⚠️ 以下所有内容总结都来自于 大语言模型的能力,如有错误,仅供参考,谨慎使用
🔴 请注意:千万不要用于严肃的学术场景,只能用于论文阅读前的初筛!
💗 如果您觉得我们的项目对您有帮助 ChatPaperFree ,还请您给我们一些鼓励!⭐️ HuggingFace免费体验
2024-12-21 更新
ProsodyFM: Unsupervised Phrasing and Intonation Control for Intelligible Speech Synthesis
Authors:Xiangheng He, Junjie Chen, Zixing Zhang, Björn W. Schuller
Prosody contains rich information beyond the literal meaning of words, which is crucial for the intelligibility of speech. Current models still fall short in phrasing and intonation; they not only miss or misplace breaks when synthesizing long sentences with complex structures but also produce unnatural intonation. We propose ProsodyFM, a prosody-aware text-to-speech synthesis (TTS) model with a flow-matching (FM) backbone that aims to enhance the phrasing and intonation aspects of prosody. ProsodyFM introduces two key components: a Phrase Break Encoder to capture initial phrase break locations, followed by a Duration Predictor for the flexible adjustment of break durations; and a Terminal Intonation Encoder which learns a bank of intonation shape tokens combined with a novel Pitch Processor for more robust modeling of human-perceived intonation change. ProsodyFM is trained with no explicit prosodic labels and yet can uncover a broad spectrum of break durations and intonation patterns. Experimental results demonstrate that ProsodyFM can effectively improve the phrasing and intonation aspects of prosody, thereby enhancing the overall intelligibility compared to four state-of-the-art (SOTA) models. Out-of-distribution experiments show that this prosody improvement can further bring ProsodyFM superior generalizability for unseen complex sentences and speakers. Our case study intuitively illustrates the powerful and fine-grained controllability of ProsodyFM over phrasing and intonation.
韵律包含超越单词字面意义的丰富信息,这对于语音的清晰度至关重要。当前模型在短语和语调方面仍然存在不足;它们在合成具有复杂结构的长句子时,不仅会遗漏或错位断句,而且会产生不自然的语调。我们提出了ProsodyFM,这是一款具有流匹配(FM)骨架的韵律感知文本到语音(TTS)合成模型,旨在增强韵律的短语和语调方面。ProsodyFM引入了两个关键组件:一个短语断句编码器,用于捕捉初始短语断句位置,随后是一个时长预测器,用于灵活地调整断句时长;以及一个终端语调编码器,它学习一系列语调形状标记,并结合一个新型音调处理器,对人类感知的语调变化进行更稳健的建模。ProsodyFM的训练不需要明确的韵律标签,但可以揭示广泛的断句时长和语调模式。实验结果表明,与四种最先进模型相比,ProsodyFM可以有效改善韵律的短语和语调方面,从而提高整体清晰度。超出分布范围的实验表明,这种韵律改进可以进一步提高ProsodyFM对未见过的复杂句子和说话者的泛化能力。我们的案例研究直观地说明了ProsodyFM在短语和语调方面的强大和精细可控性。
论文及项目相关链接
PDF Accepted by AAAI 2025
Summary
文本主要探讨了在文本转语音合成(TTS)中,韵律包含的词义之外的丰富信息对于语音清晰度的关键性。现有模型在句法和语调方面存在缺陷,无法准确把握长句复杂结构的断句位置及语调。为此,本文提出了基于流匹配的韵律感知TTS模型——ProsodyFM,旨在改善句法和语调方面的韵律问题。该模型引入了两个关键组件:短语断句编码器用于捕捉初始断句位置,时长预测器用于灵活调整断句时长;终端语调编码器学习一系列语调形状标记,并结合新颖的音调处理器,更稳健地模拟人类感知的语调变化。无需明确的韵律标签,ProsodyFM能够发现多种断句时长和语调模式。实验结果显示,相较于四种最新模型,ProsodyFM在句法、语调方面的改善有效提升了语音的整体清晰度,并且对未见复杂句子和新说话者的泛化能力更强。
Key Takeaways
- 韵律在文本转语音合成中至关重要,包含超越词汇字面意义的丰富信息,对语音的清晰度有重要影响。
- 当前TTS模型在句法(断句)和语调方面存在不足,无法准确合成复杂长句的断句位置和自然语调。
- 提出了一种新的韵律感知TTS模型——ProsodyFM,旨在解决上述问题。
- ProsodyFM包含两个核心组件:短语断句编码器和时长预测器,用于改善断句和时长调整。
- 还引入了终端语调编码器和音调处理器,以更稳健地模拟人类语调变化。
- ProsodyFM无需明确的韵律标签即可发现多种断句时长和语调模式。
点此查看论文截图
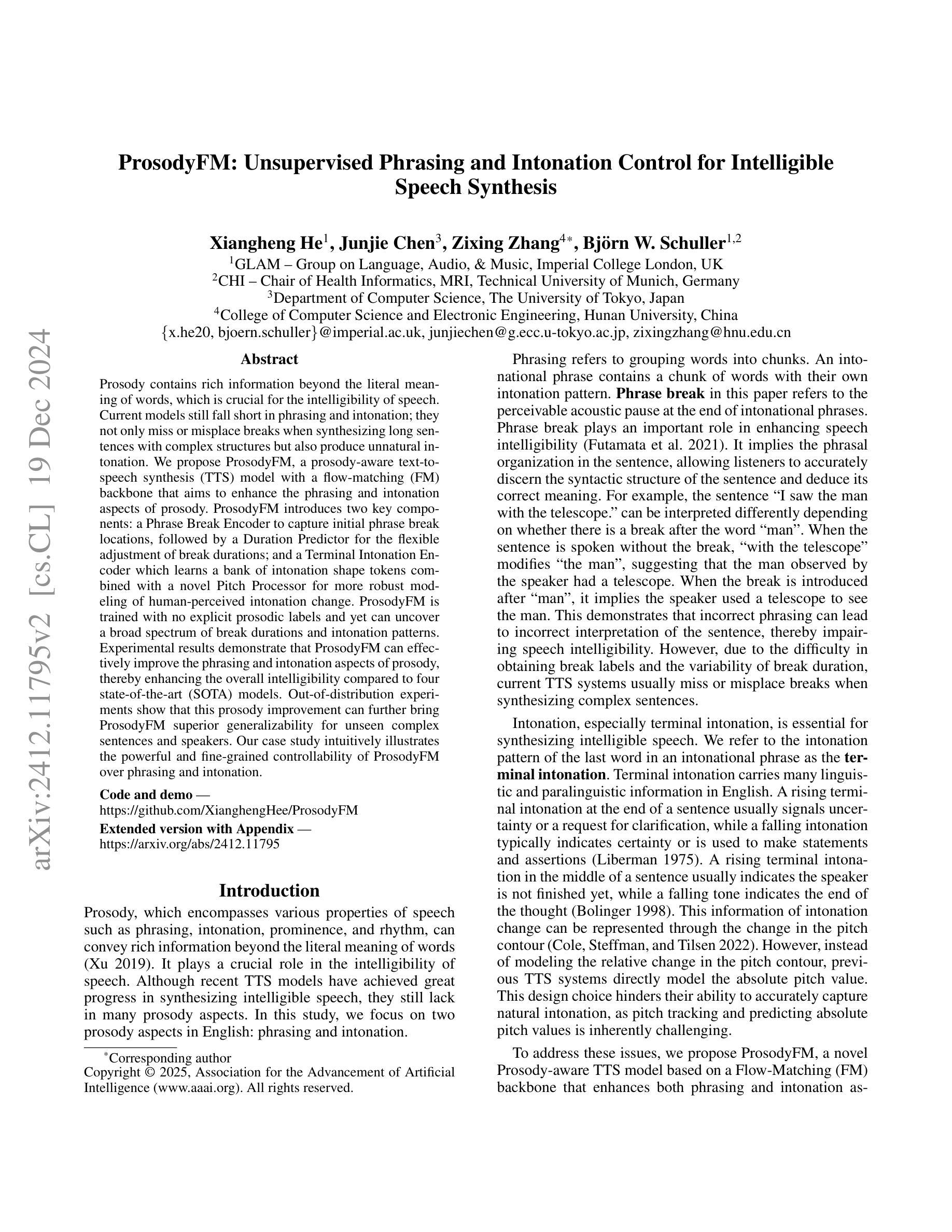
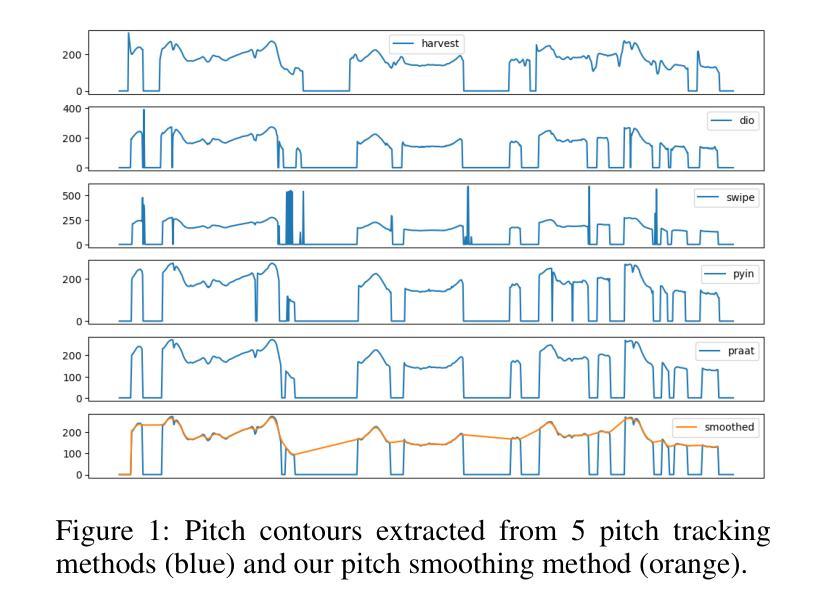
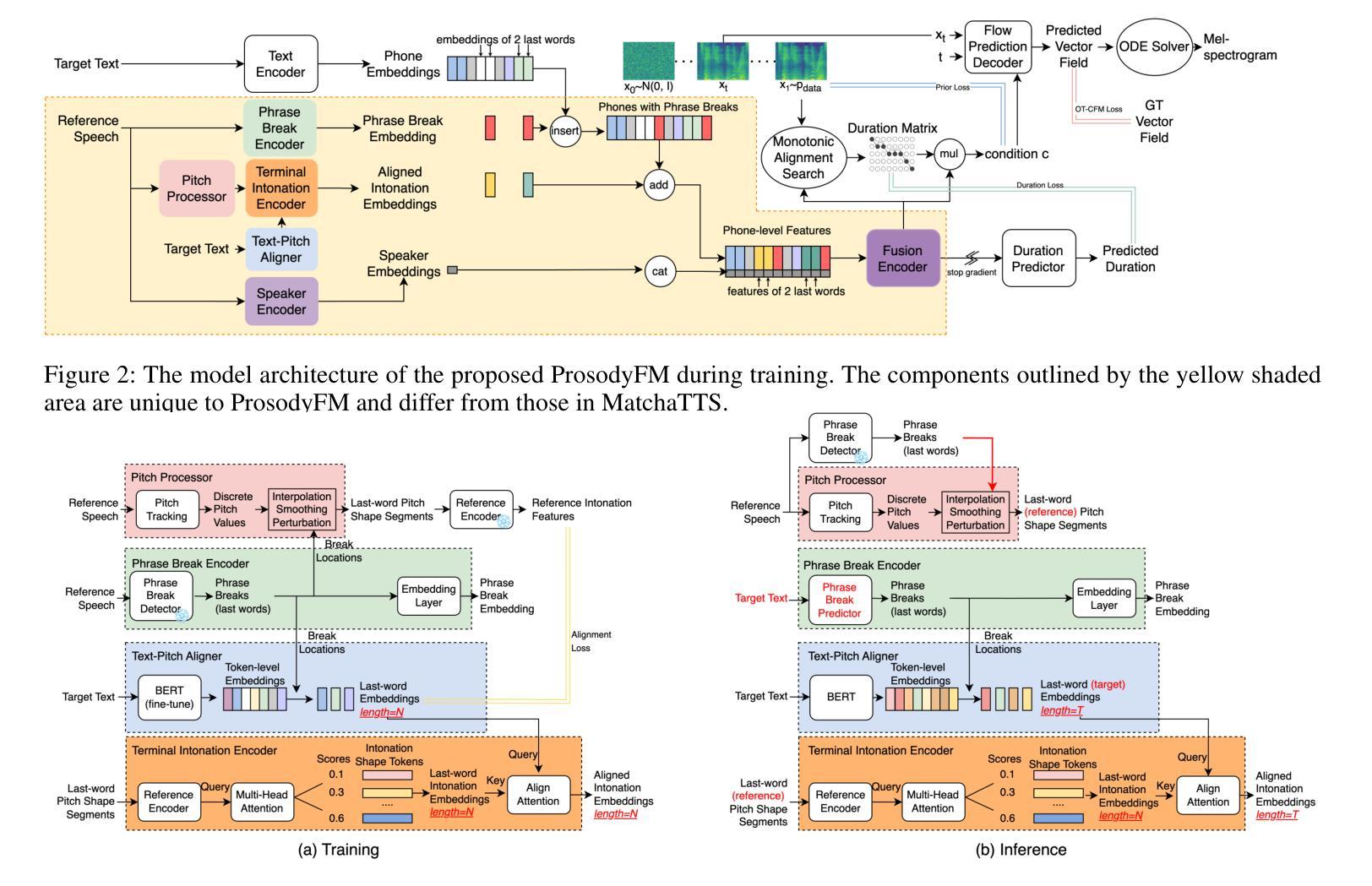
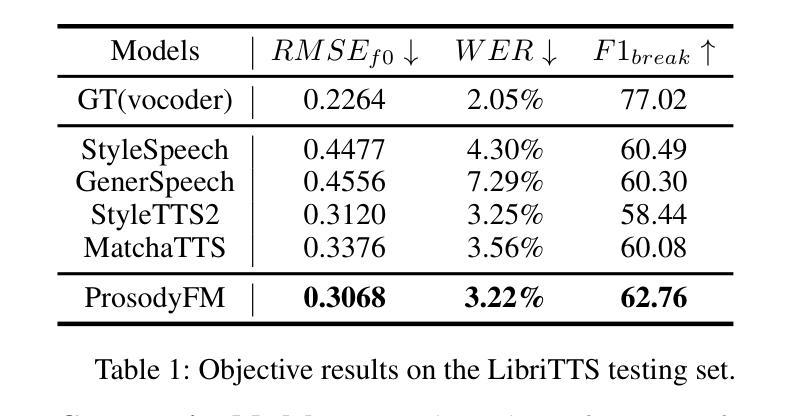
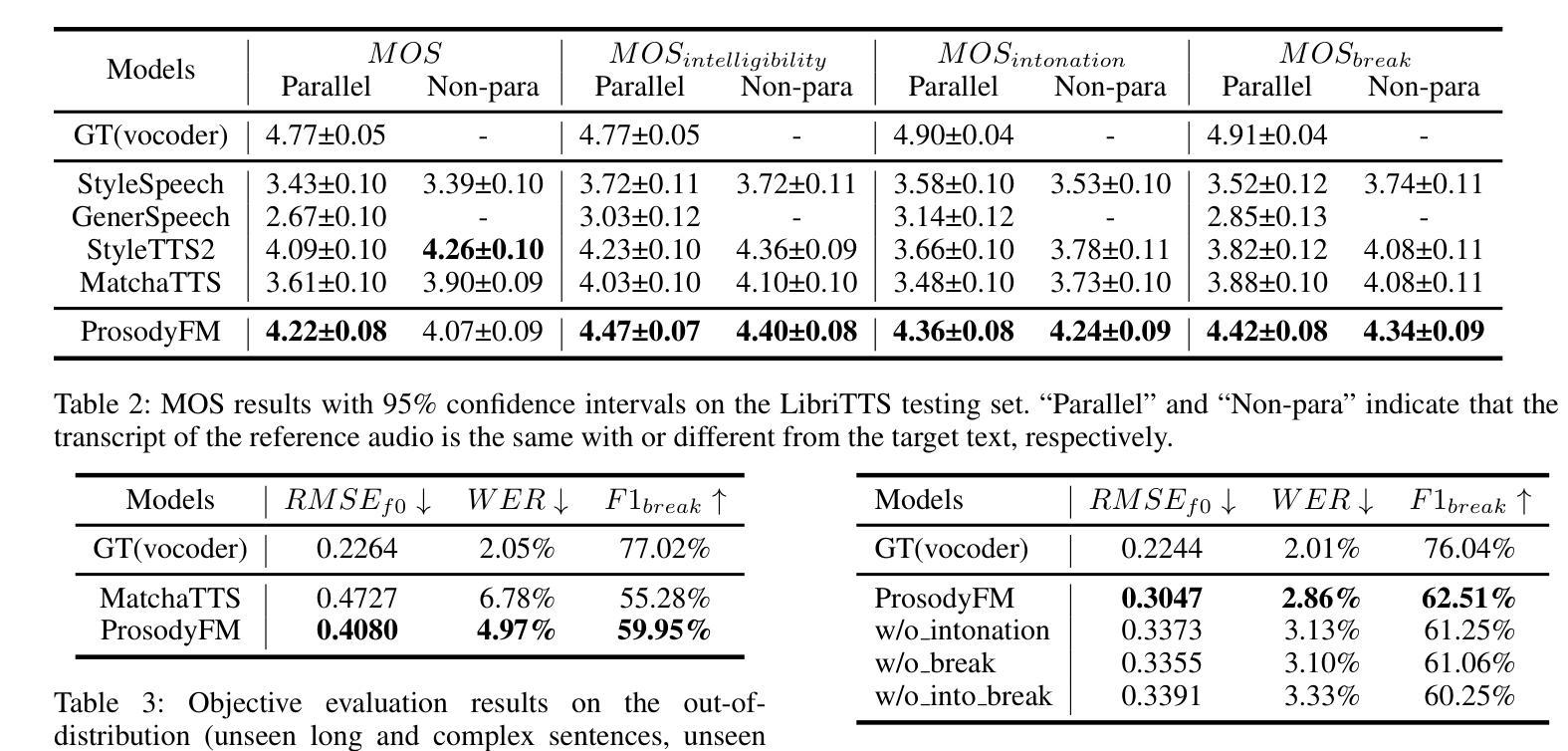