请注意:千万不要用于严肃的学术场景,只能用于论文阅读前的初筛!
💗 如果您觉得我们的项目对您有帮助 ChatPaperFree ,还请您给我们一些鼓励!⭐️ HuggingFace免费体验
2024-12-21 更新
Img-Diff: Contrastive Data Synthesis for Multimodal Large Language Models
Authors:Qirui Jiao, Daoyuan Chen, Yilun Huang, Bolin Ding, Yaliang Li, Ying Shen
High-performance Multimodal Large Language Models (MLLMs) are heavily dependent on data quality. To advance fine-grained image recognition within MLLMs, we introduce a novel data synthesis method inspired by contrastive learning and image difference captioning. Our key idea involves challenging the model to discern both matching and distinct elements by scrutinizing object differences in detailed regions across similar images. We begin by generating pairs of similar images that emphasize object variations. Following this, we employ a Difference Area Generator to pinpoint object differences, and subsequently, a Difference Captions Generator to articulate these differences. This process results in a high-quality dataset of “object replacement” samples, termed Img-Diff, which can be scaled as needed due to its automated nature. We leverage this generated dataset to fine-tune state-of-the-art (SOTA) MLLMs, such as InternVL2, achieving substantial improvements across various image difference and Visual Question Answering tasks. Notably, the trained models significantly outperform existing SOTA models like GPT-4V and Gemini on the MMVP benchmark. Additionally, we conduct comprehensive evaluations to validate the dataset’s diversity, quality, and robustness, offering several insights into the synthesis of such contrastive datasets. We release our codes and dataset to encourage further research on multimodal data synthesis and MLLMs’ fundamental capabilities for image understanding.
高性能多模态大型语言模型(MLLMs)严重依赖于数据质量。为了推进MLLMs中的精细图像识别,我们受到对比学习和图像差异字幕的启发,引入了一种新型数据合成方法。我们的核心思想是通过仔细研究相似图像中详细区域的对象差异,挑战模型来识别匹配和不同的元素。我们首先生成强调对象变化的相似图像对。接着,我们采用差异区域生成器来定位对象差异,然后采用差异字幕生成器来明确这些差异。这一过程产生了高质量的“对象替换”样本数据集,称为Img-Diff,由于其自动化特性,可以根据需要进行扩展。我们利用这个生成的数据集对最先进的MLLMs(如InternVL2)进行微调,在各种图像差异和视觉问答任务上取得了显著的提升。值得注意的是,经过训练的模型在MMVP基准测试上的表现显著优于现有的GPT-4V和Gemini等顶尖模型。此外,我们进行了全面的评估,验证了数据集的多样性、质量和稳健性,为这类对比数据集的合成提供了深入见解。我们公开了代码和数据集,以鼓励对多模态数据合成和MLLMs图像理解基本能力的进一步研究。
论文及项目相关链接
PDF 22 pages, 10 figures, 16 tables
Summary
本文介绍了一种基于对比学习和图像差异描述的新型数据合成方法,用于提高多模态大型语言模型在精细图像识别方面的性能。通过生成强调对象差异的图像对,并利用差异区域生成器和差异描述生成器,创建了一种高质量的“对象替换”样本数据集Img-Diff。使用此数据集对最先进的MLLMs进行微调,在各种图像差异和视觉问答任务上取得了显著改进。
Key Takeaways
- 引入了一种新型数据合成方法,结合对比学习和图像差异描述,旨在提高多模态大型语言模型在精细图像识别上的性能。
- 通过生成强调对象差异的图像对,增强了模型对匹配和差异元素的辨识能力。
- 利用差异区域生成器和差异描述生成器,创建了一种高质量的“对象替换”样本数据集Img-Diff。
- Img-Diff数据集可按需扩展,因其自动化性质。
- 使用Img-Diff数据集对最先进的MLLMs进行微调,实现了在各种图像差异和视觉问答任务上的显著改进。
- 训练的模型在MMVP基准测试上显著优于现有的最先进模型,如GPT-4V和Gemini。
点此查看论文截图
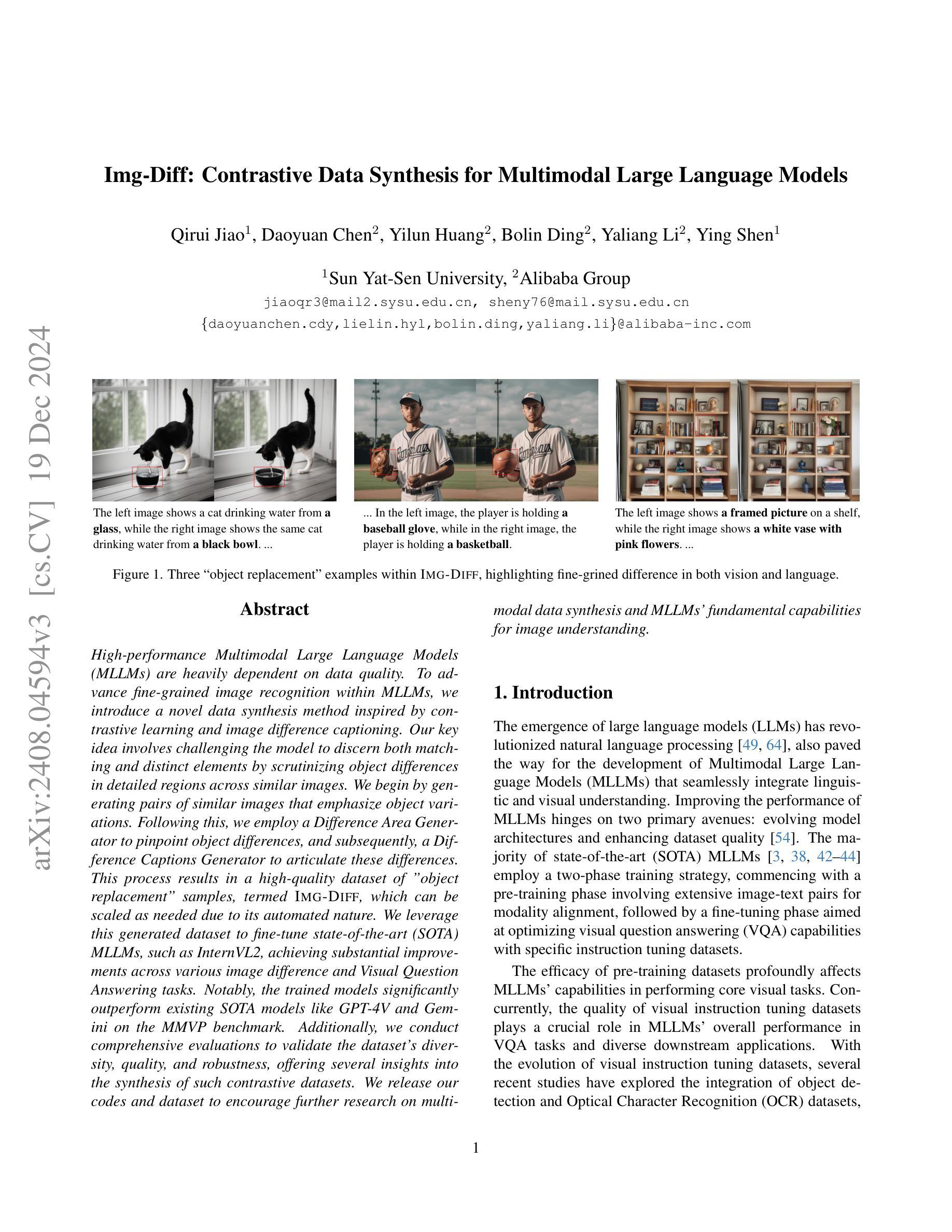
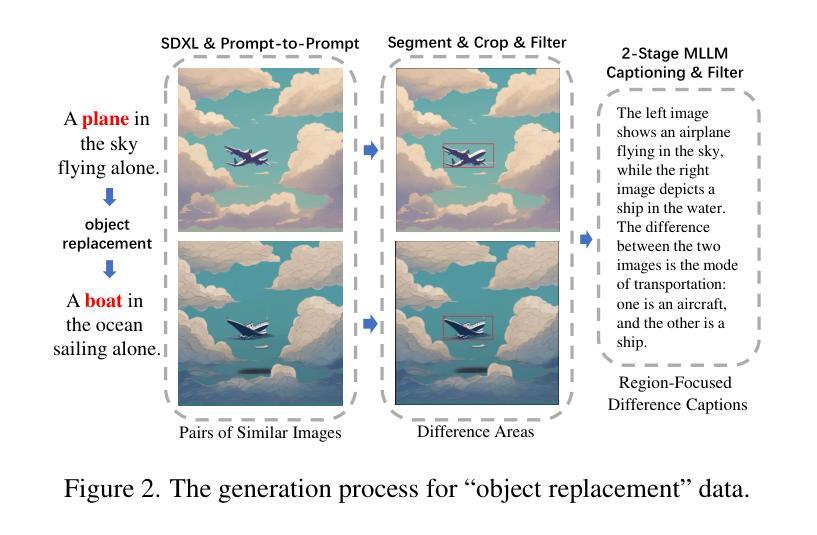
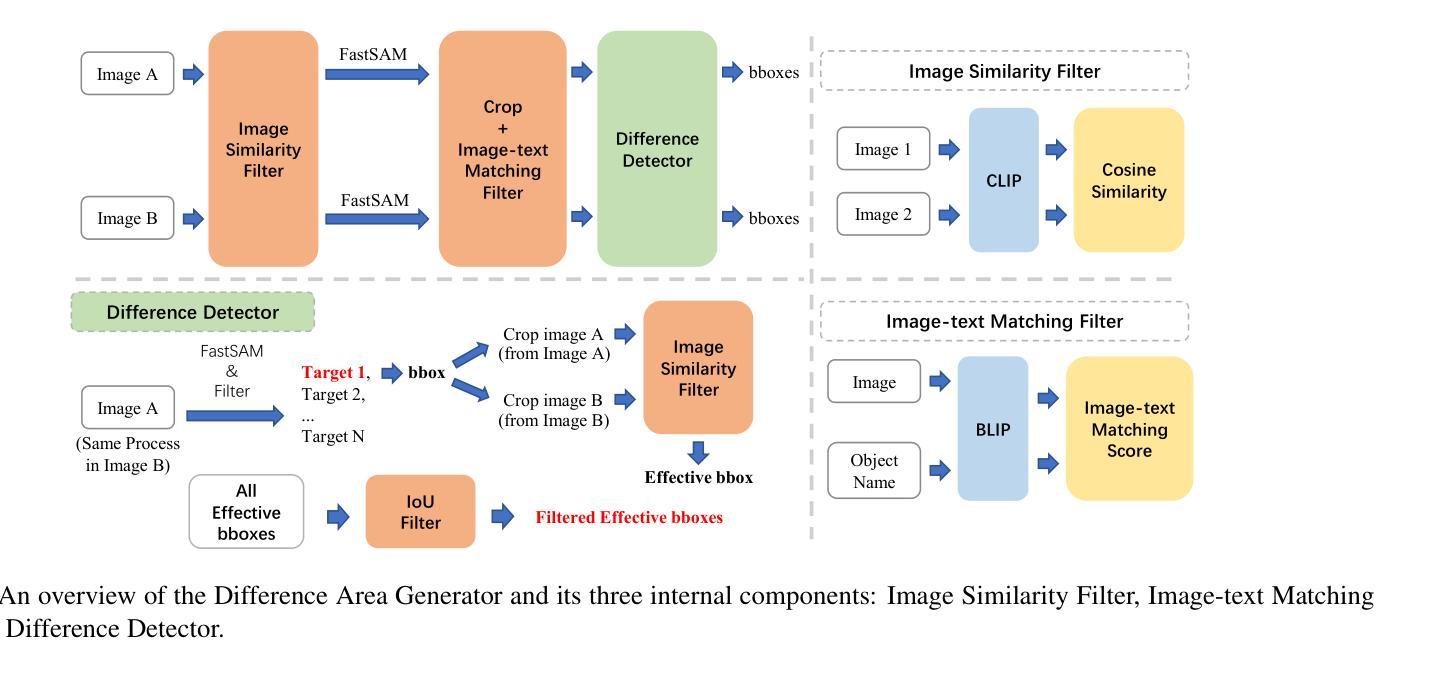
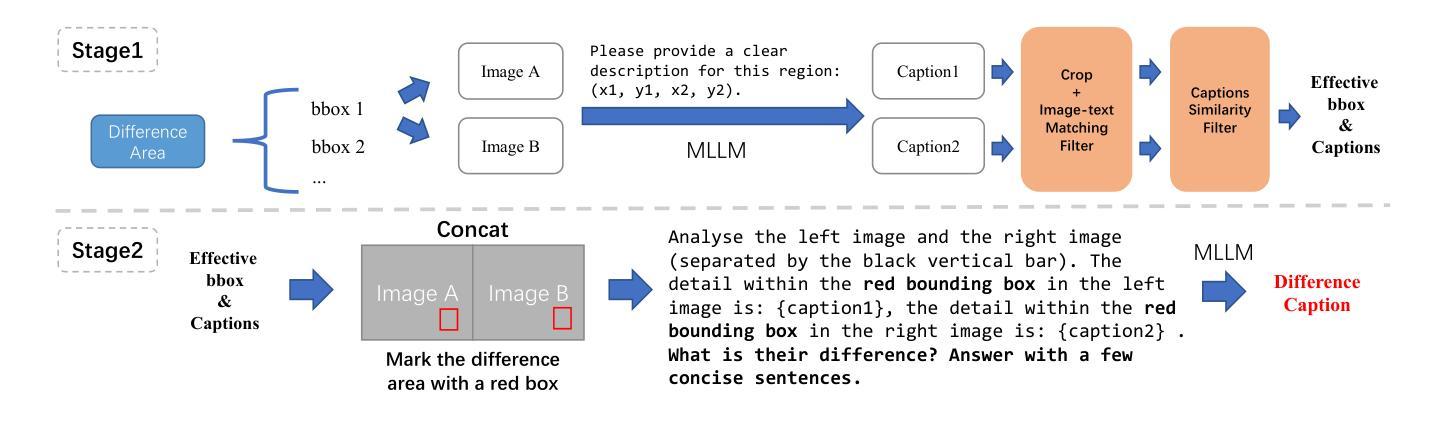
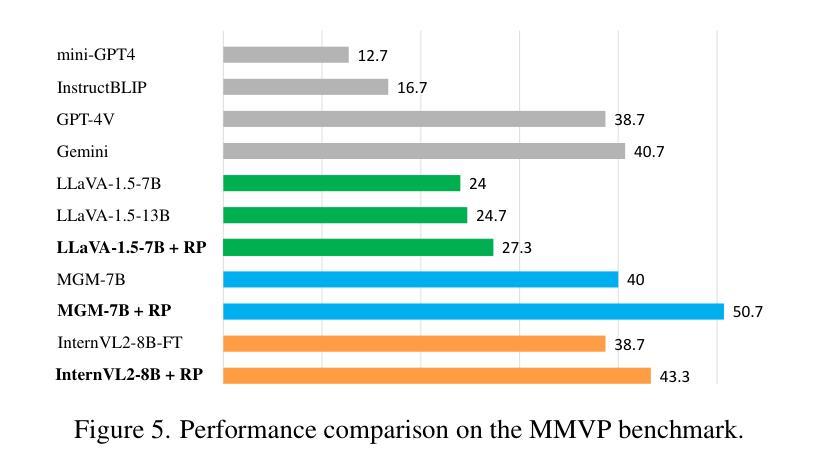
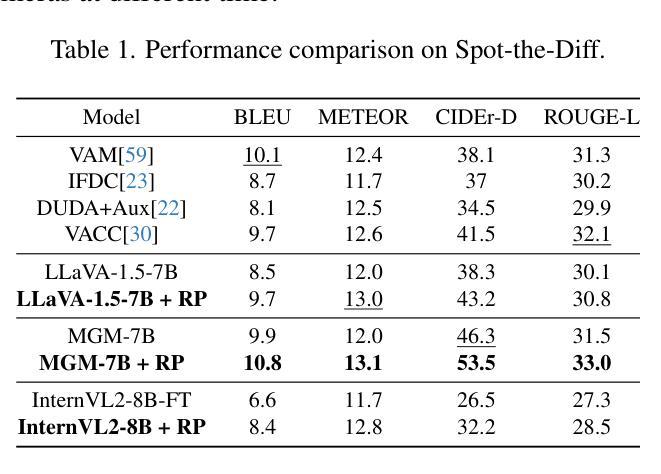