⚠️ 以下所有内容总结都来自于 大语言模型的能力,如有错误,仅供参考,谨慎使用
🔴 请注意:千万不要用于严肃的学术场景,只能用于论文阅读前的初筛!
💗 如果您觉得我们的项目对您有帮助 ChatPaperFree ,还请您给我们一些鼓励!⭐️ HuggingFace免费体验
2024-12-21 更新
Alignment-Free RGB-T Salient Object Detection: A Large-scale Dataset and Progressive Correlation Network
Authors:Kunpeng Wang, Keke Chen, Chenglong Li, Zhengzheng Tu, Bin Luo
Alignment-free RGB-Thermal (RGB-T) salient object detection (SOD) aims to achieve robust performance in complex scenes by directly leveraging the complementary information from unaligned visible-thermal image pairs, without requiring manual alignment. However, the labor-intensive process of collecting and annotating image pairs limits the scale of existing benchmarks, hindering the advancement of alignment-free RGB-T SOD. In this paper, we construct a large-scale and high-diversity unaligned RGB-T SOD dataset named UVT20K, comprising 20,000 image pairs, 407 scenes, and 1256 object categories. All samples are collected from real-world scenarios with various challenges, such as low illumination, image clutter, complex salient objects, and so on. To support the exploration for further research, each sample in UVT20K is annotated with a comprehensive set of ground truths, including saliency masks, scribbles, boundaries, and challenge attributes. In addition, we propose a Progressive Correlation Network (PCNet), which models inter- and intra-modal correlations on the basis of explicit alignment to achieve accurate predictions in unaligned image pairs. Extensive experiments conducted on unaligned and aligned datasets demonstrate the effectiveness of our method.Code and dataset are available at https://github.com/Angknpng/PCNet.
无对齐RGB-Thermal(RGB-T)显著性目标检测(SOD)旨在通过直接利用未对齐的可见光-热图像对中的互补信息,在复杂场景中实现稳健性能,而无需手动对齐。然而,收集和标注图像对的过程劳动强度大,限制了现有基准测试的规模,阻碍了无对齐的RGB-T SOD的发展。在本文中,我们构建了一个大规模、高多样性的无对齐RGB-T SOD数据集,名为UVT20K,包含20000个图像对、407个场景和1256个目标类别。所有样本都是从具有各种挑战的现实场景收集的,例如低光照、图像杂乱、复杂显著目标等。为了支持进一步的探索研究,UVT20K中的每个样本都使用一套全面的真实标注进行标注,包括显著性掩膜、涂鸦、边界和挑战属性。此外,我们提出了Progressive Correlation Network(PCNet),该网络基于显式对齐建模跨模态和内部模态关联,以实现未对齐图像对的准确预测。在未经对齐和已经对齐的数据集上进行的广泛实验证明了我们的方法的有效性。代码和数据集可在https://github.com/Angknpng/PCNet获得。
论文及项目相关链接
PDF Accepted by AAAI 2025
Summary:
无需手动对齐,直接利用可见光与热成像图像对的互补信息实现鲁棒性能,解决复杂场景下的RGB-T显著性目标检测问题。由于现有基准测试集规模受限,本文构建了一个大规模、高多样性的非对齐RGB-T显著性目标检测数据集UVT20K,包括不同的目标类别和场景挑战。同时提出一种基于显式对齐的渐进式关联网络(PCNet),对未对齐图像对进行准确预测。实验结果证明方法的有效性。代码和数据集已公开发布在GitHub上。
Key Takeaways:
一、研究背景:无需手动对齐的RGB-T显著性目标检测旨在利用可见光和热成像图像对的互补信息,实现复杂场景下的鲁棒性能。现有基准测试集规模受限,限制了研究进展。
二、数据集构建:本研究构建了大规模的非对齐RGB-T显著性目标检测数据集UVT20K,包含多样化的图像对、场景和对象类别。数据集中的样本来源于真实世界场景,包含多种挑战如低光照、图像杂乱等。每个样本都有详尽的标注信息,包括显著性掩膜、涂鸦、边界和挑战属性等。
三、方法创新:本研究提出了渐进式关联网络(PCNet),该网络基于显式对齐技术建模跨模态和内模态的关联,实现未对齐图像对的准确预测。此网络在不对齐和已对齐的数据集上的实验均证明了其有效性。
点此查看论文截图
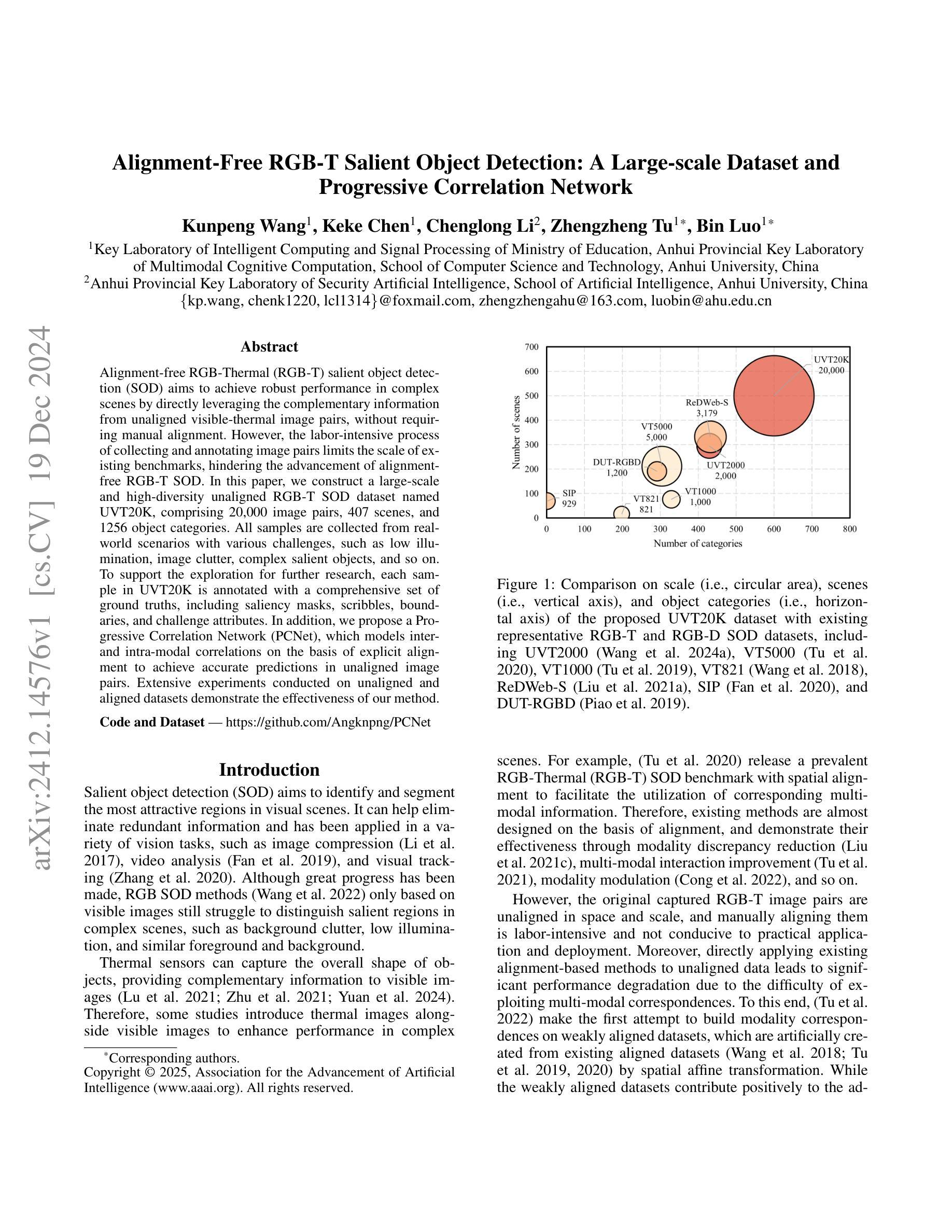
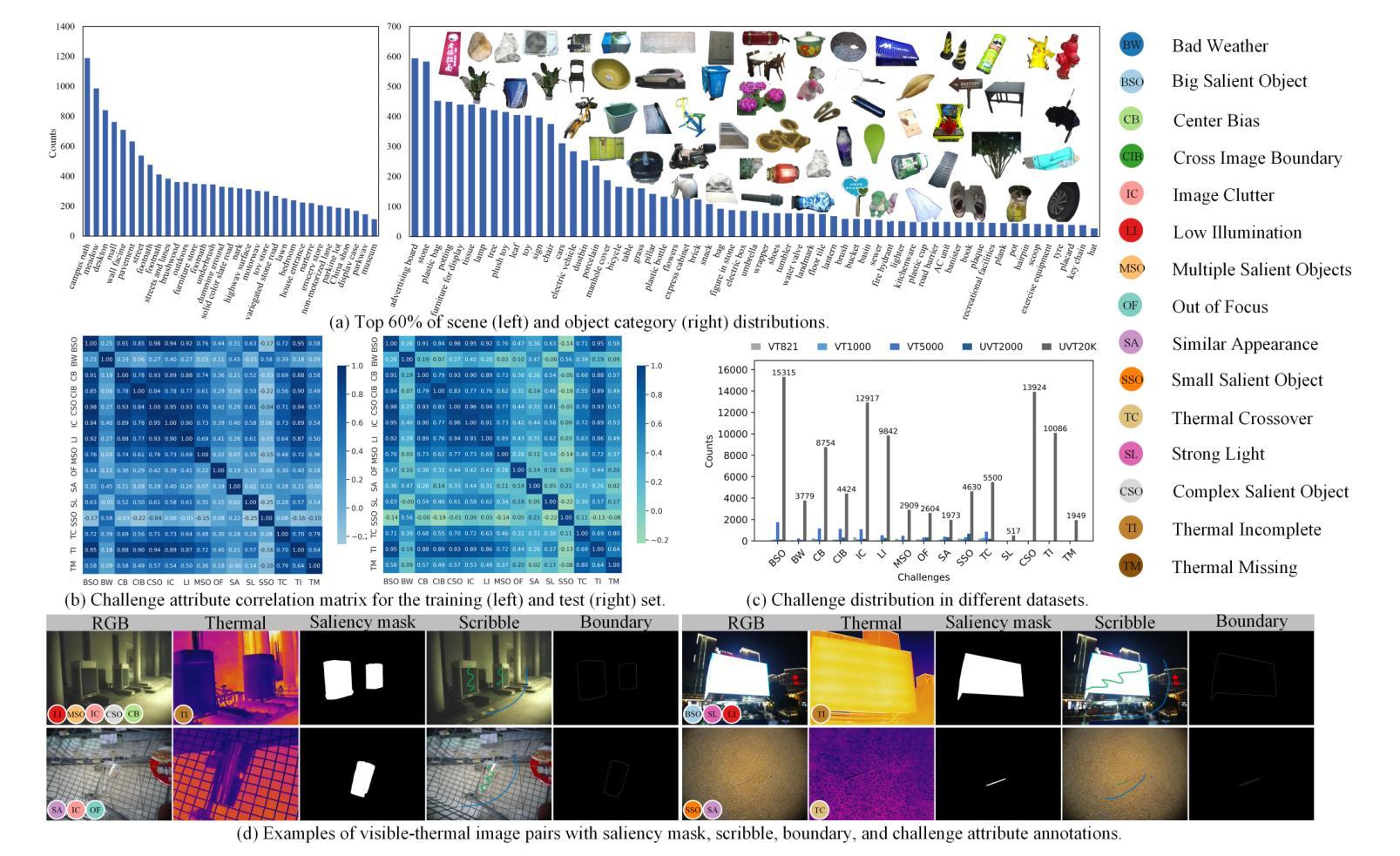
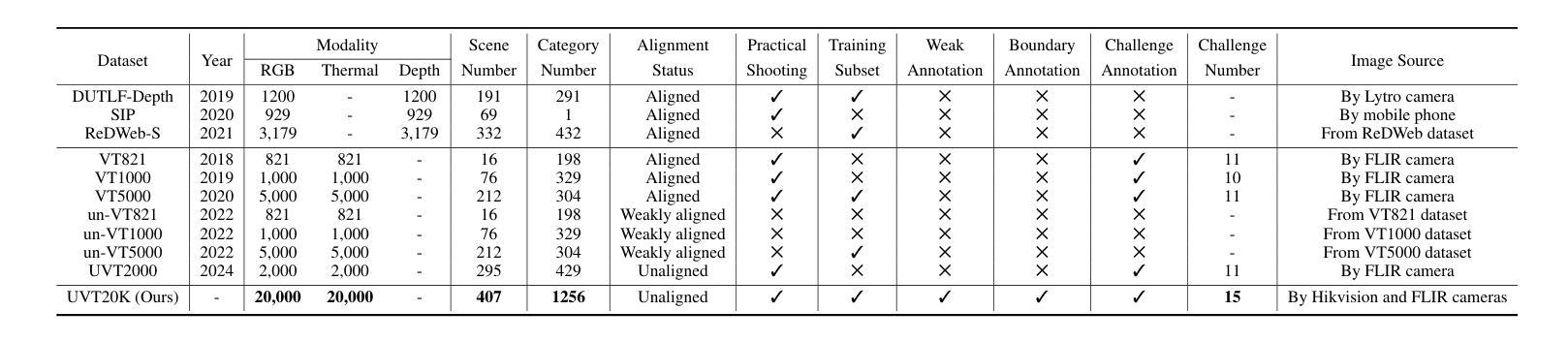
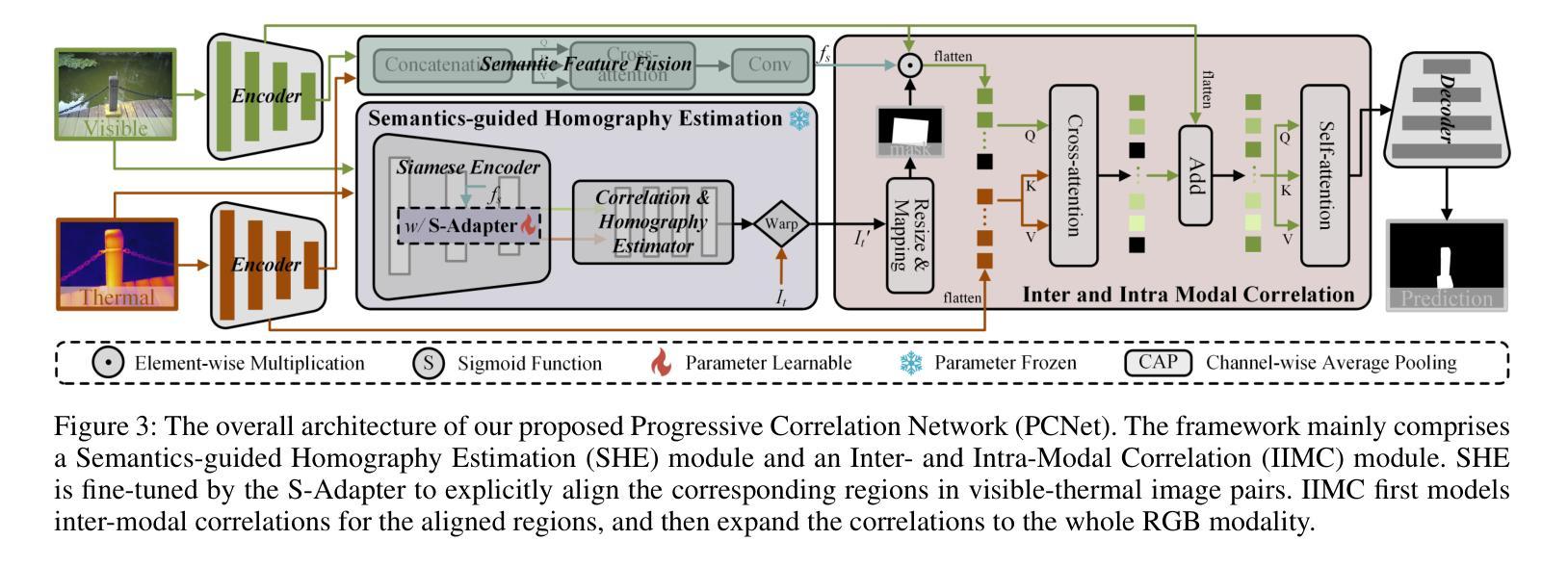
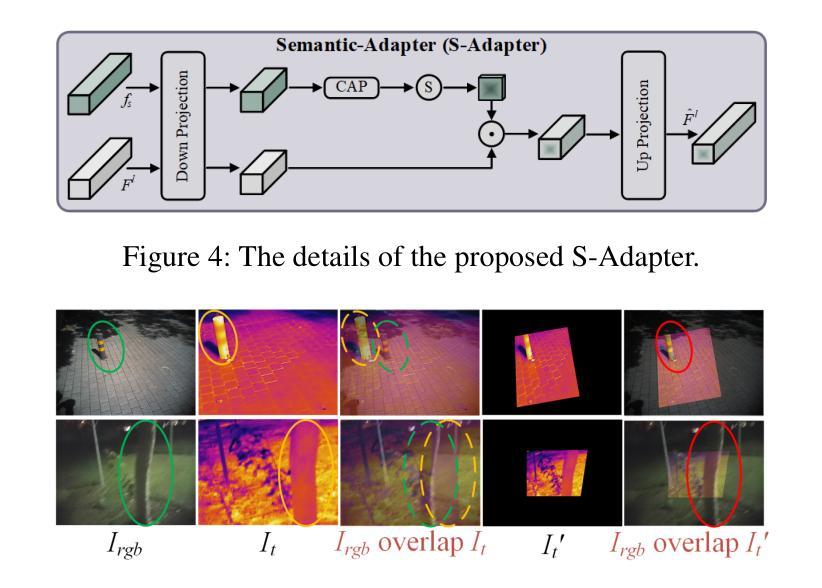
HA-RDet: Hybrid Anchor Rotation Detector for Oriented Object Detection
Authors:Phuc D. A. Nguyen
Oriented object detection in aerial images poses a significant challenge due to their varying sizes and orientations. Current state-of-the-art detectors typically rely on either two-stage or one-stage approaches, often employing Anchor-based strategies, which can result in computationally expensive operations due to the redundant number of generated anchors during training. In contrast, Anchor-free mechanisms offer faster processing but suffer from a reduction in the number of training samples, potentially impacting detection accuracy. To address these limitations, we propose the Hybrid-Anchor Rotation Detector (HA-RDet), which combines the advantages of both anchor-based and anchor-free schemes for oriented object detection. By utilizing only one preset anchor for each location on the feature maps and refining these anchors with our Orientation-Aware Convolution technique, HA-RDet achieves competitive accuracies, including 75.41 mAP on DOTA-v1, 65.3 mAP on DIOR-R, and 90.2 mAP on HRSC2016, against current anchor-based state-of-the-art methods, while significantly reducing computational resources.
空中图像中的定向目标检测由于其尺寸和方向的多样性而面临重大挑战。当前最先进的检测器通常依赖于两阶段或单阶段方法,通常采用基于锚框的策略,由于在训练过程中生成的锚框数量冗余,这可能导致计算资源消耗较高的操作。相比之下,无锚框机制处理速度更快,但受到训练样本数量减少的影响,可能影响检测精度。为了解决这些局限性,我们提出了混合锚框旋转检测器(HA-RDet),它结合了基于锚框和无锚框方案的优势,用于定向目标检测。通过在特征图上的每个位置只使用一个预设锚框,并结合我们的方向感知卷积技术进行锚框细化,HA-RDet实现了具有竞争力的准确性,包括在DOTA-v1上的75.41 mAP、DIOR-R上的65.3 mAP和HRSC2016上的90.2 mAP,与当前基于锚框的最先进方法相比,同时显著减少了计算资源。
论文及项目相关链接
PDF Bachelor thesis
Summary
面向航拍图像的定向目标检测面临尺寸和方向变化带来的挑战。当前主流检测方法主要依赖两阶段或一阶段的方法,并采用基于锚点(Anchor-based)的策略,导致计算量大且存在冗余锚点。相反,无锚点(Anchor-free)机制处理速度快但训练样本数量减少,可能影响检测精度。为此,我们提出混合锚点旋转检测器(HA-RDet),结合基于锚点和无锚点的优势进行定向目标检测。通过在特征图上每个位置仅使用一个预设锚点,并结合我们的方向感知卷积技术进行优化,HA-RDet在DOTA-v1上达到了75.41 mAP,在DIOR-R上达到了65.3 mAP,在HRSC2016上达到了90.2 mAP的竞争力准确率,与当前基于锚点的最先进方法相比,并显著减少了计算资源。
Key Takeaways
- 航拍图像中的定向目标检测面临尺寸和方向的挑战。
- 当前主流检测方法主要依赖基于锚点或无锚点的策略。
- 基于锚点的方法计算量大并存在冗余锚点,而无锚点方法训练样本数量减少可能影响精度。
- 混合锚点旋转检测器(HA-RDet)结合了基于锚点和无锚点的优势。
- HA-RDet在每个位置仅使用一个预设锚点,并使用方向感知卷积技术优化。
- HA-RDet在多个数据集上实现了高准确率,具有竞争力。
点此查看论文截图
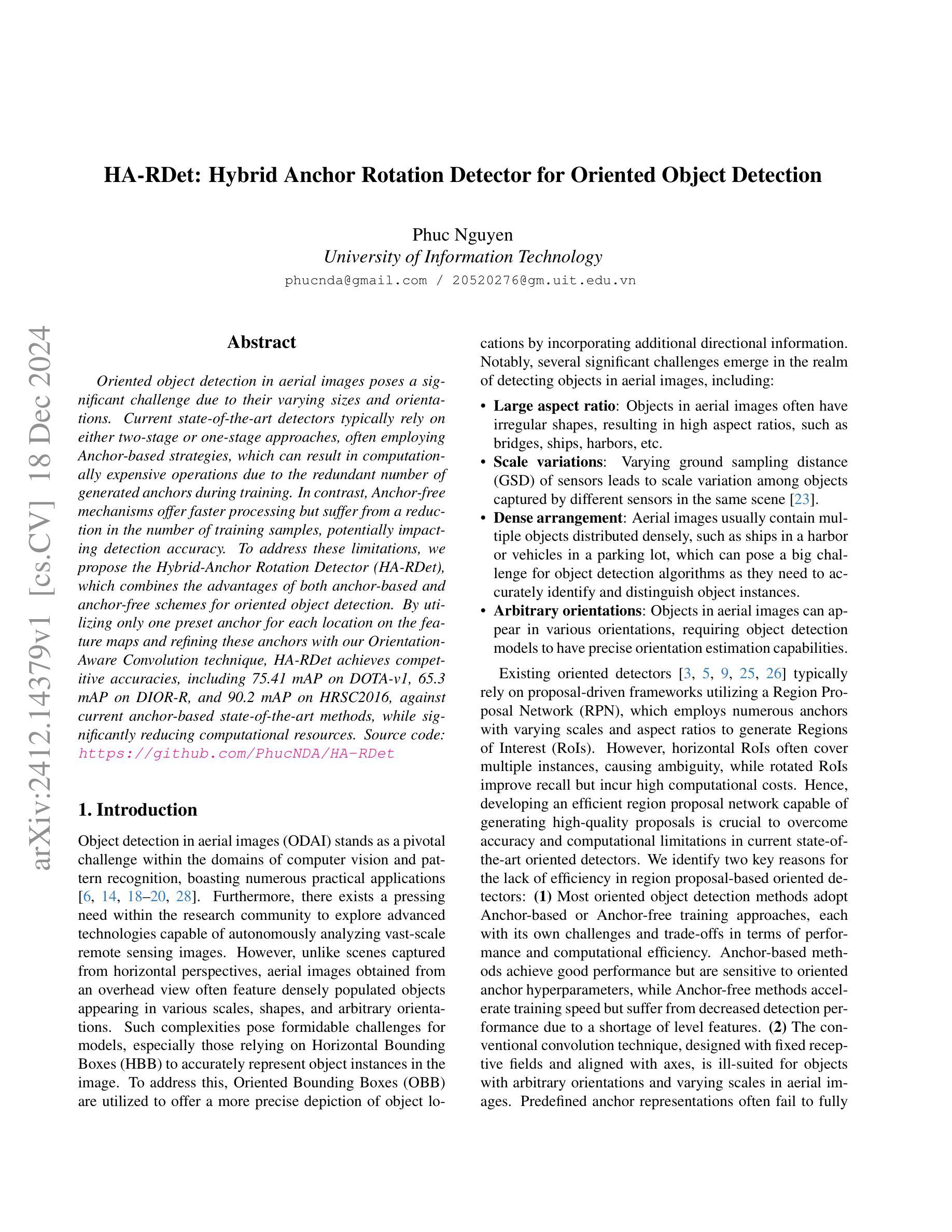
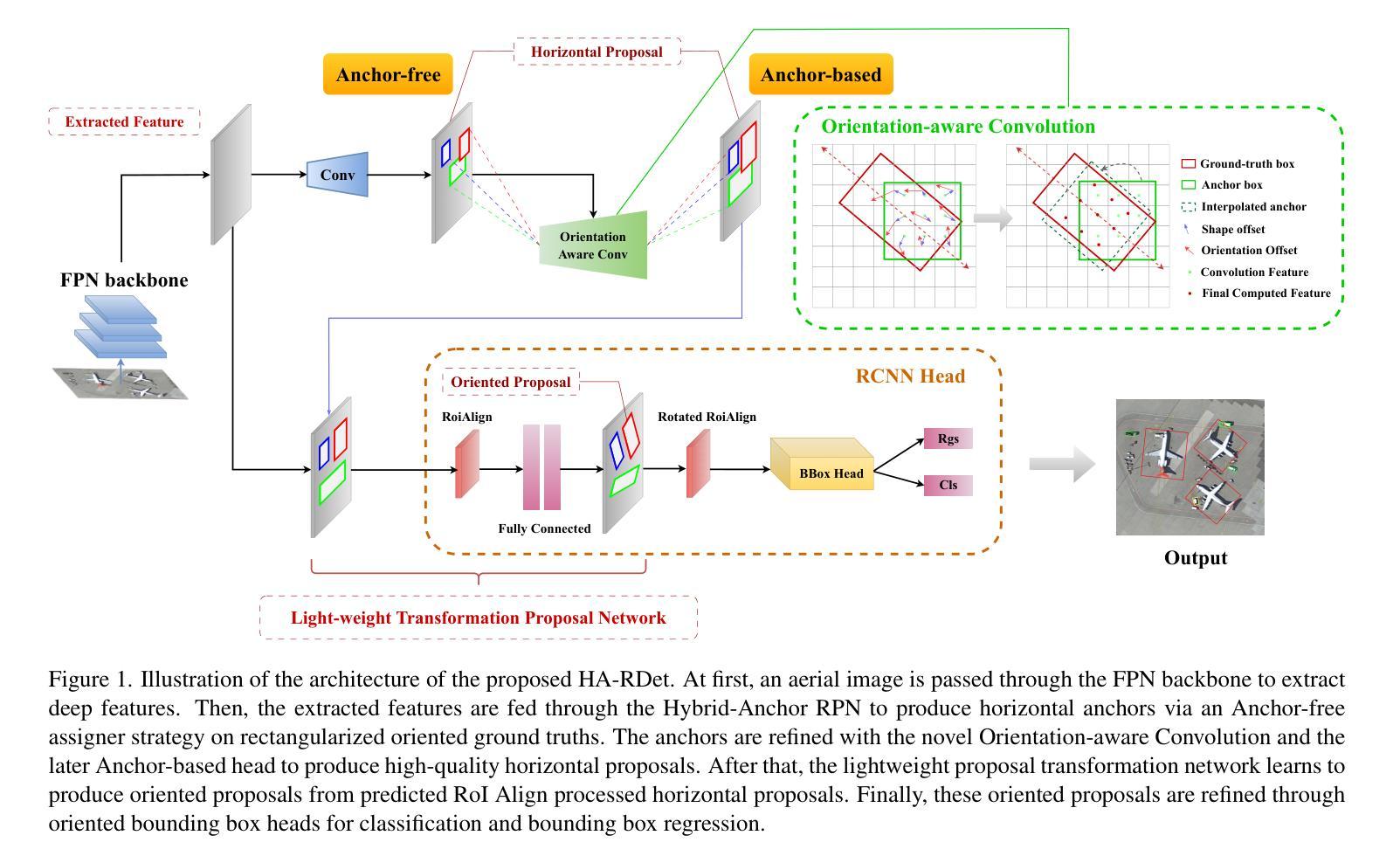
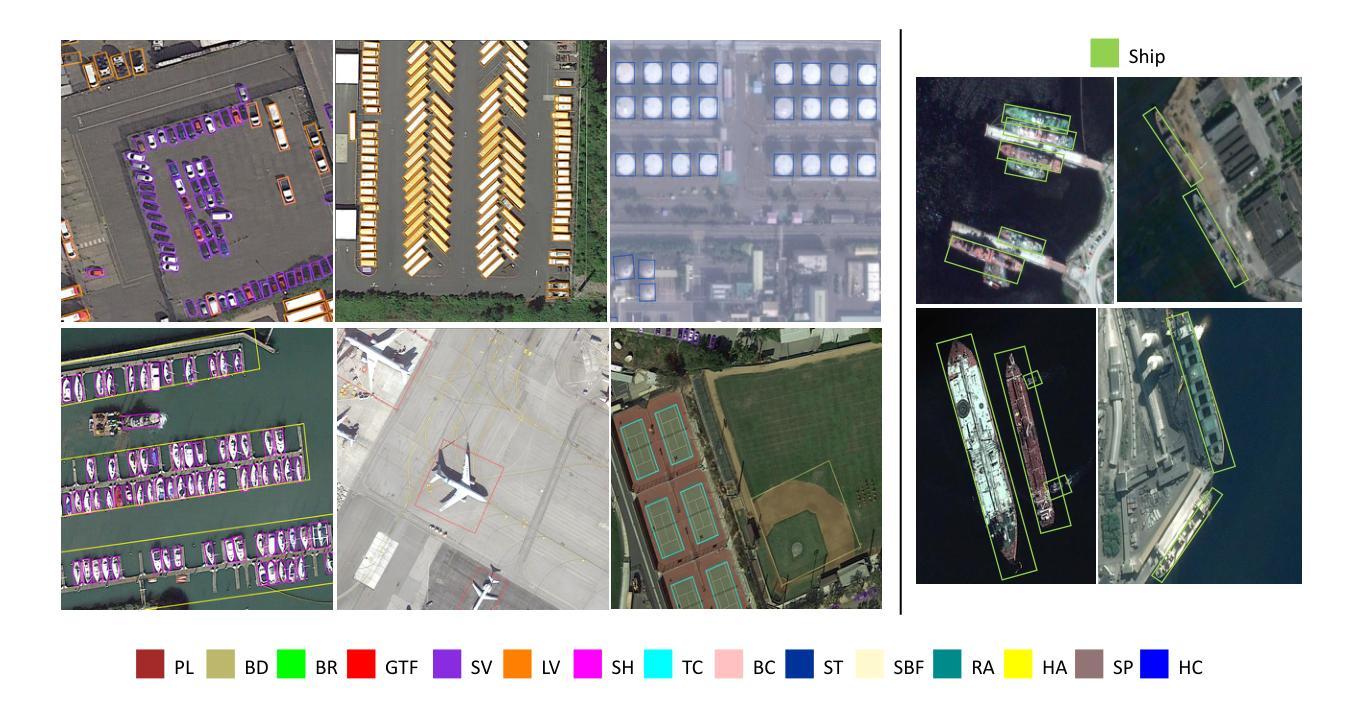
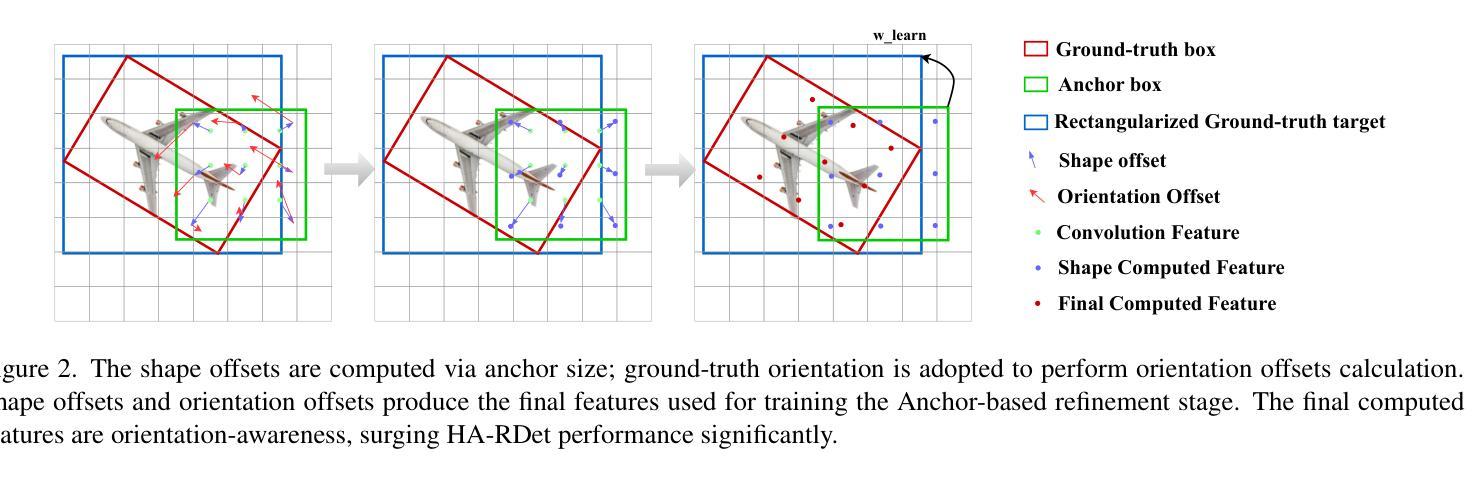
PoLaRIS Dataset: A Maritime Object Detection and Tracking Dataset in Pohang Canal
Authors:Jiwon Choi, Dongjin Cho, Gihyeon Lee, Hogyun Kim, Geonmo Yang, Joowan Kim, Younggun Cho
Maritime environments often present hazardous situations due to factors such as moving ships or buoys, which become obstacles under the influence of waves. In such challenging conditions, the ability to detect and track potentially hazardous objects is critical for the safe navigation of marine robots. To address the scarcity of comprehensive datasets capturing these dynamic scenarios, we introduce a new multi-modal dataset that includes image and point-wise annotations of maritime hazards. Our dataset provides detailed ground truth for obstacle detection and tracking, including objects as small as 10$\times$10 pixels, which are crucial for maritime safety. To validate the dataset’s effectiveness as a reliable benchmark, we conducted evaluations using various methodologies, including \ac{SOTA} techniques for object detection and tracking. These evaluations are expected to contribute to performance improvements, particularly in the complex maritime environment. To the best of our knowledge, this is the first dataset offering multi-modal annotations specifically tailored to maritime environments. Our dataset is available at https://sites.google.com/view/polaris-dataset.
海洋环境经常由于移动船只或浮标等因素而出现危险情况,这些物体在波浪的影响下会成为障碍物。在这样的挑战条件下,检测和跟踪潜在危险物体的能力对于海洋机器人的安全导航至关重要。为了解决缺乏捕捉这些动态场景的全面数据集的问题,我们引入了一个新的多模式数据集,包括海事危险的图像和点状注释。我们的数据集为障碍检测和跟踪提供了详细的真实地面信息,包括小到10x10像素的物体,这对于海事安全至关重要。为了验证数据集作为可靠基准的有效性,我们采用了各种方法进行评估,包括最先进的物体检测和跟踪技术。这些评估有望对性能提升做出贡献,特别是在复杂的海洋环境中。据我们所知,这是第一个提供专门针对海洋环境的多模式注释的数据集。我们的数据集可在https://sites.google.com/view/polaris-dataset上获取。
论文及项目相关链接
Summary:面临海洋环境中的动态挑战,检测与跟踪海上危险物体对航海安全至关重要。为解决缺少综合数据集的问题,我们引入新型多模式数据集,包含图像和点对海事危险的注释。数据集提供障碍物检测的详细真实情况,包括关键的小物体。我们对数据集进行了有效性评估,使用先进的目标检测和跟踪技术。这是首个针对海事环境定制的多模式注释数据集。
Key Takeaways:
- 海洋环境中的动态挑战要求对海上危险物体进行准确的检测与跟踪以保障航海安全。
- 缺乏专门捕捉这些动态场景的综合数据集。
- 我们引入了新型多模式数据集,涵盖图像和点对海事危险的注释,提供详细的地面真实情况。
- 数据集包含小到10x10像素的物体,这对于海事安全至关重要。
- 数据集经过各种先进的目标检测和跟踪技术的评估验证其有效性。
- 这是首个专门针对海事环境定制的多模式注释数据集。
点此查看论文截图
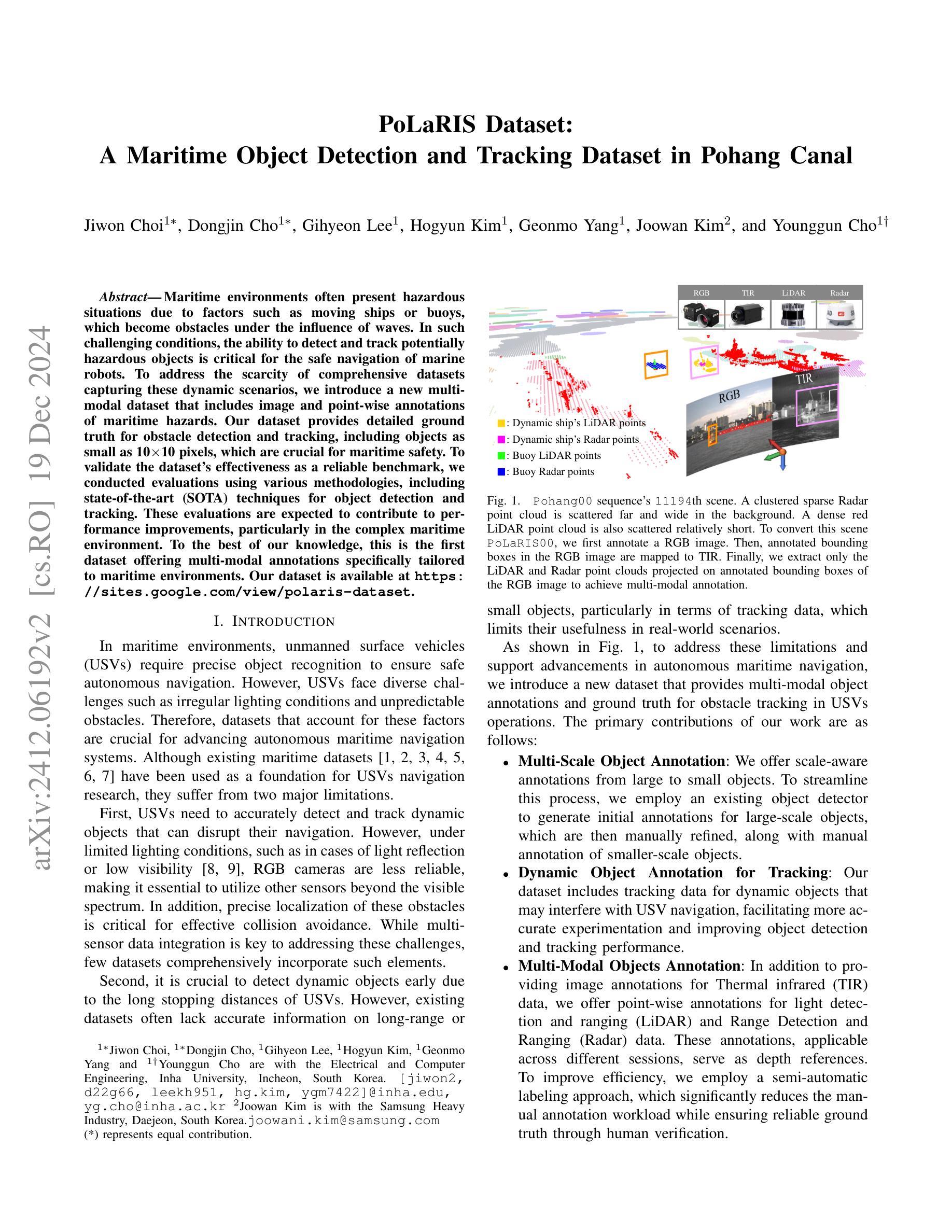
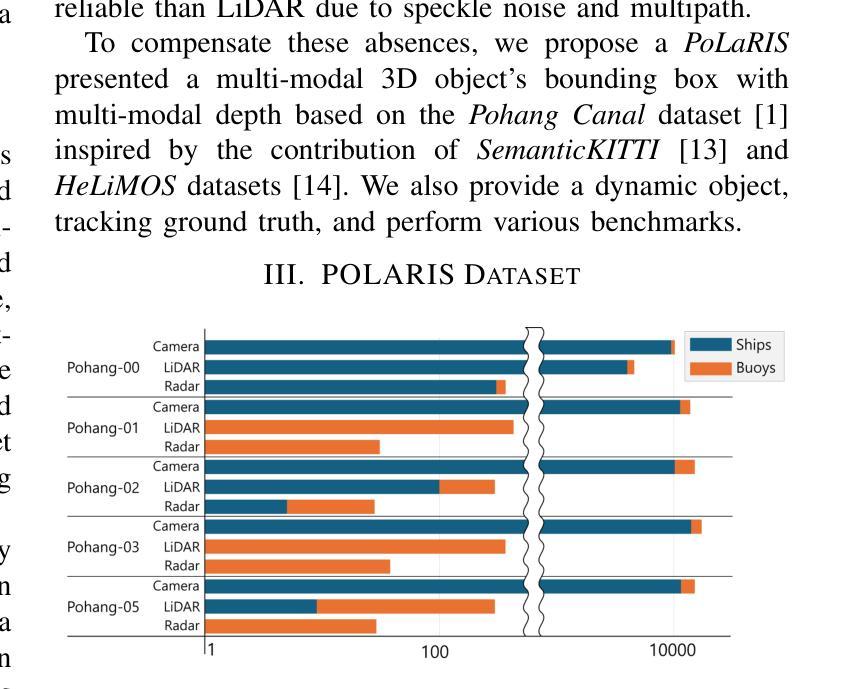
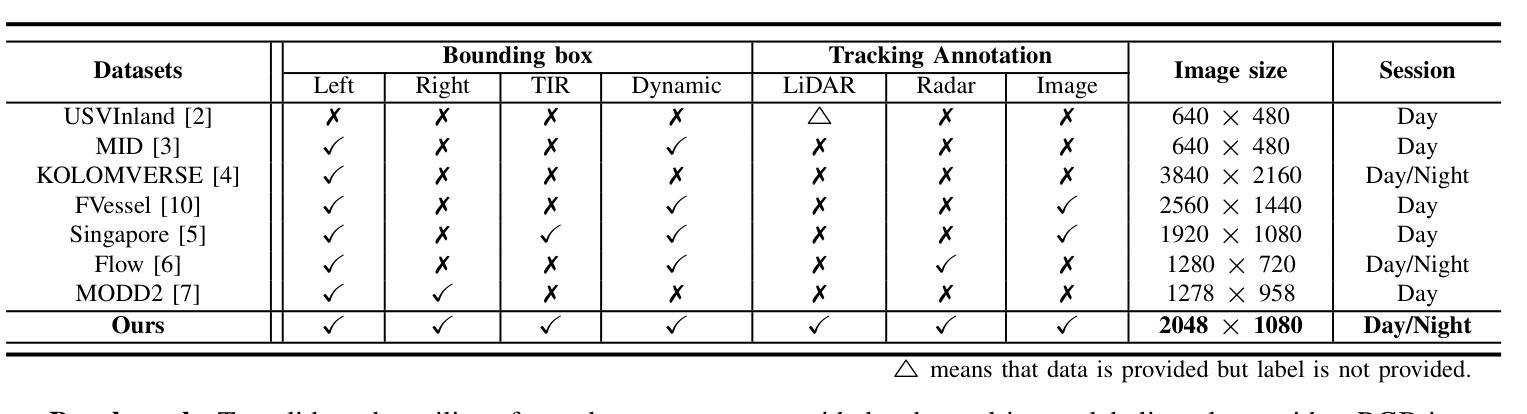
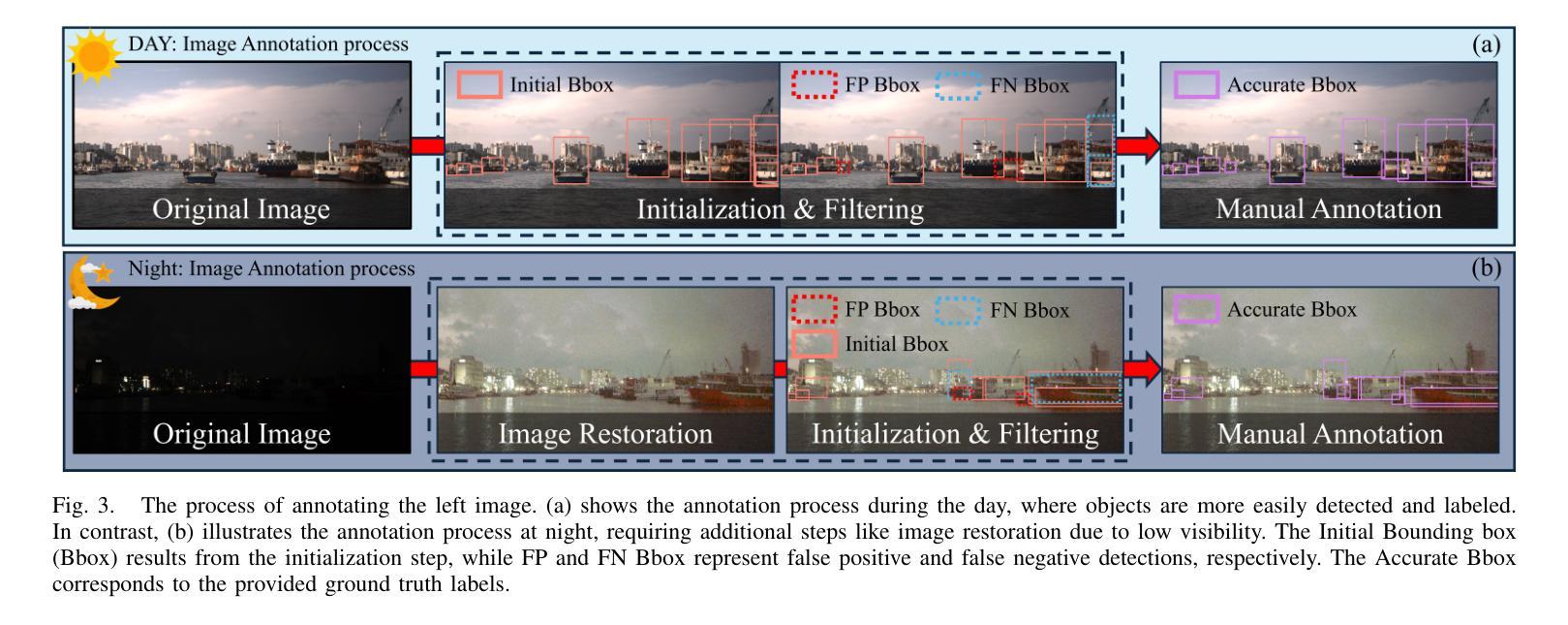
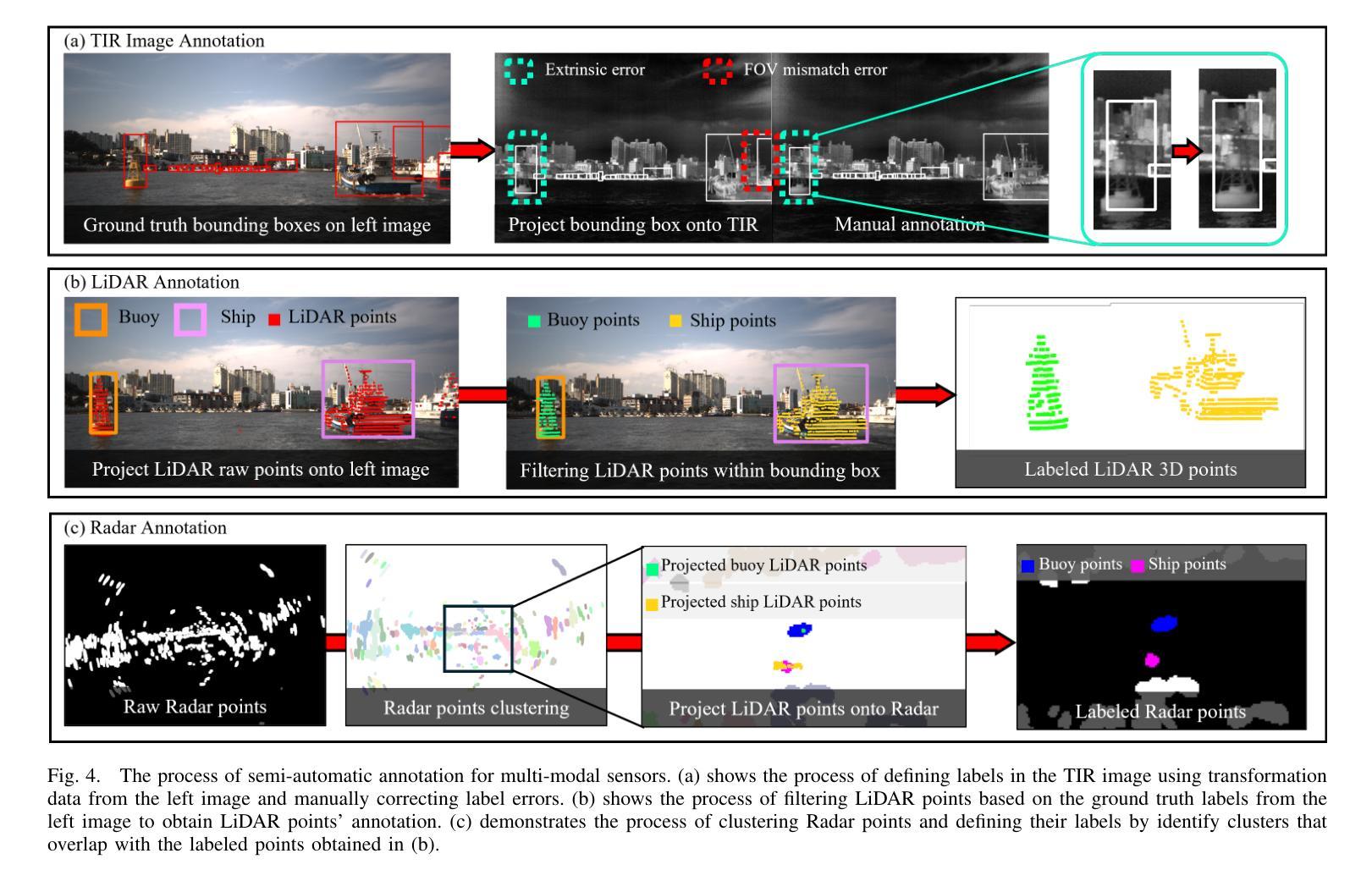
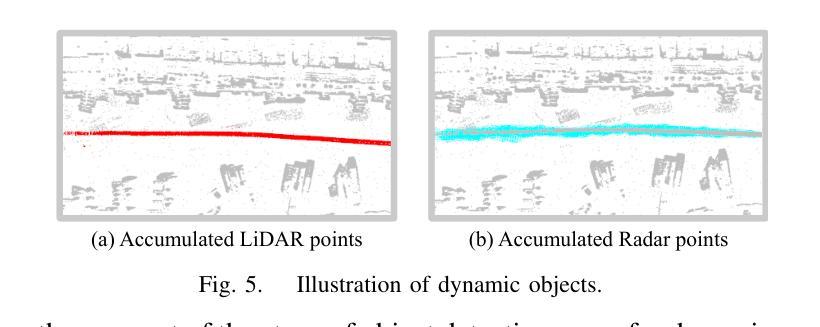
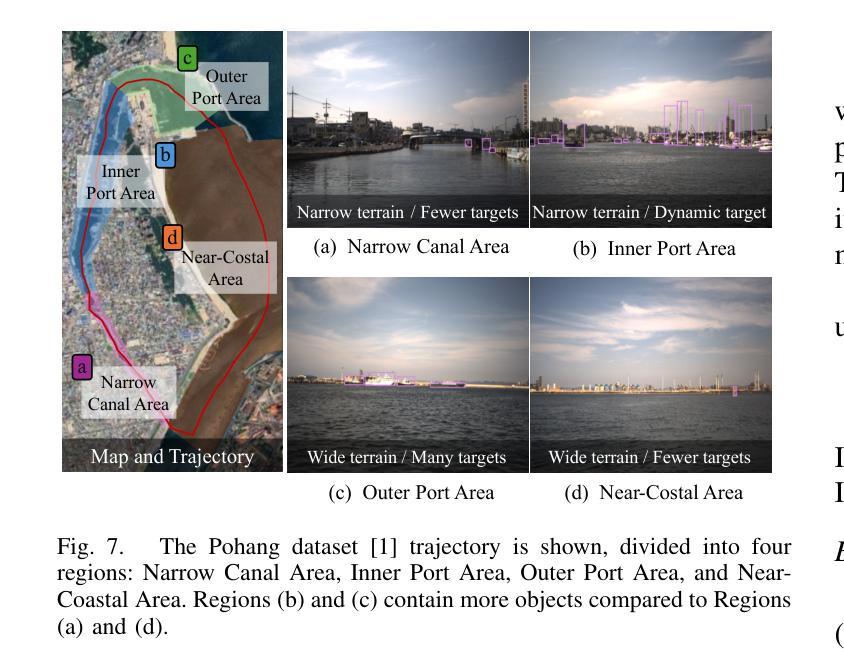
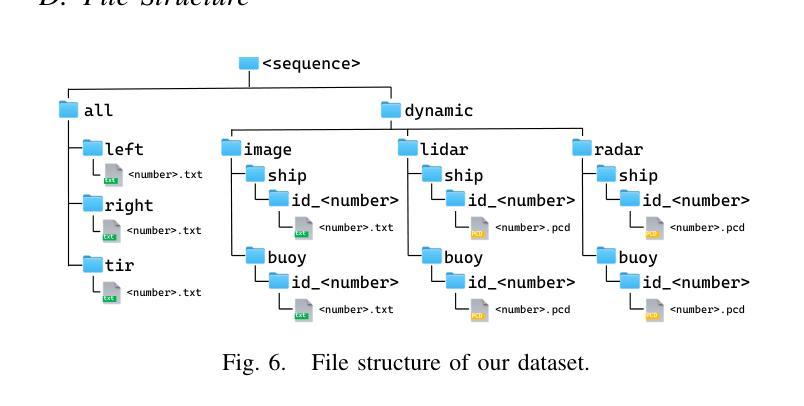