⚠️ 以下所有内容总结都来自于 大语言模型的能力,如有错误,仅供参考,谨慎使用
🔴 请注意:千万不要用于严肃的学术场景,只能用于论文阅读前的初筛!
💗 如果您觉得我们的项目对您有帮助 ChatPaperFree ,还请您给我们一些鼓励!⭐️ HuggingFace免费体验
2024-12-24 更新
Uncertainty-Guided Cross Attention Ensemble Mean Teacher for Semi-supervised Medical Image Segmentation
Authors:Meghana Karri, Amit Soni Arya, Koushik Biswas, Nicol`o Gennaro, Vedat Cicek, Gorkem Durak, Yuri S. Velichko, Ulas Bagci
This work proposes a novel framework, Uncertainty-Guided Cross Attention Ensemble Mean Teacher (UG-CEMT), for achieving state-of-the-art performance in semi-supervised medical image segmentation. UG-CEMT leverages the strengths of co-training and knowledge distillation by combining a Cross-attention Ensemble Mean Teacher framework (CEMT) inspired by Vision Transformers (ViT) with uncertainty-guided consistency regularization and Sharpness-Aware Minimization emphasizing uncertainty. UG-CEMT improves semi-supervised performance while maintaining a consistent network architecture and task setting by fostering high disparity between sub-networks. Experiments demonstrate significant advantages over existing methods like Mean Teacher and Cross-pseudo Supervision in terms of disparity, domain generalization, and medical image segmentation performance. UG-CEMT achieves state-of-the-art results on multi-center prostate MRI and cardiac MRI datasets, where object segmentation is particularly challenging. Our results show that using only 10% labeled data, UG-CEMT approaches the performance of fully supervised methods, demonstrating its effectiveness in exploiting unlabeled data for robust medical image segmentation. The code is publicly available at \url{https://github.com/Meghnak13/UG-CEMT}
本文提出了一种新型框架——不确定性引导交叉注意力集成均值教师(UG-CEMT),用于在半监督医学图像分割中实现最先进的性能。UG-CEMT结合了协同训练和知识蒸馏的优点,通过结合受视觉变压器(ViT)启发的交叉注意力集成均值教师框架(CEMT)与不确定性引导的一致性正则化和锐度感知最小化来强调不确定性。UG-CEMT在促进子网之间的高差异性的同时,维持网络架构和任务设置的一致性,从而提高了半监督性能。实验表明,与现有方法(如均值教师和交叉伪监督)相比,UG-CEMT在差异性、域泛化和医学图像分割性能方面具有明显的优势。UG-CEMT在多中心前列腺MRI和心脏MRI数据集上实现了最先进的分割结果,其中对象分割特别具有挑战性。我们的结果表明,仅使用10%的标记数据,UG-CEMT就能接近全监督方法的表现,证明了其在利用无标签数据进行稳健医学图像分割方面的有效性。相关代码已公开发布在https://github.com/Meghnak13/UG-CEMT。
论文及项目相关链接
PDF Accepted in WACV 2025
Summary
该工作提出了一种名为Uncertainty-Guided Cross Attention Ensemble Mean Teacher(UG-CEMT)的新型框架,用于在半监督医学图像分割领域实现卓越性能。UG-CEMT结合了协同训练和知识蒸馏的优点,通过将受到Vision Transformer启发的Cross-attention Ensemble Mean Teacher框架与不确定性引导的一致性正则化和关注不确定性的Sharpness-Aware Minimization相结合。UG-CEMT在促进子网之间高差异的同时,提高了半监督性能,并保持网络架构和任务设置的一致性。实验证明,与Mean Teacher和Cross-pseudo Supervision等方法相比,UG-CEMT在差异性、域通用性和医学图像分割性能方面具有明显的优势。在对象分割特别具有挑战性的多中心前列腺MRI和心脏MRI数据集上,UG-CEMT取得了最新结果。我们的结果表明,仅使用10%的标记数据,UG-CEMT就能接近全监督方法的表现,证明了其在利用未标记数据进行稳健医学图像分割方面的有效性。
Key Takeaways
- Uncertainty-Guided Cross Attention Ensemble Mean Teacher (UG-CEMT)框架结合了协同训练和知识蒸馏的优点。
- UG-CEMT利用不确定性引导的一致性正则化和Sharpness-Aware Minimization来处理不确定性。
- UG-CEMT在保持网络架构和任务设置一致性的同时,通过促进子网之间的高差异来提高半监督性能。
- 实验显示UG-CEMT在医学图像分割的差异性、域通用性和性能方面显著优于现有方法。
- UG-CEMT在多中心前列腺MRI和心脏MRI数据集上取得了最新结果,尤其在对象分割方面。
- 使用仅10%的标记数据,UG-CEMT的表现接近全监督方法。
点此查看论文截图
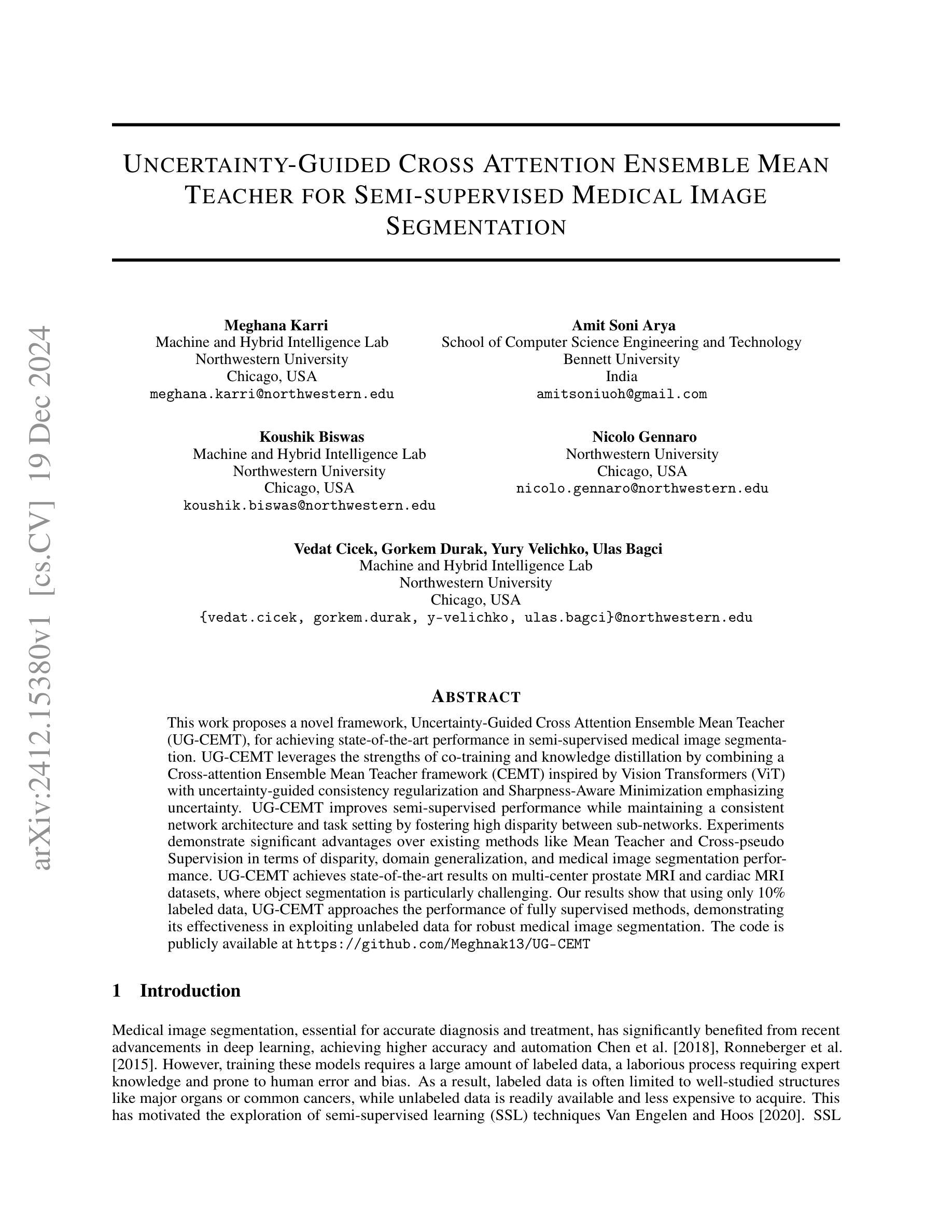
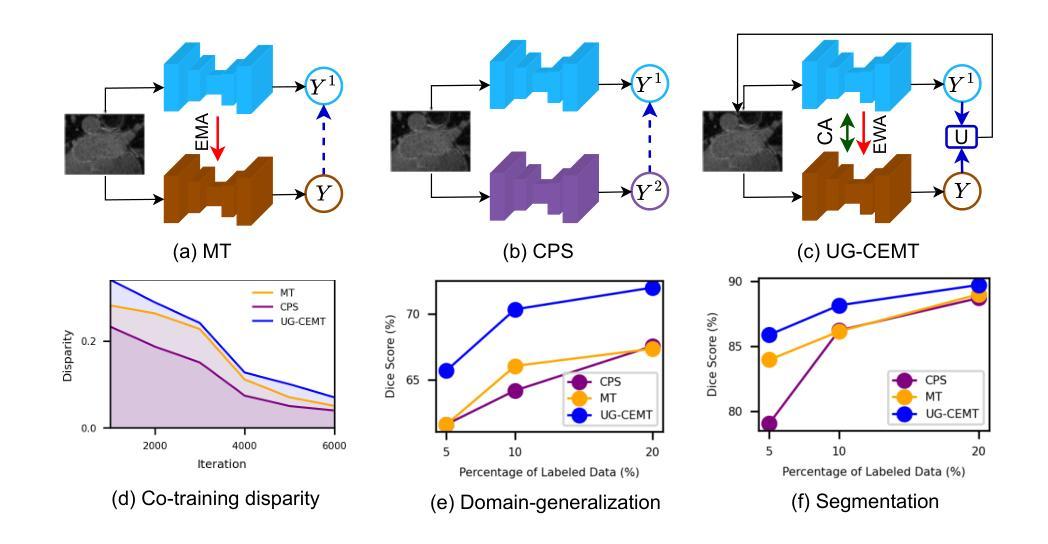
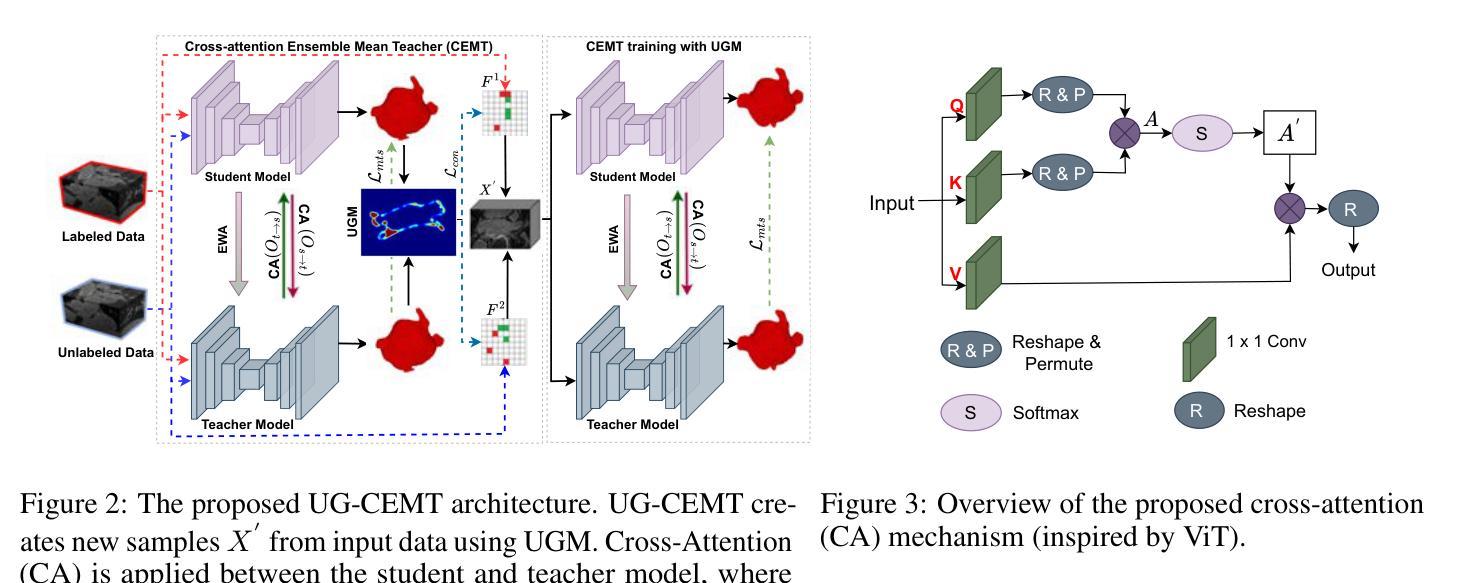
QCS:Feature Refining from Quadruplet Cross Similarity for Facial Expression Recognition
Authors:Chengpeng Wang, Li Chen, Lili Wang, Zhaofan Li, Xuebin Lv
Facial expression recognition faces challenges where labeled significant features in datasets are mixed with unlabeled redundant ones. In this paper, we introduce Cross Similarity Attention (CSA) to mine richer intrinsic information from image pairs, overcoming a limitation when the Scaled Dot-Product Attention of ViT is directly applied to calculate the similarity between two different images. Based on CSA, we simultaneously minimize intra-class differences and maximize inter-class differences at the fine-grained feature level through interactions among multiple branches. Contrastive residual distillation is utilized to transfer the information learned in the cross module back to the base network. We ingeniously design a four-branch centrally symmetric network, named Quadruplet Cross Similarity (QCS), which alleviates gradient conflicts arising from the cross module and achieves balanced and stable training. It can adaptively extract discriminative features while isolating redundant ones. The cross-attention modules exist during training, and only one base branch is retained during inference, resulting in no increase in inference time. Extensive experiments show that our proposed method achieves state-of-the-art performance on several FER datasets.
面部表情识别面临着数据集中的标记重要特征与未标记冗余特征混合的挑战。在本文中,我们引入跨相似度注意力(CSA)来从图像对中提取更丰富的内在信息,克服了当直接应用ViT的缩放点积注意力来计算两个不同图像之间的相似性时存在的局限性。基于CSA,我们通过多个分支之间的交互,在细粒度特征级别同时减小类内差异并增大类间差异。利用对比残差蒸馏将跨模块中学习到的信息转回基础网络。我们巧妙地设计了一个四分支中心对称网络,名为四元组交叉相似性(QCS),该网络缓解了跨模块产生的梯度冲突,实现了平衡稳定的训练。它可以自适应地提取判别特征,同时隔离冗余特征。跨注意力模块存在于训练过程中,而在推理过程中只保留一个基础分支,因此不会增加推理时间。大量实验表明,我们提出的方法在多个面部表情识别数据集上达到了最新技术水平。
论文及项目相关链接
Summary
面部识别技术在处理混合有未标记冗余特征的数据集时面临挑战。本研究引入跨相似度注意力(CSA)技术,从图像对中挖掘更丰富内在信息,克服直接使用ViT的缩放点积注意力在计算不同图像间相似度时的局限性。基于CSA,我们通过多分支间的交互,同时减小类内差异并最大化类间差异。本研究采用对比残差蒸馏技术,将跨模块学到的信息反馈给基础网络。设计了一种名为四元组交叉相似度(QCS)的四分支中心对称网络,缓解跨模块产生的梯度冲突,实现平衡稳定的训练。它可以自适应提取鉴别特征并隔离冗余特征。在训练过程中存在跨注意力模块,而在推理阶段仅保留一个基础分支,不会增加推理时间。实验表明,该方法在多个面部表情识别数据集上达到了最先进的性能。
Key Takeaways
- 引入跨相似度注意力(CSA)技术以处理混合特征的数据集。
- 通过多分支交互同时减小类内差异并最大化类间差异。
- 采用对比残差蒸馏技术实现跨模块信息转移至基础网络。
- 设计了四元组交叉相似度(QCS)网络以缓解梯度冲突并实现平衡稳定的训练。
- 可以自适应提取鉴别特征并隔离冗余特征。
- 跨注意力模块仅在训练阶段存在,推理阶段不增加时间成本。
点此查看论文截图
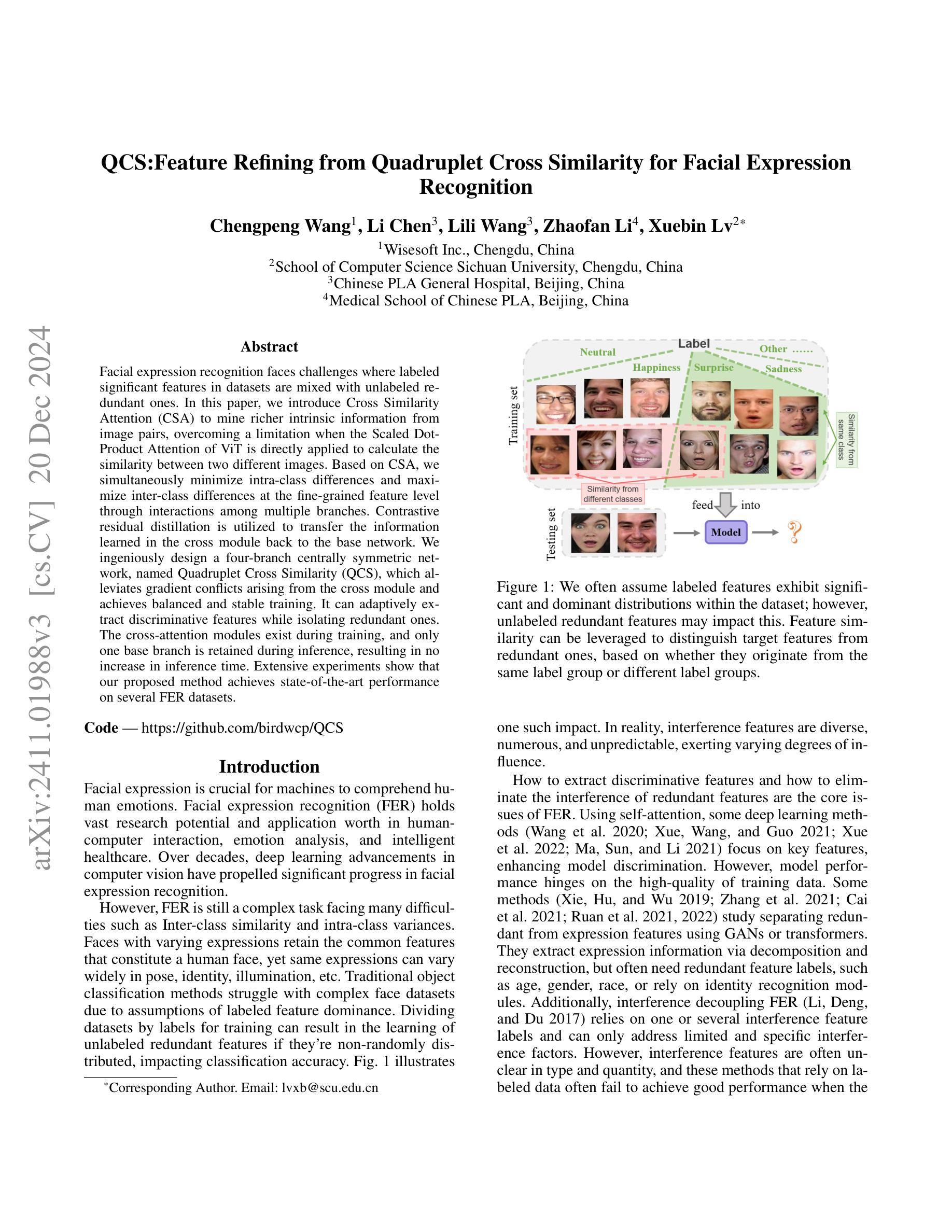
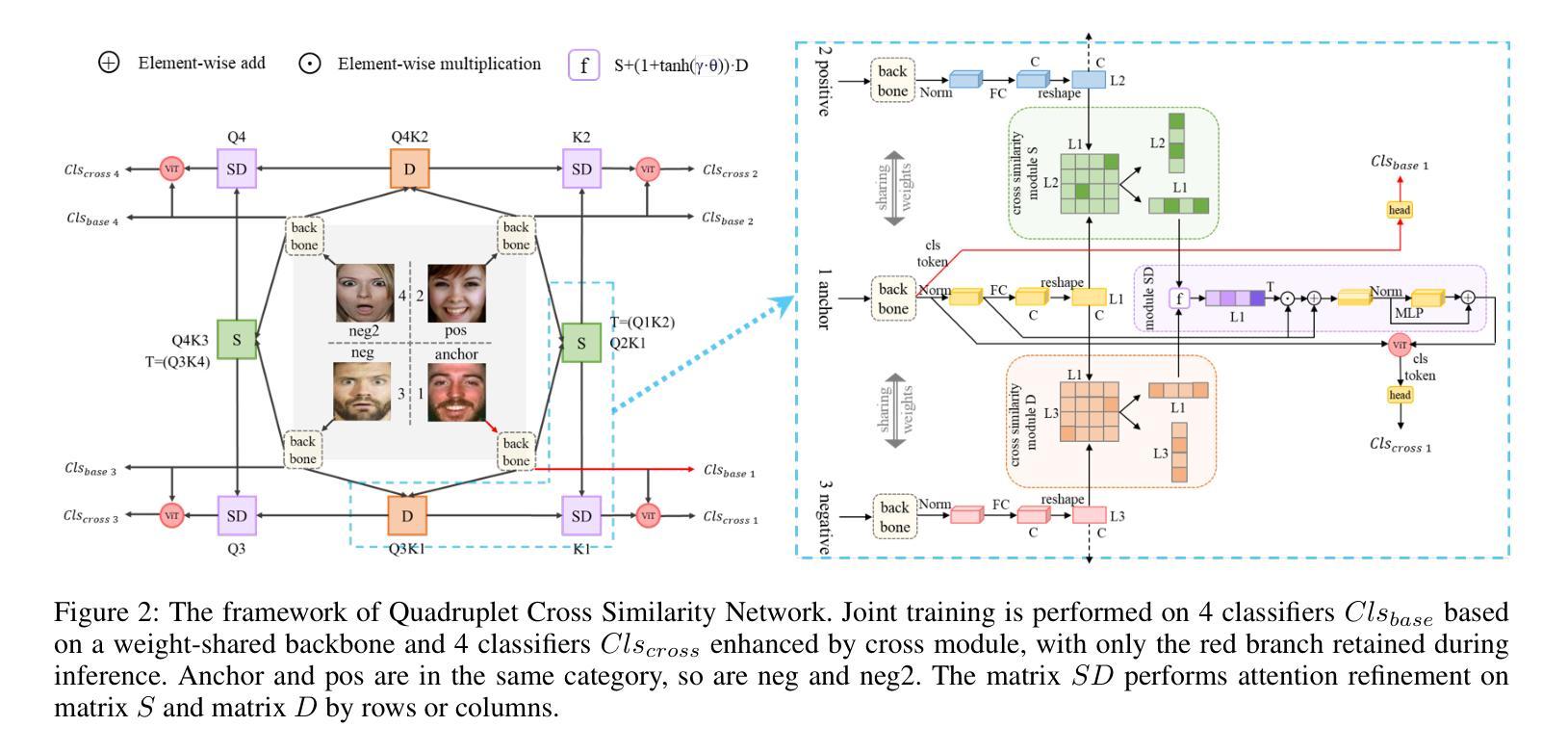
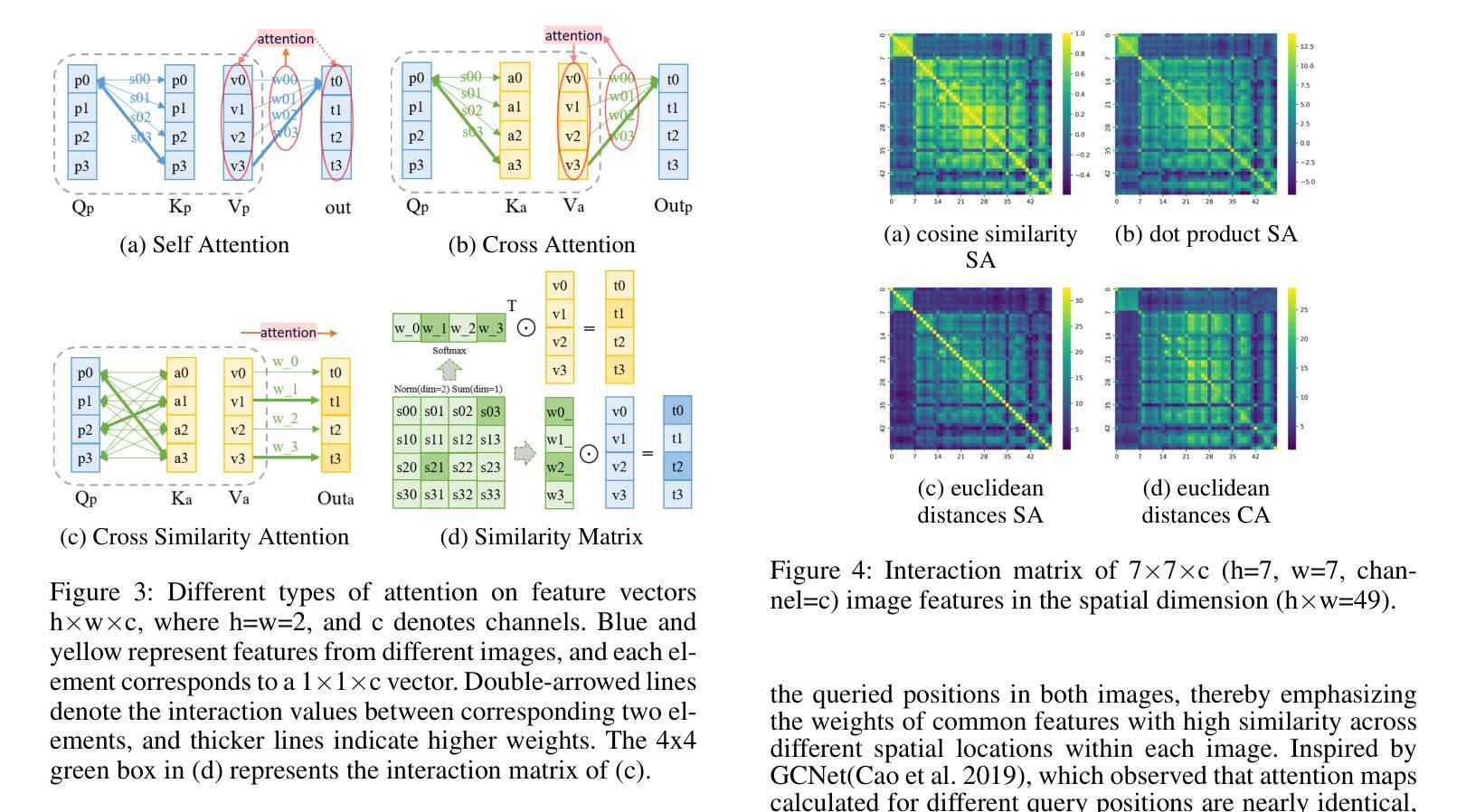
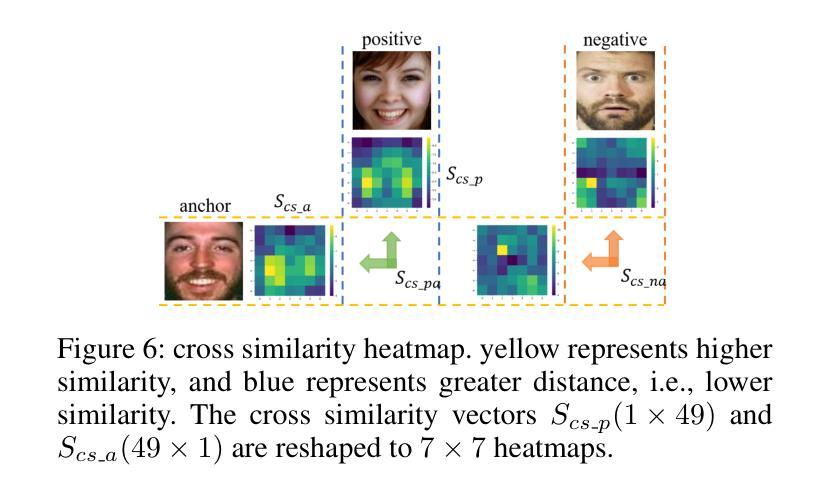
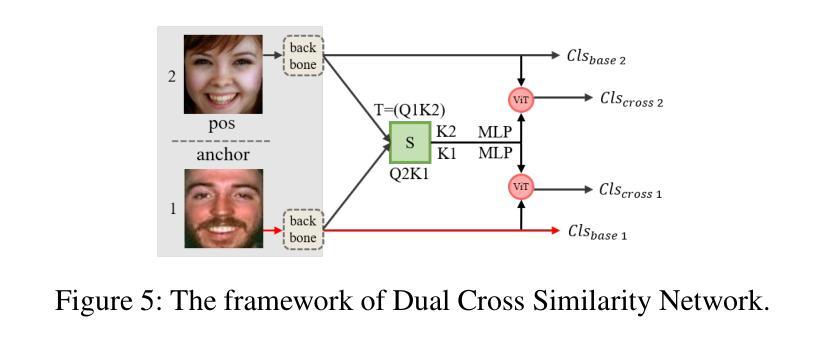
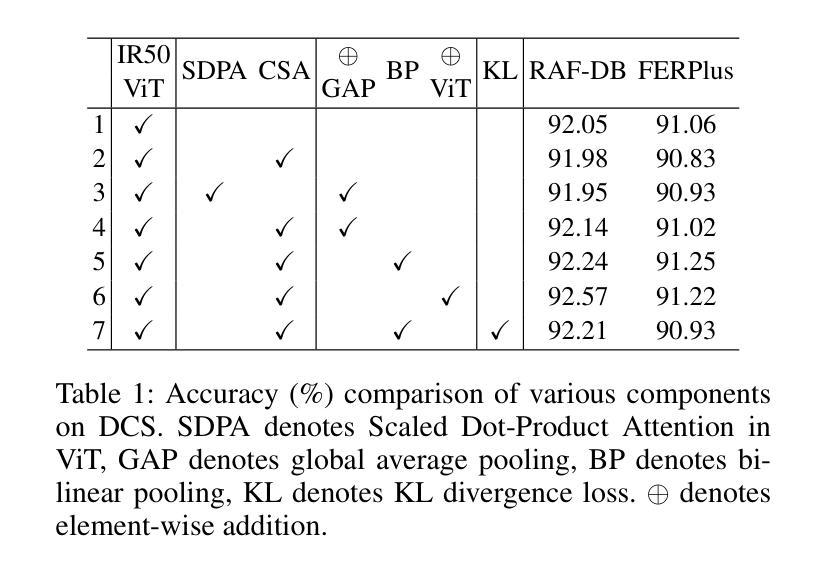
Fibottention: Inceptive Visual Representation Learning with Diverse Attention Across Heads
Authors:Ali Khaleghi Rahimian, Manish Kumar Govind, Subhajit Maity, Dominick Reilly, Christian Kümmerle, Srijan Das, Aritra Dutta
Transformer architectures such as Vision Transformers (ViT) have proven effective for solving visual perception tasks. However, they suffer from two major limitations; first, the quadratic complexity of self-attention limits the number of tokens that can be processed, and second, Transformers often require large amounts of training data to attain state-of-the-art performance. In this paper, we propose a new multi-head self-attention (MHSA) variant named Fibottention, which can replace MHSA in Transformer architectures. Fibottention is data-efficient and computationally more suitable for processing large numbers of tokens than the standard MHSA. It employs structured sparse attention based on dilated Fibonacci sequences, which, uniquely, differ across attention heads, resulting in inception-like diverse features across heads. The spacing of the Fibonacci sequences follows the Wythoff array, which minimizes the redundancy of token interactions aggregated across different attention heads, while still capturing sufficient complementary information through token pair interactions. These sparse attention patterns are unique among the existing sparse attention and lead to an $O(N \log N)$ complexity, where $N$ is the number of tokens. Leveraging only 2-6% of the elements in the self-attention heads, Fibottention embedded into popular, state-of-the-art Transformer architectures can achieve significantly improved predictive performance for domains with limited data such as image classification, video understanding, and robot learning tasks, and render reduced computational complexity. We further validated the improved diversity of feature representations resulting from different self-attention heads, and our model design against other sparse attention mechanisms.
Vision Transformer(ViT)等Transformer架构已被证明在解决视觉感知任务方面非常有效。然而,它们存在两个主要局限性:首先,自注意力的二次复杂性限制了可以处理的令牌数量;其次,为了获得最先进的性能,Transformer通常需要大量的训练数据。在本文中,我们提出了一种新的多头自注意力(MHSA)变体,名为Fibottention,它可以替代Transformer架构中的MHSA。Fibottention数据效率高,与标准MHSA相比,在计算上更适合处理大量令牌。它采用基于膨胀斐波那契序列的结构化稀疏注意力,这在不同的注意力头上是独一无二的,从而在头之间产生类似于inception的多样特征。斐波那契序列的间隔遵循维特霍夫阵列,这最小化了不同注意力头上聚合的令牌交互的冗余,同时通过令牌对交互捕获足够的互补信息。这些稀疏注意力模式在现有的稀疏注意力中是独一无二的,导致O(NlogN)的复杂性,其中N是令牌的数量。Fibottention只利用自注意力头的2-6%的元素,嵌入到流行、最先进的Transformer架构中,就可以实现对图像分类、视频理解和机器人学习任务等有限数据领域的预测性能显著提高,同时降低计算复杂度。我们进一步验证了不同自注意力头产生的特征表示多样性的改进,以及我们模型设计与其他稀疏注意力机制的优势。
论文及项目相关链接
PDF The complete implementation, including source code and evaluation scripts, is publicly available at: https://github.com/Charlotte-CharMLab/Fibottention
Summary
本文介绍了针对视觉Transformer的新多头自注意力机制——Fibottention。该机制采用基于斐波那契序列的结构化稀疏注意力,不同注意力头之间存在差异,可在处理大量令牌时提高数据效率和计算效率。Fibottention能够在仅使用自注意力头中2-6%的元素的情况下,嵌入到流行的最新Transformer架构中,在图像分类、视频理解和机器人学习任务等数据有限领域实现显著的预测性能改进,并降低计算复杂性。
Key Takeaways
- Vision Transformers (ViT) 面临两大挑战:自注意力的二次复杂度和需要大量训练数据。
- 提出了新的多头自注意力(MHSA)变体——Fibottention,适用于处理大量令牌,且数据效率高。
- Fibottention采用基于斐波那契序列的结构化稀疏注意力,实现不同注意力头之间的独特差异。
- Fibottention的自注意力模式具有O(N log N)的复杂性,其中N是令牌数量,可显著降低计算复杂性。
- 在图像分类、视频理解和机器人学习任务上,Fibottention显著提高了预测性能,特别是在数据有限的情况下。
- Fibottention的特征表示具有更高的多样性,与其他稀疏注意力机制相比具有优势。
点此查看论文截图
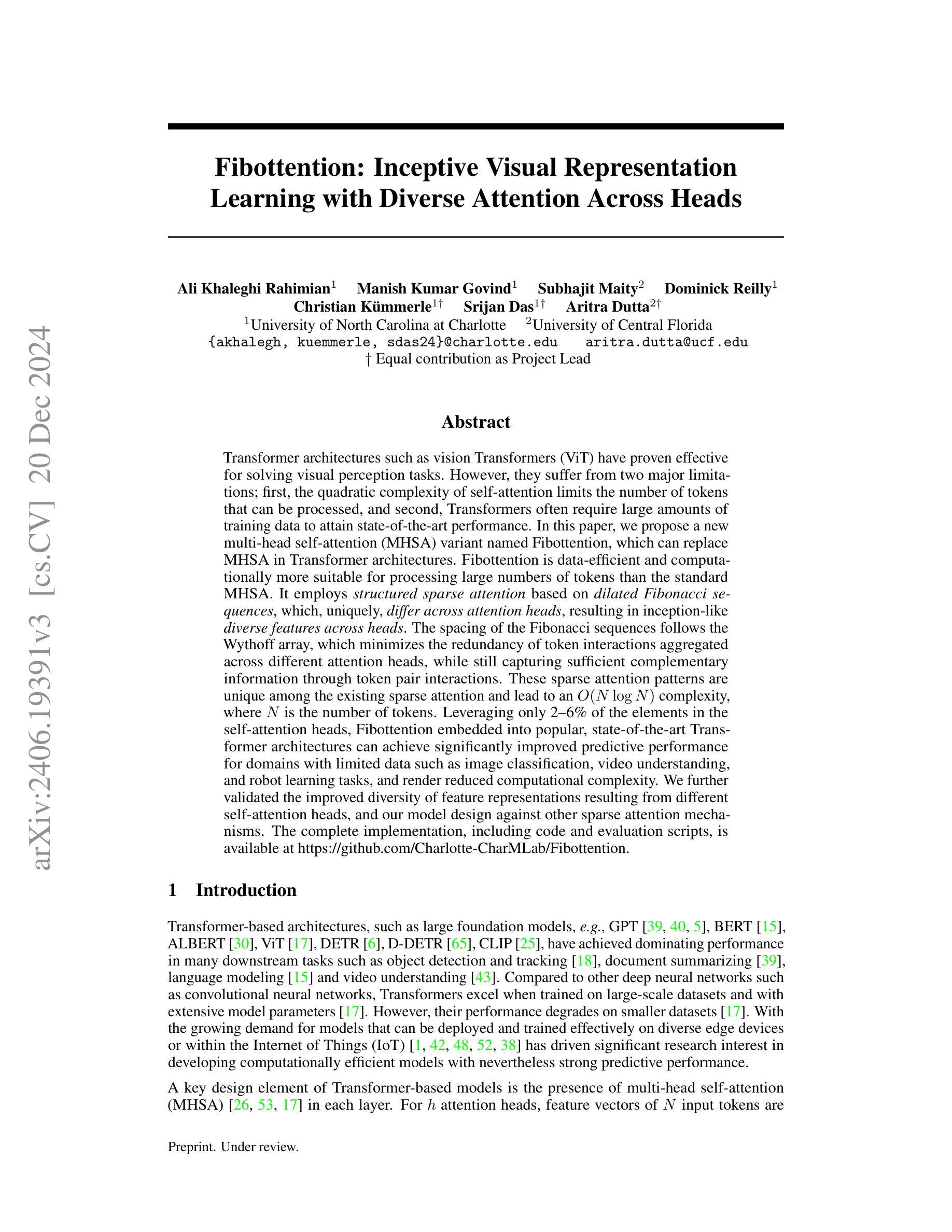
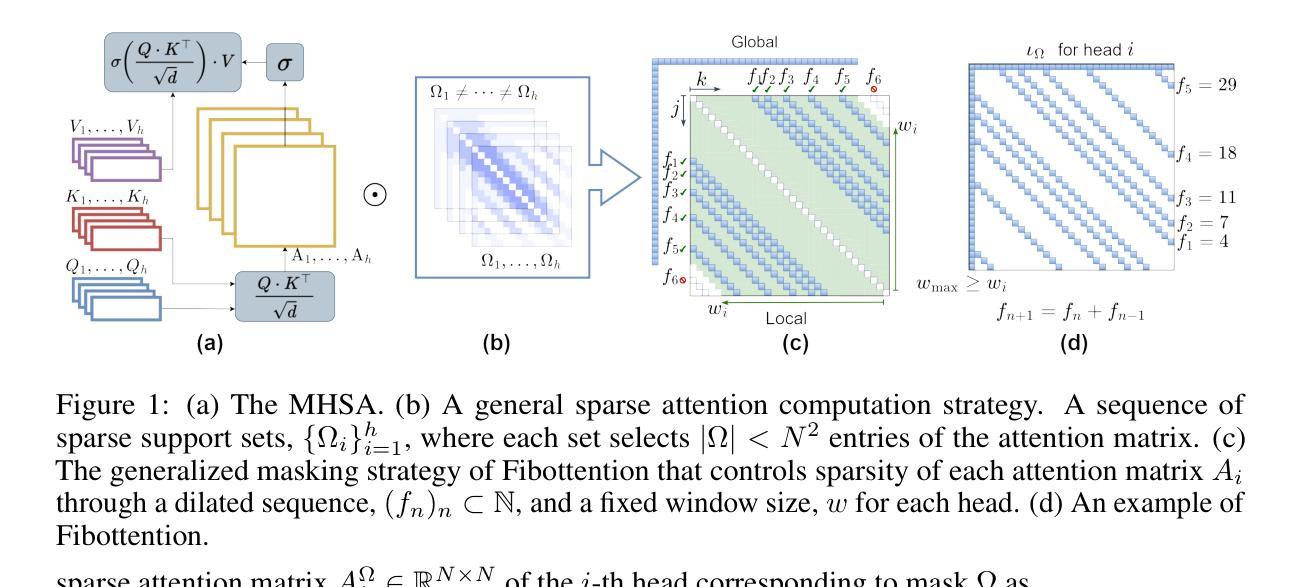
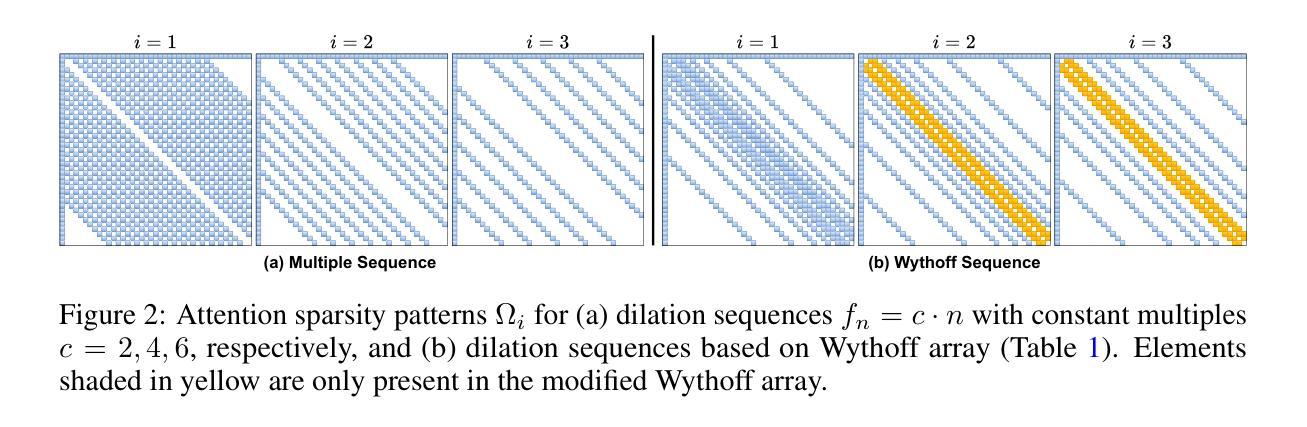
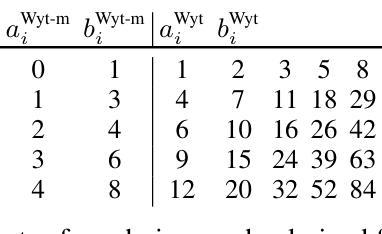