⚠️ 以下所有内容总结都来自于 大语言模型的能力,如有错误,仅供参考,谨慎使用
🔴 请注意:千万不要用于严肃的学术场景,只能用于论文阅读前的初筛!
💗 如果您觉得我们的项目对您有帮助 ChatPaperFree ,还请您给我们一些鼓励!⭐️ HuggingFace免费体验
2025-01-02 更新
Sparse Anatomical Prompt Semi-Supervised Learning with Masked Image Modeling for CBCT Tooth Segmentation
Authors:Pengyu Dai, Yafei Ou, Yuqiao Yang, Yang Liu, Yue Zhao
Accurate tooth identification and segmentation in Cone Beam Computed Tomography (CBCT) dental images can significantly enhance the efficiency and precision of manual diagnoses performed by dentists. However, existing segmentation methods are mainly developed based on large data volumes training, on which their annotations are extremely time-consuming. Meanwhile, the teeth of each class in CBCT dental images being closely positioned, coupled with subtle inter-class differences, gives rise to the challenge of indistinct boundaries when training model with limited data. To address these challenges, this study aims to propose a tasked-oriented Masked Auto-Encoder paradigm to effectively utilize large amounts of unlabeled data to achieve accurate tooth segmentation with limited labeled data. Specifically, we first construct a self-supervised pre-training framework of masked auto encoder to efficiently utilize unlabeled data to enhance the network performance. Subsequently, we introduce a sparse masked prompt mechanism based on graph attention to incorporate boundary information of the teeth, aiding the network in learning the anatomical structural features of teeth. To the best of our knowledge, we are pioneering the integration of the mask pre-training paradigm into the CBCT tooth segmentation task. Extensive experiments demonstrate both the feasibility of our proposed method and the potential of the boundary prompt mechanism.
在锥形束计算机断层扫描(CBCT)牙齿图像中进行准确的牙齿识别和分割,可以显著提高牙医进行手动诊断的效率和精度。然而,现有的分割方法主要基于大量数据进行开发训练,而这些数据的标注极为耗时。同时,CBCT牙齿图像中每一类牙齿位置紧密,类间差异细微,使用有限数据训练模型时面临边界不清晰的问题。本研究旨在提出一种面向任务的Masked Auto-Encoder范式,以有效利用大量无标签数据,在有限的有标签数据下实现准确的牙齿分割。具体来说,我们首先构建了一个基于自监督的预训练框架的掩码自动编码器,以有效利用无标签数据提高网络性能。随后,我们引入了一种基于图注意力的稀疏掩码提示机制,以融入牙齿的边界信息,帮助网络学习牙齿的解剖结构特征。据我们所知,我们是首次将掩码预训练范式集成到CBCT牙齿分割任务中。大量实验证明了我们方法的可行性以及边界提示机制的潜力。
论文及项目相关链接
PDF accepted by ISBI 2024
Summary
在锥束计算机断层扫描(CBCT)牙齿图像中准确进行牙齿识别和分割,能显著提高牙医手动诊断的效率和精确度。然而,现有分割方法主要基于大量数据进行训练,标注工作极为耗时。在CBCT牙齿图像中,各类牙齿紧密排列,加上微妙的类间差异,使得在有限数据训练模型时存在边界模糊的挑战。为解决这些挑战,本研究提出任务导向的掩码自动编码器范式,有效利用大量未标记数据,实现用少量标记数据进行准确牙齿分割。我们构建了自我监督的预训练框架,引入基于图注意的稀疏掩模提示机制,结合牙齿的边界信息,帮助网络学习牙齿的解剖结构特征。
Key Takeaways
- 准确牙齿识别和分割在CBCT牙齿图像诊断中的重要性。
- 现有牙齿分割方法面临的挑战:需要大量标注数据且边界模糊问题。
- 本研究提出任务导向的掩码自动编码器范式来解决上述问题。
- 利用自我监督预训练框架有效利用未标记数据提升网络性能。
- 引入基于图注意的稀疏掩模提示机制结合牙齿边界信息。
- 研究为CBCT牙齿分割任务引入了掩预训练范式的创新尝试。
点此查看论文截图
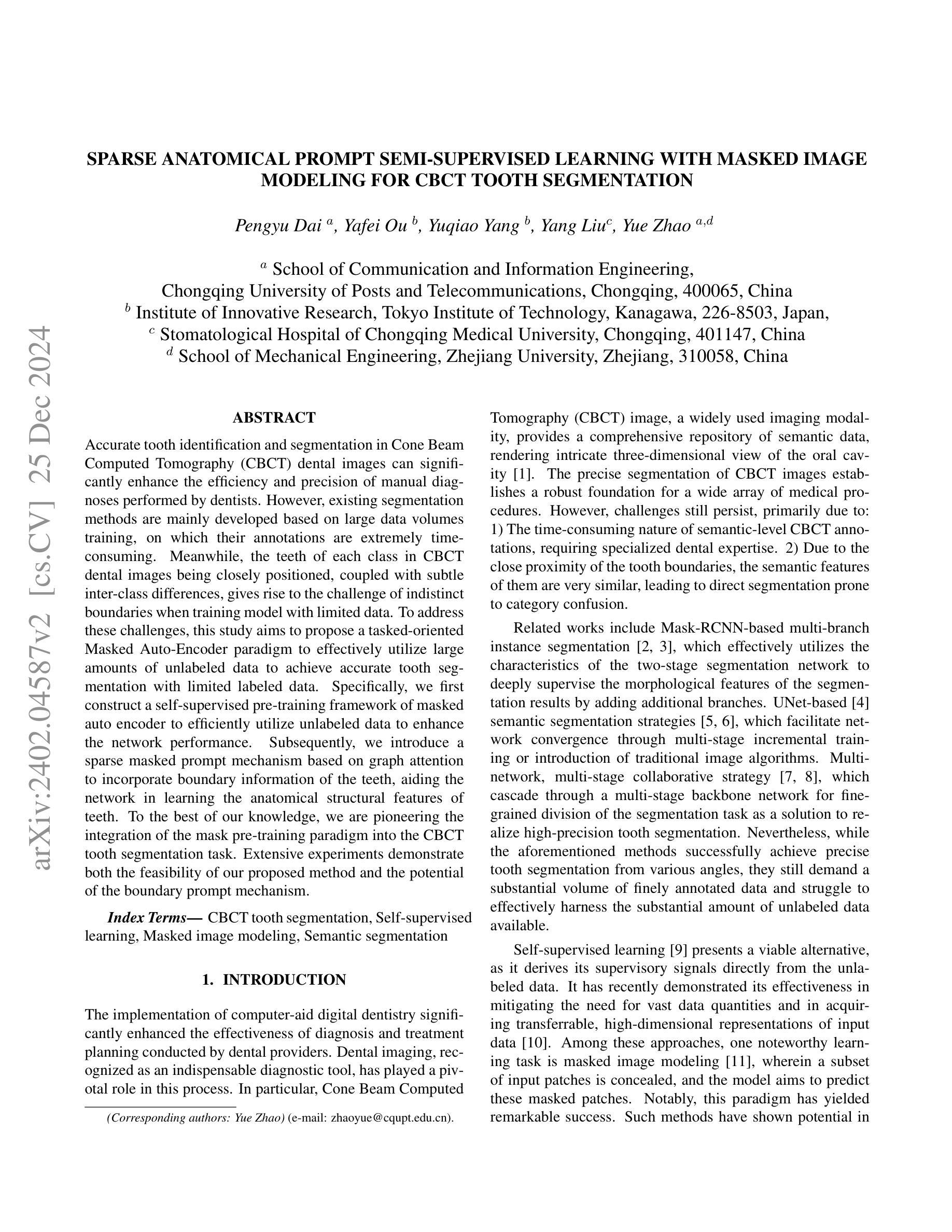
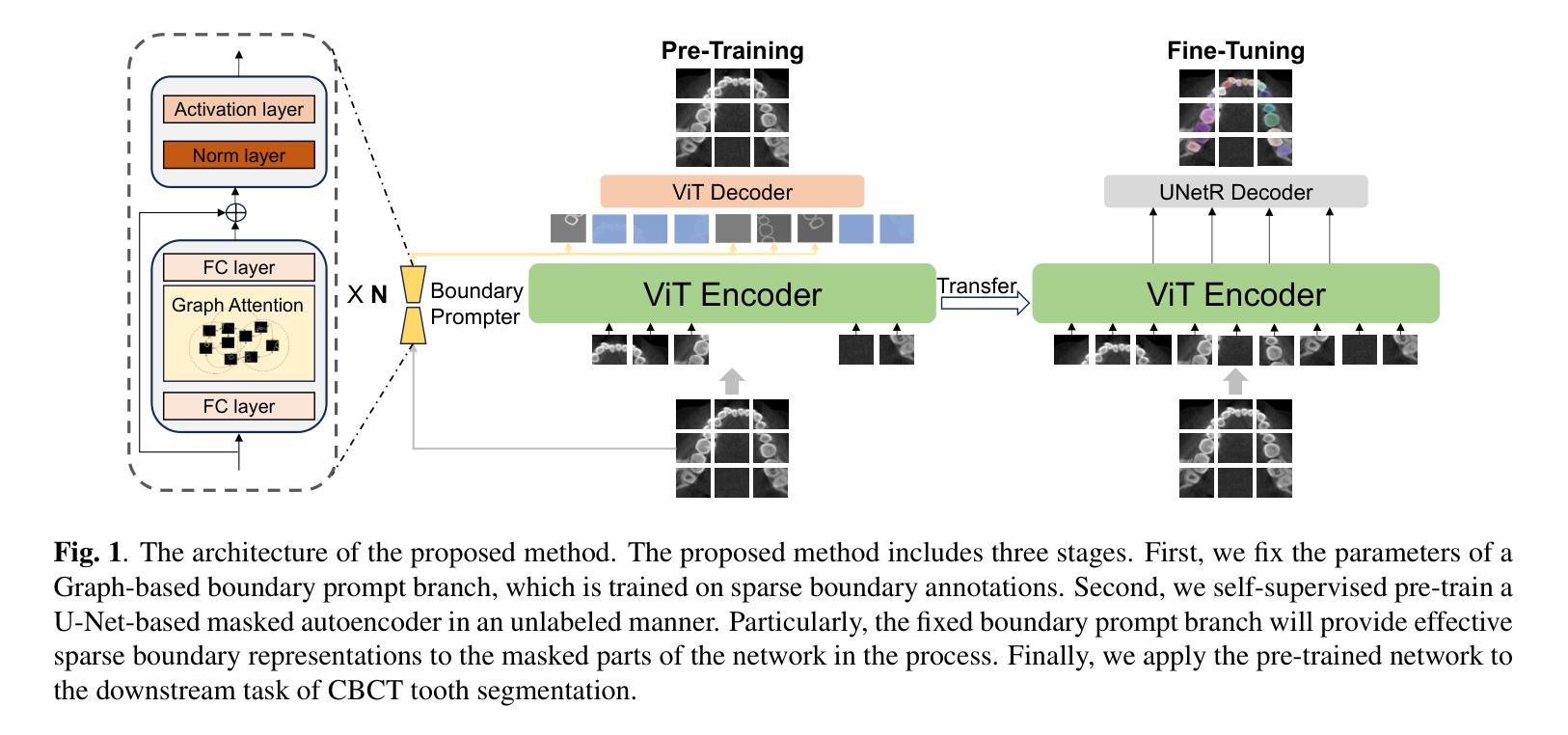
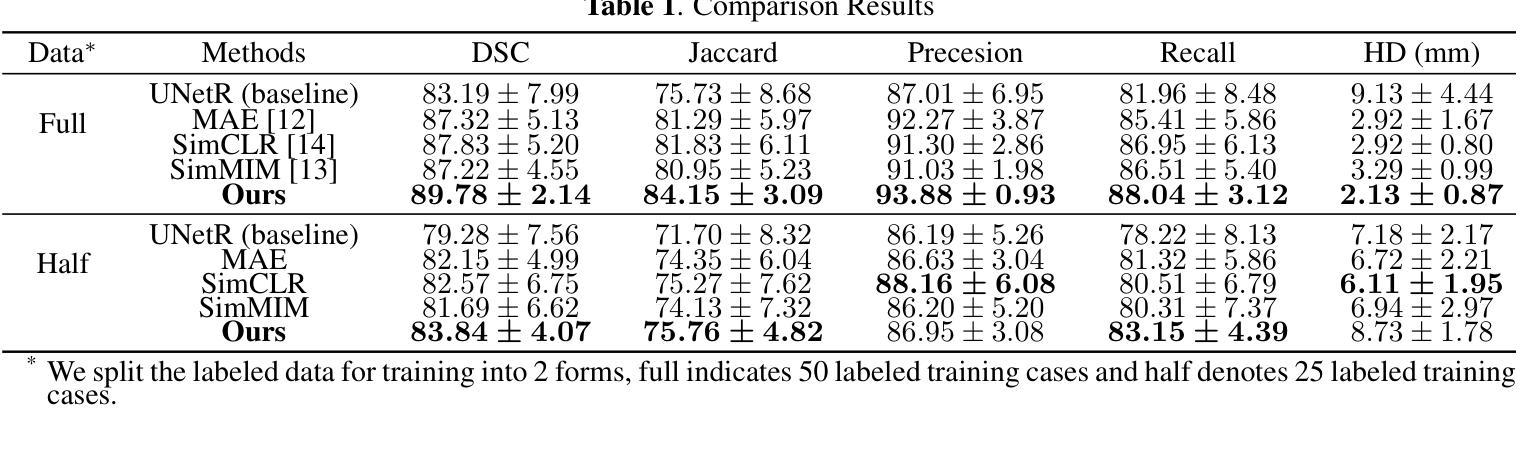
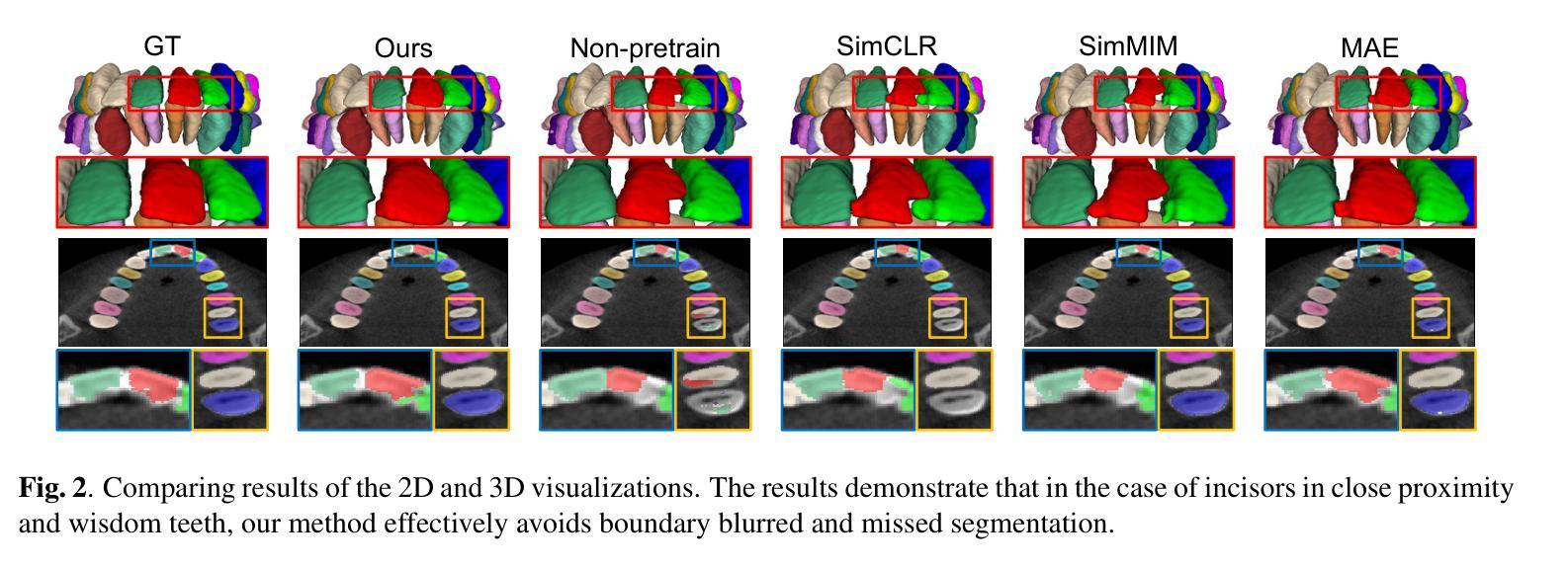