⚠️ 以下所有内容总结都来自于 大语言模型的能力,如有错误,仅供参考,谨慎使用
🔴 请注意:千万不要用于严肃的学术场景,只能用于论文阅读前的初筛!
💗 如果您觉得我们的项目对您有帮助 ChatPaperFree ,还请您给我们一些鼓励!⭐️ HuggingFace免费体验
2024-12-25 更新
FADA: Fast Diffusion Avatar Synthesis with Mixed-Supervised Multi-CFG Distillation
Authors:Tianyun Zhong, Chao Liang, Jianwen Jiang, Gaojie Lin, Jiaqi Yang, Zhou Zhao
Diffusion-based audio-driven talking avatar methods have recently gained attention for their high-fidelity, vivid, and expressive results. However, their slow inference speed limits practical applications. Despite the development of various distillation techniques for diffusion models, we found that naive diffusion distillation methods do not yield satisfactory results. Distilled models exhibit reduced robustness with open-set input images and a decreased correlation between audio and video compared to teacher models, undermining the advantages of diffusion models. To address this, we propose FADA (Fast Diffusion Avatar Synthesis with Mixed-Supervised Multi-CFG Distillation). We first designed a mixed-supervised loss to leverage data of varying quality and enhance the overall model capability as well as robustness. Additionally, we propose a multi-CFG distillation with learnable tokens to utilize the correlation between audio and reference image conditions, reducing the threefold inference runs caused by multi-CFG with acceptable quality degradation. Extensive experiments across multiple datasets show that FADA generates vivid videos comparable to recent diffusion model-based methods while achieving an NFE speedup of 4.17-12.5 times. Demos are available at our webpage http://fadavatar.github.io.
基于扩散的音频驱动说话人偶方法因其高保真、生动、富有表现力的结果而最近受到关注。然而,它们较慢的推理速度限制了实际应用。尽管针对扩散模型开发了各种蒸馏技术,但我们发现简单的扩散蒸馏方法并不能产生令人满意的结果。与教师模型相比,蒸馏模型对开放集输入图像的稳健性降低,音频和视频的关联性也降低,这削弱了扩散模型的优势。为了解决这一问题,我们提出了FADA(基于混合监督多CFG蒸馏的快速扩散人偶合成)。我们首先设计了一种混合监督损失,以利用不同质量的数据,提高模型的总体能力和稳健性。此外,我们提出了一种带有可学习标记的多CFG蒸馏,以利用音频和参考图像条件之间的关联性,减少因多CFG导致的三倍推理运行,并接受可接受的质量下降。在多个数据集上的广泛实验表明,FADA生成的视频生动,与最近的扩散模型方法相当,同时实现了4.17-12.5倍的NFE加速。演示可在我们的网页上查看:[网站链接](具体链接请替换为真实的网页链接)。
论文及项目相关链接
Summary
本文介绍了基于扩散模型的音频驱动说话虚拟人物技术,虽然该技术能够生成高质量、生动且逼真的结果,但其推理速度慢限制了实际应用。为了解决这一问题,作者提出了一种名为FADA的新方法,通过混合监督损失来提高模型能力和鲁棒性,并采用多CFG蒸馏技术来利用音频和参考图像之间的关联,从而减少推理运行时间。实验表明,FADA方法生成的虚拟视频质量出色,并且大大提高了推理速度。
Key Takeaways
- 扩散模型在音频驱动的说话虚拟人物技术中受到关注,但其推理速度慢限制了实际应用。
- 现有蒸馏技术不能满足需求,因为蒸馏模型在开放集输入图像下的鲁棒性降低,且音频与视频的关联性减弱。
- FADA方法通过混合监督损失来提高模型能力和鲁棒性,并利用多CFG蒸馏技术减少推理时间。
- FADA方法能够生成与扩散模型相当的高质量虚拟视频。
- FADA方法实现了4.17至12.5倍的推理速度提升。
- 作者提供了演示网页供公众查看其研究成果。
点此查看论文截图
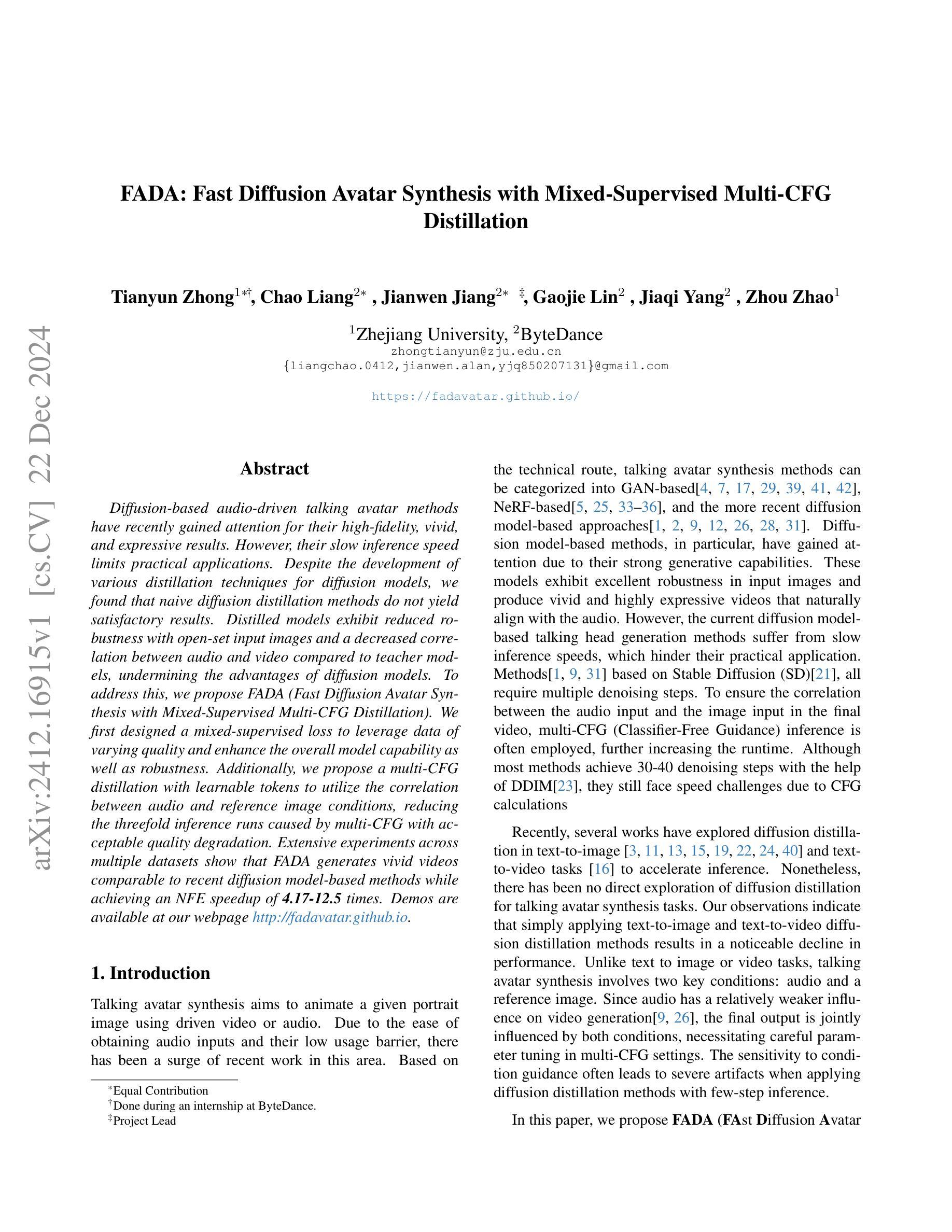
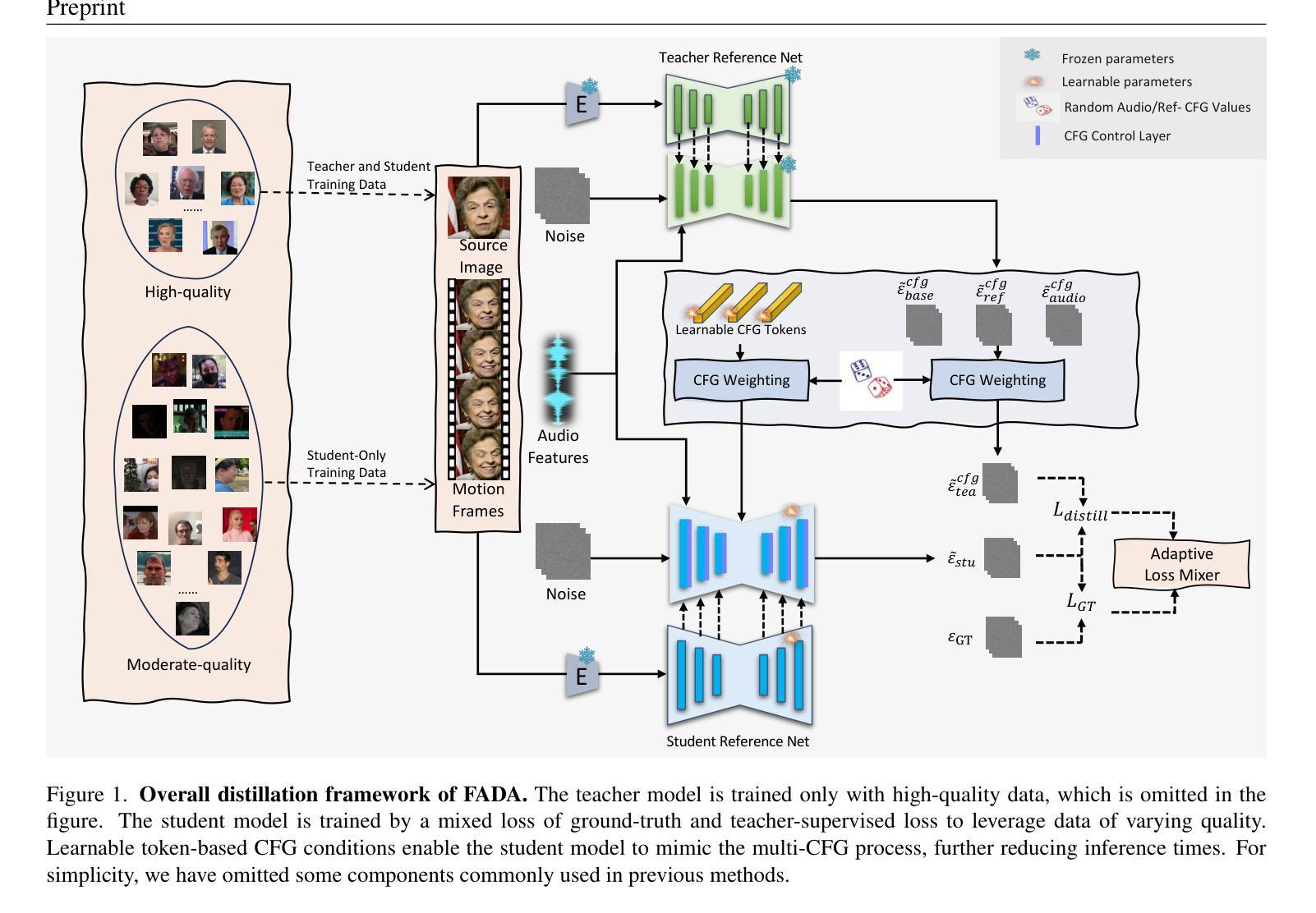
SqueezeMe: Efficient Gaussian Avatars for VR
Authors:Shunsuke Saito, Stanislav Pidhorskyi, Igor Santesteban, Forrest Iandola, Divam Gupta, Anuj Pahuja, Nemanja Bartolovic, Frank Yu, Emanuel Garbin, Tomas Simon
Gaussian Splatting has enabled real-time 3D human avatars with unprecedented levels of visual quality. While previous methods require a desktop GPU for real-time inference of a single avatar, we aim to squeeze multiple Gaussian avatars onto a portable virtual reality headset with real-time drivable inference. We begin by training a previous work, Animatable Gaussians, on a high quality dataset captured with 512 cameras. The Gaussians are animated by controlling base set of Gaussians with linear blend skinning (LBS) motion and then further adjusting the Gaussians with a neural network decoder to correct their appearance. When deploying the model on a Meta Quest 3 VR headset, we find two major computational bottlenecks: the decoder and the rendering. To accelerate the decoder, we train the Gaussians in UV-space instead of pixel-space, and we distill the decoder to a single neural network layer. Further, we discover that neighborhoods of Gaussians can share a single corrective from the decoder, which provides an additional speedup. To accelerate the rendering, we develop a custom pipeline in Vulkan that runs on the mobile GPU. Putting it all together, we run 3 Gaussian avatars concurrently at 72 FPS on a VR headset. Demo videos are at https://forresti.github.io/squeezeme.
高斯拼贴技术已经实现了具有前所未有的视觉品质的实时3D人类化身。虽然之前的方法需要一个桌面GPU来进行单个化身实时推理,但我们的目标是将多个高斯化身挤压到便携式虚拟现实头盔上,实现实时可驱动推理。我们从训练可动画高斯(基于前期工作)开始,该训练在一个用512台摄像机捕获的高质量数据集上进行。这些高斯是通过线性混合蒙皮技术控制基础高斯集来动画化,然后通过神经网络解码器进一步调整它们的高斯形态以校正外观。当在Meta Quest 3虚拟现实头盔上部署模型时,我们发现两个主要的计算瓶颈:解码器和渲染器。为了加速解码器,我们在UV空间而不是像素空间训练高斯,并将解码器蒸馏到一个单一的神经网络层。此外,我们发现高斯邻域可以从解码器中共享一个校正,这提供了额外的加速。为了加速渲染器,我们开发了一个在移动GPU上运行的Vulkan自定义管道。将所有内容整合在一起,我们在VR头盔上同时运行三个高斯化身,帧速率为每秒72帧。演示视频请访问:https://forresti.github.io/squeezeme。
论文及项目相关链接
PDF v2
Summary
实现多个实时动态高斯虚拟人头像在VR头盔上的渲染。通过训练动画化高斯模型,采用线性混合蒙皮技术并辅以神经网络修正器。为提高渲染性能,进行Vulkan中的定制管道优化并在移动GPU上运行,实现了在VR头盔上同时渲染三个高斯头像并达到72帧/秒的帧率。具体细节参见演示视频。
Key Takeaways
- 高斯Splatting技术用于创建具有前所未有的视觉质量的实时3D人类头像。
- 实现将多个高斯虚拟人头像在便携式虚拟现实头盔上进行实时驱动推理。
- 通过控制基础高斯集并使用线性混合蒙皮技术对其进行动画处理,再使用神经网络解码器进行外观校正。
- 在Meta Quest 3 VR头盔上部署模型时,发现了两个主要的计算瓶颈:解码器和渲染器。
- 通过在UV空间而不是像素空间训练高斯模型,并将解码器简化为单个神经网络层来加速解码器。
- 邻近的高斯模型可以共享解码器的单个修正,这提供了额外的加速。
点此查看论文截图
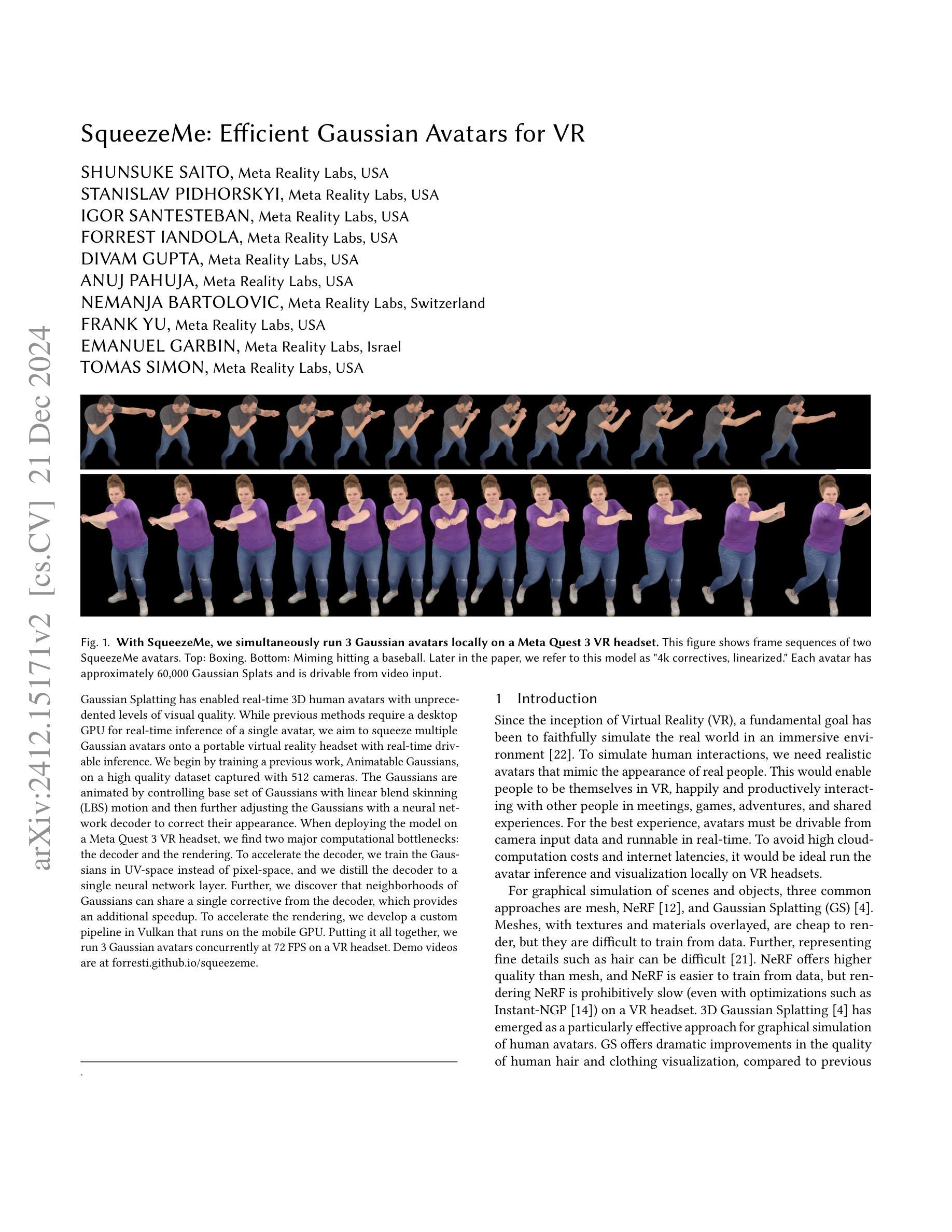
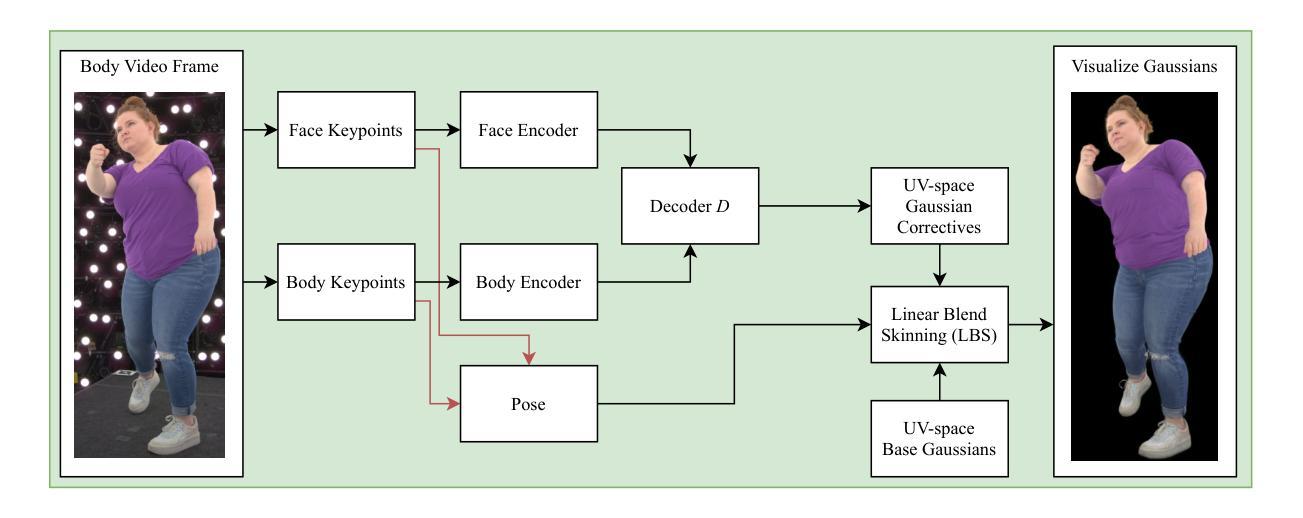
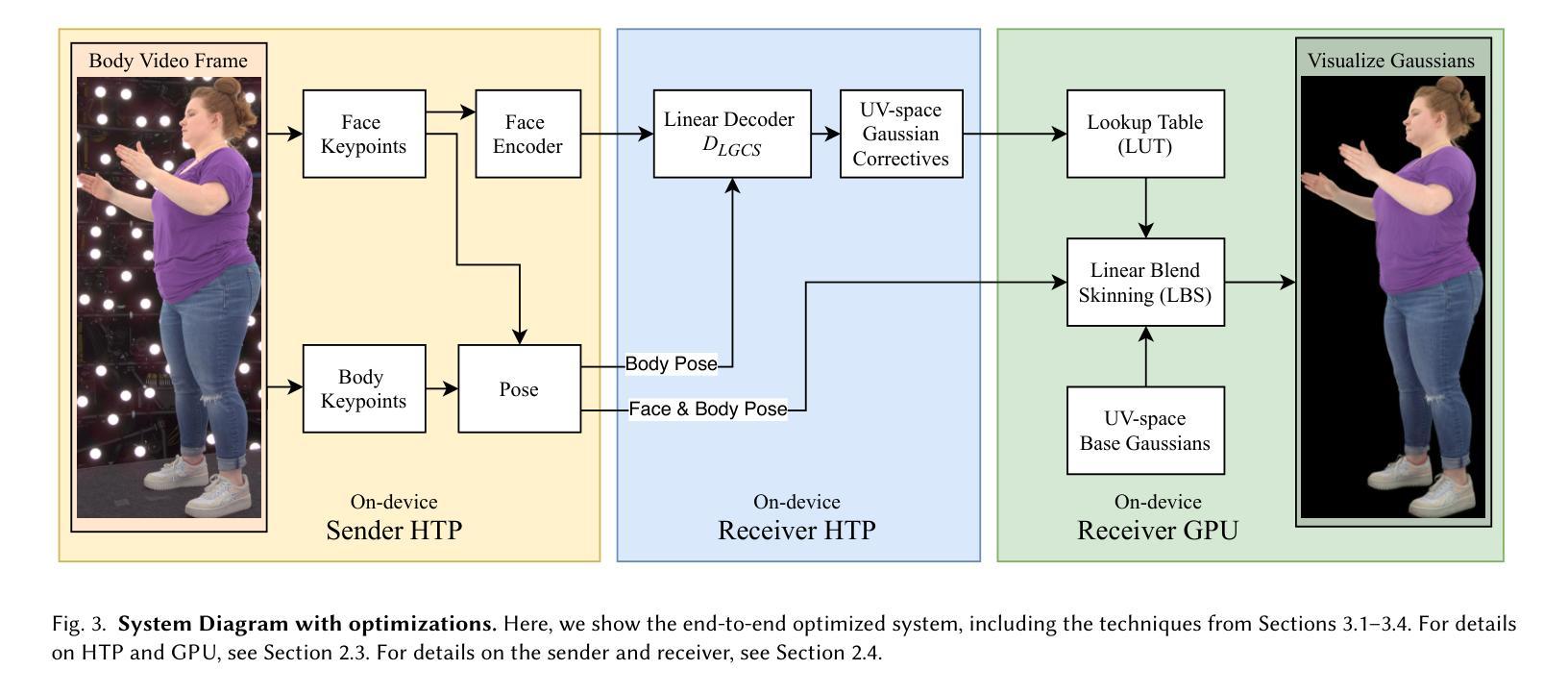
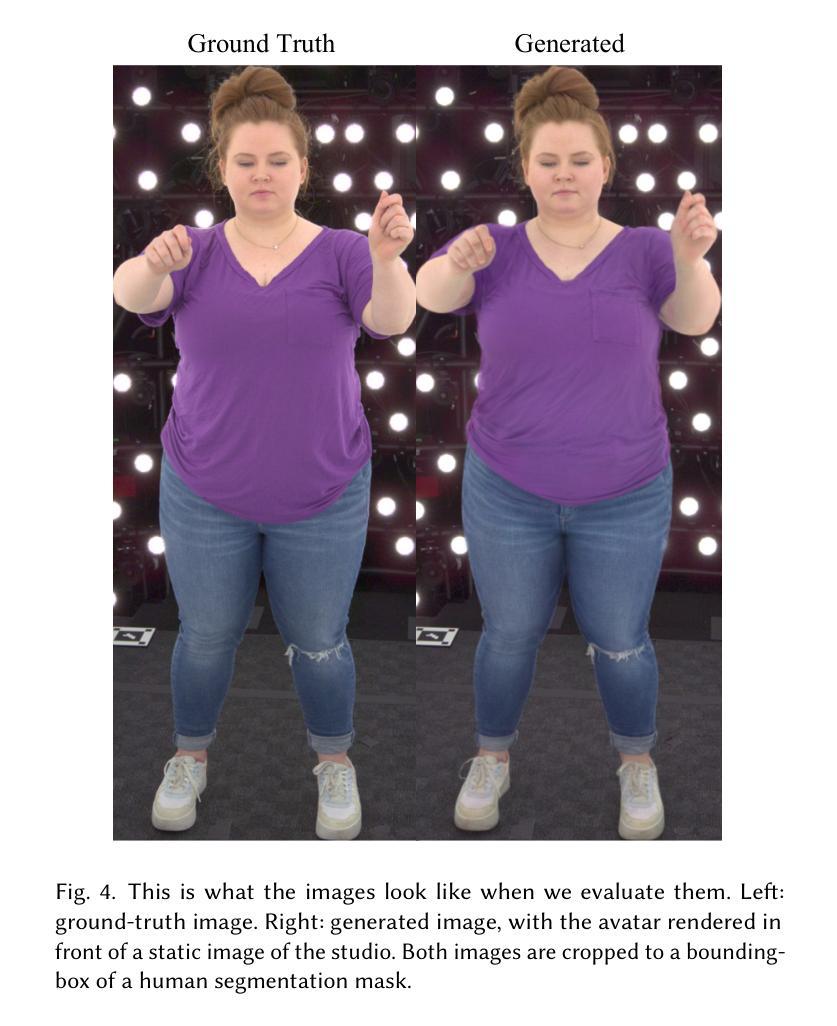
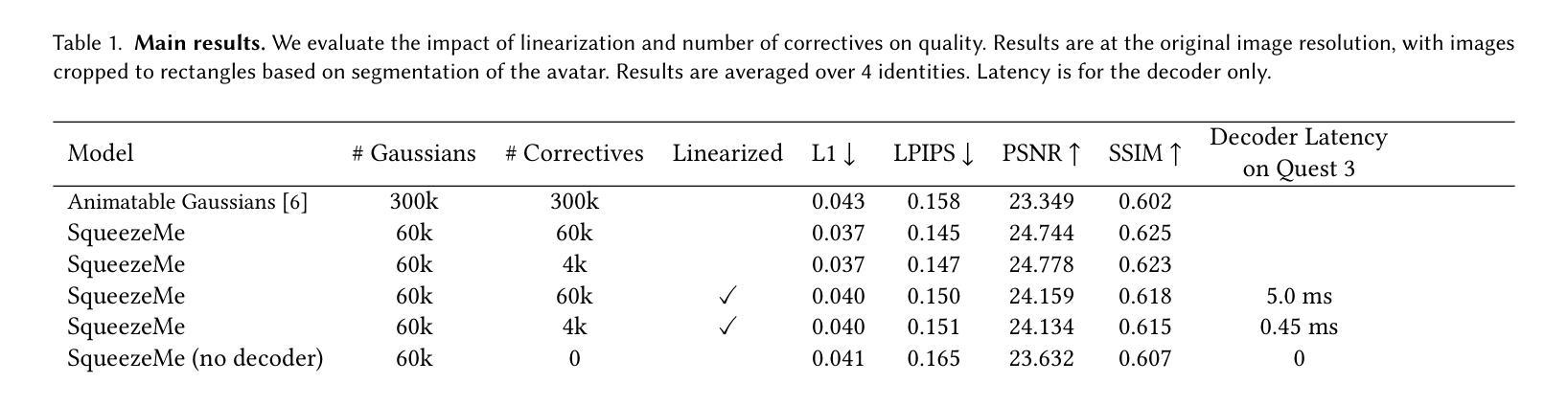
HeadStudio: Text to Animatable Head Avatars with 3D Gaussian Splatting
Authors:Zhenglin Zhou, Fan Ma, Hehe Fan, Zongxin Yang, Yi Yang
Creating digital avatars from textual prompts has long been a desirable yet challenging task. Despite the promising results achieved with 2D diffusion priors, current methods struggle to create high-quality and consistent animated avatars efficiently. Previous animatable head models like FLAME have difficulty in accurately representing detailed texture and geometry. Additionally, high-quality 3D static representations face challenges in semantically driving with dynamic priors. In this paper, we introduce \textbf{HeadStudio}, a novel framework that utilizes 3D Gaussian splatting to generate realistic and animatable avatars from text prompts. Firstly, we associate 3D Gaussians with animatable head prior model, facilitating semantic animation on high-quality 3D representations. To ensure consistent animation, we further enhance the optimization from initialization, distillation, and regularization to jointly learn the shape, texture, and animation. Extensive experiments demonstrate the efficacy of HeadStudio in generating animatable avatars from textual prompts, exhibiting appealing appearances. The avatars are capable of rendering high-quality real-time ($\geq 40$ fps) novel views at a resolution of 1024. Moreover, These avatars can be smoothly driven by real-world speech and video. We hope that HeadStudio can enhance digital avatar creation and gain popularity in the community. Code is at: https://github.com/ZhenglinZhou/HeadStudio.
从文本提示创建数字化身一直是一项令人向往但具有挑战性的任务。尽管二维扩散先验技术取得了有前景的结果,但现有方法仍然难以高效创建高质量且连贯的动画化身。像FLAME这样的以往可动画头部模型很难准确表示详细的纹理和几何形状。此外,高质量的三维静态表示在语义驱动方面面临挑战,与动态先验技术相结合时尤其如此。在本文中,我们介绍了HeadStudio这一新型框架,它利用三维高斯拼贴技术,从文本提示生成逼真且可动画的化身。首先,我们将三维高斯与可动画头部先验模型相关联,实现在高质量三维表示上的语义动画。为确保连贯的动画效果,我们从初始化、蒸馏和正则化三个方面进一步优化,以联合学习形状、纹理和动画。大量实验表明,HeadStudio在从文本来创建可动画化身方面非常有效,呈现出吸引人的外观。这些化身能够以高于或等于40帧/秒的速度呈现高质量实时新视角(分辨率为1024)。此外,这些化身可以被现实世界中的语音和视频顺畅驱动。我们希望HeadStudio能够提升数字化身的创建能力,并在社区中广受欢迎。代码地址:https://github.com/ZhenglinZhou/HeadStudio。
论文及项目相关链接
PDF 26 pages, 18 figures, accepted by ECCV 2024
Summary
本文介绍了一个名为HeadStudio的新框架,它使用3D高斯贴图技术从文本提示生成逼真且可动画的虚拟头像。该框架通过优化初始化、蒸馏和正则化过程联合学习形状、纹理和动画,确保动画的一致性。实验表明,HeadStudio能够高效地从文本提示生成可动画的虚拟头像,这些头像能够以高于40帧的速率渲染出高质量的画面,并可以由真实世界的语音和视频驱动。
Key Takeaways
- HeadStudio是一个全新的框架,使用3D高斯贴图技术生成从文本提示的动画头像。
- 该框架使用可动画的头部先验模型与3D高斯相关联,实现高质量3D表示上的语义动画。
- 通过优化初始化、蒸馏和正则化过程,确保动画的一致性。
- 实验证明HeadStudio能够高效生成可动画的虚拟头像,并具备高质量实时渲染能力。
- 这些头像能够以高于40帧的速率渲染出高质量的画面,并具备真实世界的语音和视频驱动能力。
- HeadStudio有望增强数字虚拟头像的创建,并在社区中获得普及。
点此查看论文截图
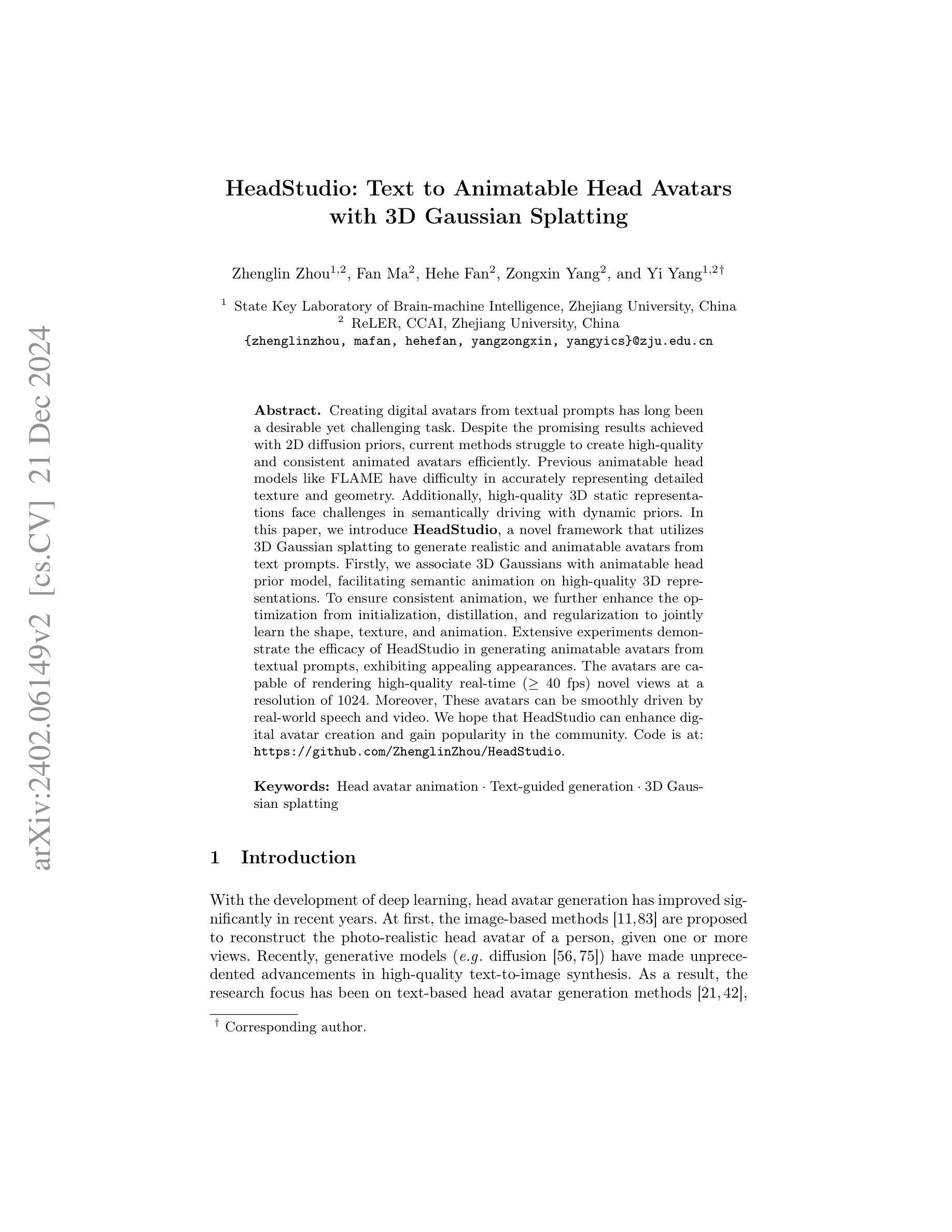
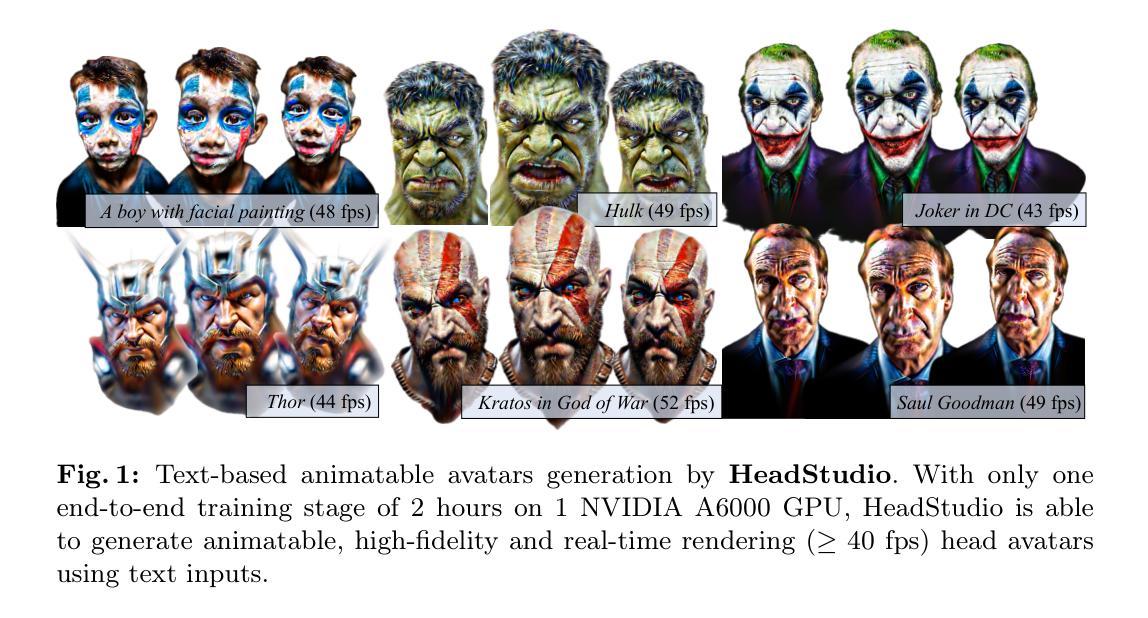
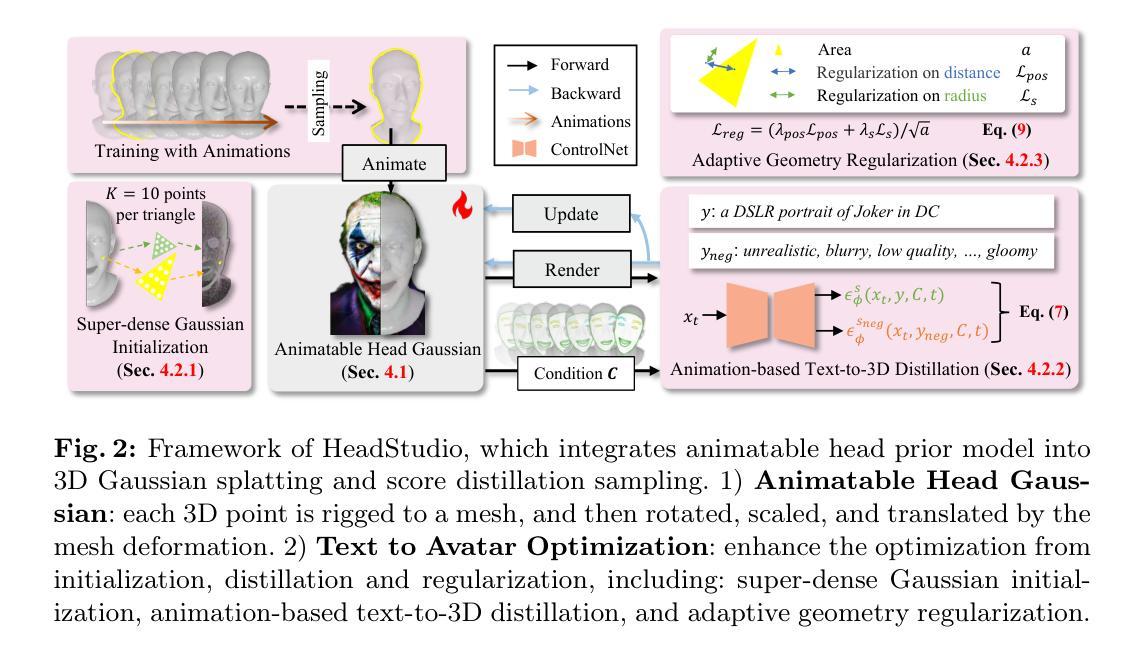