⚠️ 以下所有内容总结都来自于 大语言模型的能力,如有错误,仅供参考,谨慎使用
🔴 请注意:千万不要用于严肃的学术场景,只能用于论文阅读前的初筛!
💗 如果您觉得我们的项目对您有帮助 ChatPaperFree ,还请您给我们一些鼓励!⭐️ HuggingFace免费体验
2024-12-25 更新
PINN-EMFNet: PINN-based and Enhanced Multi-Scale Feature Fusion Network for Breast Ultrasound Images Segmentation
Authors:Jiajun Ding, Beiyao Zhu, Wenjie Wang, Shurong Zhang, Dian Zhua, Zhao Liua
With the rapid development of deep learning and computer vision technologies, medical image segmentation plays a crucial role in the early diagnosis of breast cancer. However, due to the characteristics of breast ultrasound images, such as low contrast, speckle noise, and the highly diverse morphology of tumors, existing segmentation methods exhibit significant limitations in terms of accuracy and robustness. To address these challenges, this study proposes a PINN-based and Enhanced Multi-Scale Feature Fusion Network. The network introduces a Hierarchical Aggregation Encoder in the backbone, which efficiently integrates and globally models multi-scale features through several structural innovations and a novel PCAM module. In the decoder section, a Multi-Scale Feature Refinement Decoder is employed, which, combined with a Multi-Scale Supervision Mechanism and a correction module, significantly improves segmentation accuracy and adaptability. Additionally, the loss function incorporating the PINN mechanism introduces physical constraints during the segmentation process, enhancing the model’s ability to accurately delineate tumor boundaries. Comprehensive evaluations on two publicly available breast ultrasound datasets, BUSIS and BUSI, demonstrate that the proposed method outperforms previous segmentation approaches in terms of segmentation accuracy and robustness, particularly under conditions of complex noise and low contrast, effectively improving the accuracy and reliability of tumor segmentation. This method provides a more precise and robust solution for computer-aided diagnosis of breast ultrasound images.
随着深度学习和计算机视觉技术的快速发展,医学图像分割在乳腺癌的早期诊断中发挥着至关重要的作用。然而,由于乳腺超声图像的特性,如低对比度、斑点噪声和肿瘤形态的高度多样性,现有的分割方法在准确性和稳健性方面存在显著局限性。为了解决这些挑战,本研究提出了一种基于PINN的增强多尺度特征融合网络。该网络在主干中引入分层聚合编码器,通过若干结构创新和新型PCAM模块,有效地整合并全局建模多尺度特征。在解码器部分,采用了多尺度特征细化解码器,结合多尺度监督机制和校正模块,显著提高了分割的准确性和适应性。此外,损失函数结合了PINN机制,在分割过程中引入了物理约束,增强了模型精确描绘肿瘤边界的能力。在公开可用的两个乳腺超声数据集BUSIS和BUSI上的综合评估表明,所提出的方法在分割准确性和稳健性方面优于先前的分割方法,特别是在复杂噪声和低对比度条件下,有效提高肿瘤分割的准确性和可靠性。该方法为计算机辅助诊断乳腺超声图像提供了更精确和稳健的解决方案。
论文及项目相关链接
Summary
乳腺超声图像分割对乳腺癌的早期诊断至关重要。本研究针对乳腺超声图像特性,提出一种基于PINN的增强多尺度特征融合网络,通过引入层次聚合编码器和多尺度特征细化解码器,提高分割精度和适应性。该方法在公开数据集上表现出优异性能,为计算机辅助诊断乳腺超声图像提供更精确、稳健的解决方案。
Key Takeaways
- 医学图像分割在乳腺癌早期诊断中起关键作用。
- 乳腺超声图像特性如低对比度、斑点噪声和肿瘤形态多样性给分割带来挑战。
- 研究提出一种基于PINN的增强多尺度特征融合网络,以应对这些挑战。
- 网络引入层次聚合编码器和多尺度特征细化解码器,通过结构创新和PCAM模块提高分割精度和适应性。
- PINN机制的损失函数在分割过程中引入物理约束,提高模型准确勾勒肿瘤边界的能力。
- 在公开数据集上的综合评价显示,该方法在分割精度和稳健性方面优于先前方法,特别是在复杂噪声和低对比度条件下。
点此查看论文截图
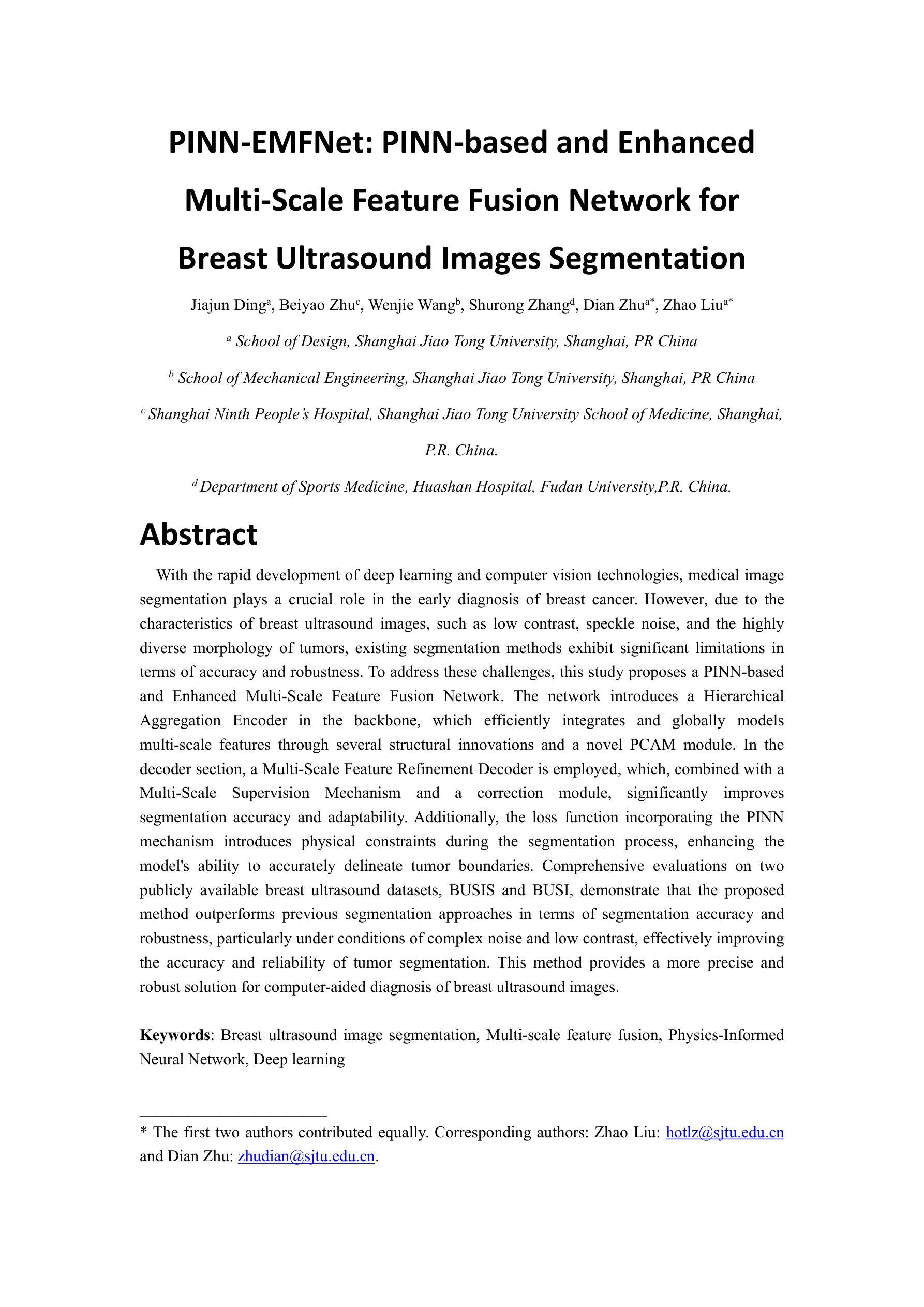
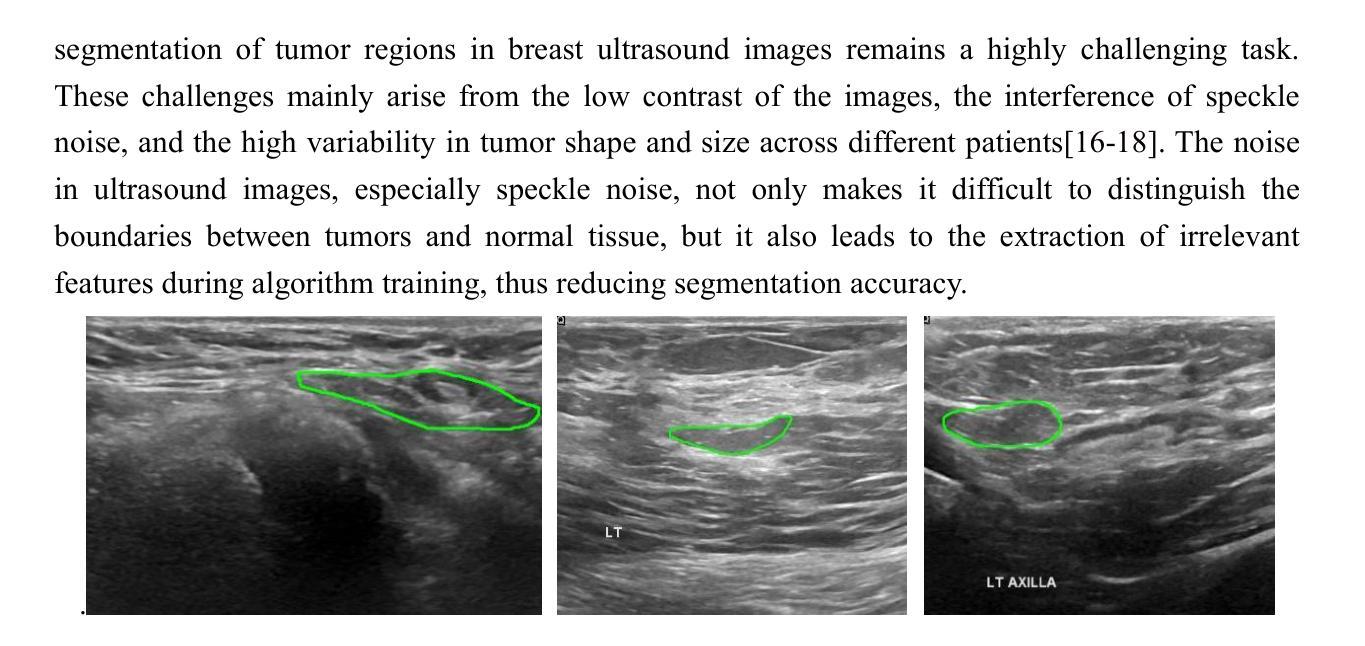
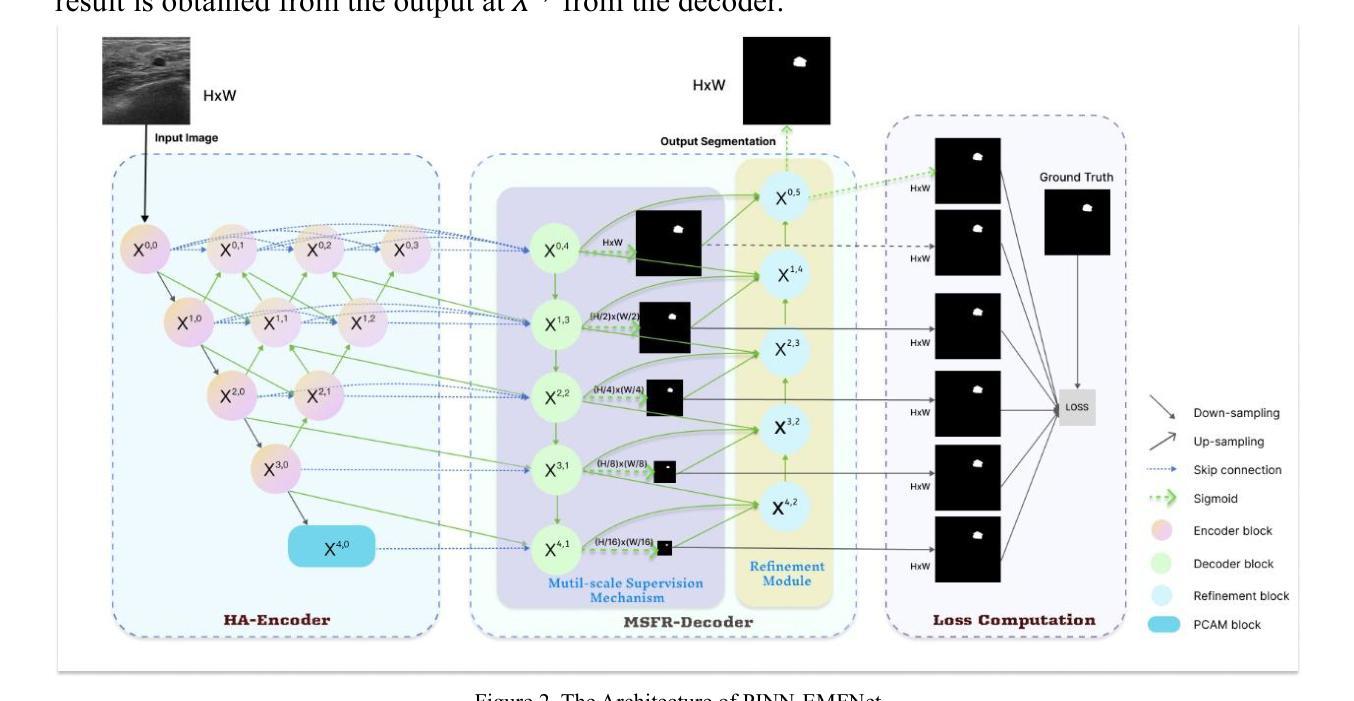