⚠️ 以下所有内容总结都来自于 大语言模型的能力,如有错误,仅供参考,谨慎使用
🔴 请注意:千万不要用于严肃的学术场景,只能用于论文阅读前的初筛!
💗 如果您觉得我们的项目对您有帮助 ChatPaperFree ,还请您给我们一些鼓励!⭐️ HuggingFace免费体验
2024-12-21 更新
EnergyMoGen: Compositional Human Motion Generation with Energy-Based Diffusion Model in Latent Space
Authors:Jianrong Zhang, Hehe Fan, Yi Yang
Diffusion models, particularly latent diffusion models, have demonstrated remarkable success in text-driven human motion generation. However, it remains challenging for latent diffusion models to effectively compose multiple semantic concepts into a single, coherent motion sequence. To address this issue, we propose EnergyMoGen, which includes two spectrums of Energy-Based Models: (1) We interpret the diffusion model as a latent-aware energy-based model that generates motions by composing a set of diffusion models in latent space; (2) We introduce a semantic-aware energy model based on cross-attention, which enables semantic composition and adaptive gradient descent for text embeddings. To overcome the challenges of semantic inconsistency and motion distortion across these two spectrums, we introduce Synergistic Energy Fusion. This design allows the motion latent diffusion model to synthesize high-quality, complex motions by combining multiple energy terms corresponding to textual descriptions. Experiments show that our approach outperforms existing state-of-the-art models on various motion generation tasks, including text-to-motion generation, compositional motion generation, and multi-concept motion generation. Additionally, we demonstrate that our method can be used to extend motion datasets and improve the text-to-motion task.
扩散模型,特别是潜在扩散模型,在文本驱动的人类运动生成方面取得了显著的成功。然而,对于潜在扩散模型来说,如何将多个语义概念有效地组合成一个连贯的运动序列仍然是一个挑战。为了解决这一问题,我们提出了EnergyMoGen,它包括两种基于能量的模型谱系:一、我们将扩散模型解释为具有潜在意识的能量模型,通过在一组潜在空间中扩散模型的组合来生成运动;二、我们引入了一种基于交叉注意力的语义感知能量模型,实现了语义组合和文本嵌入的自适应梯度下降。为了克服这两个谱系之间语义不一致和运动失真的挑战,我们引入了协同能量融合设计。这一设计允许运动潜在扩散模型通过结合与文本描述相对应的多项能量术语来合成高质量、复杂的运动。实验表明,我们的方法在多种运动生成任务上超过了现有的最先进的模型,包括文本到运动生成、组合运动生成和多概念运动生成。此外,我们还证明了我们的方法可用于扩展运动数据集并改进文本到运动的任务。
论文及项目相关链接
PDF Project page: https://jiro-zhang.github.io/EnergyMoGen/
Summary
文本介绍了Diffusion模型及其在文本驱动的人类运动生成方面的显著成功,特别是在潜在扩散模型方面。然而,如何将多个语义概念组合成单一连贯的运动序列仍是潜在扩散模型的挑战。为此,文章提出了EnergyMoGen,包含两种基于能量的模型:一是将扩散模型解释为感知潜伏的基于能量的模型;二是引入基于交叉注意力的语义感知能量模型。为克服两者之间的语义不一致和运动失真挑战,文章引入了协同能量融合设计,使运动潜在扩散模型能够通过结合与文本描述相对应的多项能量术语来合成高质量、复杂的运动。实验表明,该方法在多种运动生成任务上优于现有最先进的模型,并可用于扩展运动数据集,提高文本到运动的任务性能。
Key Takeaways
- 扩散模型,特别是潜在扩散模型,在文本驱动的人类运动生成方面表现出显著成功。
- 将多个语义概念组合成单一连贯的运动序列是潜在扩散模型的挑战。
- EnergyMoGen包括两种基于能量的模型:感知潜伏的基于能量模型和语义感知能量模型。
- 交叉注意力机制被用于引入语义感知能量模型。
- 协同能量融合设计解决了语义不一致和运动失真挑战。
- 该方法在多种运动生成任务上优于现有模型。
点此查看论文截图
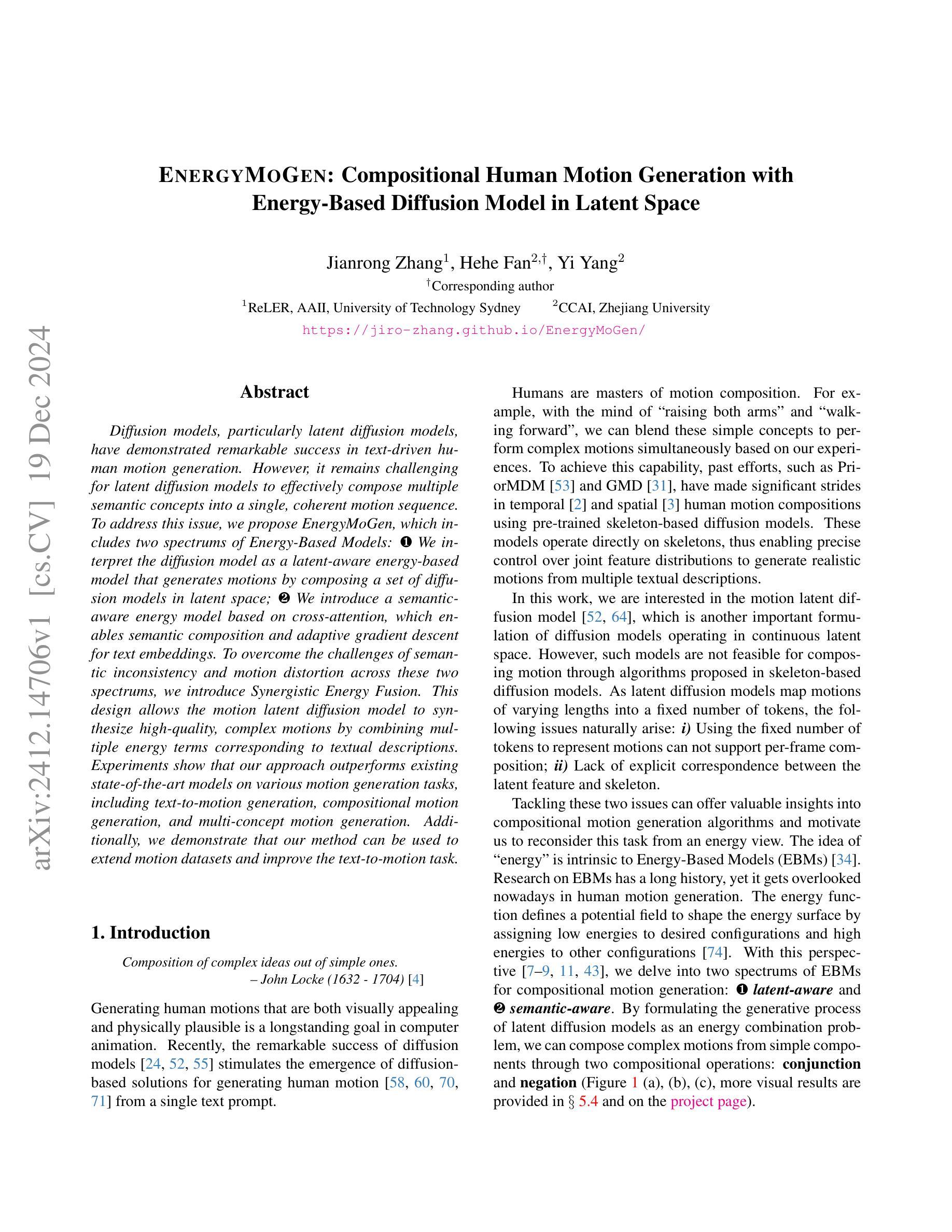
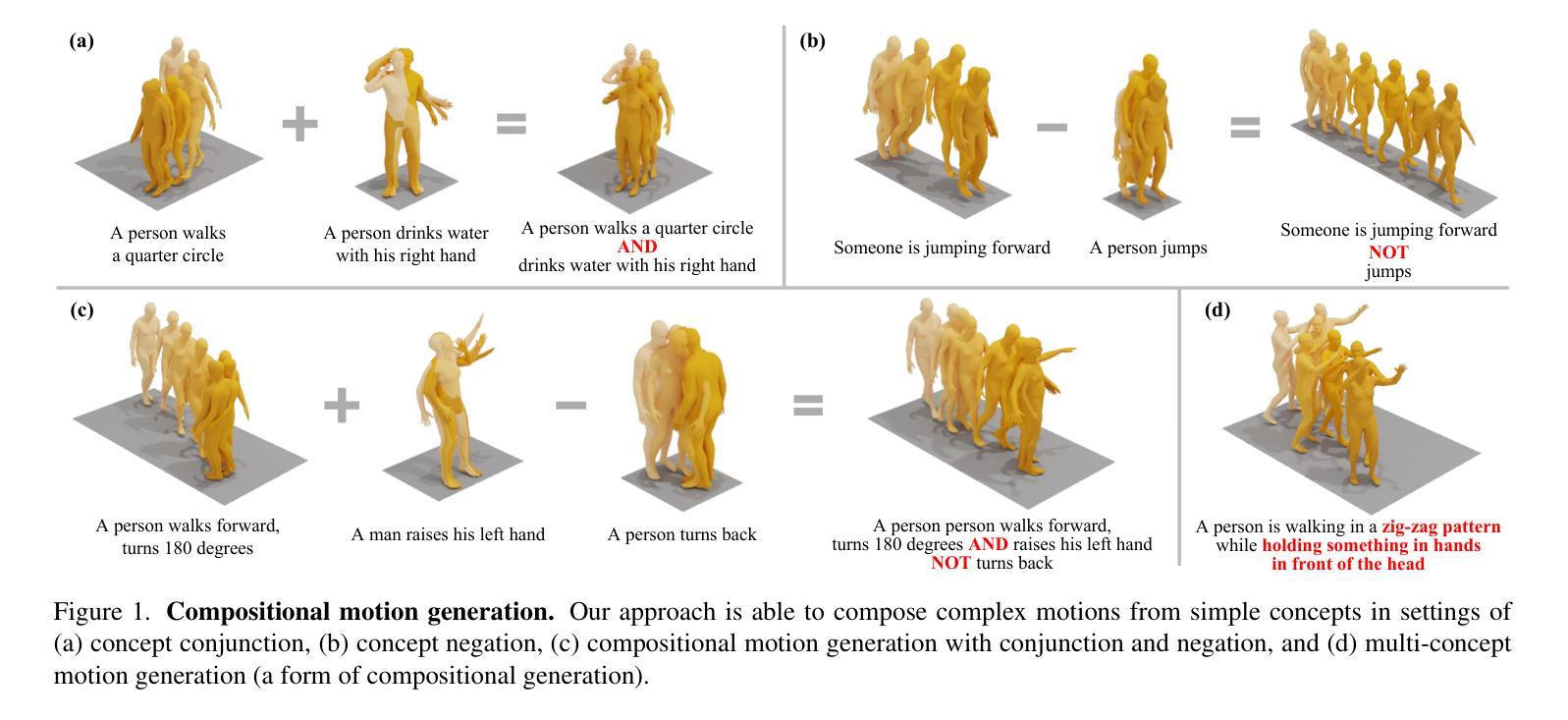
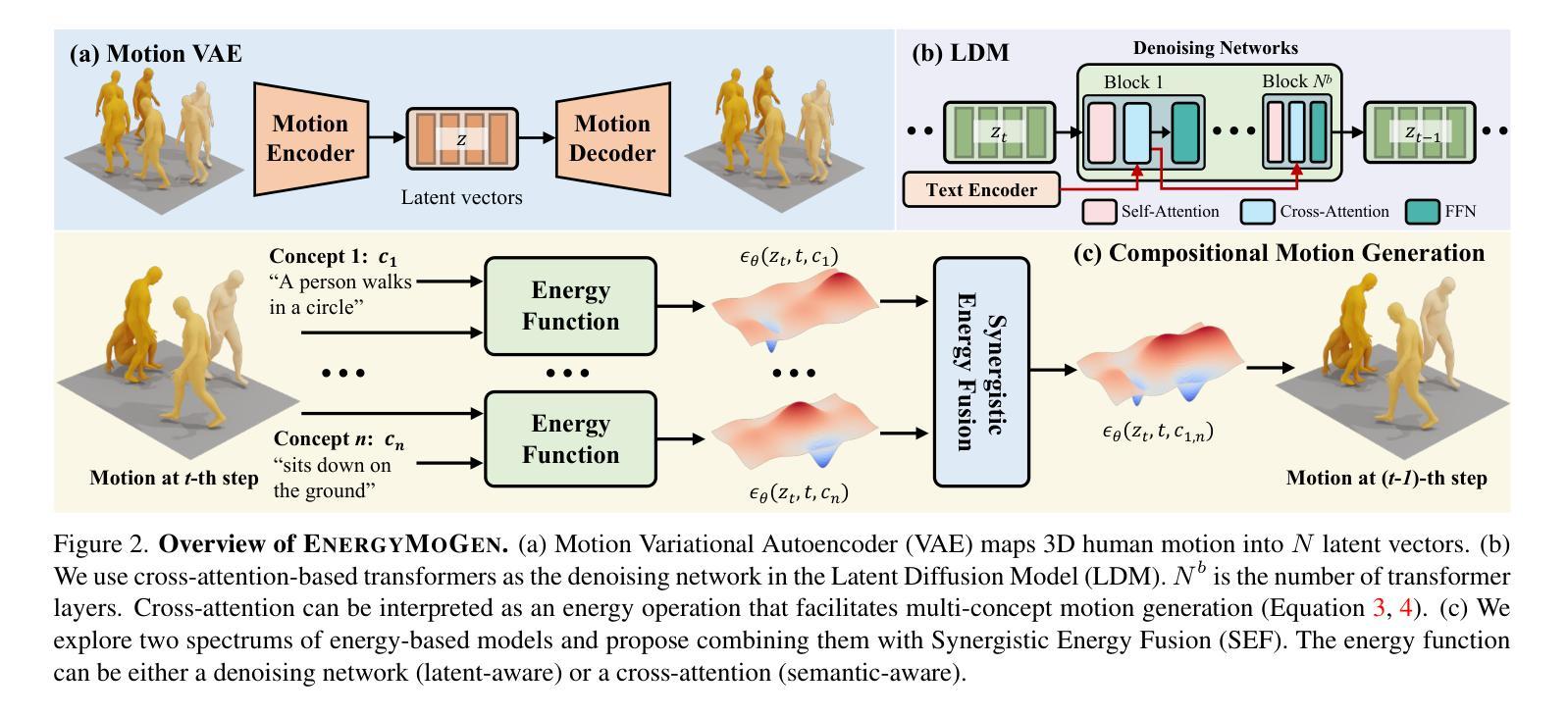
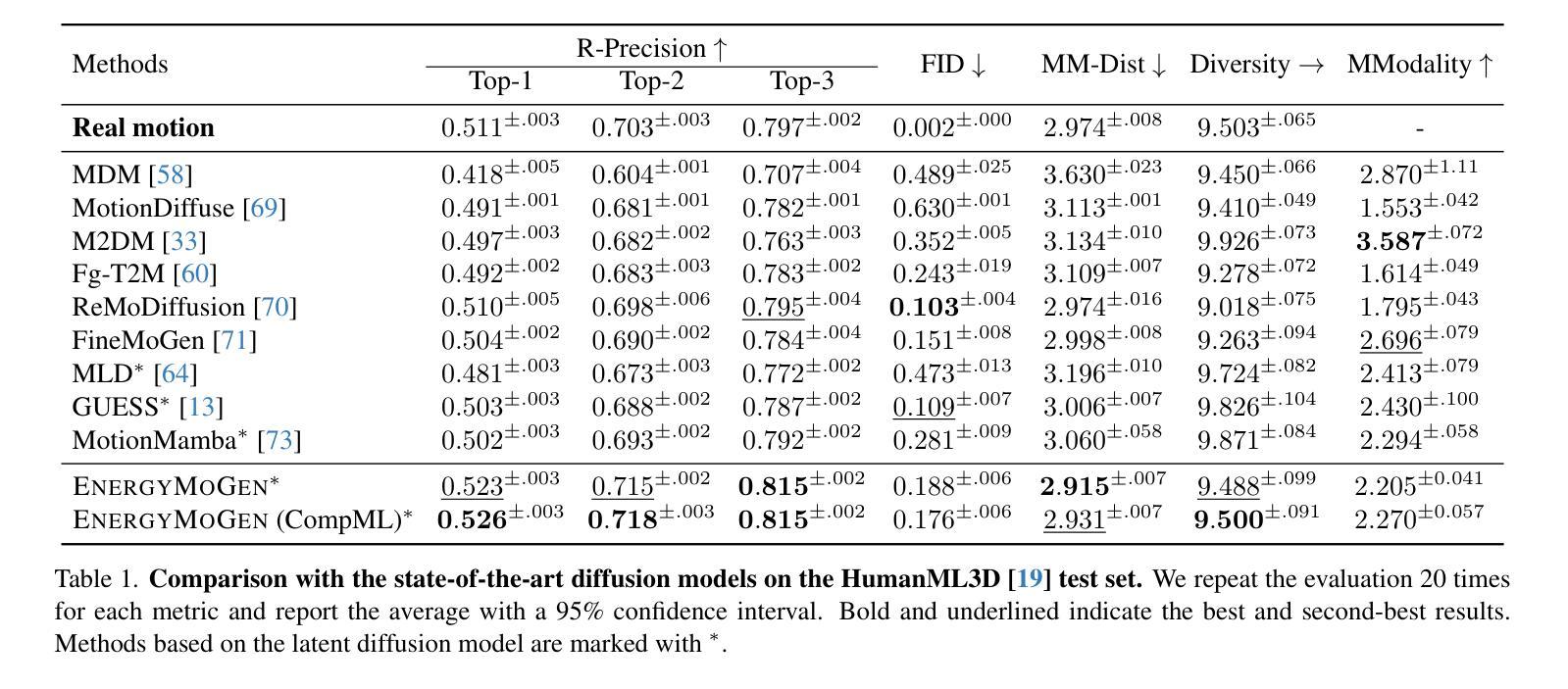
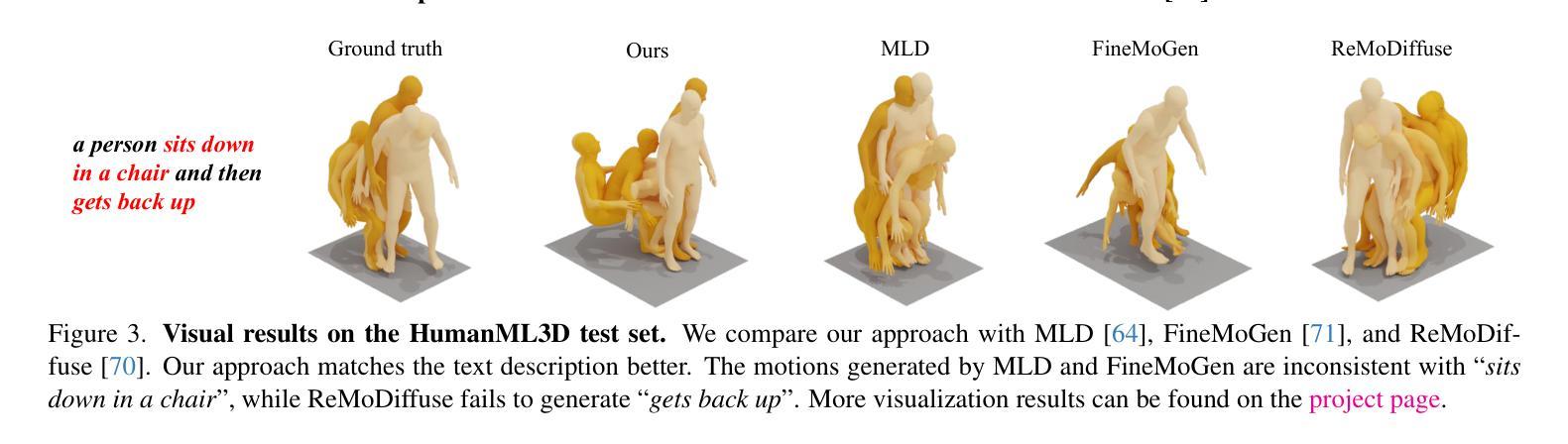
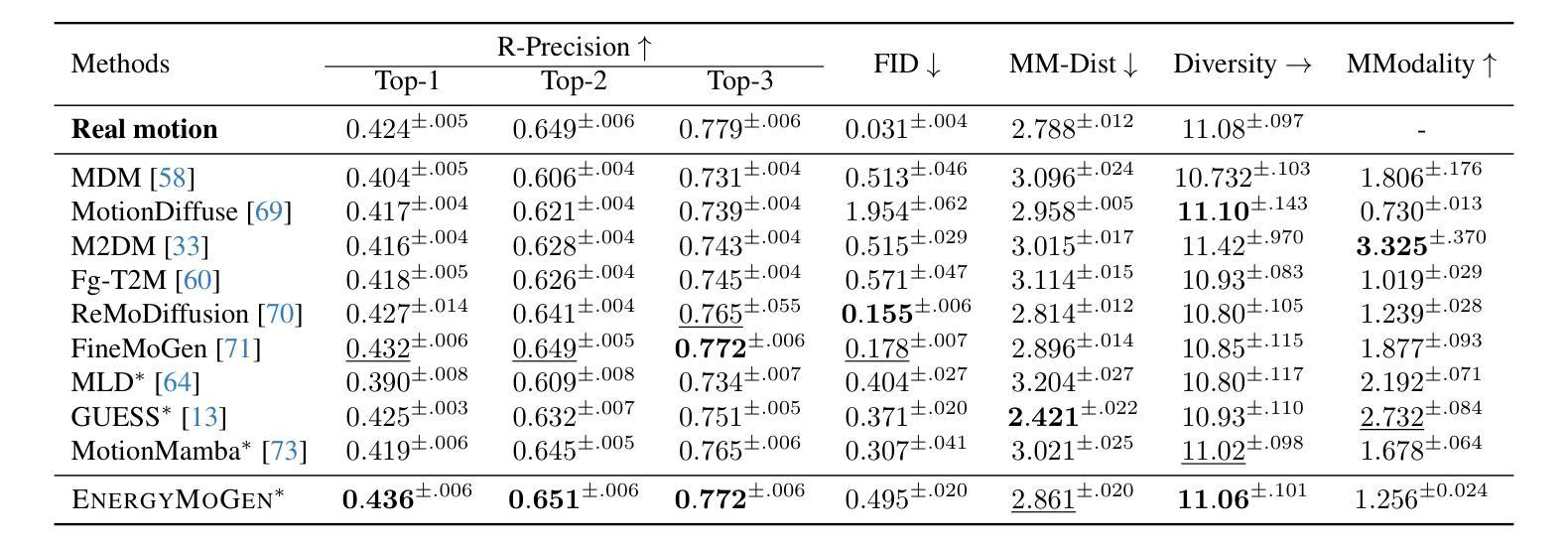