⚠️ 以下所有内容总结都来自于 大语言模型的能力,如有错误,仅供参考,谨慎使用
🔴 请注意:千万不要用于严肃的学术场景,只能用于论文阅读前的初筛!
💗 如果您觉得我们的项目对您有帮助 ChatPaperFree ,还请您给我们一些鼓励!⭐️ HuggingFace免费体验
2025-01-02 更新
Distilled Transformers with Locally Enhanced Global Representations for Face Forgery Detection
Authors:Yaning Zhang, Qiufu Li, Zitong Yu, Linlin Shen
Face forgery detection (FFD) is devoted to detecting the authenticity of face images. Although current CNN-based works achieve outstanding performance in FFD, they are susceptible to capturing local forgery patterns generated by various manipulation methods. Though transformer-based detectors exhibit improvements in modeling global dependencies, they are not good at exploring local forgery artifacts. Hybrid transformer-based networks are designed to capture local and global manipulated traces, but they tend to suffer from the attention collapse issue as the transformer block goes deeper. Besides, soft labels are rarely available. In this paper, we propose a distilled transformer network (DTN) to capture both rich local and global forgery traces and learn general and common representations for different forgery faces. Specifically, we design a mixture of expert (MoE) module to mine various robust forgery embeddings. Moreover, a locally-enhanced vision transformer (LEVT) module is proposed to learn locally-enhanced global representations. We design a lightweight multi-attention scaling (MAS) module to avoid attention collapse, which can be plugged and played in any transformer-based models with only a slight increase in computational costs. In addition, we propose a deepfake self-distillation (DSD) scheme to provide the model with abundant soft label information. Extensive experiments show that the proposed method surpasses the state of the arts on five deepfake datasets.
人脸识别伪造检测(FFD)致力于检测人脸图像的真实性。尽管基于CNN的工作在FFD中取得了出色的性能,但它们容易捕获由各种操作手法生成的各种局部伪造模式。虽然基于转换器的检测器在建模全局依赖性方面有所改进,但它们并不擅长探索局部伪造特征。混合基于转换器的网络旨在捕获局部和全局操纵痕迹,但随着转换器块深入,它们往往会受到注意力崩溃问题的影响。此外,很少使用软标签。在本文中,我们提出了一种蒸馏转换器网络(DTN),以捕获丰富的局部和全局伪造痕迹,并学习不同伪造人脸的一般和通用表示。具体来说,我们设计了一个专家混合(MoE)模块来挖掘各种稳健的伪造嵌入。此外,还提出了一种局部增强视觉转换器(LEVT)模块,用于学习局部增强的全局表示。我们设计了一个轻量级的注意力缩放(MAS)模块来避免注意力崩溃问题,该模块可以在任何基于转换器的模型中进行插拔操作,并且只会导致计算成本轻微增加。此外,我们提出了一种深度伪造自我蒸馏(DSD)方案,为模型提供丰富的软标签信息。大量实验表明,该方法在五个深度伪造数据集上的表现超过了现有技术。
论文及项目相关链接
PDF Accepted by Pattern Recognition
Summary
人脸识别伪造检测(FFD)旨在检测人脸图像的真实性。当前基于CNN的方法在FFD中表现优异,但易受到各种操作手法生成的局部伪造模式的影响。基于Transformer的探测器在建模全局依赖性方面有所改进,但不擅长探索局部伪造特征。本文提出了一种蒸馏Transformer网络(DTN),用于捕捉丰富的局部和全局伪造痕迹,并学习不同伪造面孔的一般和通用表示。具体设计了混合专家(MoE)模块来挖掘各种稳健的伪造嵌入,并引入了局部增强视觉Transformer(LEVT)模块来学习局部增强的全局表示。为解决注意力崩溃问题,设计了一种轻量级的多注意力缩放(MAS)模块,可插入任何基于Transformer的模型中,且仅增加少量的计算成本。此外,还提出了深度伪造自我蒸馏(DSD)方案,为模型提供丰富的软标签信息。实验表明,该方法在五个深度伪造数据集上的表现超越了现有技术。
Key Takeaways
- 人脸伪造检测(FFD)主要目标是检测人脸图像的真实性。
- 当前CNN和Transformer模型在FFD中的优缺点。
- 论文提出了一种蒸馏Transformer网络(DTN),旨在捕捉局部和全局伪造痕迹。
- MoE模块用于挖掘稳健的伪造嵌入,而LEVT模块则学习局部增强的全局表示。
- 为解决注意力崩溃问题,引入了轻量级MAS模块。
- 提出了深度伪造自我蒸馏(DSD)方案,提供软标签信息。
点此查看论文截图
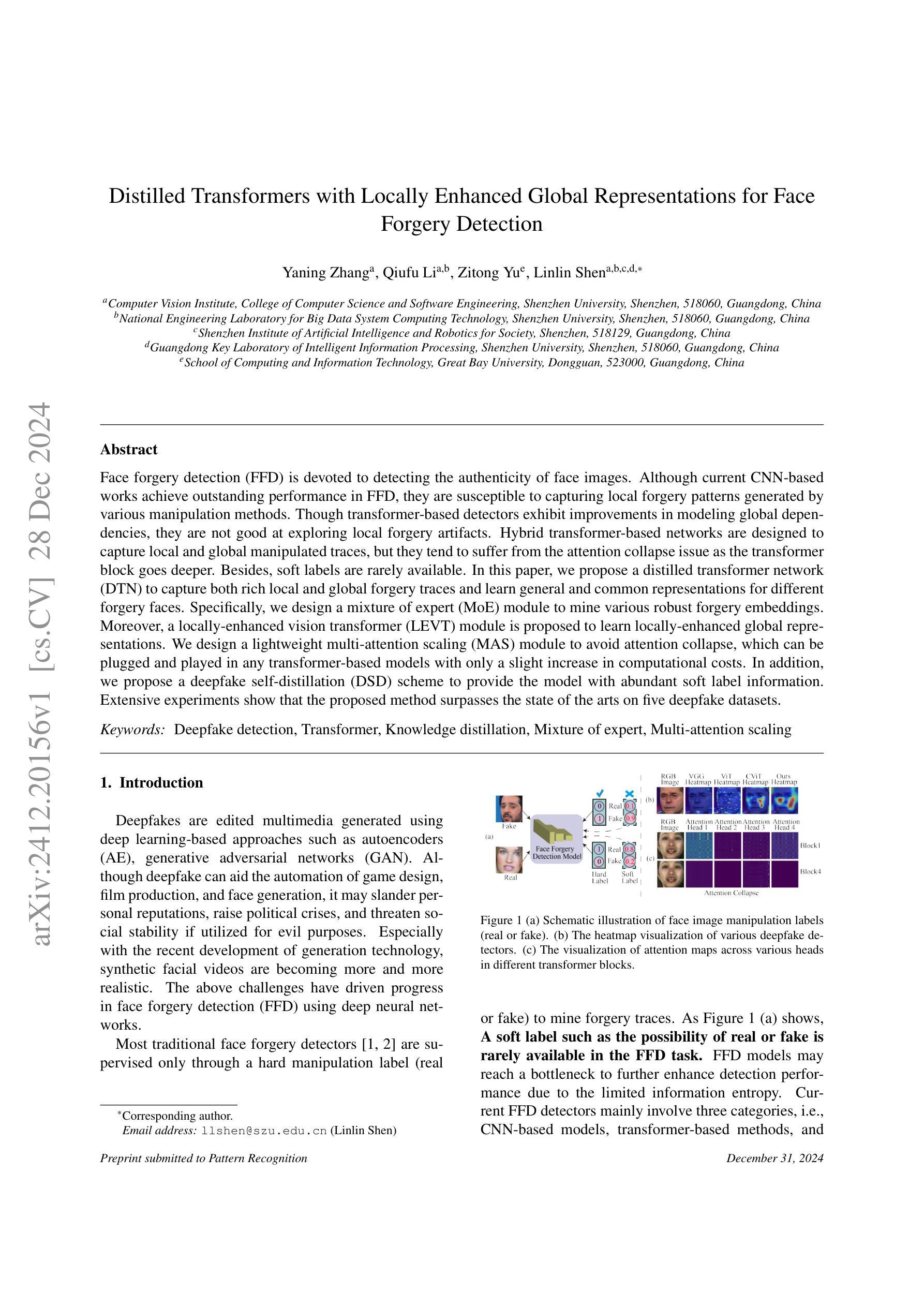
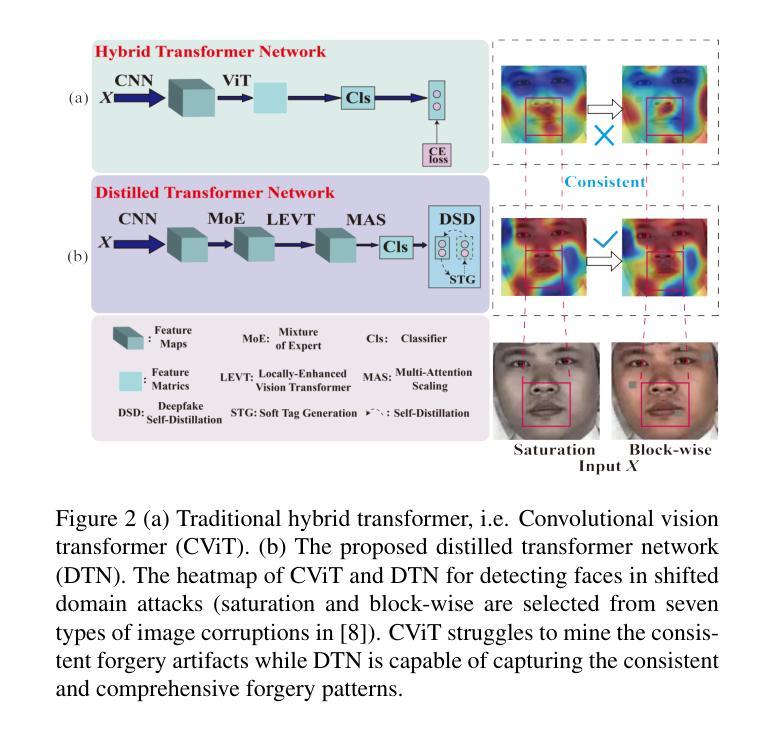
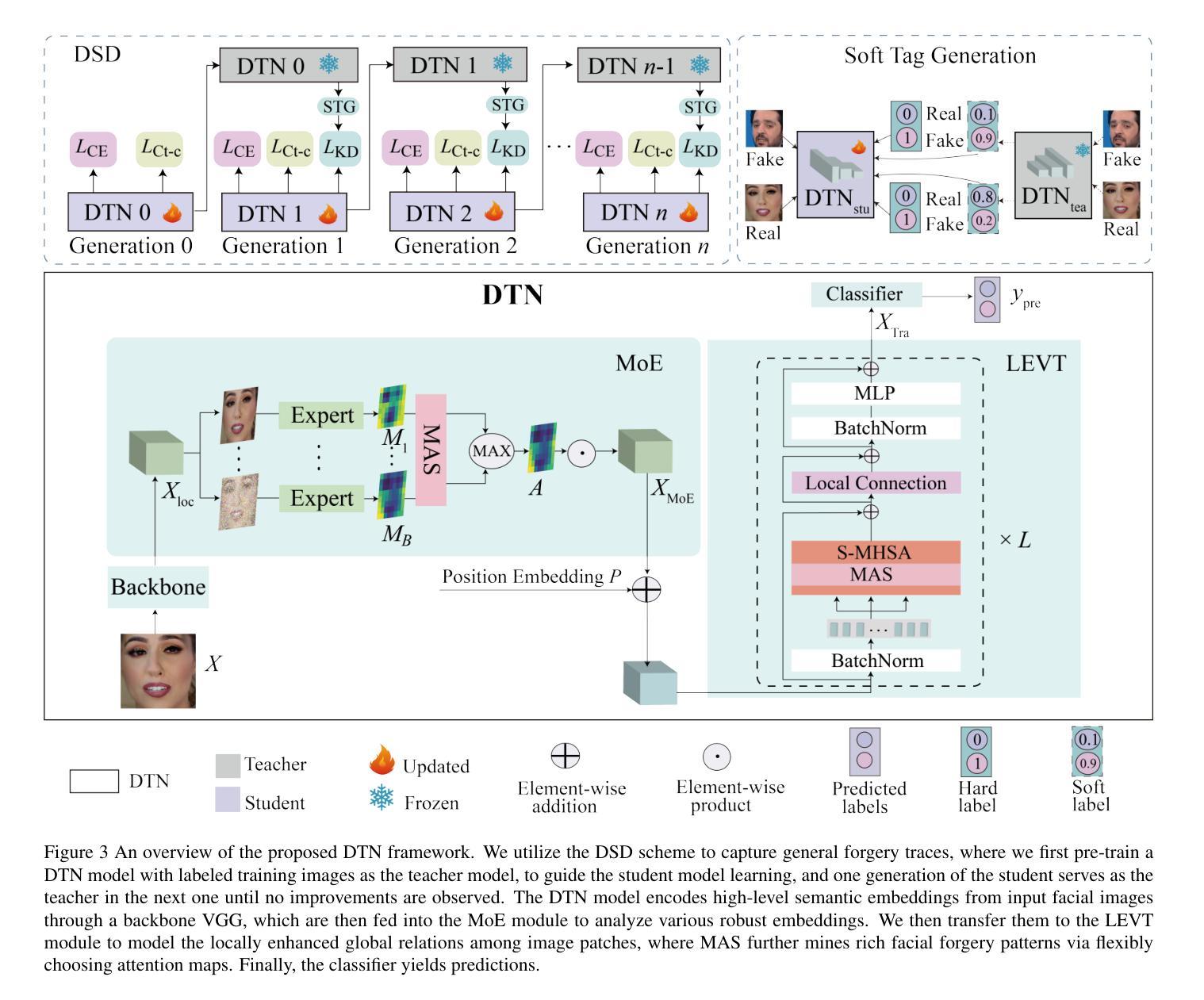
ErasableMask: A Robust and Erasable Privacy Protection Scheme against Black-box Face Recognition Models
Authors:Sipeng Shen, Yunming Zhang, Dengpan Ye, Xiuwen Shi, Long Tang, Haoran Duan, Jiacheng Deng, Ziyi Liu
While face recognition (FR) models have brought remarkable convenience in face verification and identification, they also pose substantial privacy risks to the public. Existing facial privacy protection schemes usually adopt adversarial examples to disrupt face verification of FR models. However, these schemes often suffer from weak transferability against black-box FR models and permanently damage the identifiable information that cannot fulfill the requirements of authorized operations such as forensics and authentication. To address these limitations, we propose ErasableMask, a robust and erasable privacy protection scheme against black-box FR models. Specifically, via rethinking the inherent relationship between surrogate FR models, ErasableMask introduces a novel meta-auxiliary attack, which boosts black-box transferability by learning more general features in a stable and balancing optimization strategy. It also offers a perturbation erasion mechanism that supports the erasion of semantic perturbations in protected face without degrading image quality. To further improve performance, ErasableMask employs a curriculum learning strategy to mitigate optimization conflicts between adversarial attack and perturbation erasion. Extensive experiments on the CelebA-HQ and FFHQ datasets demonstrate that ErasableMask achieves the state-of-the-art performance in transferability, achieving over 72% confidence on average in commercial FR systems. Moreover, ErasableMask also exhibits outstanding perturbation erasion performance, achieving over 90% erasion success rate.
人脸识别(FR)模型在面部验证和识别方面带来了极大的便利,但同时也给公众带来了严重的隐私风险。现有的面部隐私保护方案通常采用对抗性样本的方法来破坏人脸识别模型的面部验证。然而,这些方案通常对黑箱人脸识别模型的迁移性较弱,并且会永久损坏身份信息,无法满足司法和身份验证等授权操作的要求。为了解决这些局限性,我们提出了ErasableMask,这是一种针对黑箱人脸识别模型的稳健且可擦除的隐私保护方案。具体来说,ErasableMask通过重新思考代理人脸识别模型之间的内在关系,引入了一种新型的元辅助攻击,通过稳定且平衡的优化策略学习更通用的特征,提高了黑箱迁移性。它还提供了一个扰动消除机制,可以在不降低图像质量的情况下,消除受保护面部中的语义扰动。为了进一步改进性能,ErasableMask采用了一种课程学习策略,以减轻对抗性攻击和扰动消除之间的优化冲突。在CelebA-HQ和FFHQ数据集上的大量实验表明,ErasableMask在迁移性方面达到了最新技术水平,在商用人脸识别系统上的平均置信度超过72%。此外,ErasableMask还表现出出色的扰动消除性能,消除成功率超过90%。
论文及项目相关链接
Summary
人脸识别技术带来便利的同时,也存在隐私泄露风险。现有面部隐私保护方案通常采用对抗样本干扰人脸识别模型,但存在黑箱模型迁移能力差和信息永久性损害等问题。本研究提出了一种对抗黑箱人脸识别模型的ErasableMask方案,通过优化策略和扰动消除机制提高隐私保护效果和图像质量。采用课程学习策略缓解优化冲突,实现卓越性能。
Key Takeaways
- 人脸识别技术带来的隐私风险。
- 现有面部隐私保护方案的问题:对抗样本迁移能力弱、信息永久性损害。
- ErasableMask方案提出:针对黑箱人脸识别模型的隐私保护。
- ErasableMask采用新型元辅助攻击提高黑箱迁移能力。
- ErasableMask有扰动消除机制,保护隐私同时不降低图像质量。
- 采用课程学习策略缓解优化冲突,提高性能。
点此查看论文截图
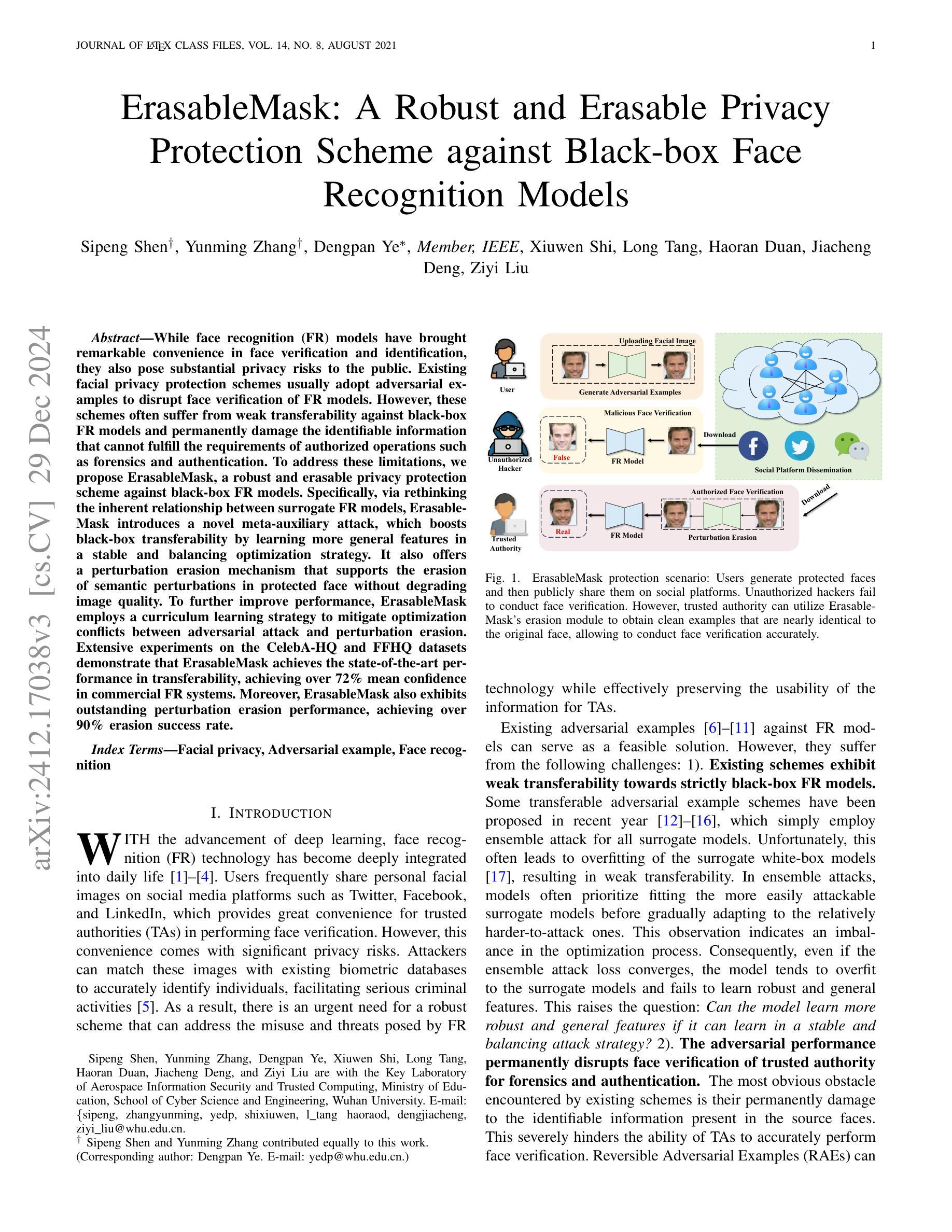
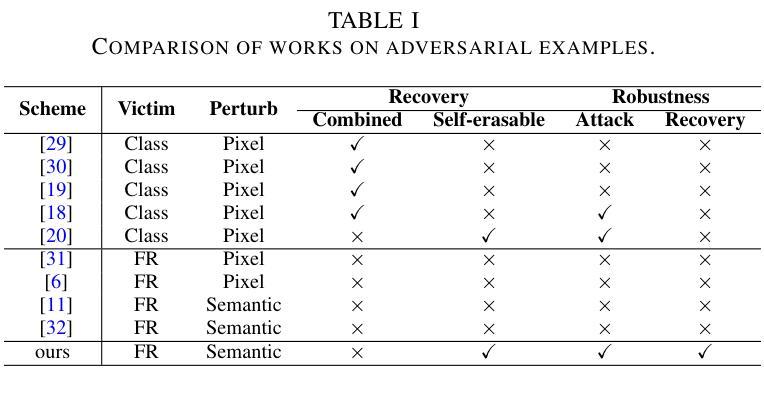
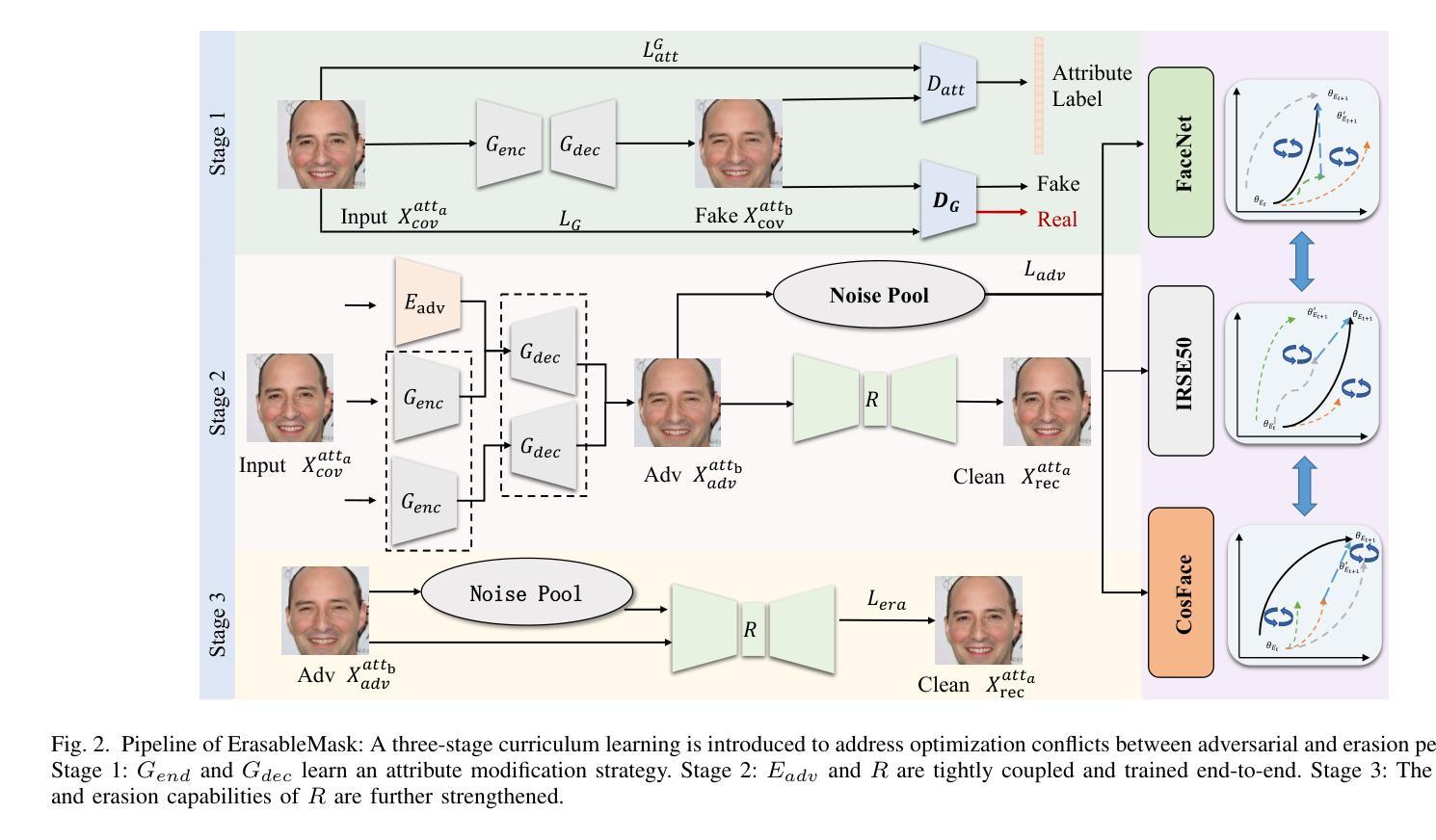
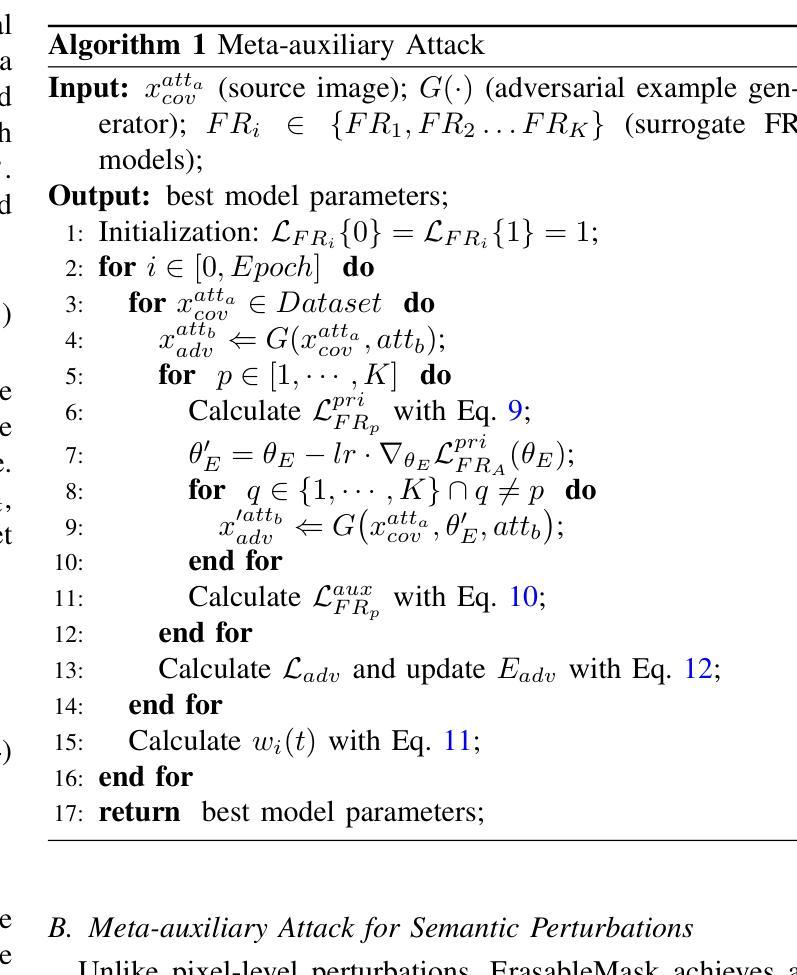
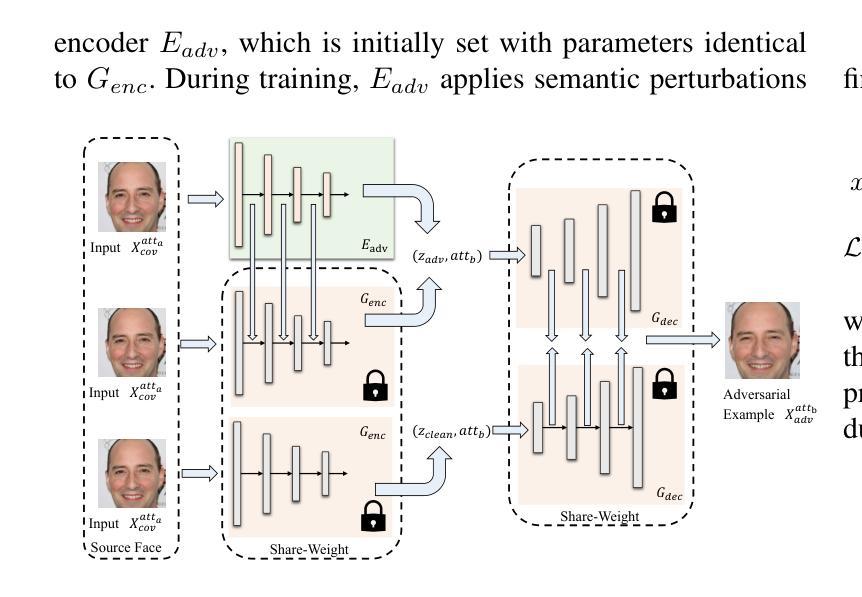
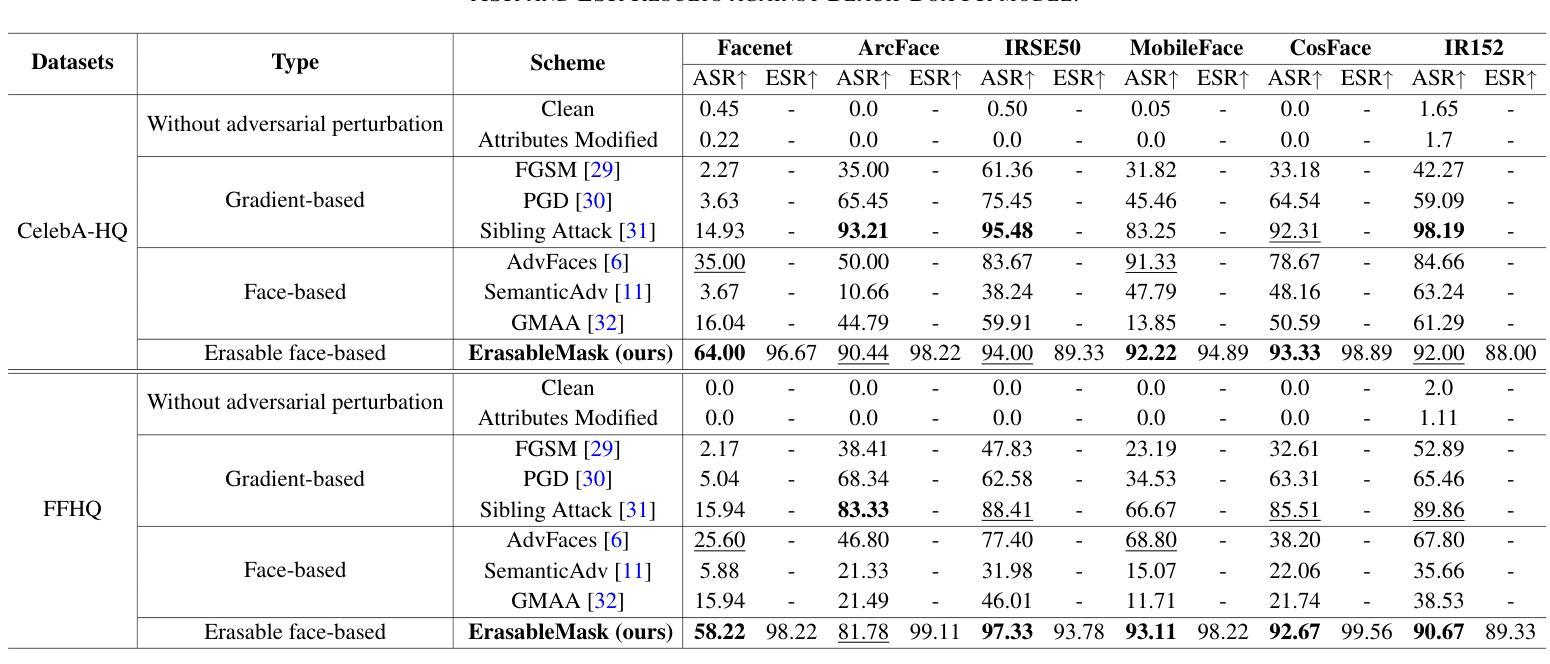
TetraLoss: Improving the Robustness of Face Recognition against Morphing Attacks
Authors:Mathias Ibsen, Lázaro J. González-Soler, Christian Rathgeb, Christoph Busch
Face recognition systems are widely deployed in high-security applications such as for biometric verification at border controls. Despite their high accuracy on pristine data, it is well-known that digital manipulations, such as face morphing, pose a security threat to face recognition systems. Malicious actors can exploit the facilities offered by the identity document issuance process to obtain identity documents containing morphed images. Thus, subjects who contributed to the creation of the morphed image can with high probability use the identity document to bypass automated face recognition systems. In recent years, no-reference (i.e., single image) and differential morphing attack detectors have been proposed to tackle this risk. These systems are typically evaluated in isolation from the face recognition system that they have to operate jointly with and do not consider the face recognition process. Contrary to most existing works, we present a novel method for adapting deep learning-based face recognition systems to be more robust against face morphing attacks. To this end, we introduce TetraLoss, a novel loss function that learns to separate morphed face images from its contributing subjects in the embedding space while still achieving high biometric verification performance. In a comprehensive evaluation, we show that the proposed method can significantly enhance the original system while also significantly outperforming other tested baseline methods.
人脸识别系统已广泛应用于高安全性的应用,例如在边境控制的生物识别验证中。尽管人脸识别系统在原始数据上具有高精度,但众所周知,数字操作,例如面部变形,对人脸识别系统构成安全威胁。恶意行为者可以利用身份文件发放流程所提供的设施获得包含变形图像的身份文件。因此,为变形图像的创建做出贡献的主体很有可能使用该身份文件绕过自动化人脸识别系统。近年来,无参考(即单张图像)和差分变形攻击检测器已被提出,以应对这种风险。这些系统的评估通常是与人脸识别系统分开进行的,而它们必须与该系统一起运行,并且不考虑人脸识别过程。与大多数现有工作相反,我们提出了一种使基于深度学习的人脸识别系统更适应对抗面部变形攻击的新方法。为此,我们引入了TetraLoss,这是一种新型损失函数,能够在嵌入空间中分离变形的面部图像与其贡献主体,同时仍可实现高生物识别验证性能。在全面评估中,我们证明了所提出的方法可以显着增强原始系统,并且显著优于其他经过测试的基线方法。
论文及项目相关链接
PDF Accepted to the IEEE International Conference on Automatic Face & Gesture Recognition 2024 (FG’24)
Summary
人脸识别系统在高安全应用如边境控制生物识别验证中广泛应用。然而,数字操作如人脸合成对其构成安全威胁。恶意行为者可能利用身份文件发放过程中的设施获取包含合成图像的身份文件。因此,参与创建合成图像的个体很可能使用该身份文件绕过自动化人脸识别系统。近年虽有无参考(即单张图像)和差分合成攻击检测器应对此风险,但它们通常与需要协同运作的人脸识别系统分离评估,并未考虑人脸识别过程。本文提出一种使深度学习为基础的人脸识别系统更抗人脸合成攻击的新方法。为此,我们引入了Tetraloss这一新型损失函数,它能在嵌入空间中分离合成人脸图像及其参与主体,同时仍能保持高生物识别验证性能。全面评估表明,该方法能显著提升原系统性能并显著优于其他测试基线方法。
Key Takeaways
- 人脸识别系统在高安全应用中广泛应用,但数字操作如人脸合成构成安全威胁。
- 恶意行为者可能利用身份文件发放过程中的设施获取包含合成图像的身份文件。
- 现有检测器通常与人脸识别系统分离评估,未全面考虑人脸识别过程。
- 引入了一种新型方法,通过Tetraloss损失函数提升人脸识别系统对抗人脸合成的稳健性。
- Tetraloss能在嵌入空间中分离合成人脸图像及其参与主体。
- 该方法在提高系统抗攻击能力的同时保持了高生物识别验证性能。
点此查看论文截图
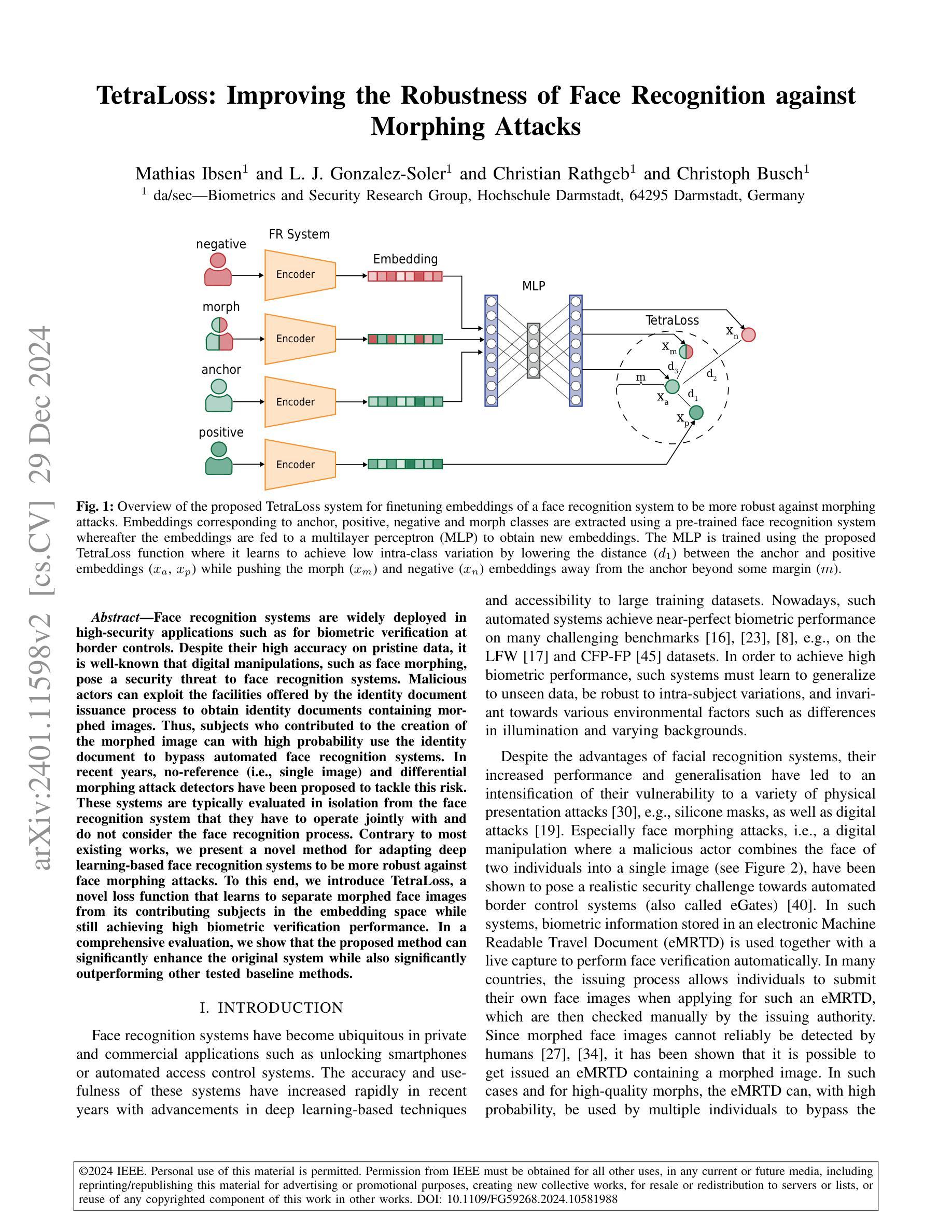
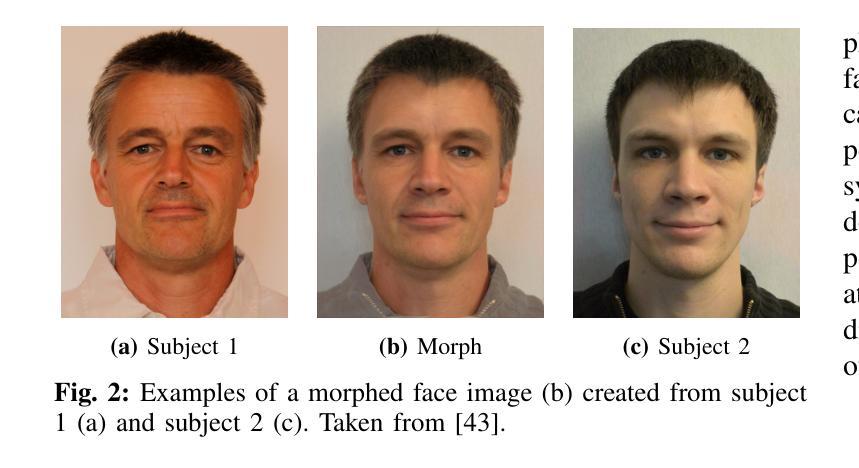
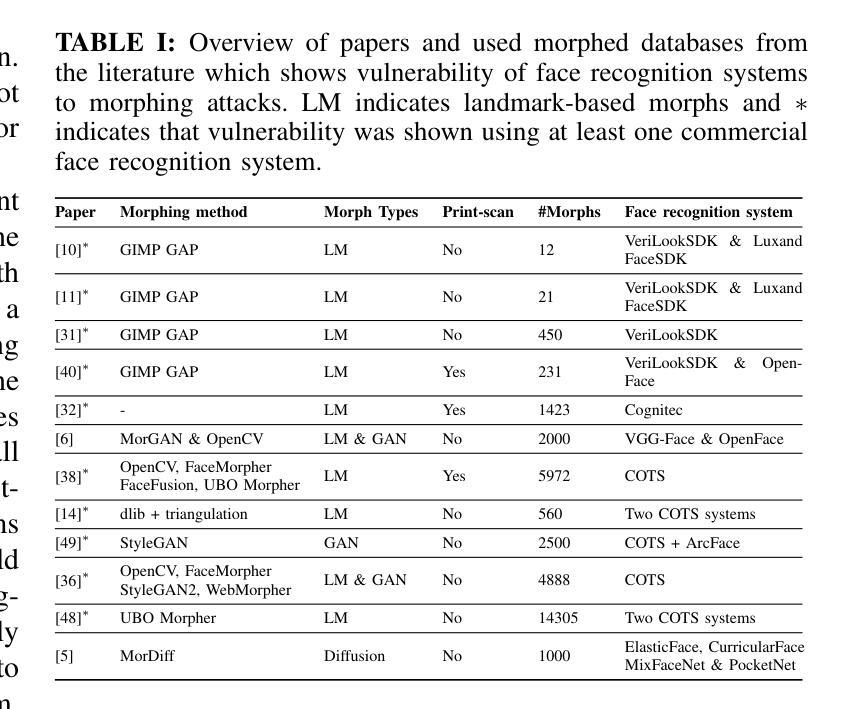
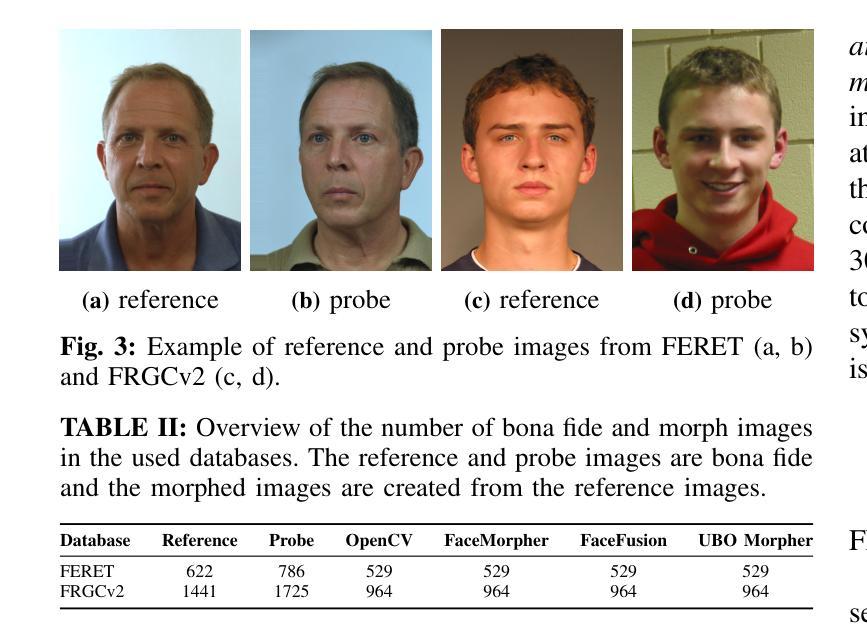
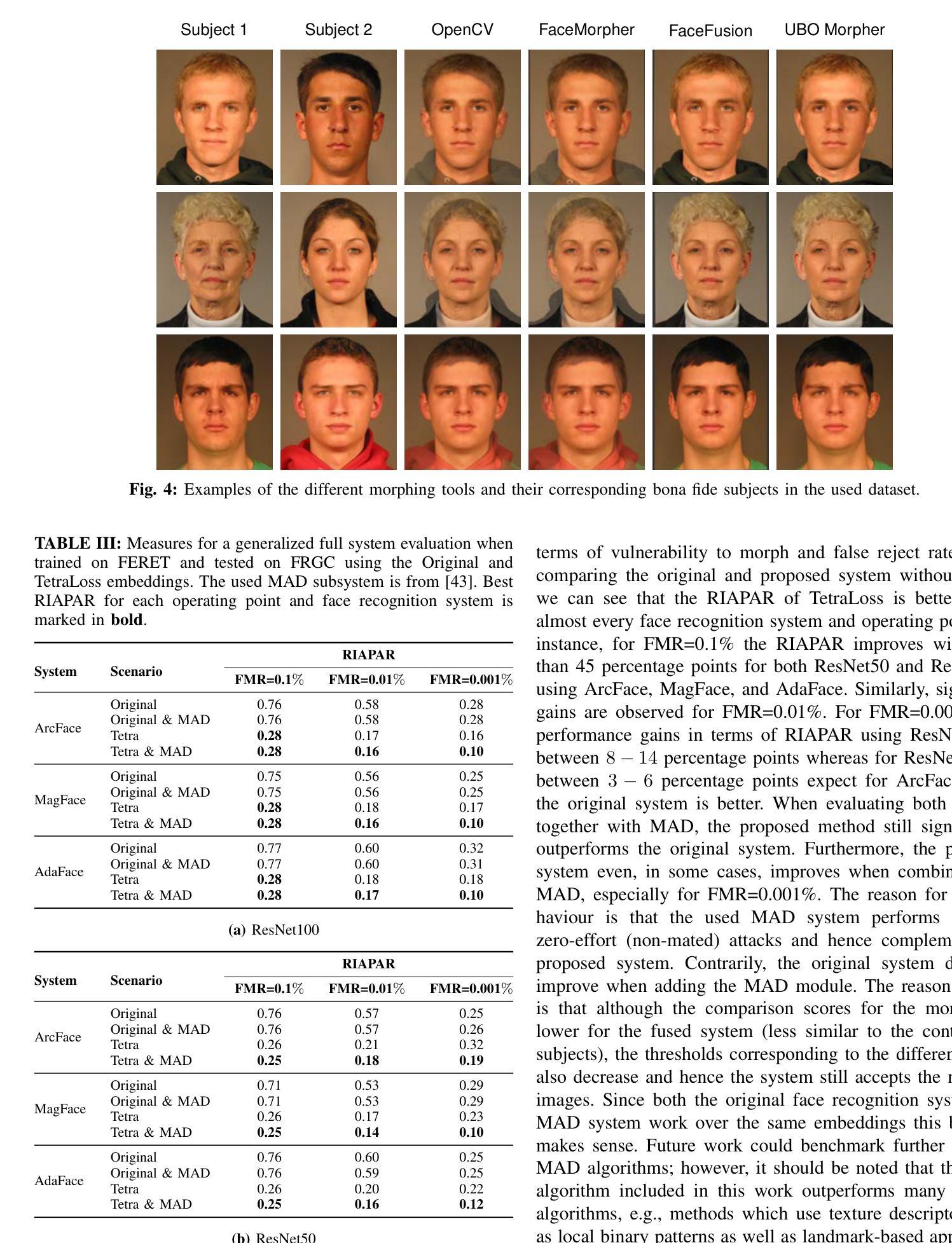
Multi-Channel Cross Modal Detection of Synthetic Face Images
Authors:M. Ibsen, C. Rathgeb, S. Marcel, C. Busch
Synthetically generated face images have shown to be indistinguishable from real images by humans and as such can lead to a lack of trust in digital content as they can, for instance, be used to spread misinformation. Therefore, the need to develop algorithms for detecting entirely synthetic face images is apparent. Of interest are images generated by state-of-the-art deep learning-based models, as these exhibit a high level of visual realism. Recent works have demonstrated that detecting such synthetic face images under realistic circumstances remains difficult as new and improved generative models are proposed with rapid speed and arbitrary image post-processing can be applied. In this work, we propose a multi-channel architecture for detecting entirely synthetic face images which analyses information both in the frequency and visible spectra using Cross Modal Focal Loss. We compare the proposed architecture with several related architectures trained using Binary Cross Entropy and show in cross-model experiments that the proposed architecture supervised using Cross Modal Focal Loss, in general, achieves most competitive performance.
合成生成的面部图像已经证明人类无法将其与真实图像区分开,因此可能导致对数字内容的信任缺失,因为它们可能被用于传播错误信息。因此,开发用于检测完全合成面部图像的算法的需求变得显而易见。人们感兴趣的图像是由最新深度学习模型生成的,因为这些图像具有高度的视觉逼真性。近期的工作表明,随着新改进的生成模型迅速被提出,在现实中检测此类合成面部图像变得困难重重,还可以应用任意图像的后处理。在这项工作中,我们提出了一种用于检测完全合成面部图像的多通道架构,该架构通过跨模态焦点损失来分析频率和可见光谱中的信息。我们将所提出的架构与几种使用二元交叉熵训练的相关架构进行了比较,并在跨模型实验中发现,与使用跨模态焦点损失监督的架构通常具有最具竞争力的性能表现。
论文及项目相关链接
PDF Accepted to the 2024 International Workshop on Biometrics and Forensics (IWBF)
Summary
新一代深度学习的合成面部图像生成技术已经实现了高度的视觉逼真度,但同时也带来了信任危机问题,因为它们可能被用于传播虚假信息。因此,开发检测完全合成面部图像的算法变得至关重要。本研究提出了一种多通道架构用于检测完全合成的面部图像,该架构在频率和可见光谱上进行分析,并使用跨模态焦点损失进行监督。实验表明,与其他模型相比,该架构在跨模型实验中具有最佳的竞争性能。
Key Takeaways
- 合成面部图像已经高度逼真,引发信任危机问题。
- 检测完全合成的面部图像对于防止虚假信息的传播至关重要。
- 提出了一种多通道架构用于检测合成面部图像。
- 该架构结合频率和可见光谱分析技术。
- 该架构采用跨模态焦点损失进行监督训练。
- 实验结果表明该架构具有最佳的性能表现。
点此查看论文截图
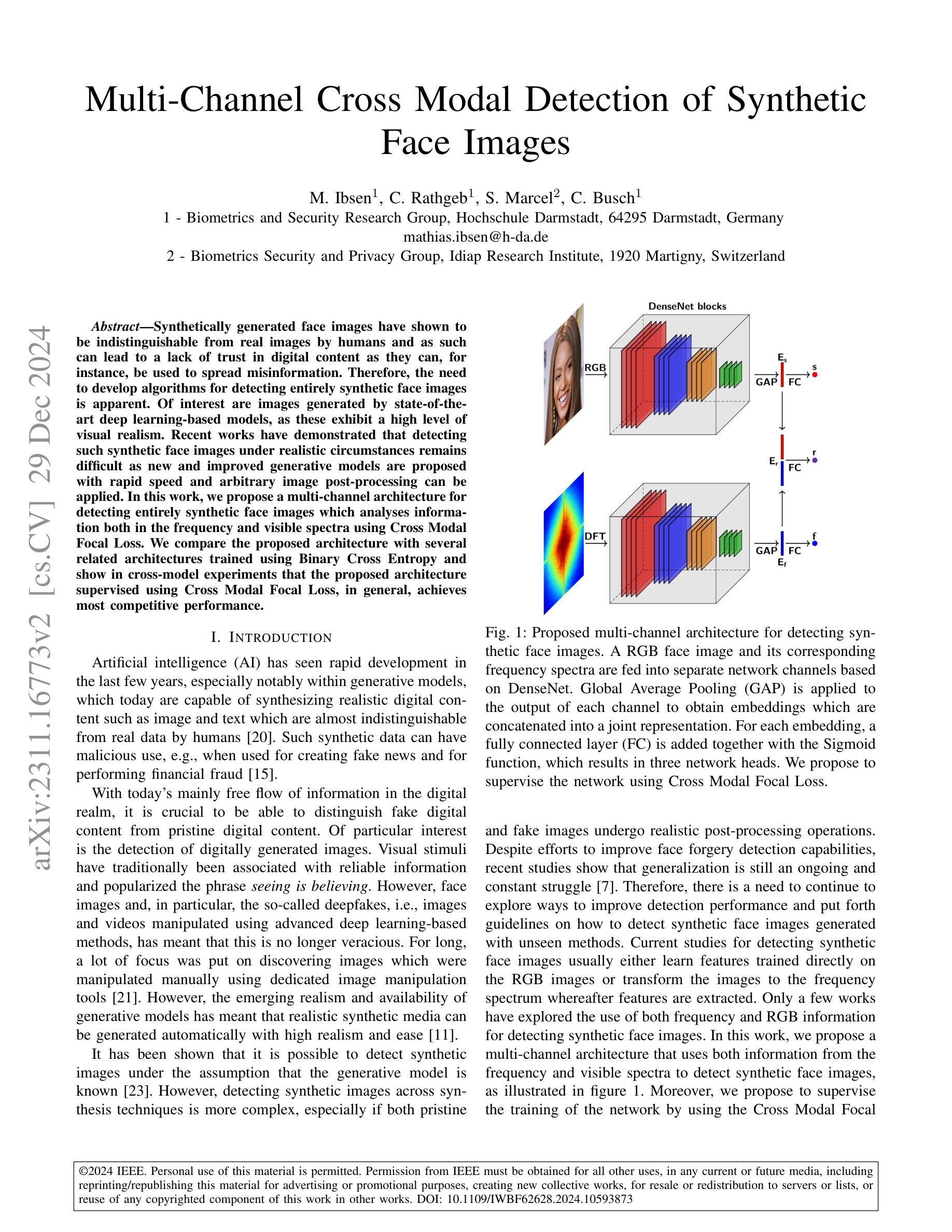
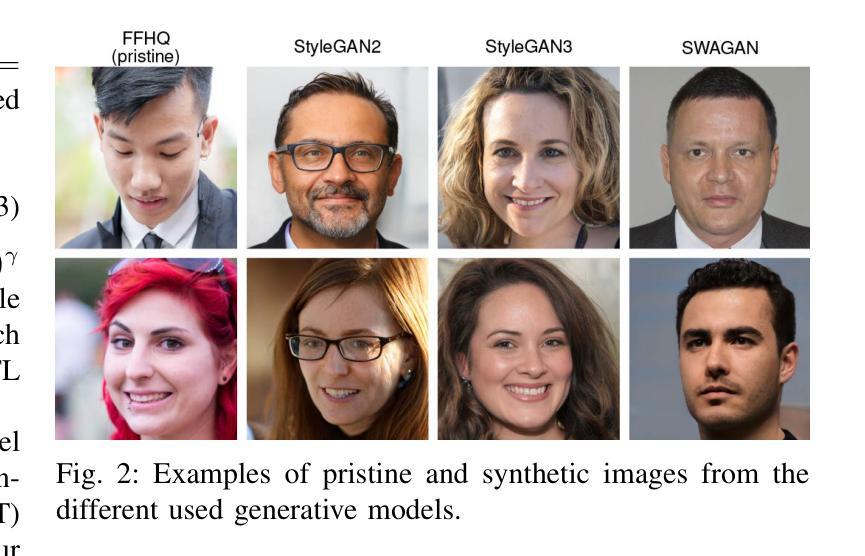
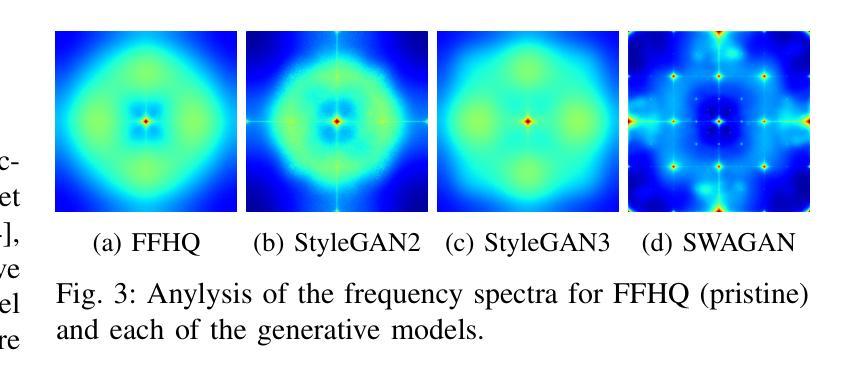
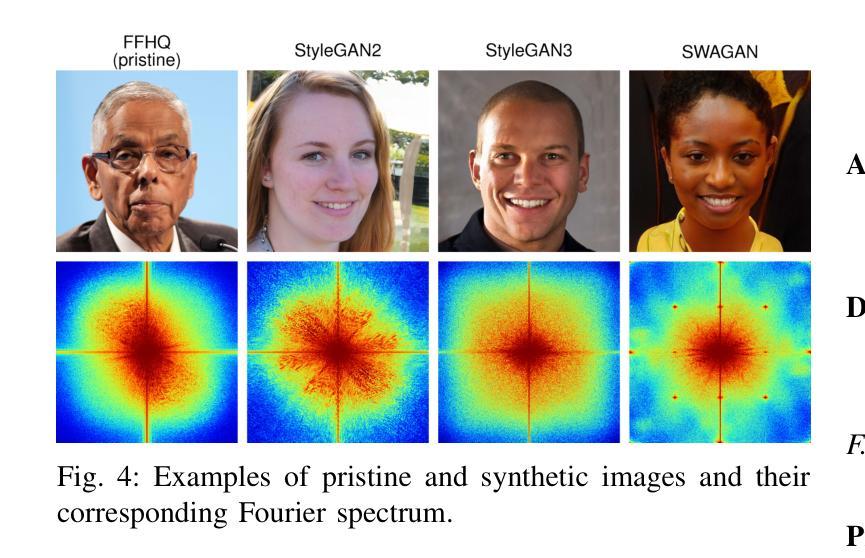
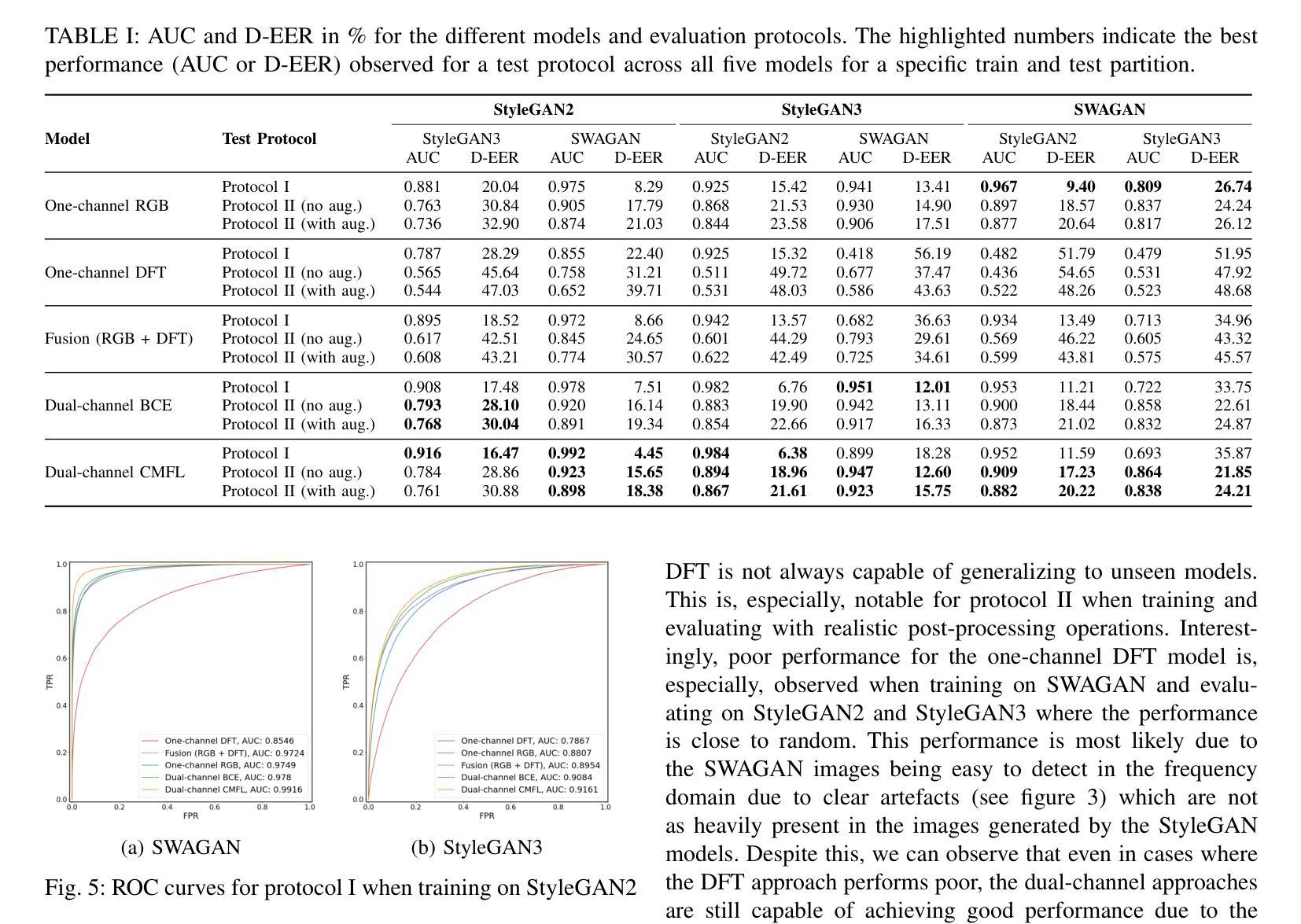
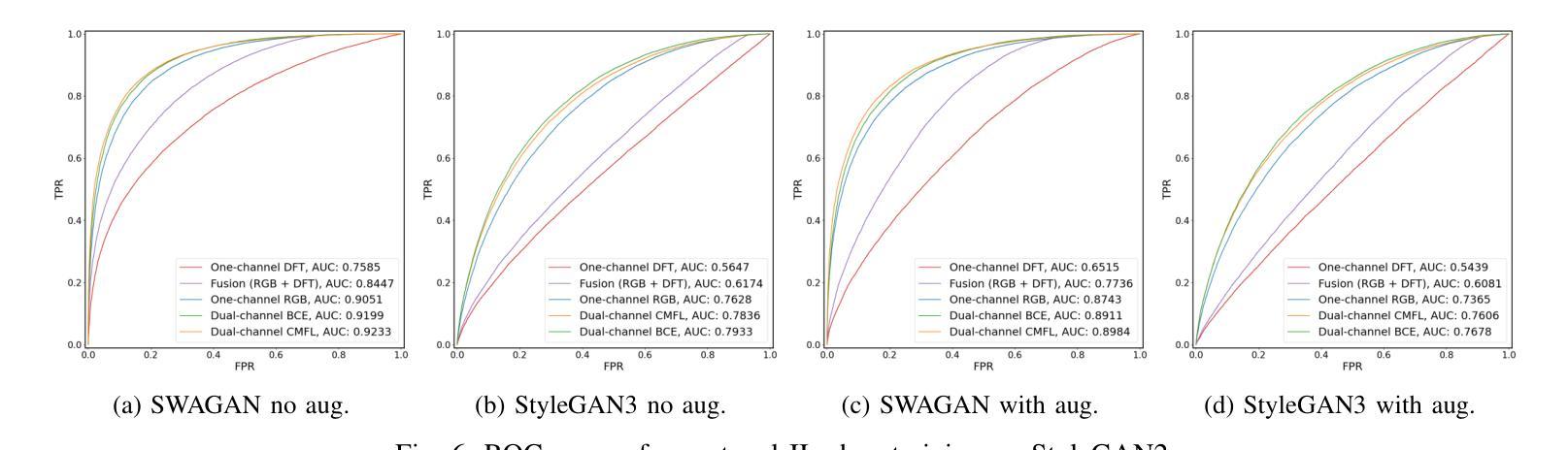