⚠️ 以下所有内容总结都来自于 大语言模型的能力,如有错误,仅供参考,谨慎使用
🔴 请注意:千万不要用于严肃的学术场景,只能用于论文阅读前的初筛!
💗 如果您觉得我们的项目对您有帮助 ChatPaperFree ,还请您给我们一些鼓励!⭐️ HuggingFace免费体验
2024-12-25 更新
RF-GML: Reference-Free Generative Machine Listener
Authors:Arijit Biswas, Guanxin Jiang
This paper introduces a novel reference-free (RF) audio quality metric called the RF-Generative Machine Listener (RF-GML), designed to evaluate coded mono, stereo, and binaural audio at a 48 kHz sample rate. RF-GML leverages transfer learning from a state-of-the-art full-reference (FR) Generative Machine Listener (GML) with minimal architectural modifications. The term “generative” refers to the model’s ability to generate an arbitrary number of simulated listening scores. Unlike existing RF models, RF-GML accurately predicts subjective quality scores across diverse content types and codecs. Extensive evaluations demonstrate its superiority in rating unencoded audio and distinguishing different levels of coding artifacts. RF-GML’s performance and versatility make it a valuable tool for coded audio quality assessment and monitoring in various applications, all without the need for a reference signal.
本文介绍了一种新型的无参考(RF)音频质量指标,称为RF生成机器监听器(RF-GML),旨在评估编码的单声道、立体声和双声道音频在48kHz采样率时的质量。RF-GML通过利用前沿的全参考(FR)生成机器监听器(GML)的迁移学习,仅进行少量的架构修改。术语“生成式”指的是模型能够生成任意数量的模拟监听分数的能力。与现有的RF模型不同,RF-GML能够准确预测各种内容类型和编码格式的的主观质量分数。广泛的评估表明,它在评估未编码的音频和区分不同级别的编码伪影方面具有优越性。RF-GML的性能和通用性使其成为各种应用中编码音频质量评估和监控的重要工具,并且无需参考信号。
论文及项目相关链接
PDF Accepted to 50th IEEE International Conference on Acoustics, Speech and Signal Processing (ICASSP), Hyderabad, India, 06-11 April 2025
Summary
RF-GML是一种无需参考的音频质量评估方法,利用迁移学习技术从全参考GML模型中发展而来,能够评估编码的单声道、立体声和双声道音频在48kHz采样率下的质量。其能够准确预测不同内容和编码方式下的主观质量得分,且性能优越,无需参考信号即可进行编码音频的质量评估和监控。
Key Takeaways
- RF-GML是一种新颖的无需参考的音频质量评估方法。
- RF-GML基于迁移学习技术,来源于全参考的GML模型。
- RF-GML能够评估编码的单声道、立体声和双声道音频的质量。
- RF-GML可以准确预测不同内容和编码方式下的主观质量得分。
- RF-GML在评估未编码音频和区分不同级别的编码伪影方面表现出卓越的性能。
- RF-GML具有广泛的应用价值,适用于多种编码音频的质量评估和监控。
点此查看论文截图
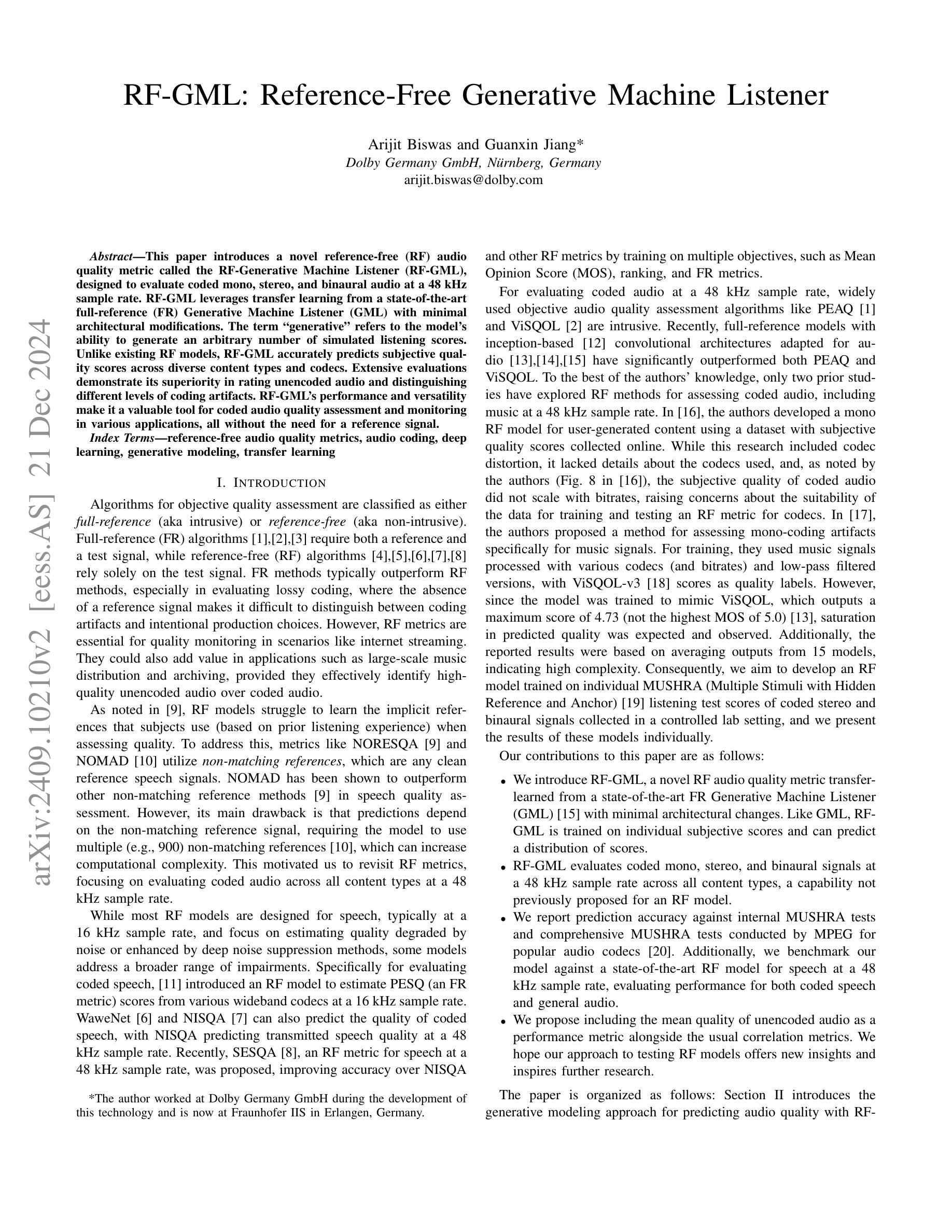
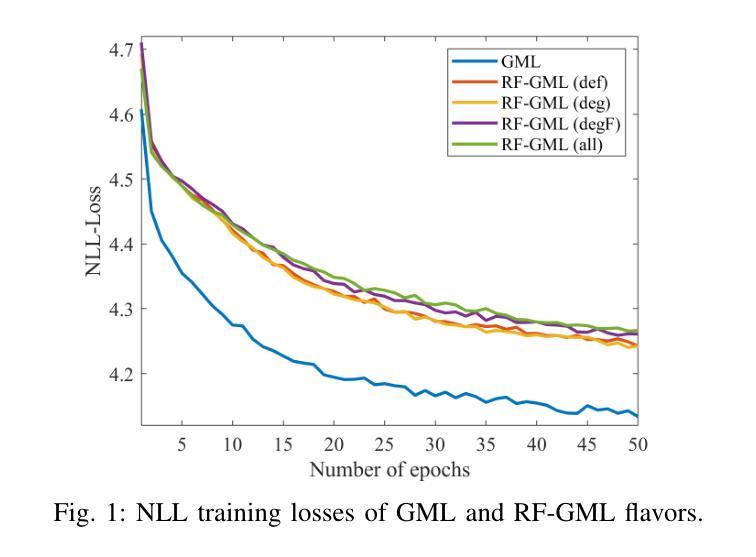
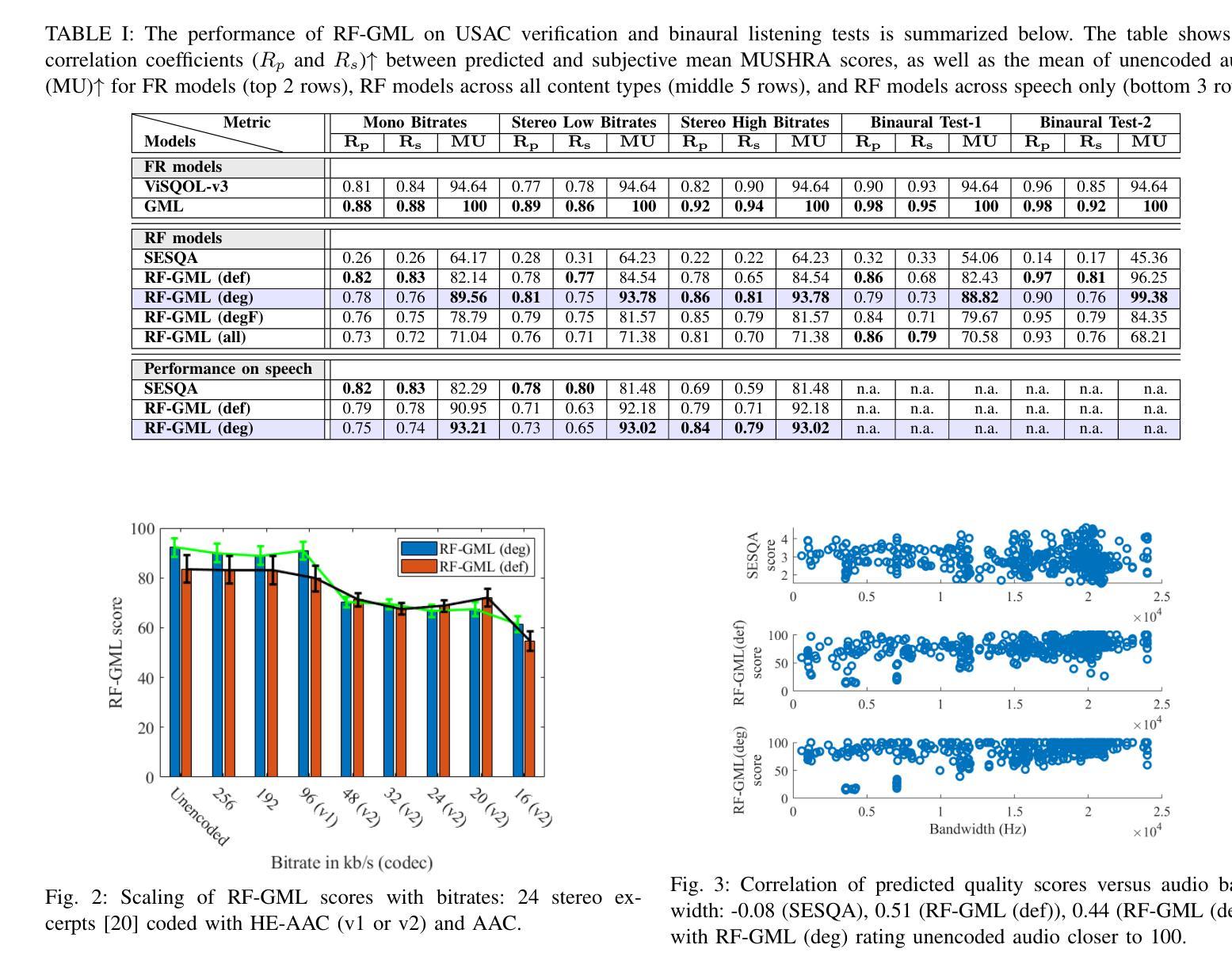