⚠️ 以下所有内容总结都来自于 大语言模型的能力,如有错误,仅供参考,谨慎使用
🔴 请注意:千万不要用于严肃的学术场景,只能用于论文阅读前的初筛!
💗 如果您觉得我们的项目对您有帮助 ChatPaperFree ,还请您给我们一些鼓励!⭐️ HuggingFace免费体验
2025-01-02 更新
Neighbor Does Matter: Density-Aware Contrastive Learning for Medical Semi-supervised Segmentation
Authors:Feilong Tang, Zhongxing Xu, Ming Hu, Wenxue Li, Peng Xia, Yiheng Zhong, Hanjun Wu, Jionglong Su, Zongyuan Ge
In medical image analysis, multi-organ semi-supervised segmentation faces challenges such as insufficient labels and low contrast in soft tissues. To address these issues, existing studies typically employ semi-supervised segmentation techniques using pseudo-labeling and consistency regularization. However, these methods mainly rely on individual data samples for training, ignoring the rich neighborhood information present in the feature space. In this work, we argue that supervisory information can be directly extracted from the geometry of the feature space. Inspired by the density-based clustering hypothesis, we propose using feature density to locate sparse regions within feature clusters. Our goal is to increase intra-class compactness by addressing sparsity issues. To achieve this, we propose a Density-Aware Contrastive Learning (DACL) strategy, pushing anchored features in sparse regions towards cluster centers approximated by high-density positive samples, resulting in more compact clusters. Specifically, our method constructs density-aware neighbor graphs using labeled and unlabeled data samples to estimate feature density and locate sparse regions. We also combine label-guided co-training with density-guided geometric regularization to form complementary supervision for unlabeled data. Experiments on the Multi-Organ Segmentation Challenge dataset demonstrate that our proposed method outperforms state-of-the-art methods, highlighting its efficacy in medical image segmentation tasks.
在医学图像分析领域,多器官半监督分割面临着标签不足和软组织对比度低等挑战。为了应对这些问题,现有研究通常采用基于伪标签和一致性正则化的半监督分割技术。然而,这些方法主要依赖于单个数据样本进行训练,忽略了特征空间中存在的丰富邻域信息。在这项工作中,我们认为监督信息可以直接从特征空间的几何形状中提取。受基于密度的聚类假设的启发,我们提出使用特征密度来定位特征聚类中的稀疏区域。我们的目标是通过解决稀疏性问题来增加类内紧凑性。为了实现这一目标,我们提出了一种密度感知对比学习(DACL)策略,该策略将锚点特征从稀疏区域推向由高密度正样本近似的聚类中心,从而形成更紧凑的聚类。具体来说,我们的方法使用有标签和无标签数据样本构建密度感知邻居图来估计特征密度并定位稀疏区域。我们还结合了标签引导的协同训练和密度引导几何正则化,为无标签数据形成互补监督。在Multi-Organ Segmentation Challenge数据集上的实验表明,我们提出的方法优于最新方法,突显其在医学图像分割任务中的有效性。
论文及项目相关链接
Summary
本文提出一种基于特征密度的对比学习(DACL)策略,用于解决医学图像分析中多器官半监督分割面临的挑战,如标签不足和软组织对比度低等问题。该方法通过利用特征空间的几何信息来寻找稀疏区域,提高类内紧凑性,并通过构建密度感知邻接图和使用标签引导的协同训练与密度引导几何正则化,为未标记数据提供互补监督。在Multi-Organ Segmentation Challenge数据集上的实验表明,该方法优于现有技术,尤其在医学图像分割任务中效果显著。
Key Takeaways
- 医学图像分析中多器官半监督分割面临标签不足和软组织对比度低的问题。
- 现有方法主要依赖个体数据样本进行训练,忽略了特征空间中的丰富邻域信息。
- 本文受密度聚类假设启发,提出使用特征密度来定位特征簇中的稀疏区域。
- 提出密度感知对比学习(DACL)策略,通过推动锚定特征向高密度正样例子集的簇中心移动,形成更紧凑的簇。
- 构建密度感知邻接图,利用有标签和无标签数据样本估计特征密度并定位稀疏区域。
- 结合标签引导的协同训练和密度引导几何正则化,为未标记数据提供互补监督。
点此查看论文截图
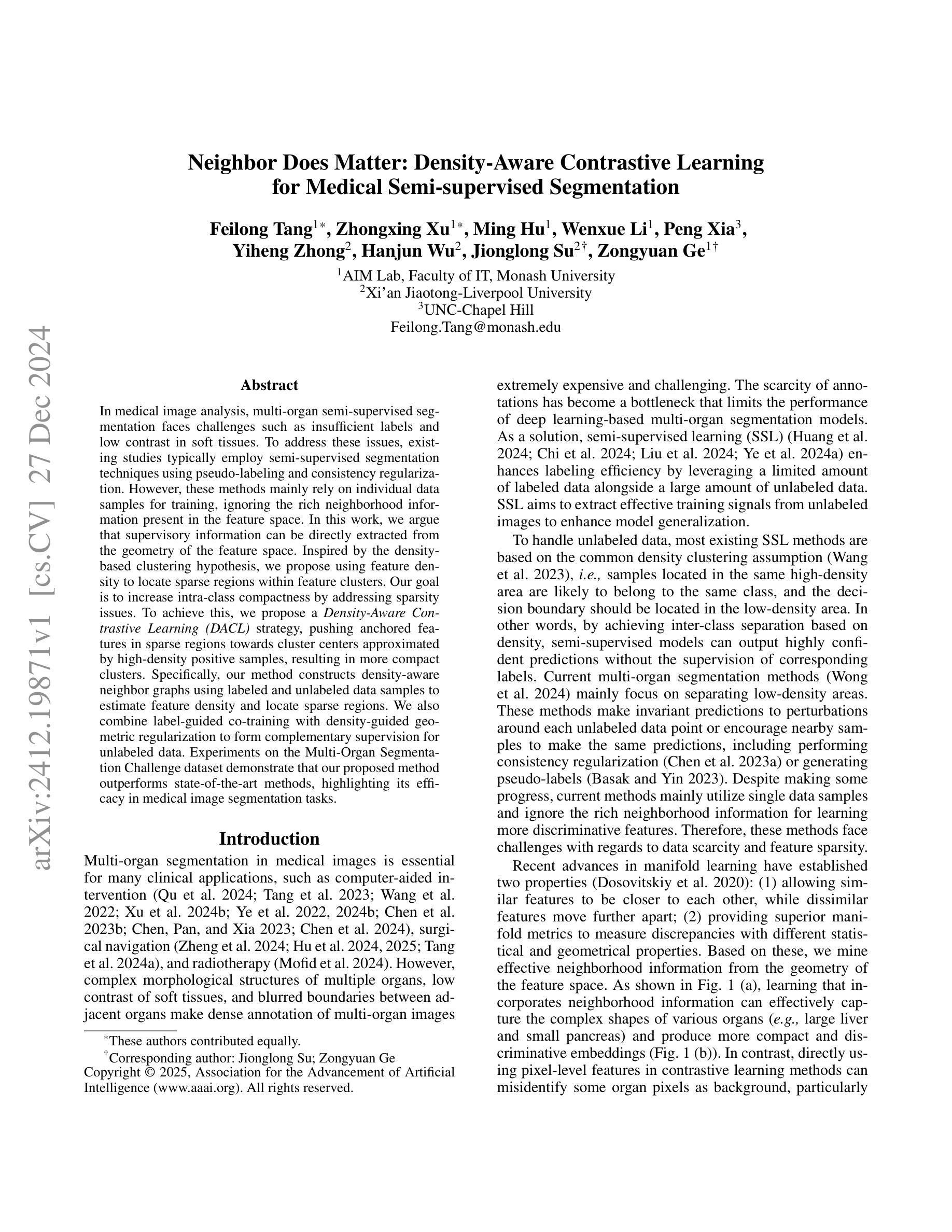
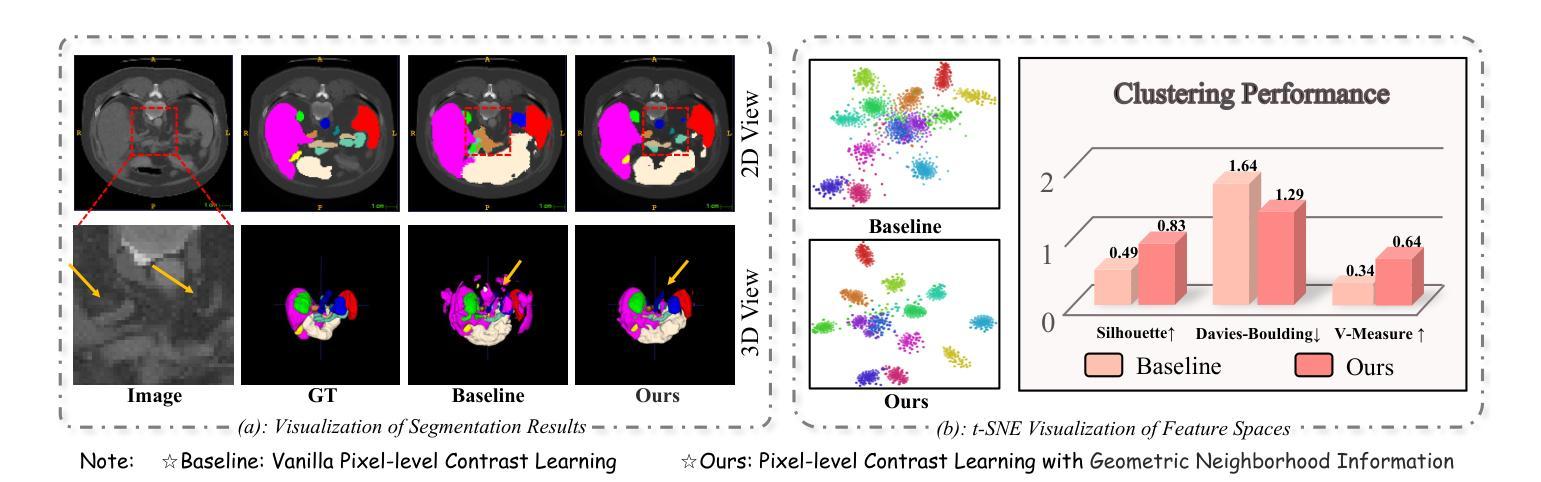
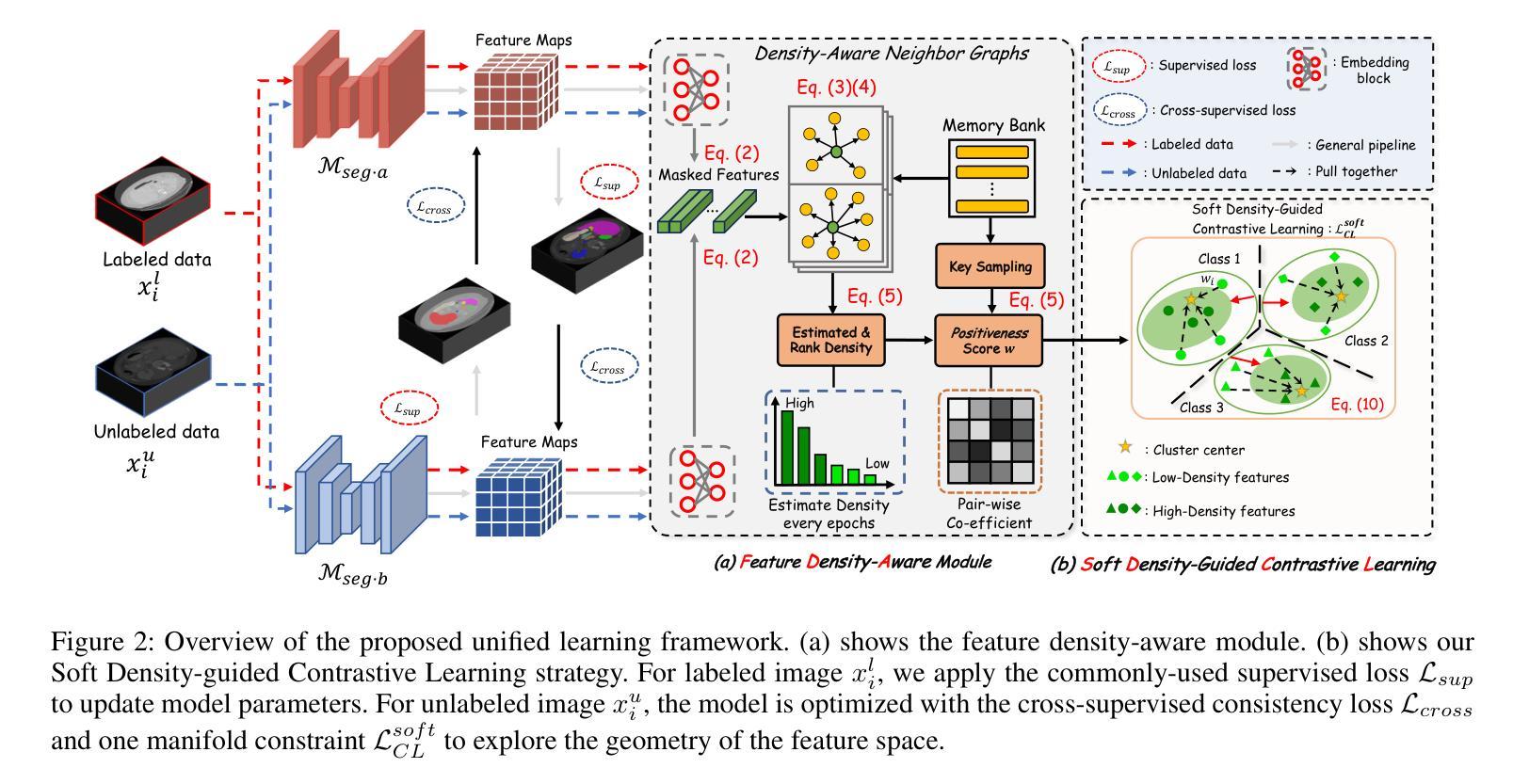
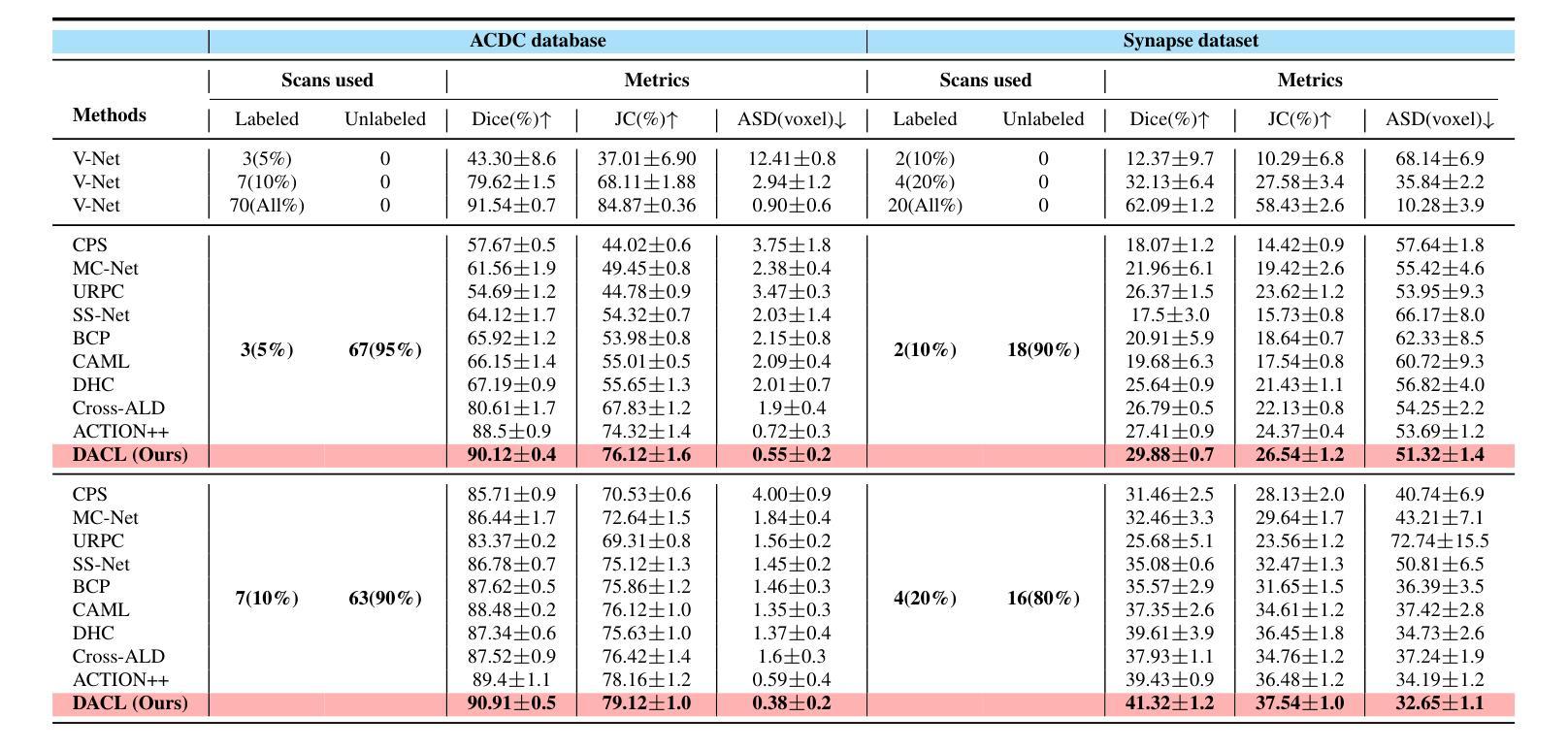
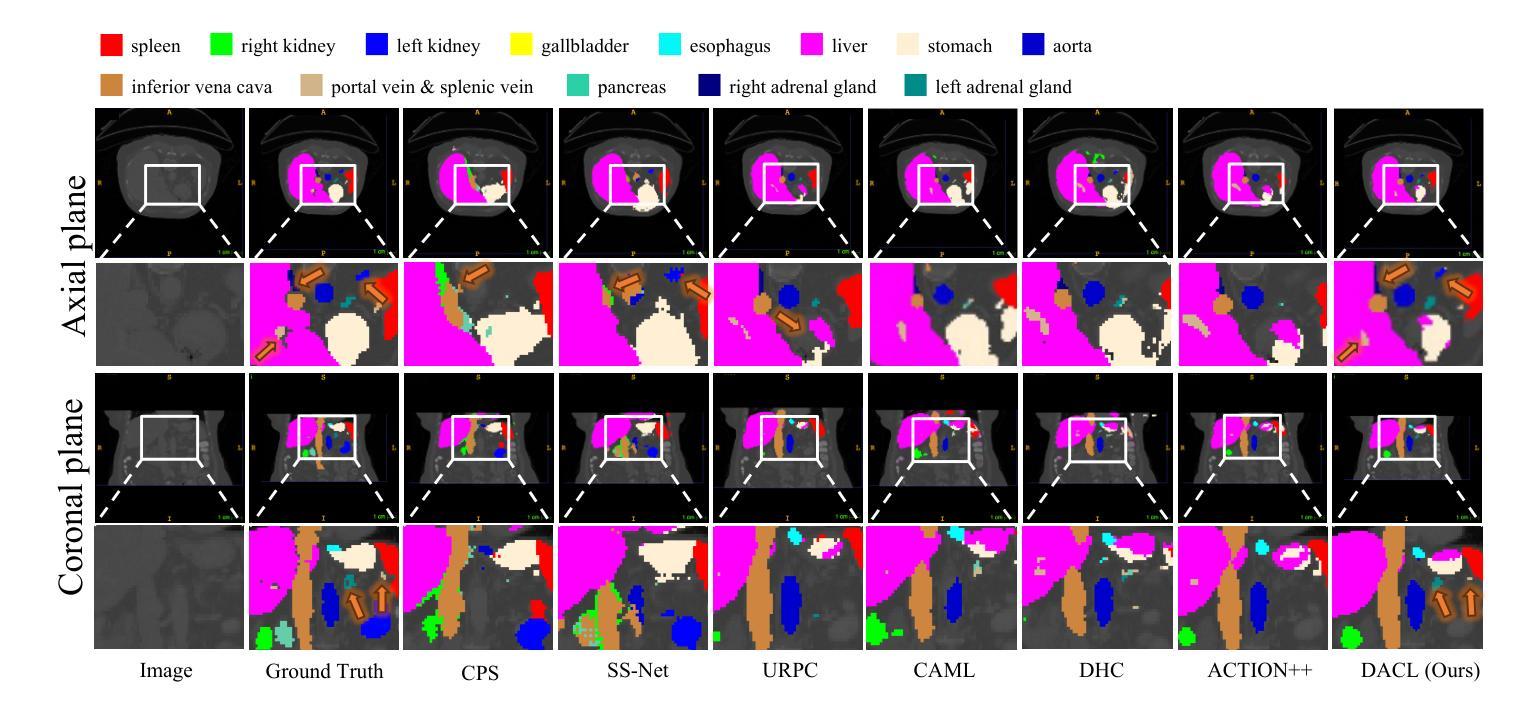
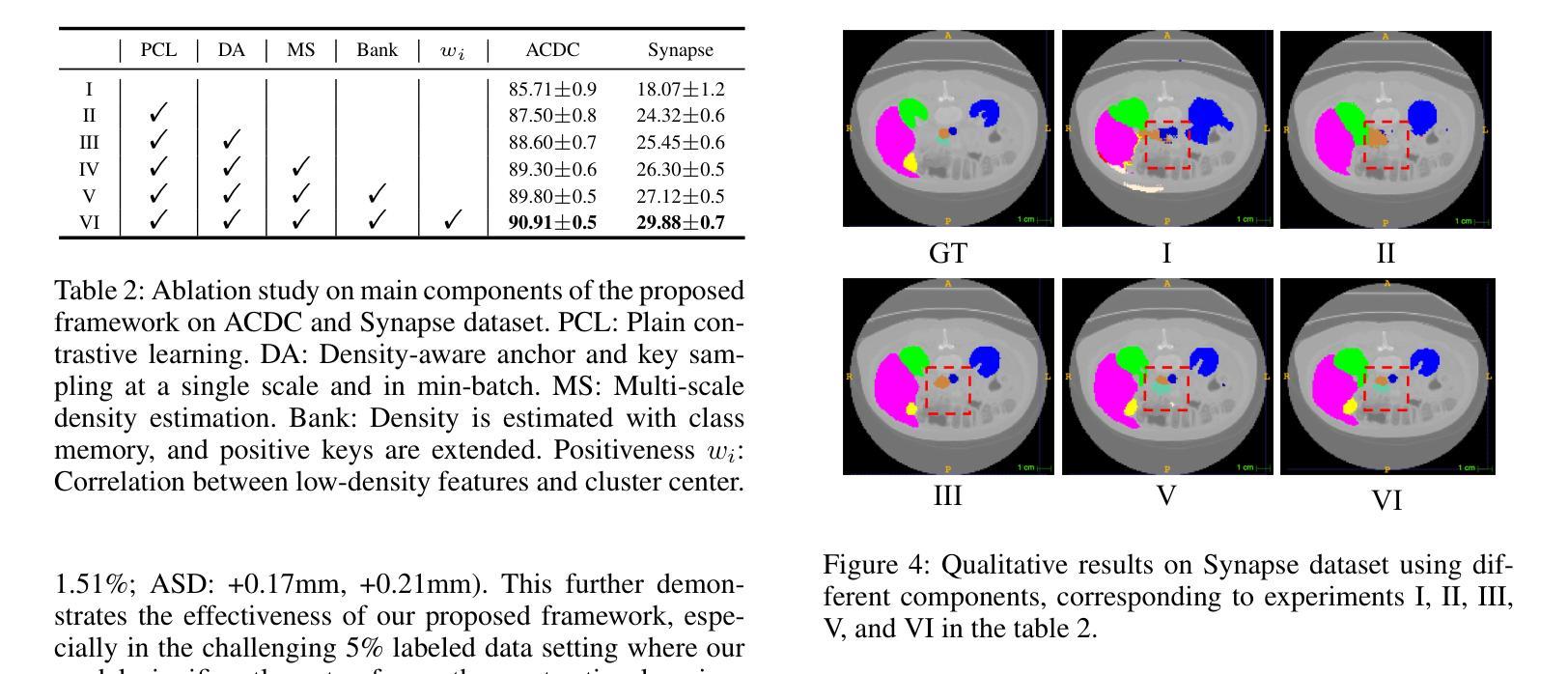
NijiGAN: Transform What You See into Anime with Contrastive Semi-Supervised Learning and Neural Ordinary Differential Equations
Authors:Kevin Putra Santoso, Anny Yuniarti, Dwiyasa Nakula, Dimas Prihady Setyawan, Adam Haidar Azizi, Jeany Aurellia P. Dewati, Farah Dhia Fadhila, Maria T. Elvara Bumbungan
Generative AI has transformed the animation industry. Several models have been developed for image-to-image translation, particularly focusing on converting real-world images into anime through unpaired translation. Scenimefy, a notable approach utilizing contrastive learning, achieves high fidelity anime scene translation by addressing limited paired data through semi-supervised training. However, it faces limitations due to its reliance on paired data from a fine-tuned StyleGAN in the anime domain, often producing low-quality datasets. Additionally, Scenimefy’s high parameter architecture presents opportunities for computational optimization. This research introduces NijiGAN, a novel model incorporating Neural Ordinary Differential Equations (NeuralODEs), which offer unique advantages in continuous transformation modeling compared to traditional residual networks. NijiGAN successfully transforms real-world scenes into high fidelity anime visuals using half of Scenimefy’s parameters. It employs pseudo-paired data generated through Scenimefy for supervised training, eliminating dependence on low-quality paired data and improving the training process. Our comprehensive evaluation includes ablation studies, qualitative, and quantitative analysis comparing NijiGAN to similar models. The testing results demonstrate that NijiGAN produces higher-quality images compared to AnimeGAN, as evidenced by a Mean Opinion Score (MOS) of 2.192, it surpasses AnimeGAN’s MOS of 2.160. Furthermore, our model achieved a Frechet Inception Distance (FID) score of 58.71, outperforming Scenimefy’s FID score of 60.32. These results demonstrate that NijiGAN achieves competitive performance against existing state-of-the-arts, especially Scenimefy as the baseline model.
生成式人工智能已经改变了动画产业。已经开发了几种图像到图像的翻译模型,特别专注于通过非配对翻译将现实世界图像转换为动漫。Scenimefy是一种利用对比学习的方法,通过半监督训练解决配对数据有限的问题,实现了高保真动漫场景翻译。然而,它依赖于精细调整的动漫领域StyleGAN的配对数据,因此存在局限性,常常产生低质量的数据集。此外,Scenimefy的高参数架构也提供了计算优化的机会。本研究介绍了NijiGAN,这是一个结合神经常微分方程(NeuralODEs)的新模型,与传统的残差网络相比,它在连续变换建模方面具有独特优势。NijiGAN成功地将现实世界场景转变为高保真动漫视觉,使用的是Scenimefy一半的参数。它采用通过Scenimefy生成的伪配对数据进行监督训练,消除了对低质量配对数据的依赖,改进了训练过程。我们的综合评估包括消融研究、定性和定量分析,比较了NijiGAN与类似模型的表现。测试结果表明,NijiGAN产生的图像质量高于AnimeGAN,平均意见得分(MOS)为2.192,超过了AnimeGAN的MOS得分2.160。此外,我们的模型达到了Frechet Inception Distance(FID)得分58.71,优于Scenimefy的FID得分60.32。这些结果表明,NijiGAN在现有先进技术中表现具有竞争力,尤其是相对于基线模型Scenimefy。
论文及项目相关链接
Summary
生成式人工智能已经改变了动画产业。最新研究引入了NijiGAN模型,它结合了神经常微分方程(NeuralODEs),能够实现真实场景到高质量动漫视觉的转换。该模型通过伪配对数据实现监督训练,提高了训练过程的质量,并超越了现有的先进模型,如Scenimefy和AnimeGAN。
Key Takeaways
- 生成式AI在动画产业中发挥着重要作用,特别是图像转换领域。
- Scenimefy采用对比学习处理配对数据不足的问题,但仍存在质量低和数据集限制的问题。
- NijiGAN模型结合神经常微分方程(NeuralODEs)实现了真实场景到高质量动漫图像的转换。
- NijiGAN通过伪配对数据实现监督训练,消除了对低质量配对数据的依赖。
- NijiGAN的训练结果相较于AnimeGAN有更高的质量,体现在Mean Opinion Score(MOS)和Frechet Inception Distance(FID)得分上。
- NijiGAN在性能上超越了基准模型Scenimefy。
- NijiGAN模型具有优化计算参数的机会。
点此查看论文截图
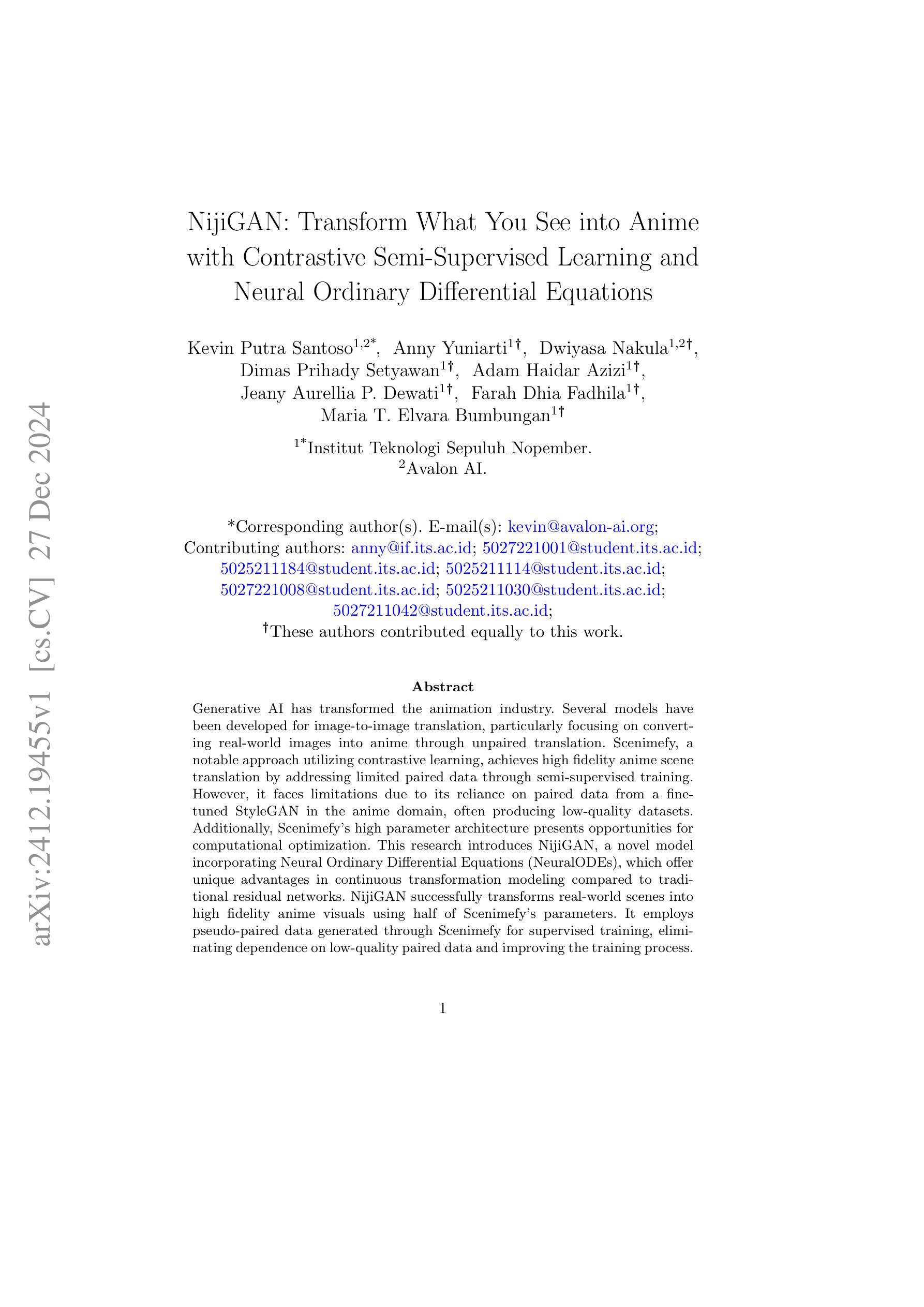
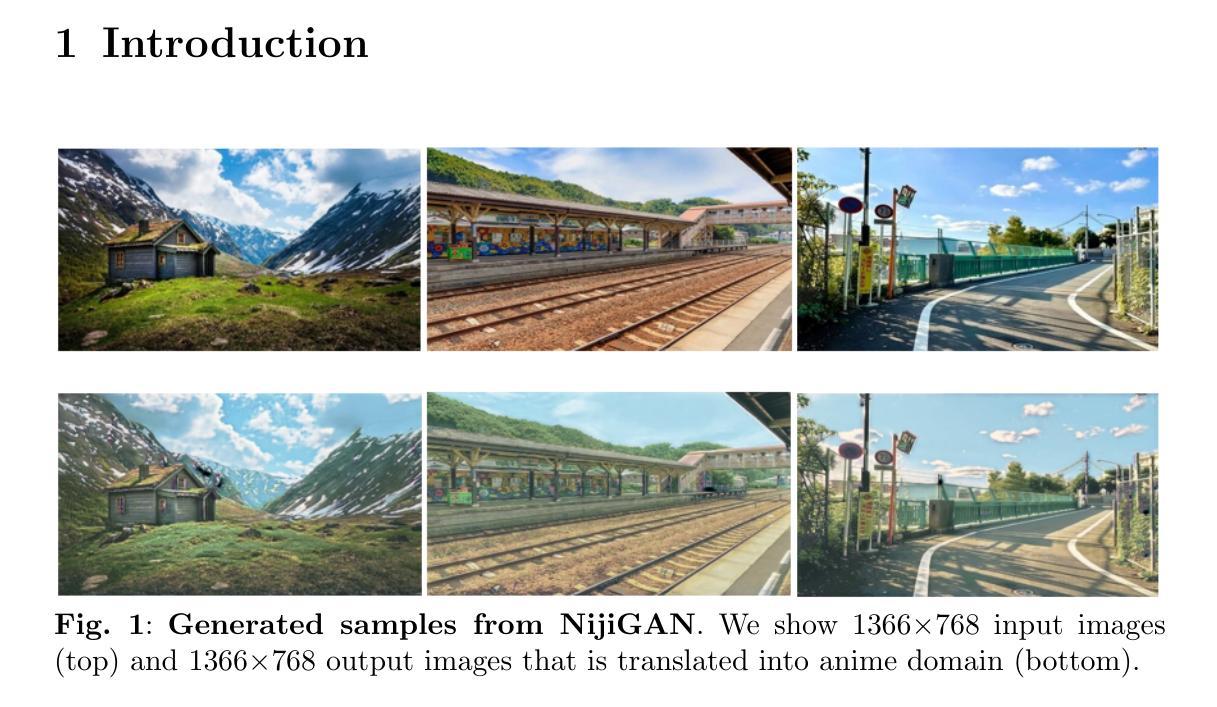
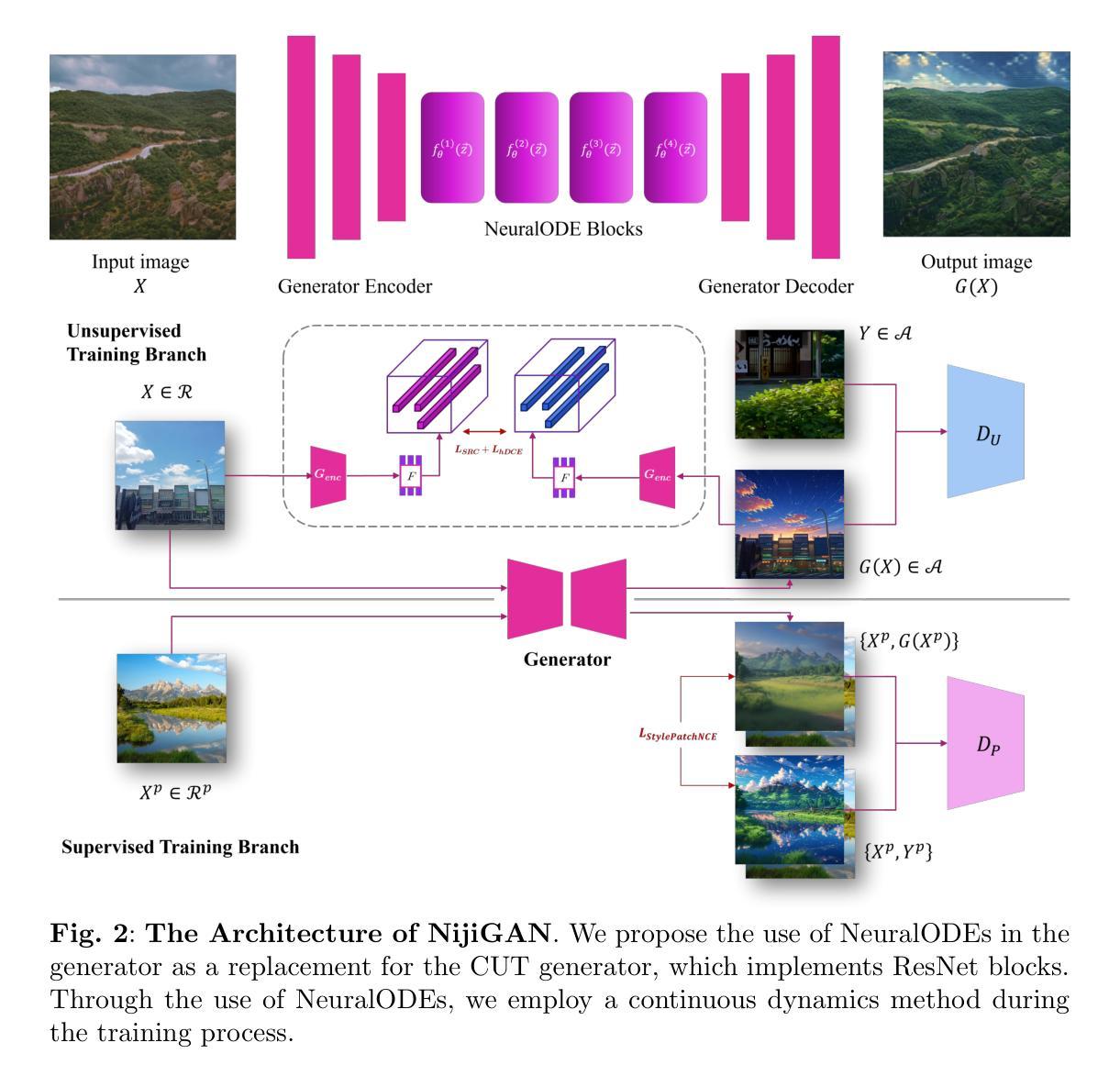