⚠️ 以下所有内容总结都来自于 大语言模型的能力,如有错误,仅供参考,谨慎使用
🔴 请注意:千万不要用于严肃的学术场景,只能用于论文阅读前的初筛!
💗 如果您觉得我们的项目对您有帮助 ChatPaperFree ,还请您给我们一些鼓励!⭐️ HuggingFace免费体验
2025-01-02 更新
ReFlow6D: Refraction-Guided Transparent Object 6D Pose Estimation via Intermediate Representation Learning
Authors:Hrishikesh Gupta, Stefan Thalhammer, Jean-Baptiste Weibel, Alexander Haberl, Markus Vincze
Transparent objects are ubiquitous in daily life, making their perception and robotics manipulation important. However, they present a major challenge due to their distinct refractive and reflective properties when it comes to accurately estimating the 6D pose. To solve this, we present ReFlow6D, a novel method for transparent object 6D pose estimation that harnesses the refractive-intermediate representation. Unlike conventional approaches, our method leverages a feature space impervious to changes in RGB image space and independent of depth information. Drawing inspiration from image matting, we model the deformation of the light path through transparent objects, yielding a unique object-specific intermediate representation guided by light refraction that is independent of the environment in which objects are observed. By integrating these intermediate features into the pose estimation network, we show that ReFlow6D achieves precise 6D pose estimation of transparent objects, using only RGB images as input. Our method further introduces a novel transparent object compositing loss, fostering the generation of superior refractive-intermediate features. Empirical evaluations show that our approach significantly outperforms state-of-the-art methods on TOD and Trans32K-6D datasets. Robot grasping experiments further demonstrate that ReFlow6D’s pose estimation accuracy effectively translates to real-world robotics task. The source code is available at: https://github.com/StoicGilgamesh/ReFlow6D and https://github.com/StoicGilgamesh/matting_rendering.
透明物体在日常生活中无处不在,因此它们的感知和机器人操作非常重要。然而,它们在准确估计6D姿态时,由于其独特的折射和反射属性,呈现出重大挑战。为解决此问题,我们提出了ReFlow6D,这是一种用于透明物体6D姿态估计的新方法,它利用折射中间表示。与传统的方法不同,我们的方法利用一个不受RGB图像空间变化影响的特征空间,并且独立于深度信息。我们从图像抠图中汲取灵感,对光线通过透明物体的路径变形进行建模,产生了一种独特的、以光线折射引导的对象特定中间表示,它独立于观察物体的环境。通过将这些中间特征集成到姿态估计网络中,我们表明ReFlow6D仅使用RGB图像作为输入,就能实现对透明物体的精确6D姿态估计。我们的方法还引入了一种新的透明物体合成损失,促进生成优质折射中间特征。经验评估表明,我们的方法在TOD和Trans32K-6D数据集上显著优于最新方法。机器人抓取实验进一步证明,ReFlow6D的姿态估计精度有效地转化为现实世界的机器人任务。源代码可在以下链接中找到:https://github.com/StoicGilgamesh/ReFlow6D和https://github.com/StoicGilgamesh/matting_rendering。
论文及项目相关链接
Summary
本文介绍了一种名为ReFlow6D的新型透明物体6D姿态估计方法。该方法利用折射中间表示,通过模拟光线通过透明物体的路径变形,实现对透明物体的精准姿态估计。仅使用RGB图像作为输入,该方法就能达成目标,并在TOD和Trans32K-6D数据集上显著优于现有方法。此外,该方法还引入了一种新的透明物体复合损失,以生成更优质的折射中间特征。ReFlow6D的成功应用在机器人抓取实验中得到了验证。
Key Takeaways
- ReFlow6D是一种用于透明物体6D姿态估计的新型方法。
- 该方法利用折射中间表示,通过模拟光线在透明物体内部的路径变形来实现精确姿态估计。
- ReFlow6D使用RGB图像作为输入,不需要深度信息。
- ReFlow6D引入了透明物体复合损失,提升了折射中间特征的生成质量。
- ReFlow6D在TOD和Trans32K-6D数据集上的表现显著优于现有方法。
- 机器人抓取实验验证了ReFlow6D在实际应用中的有效性。
点此查看论文截图
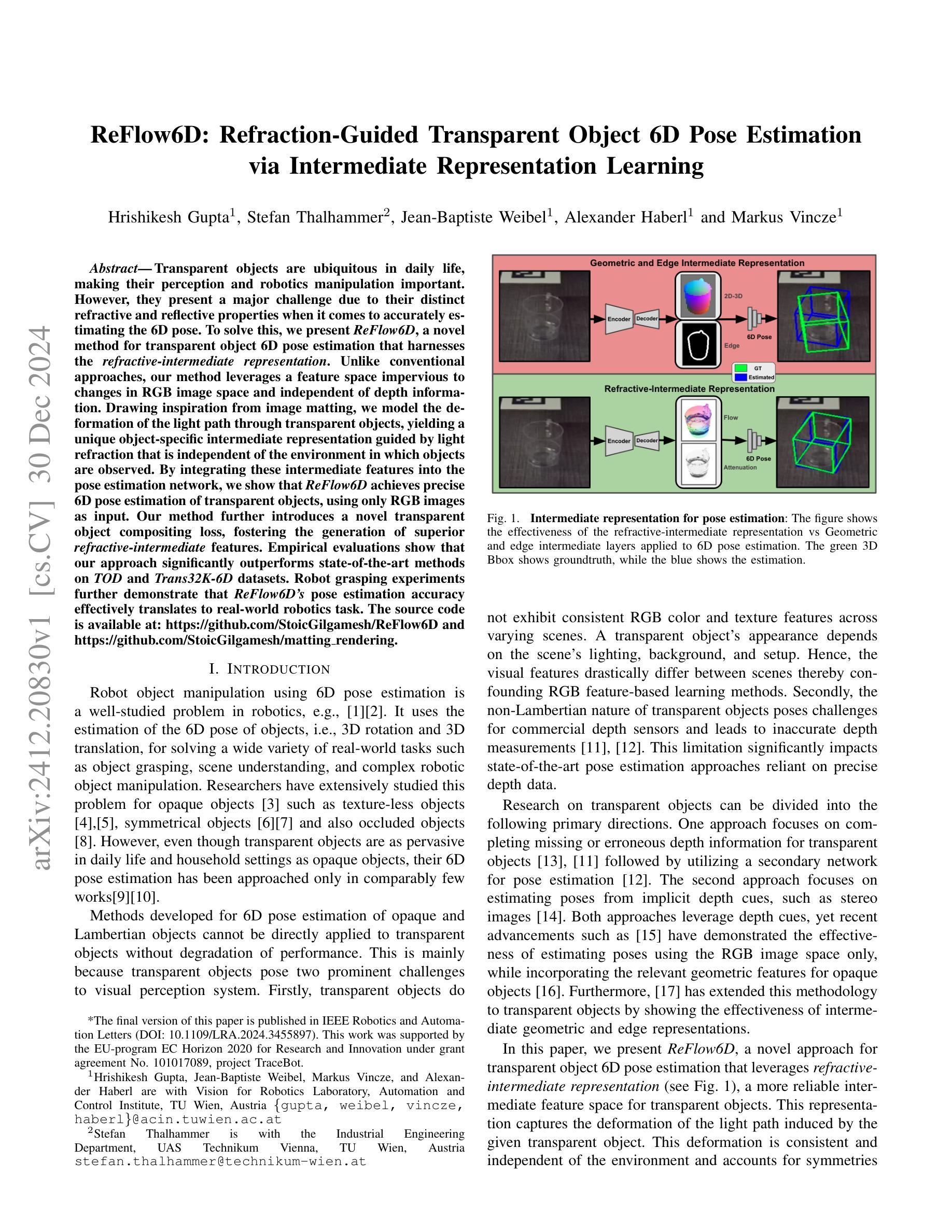
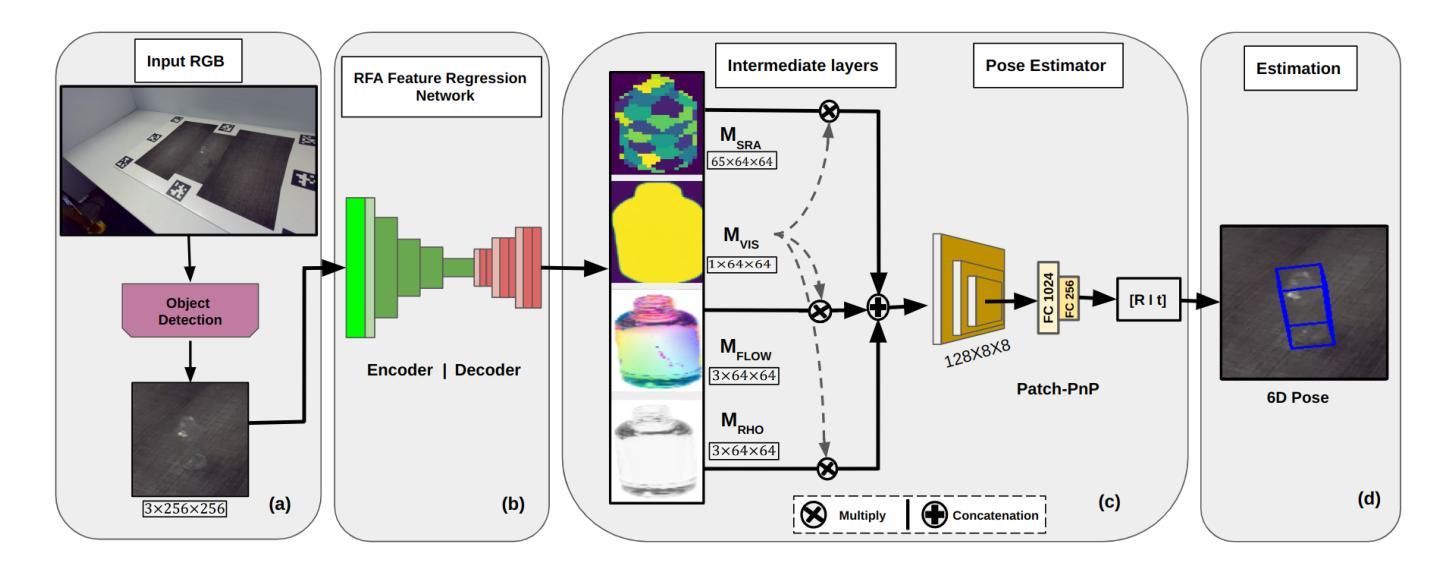
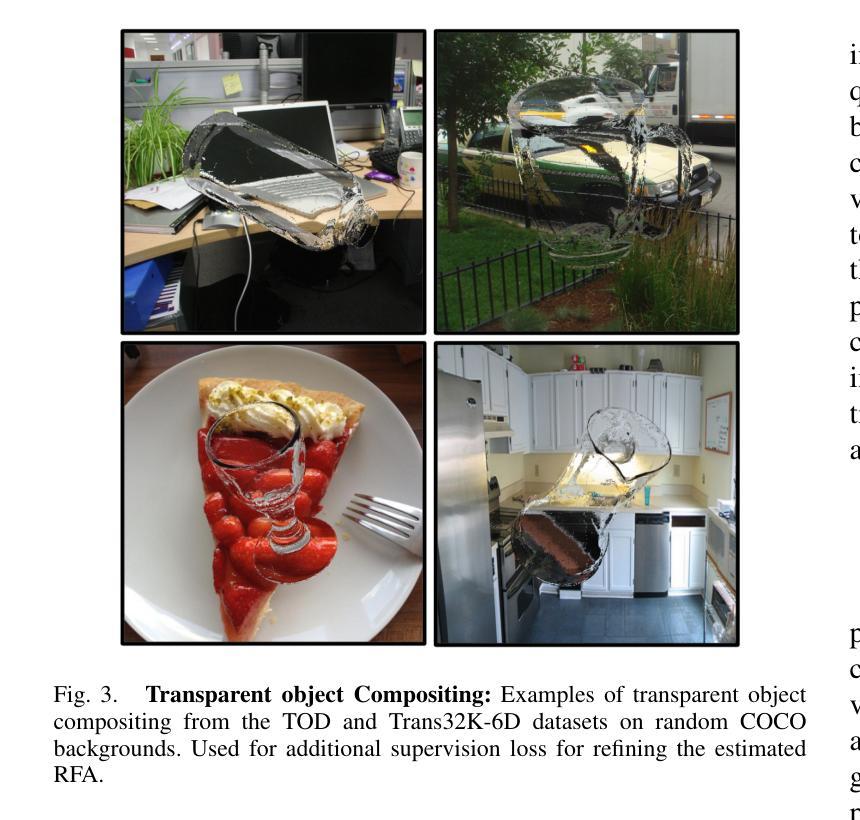
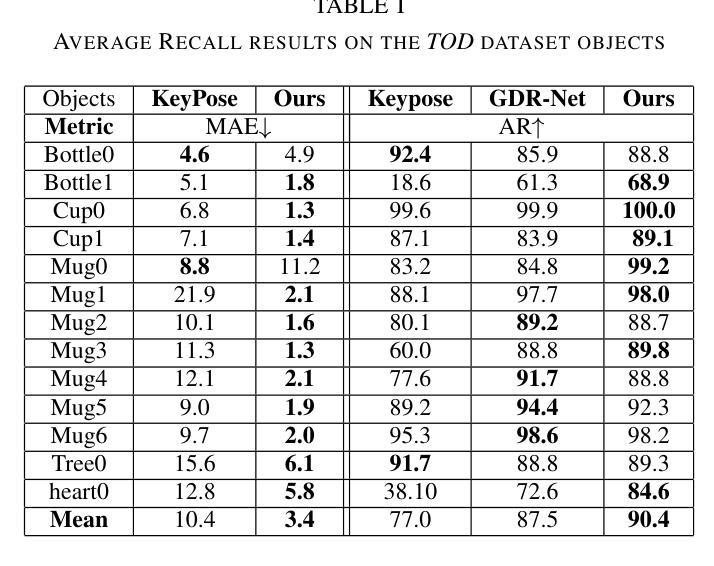
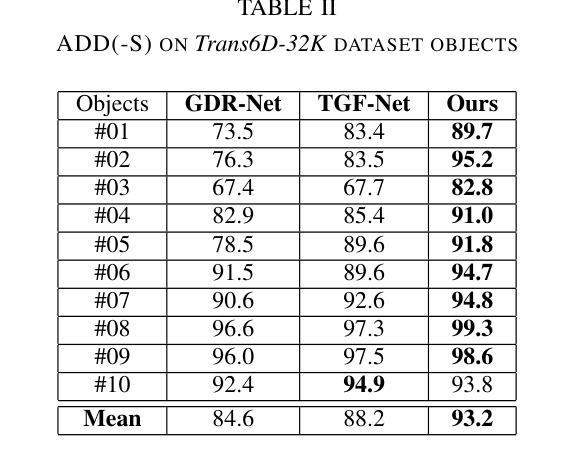
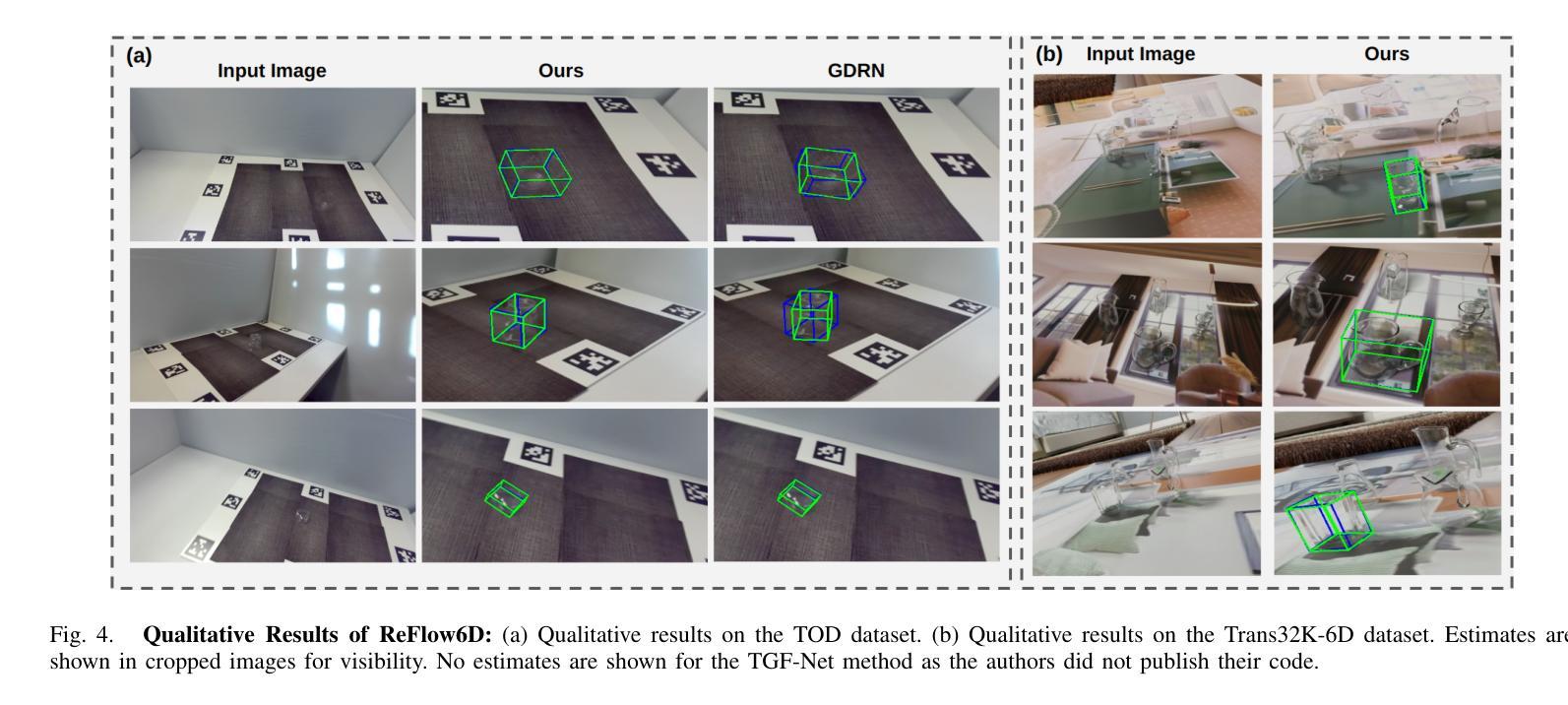
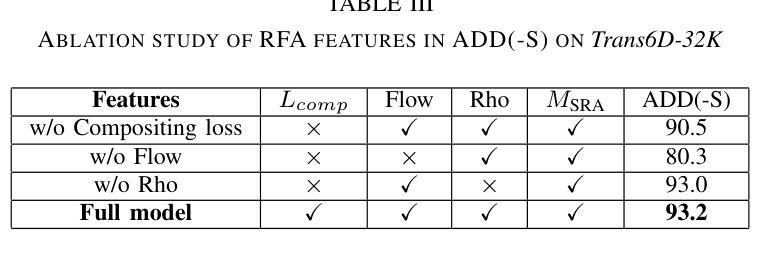
An Ordinary Differential Equation Sampler with Stochastic Start for Diffusion Bridge Models
Authors:Yuang Wang, Pengfei Jin, Li Zhang, Quanzheng Li, Zhiqiang Chen, Dufan Wu
Diffusion bridge models have demonstrated promising performance in conditional image generation tasks, such as image restoration and translation, by initializing the generative process from corrupted images instead of pure Gaussian noise. However, existing diffusion bridge models often rely on Stochastic Differential Equation (SDE) samplers, which result in slower inference speed compared to diffusion models that employ high-order Ordinary Differential Equation (ODE) solvers for acceleration. To mitigate this gap, we propose a high-order ODE sampler with a stochastic start for diffusion bridge models. To overcome the singular behavior of the probability flow ODE (PF-ODE) at the beginning of the reverse process, a posterior sampling approach was introduced at the first reverse step. The sampling was designed to ensure a smooth transition from corrupted images to the generative trajectory while reducing discretization errors. Following this stochastic start, Heun’s second-order solver is applied to solve the PF-ODE, achieving high perceptual quality with significantly reduced neural function evaluations (NFEs). Our method is fully compatible with pretrained diffusion bridge models and requires no additional training. Extensive experiments on image restoration and translation tasks, including super-resolution, JPEG restoration, Edges-to-Handbags, and DIODE-Outdoor, demonstrated that our sampler outperforms state-of-the-art methods in both visual quality and Frechet Inception Distance (FID).
扩散桥模型在条件图像生成任务中表现出了良好的性能,例如图像恢复和翻译,它通过从损坏的图像而不是纯高斯噪声开始生成过程。然而,现有的扩散桥模型通常依赖于随机微分方程(SDE)采样器,与采用高阶常微分方程(ODE)求解器进行加速的扩散模型相比,推理速度较慢。为了弥补这一差距,我们为扩散桥模型提出了一种具有随机起始的高阶ODE采样器。为了解决概率流ODE(PF-ODE)在反向过程开始时出现的奇异行为,我们在第一次反向步骤中引入了后采样方法。采样的设计是为了确保从损坏的图像到生成轨迹的平稳过渡,同时减少离散化误差。随后采用Heun的二阶求解器解决PF-ODE,在保证感知质量的同时大大减少神经功能评估(NFEs)。我们的方法与预训练的扩散桥模型完全兼容,无需额外训练。在图像恢复和翻译任务的大量实验,包括超分辨率、JPEG恢复、“边缘到手袋”和DIODE-Outdoor等任务,证明我们的采样器在视觉质量和Frechet Inception Distance(FID)方面都优于最先进的方法。
论文及项目相关链接
PDF 9 pages, 5 figures, This work has been submitted to the IEEE for possible publication
Summary:
扩散桥模型通过从失真图像而非纯高斯噪声开始生成过程,在图像恢复和翻译等条件图像生成任务中展现出良好性能。为缩短与采用高阶常微分方程(ODE)求解器进行加速的扩散模型的推理速度差距,我们提出了带有随机起始的高阶ODE采样器。针对反向过程中概率流常微分方程(PF-ODE)在起始处的奇异行为,我们在第一次反向步骤中引入了后采样方法,确保从失真图像到生成轨迹的平滑过渡,同时减少离散化误差。随后使用Heun的二阶求解器解决PF-ODE,在显著降低神经功能评估(NFEs)的同时实现高感知质量。我们的方法与预训练的扩散桥模型完全兼容,无需额外训练。在图像恢复和翻译任务的大量实验中,包括超分辨率、JPEG恢复、Edges-to-Handbags和DIODE-Outdoor等任务,我们的采样器在视觉质量和Frechet Inception Distance(FID)方面都优于最新方法。
Key Takeaways:
- 扩散桥模型能从失真图像开始生成过程,在条件图像生成任务中有良好表现。
- 现有扩散桥模型常使用随机微分方程(SDE)采样器,推理速度较慢。
- 为提高推理速度,提出带有随机起始的高阶ODE采样器。
- 在反向过程的起始阶段引入后采样方法,确保从失真图像到生成轨迹的平滑过渡。
- 使用Heun的二阶求解器解决概率流常微分方程(PF-ODE),提高感知质量并降低神经功能评估。
- 方法与预训练的扩散桥模型兼容,无需额外训练。
点此查看论文截图
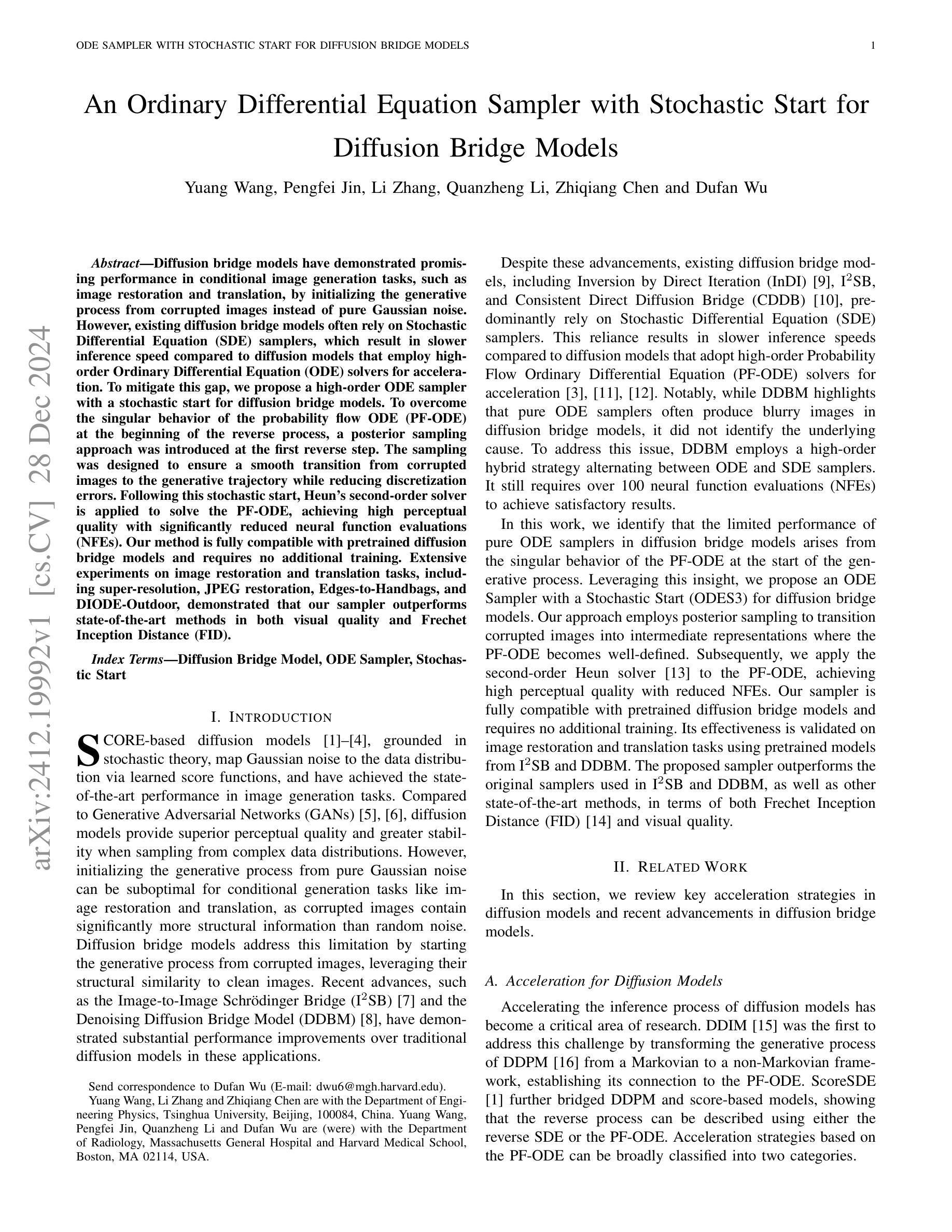
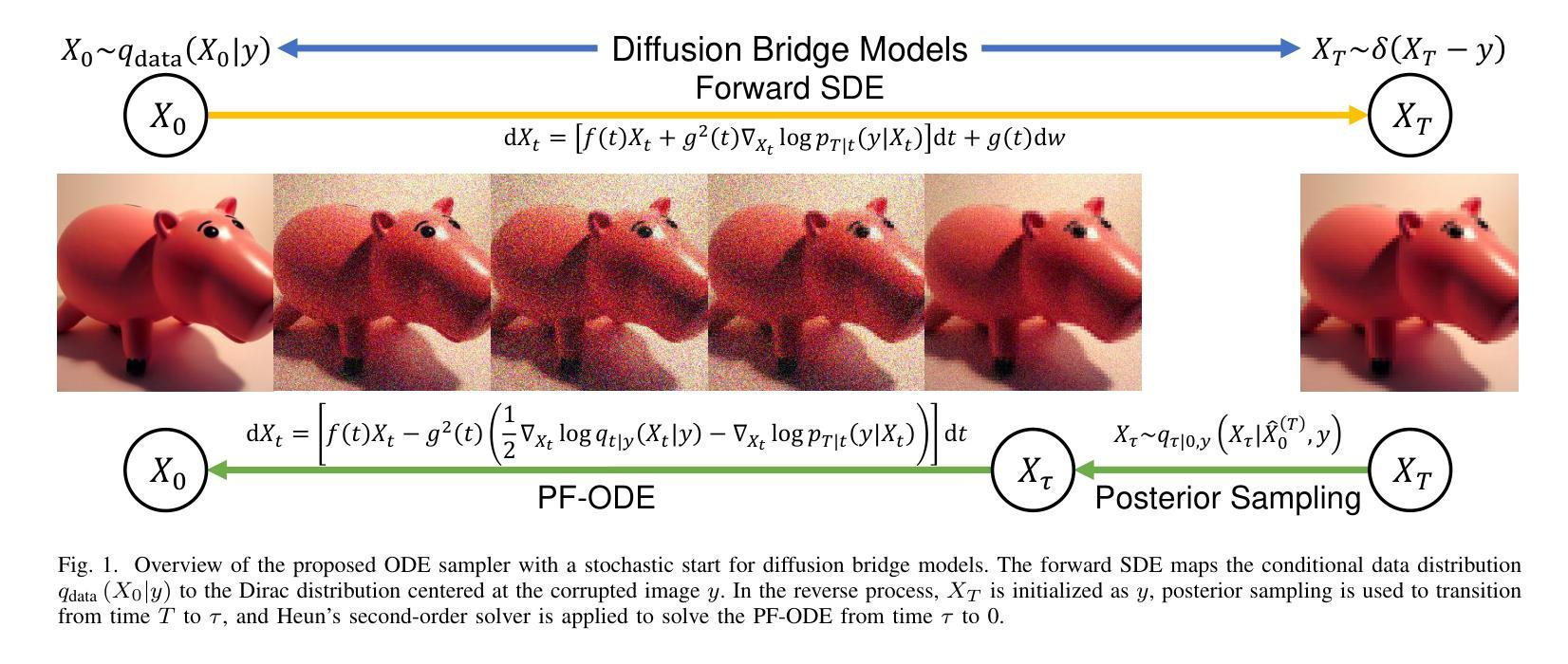
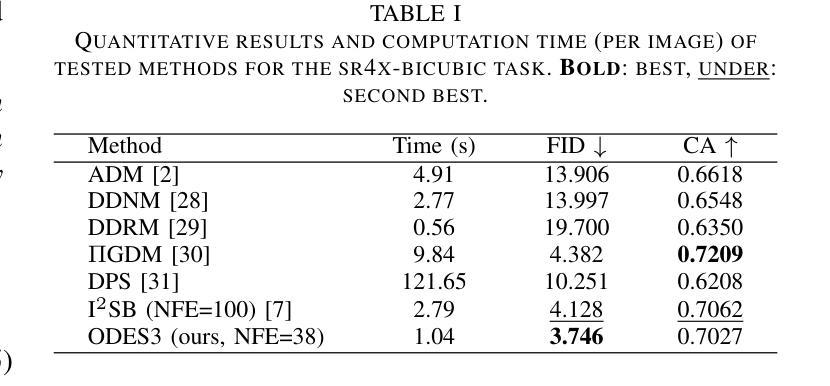
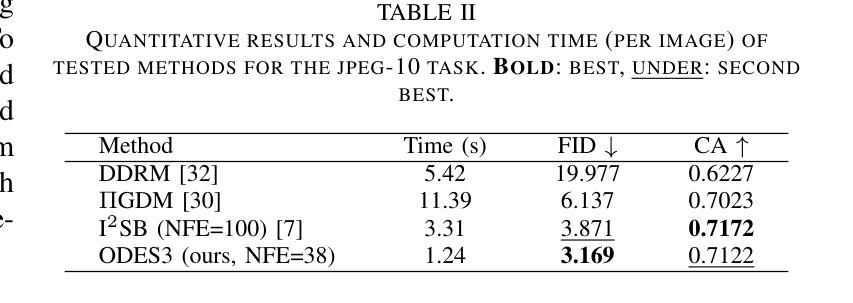
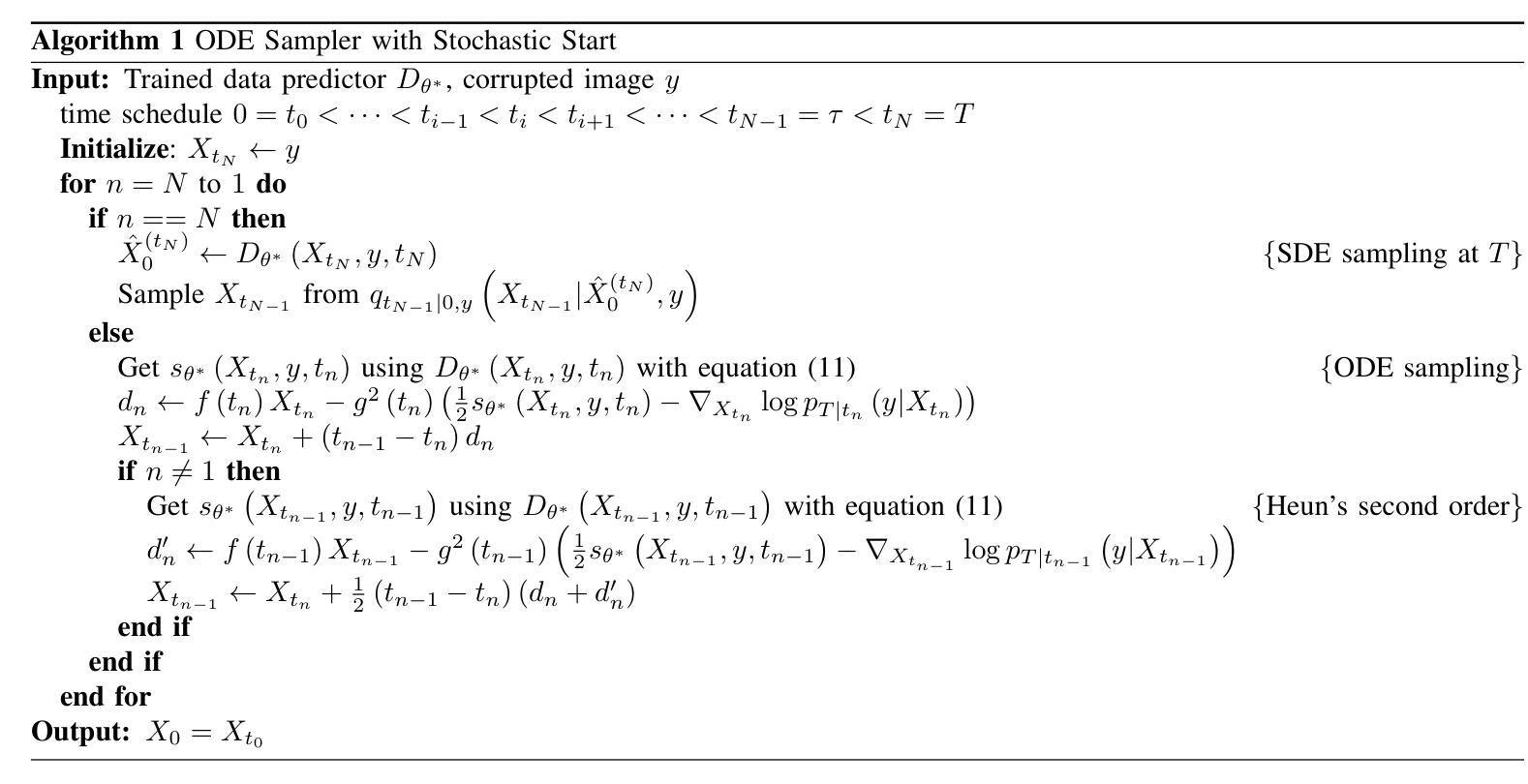
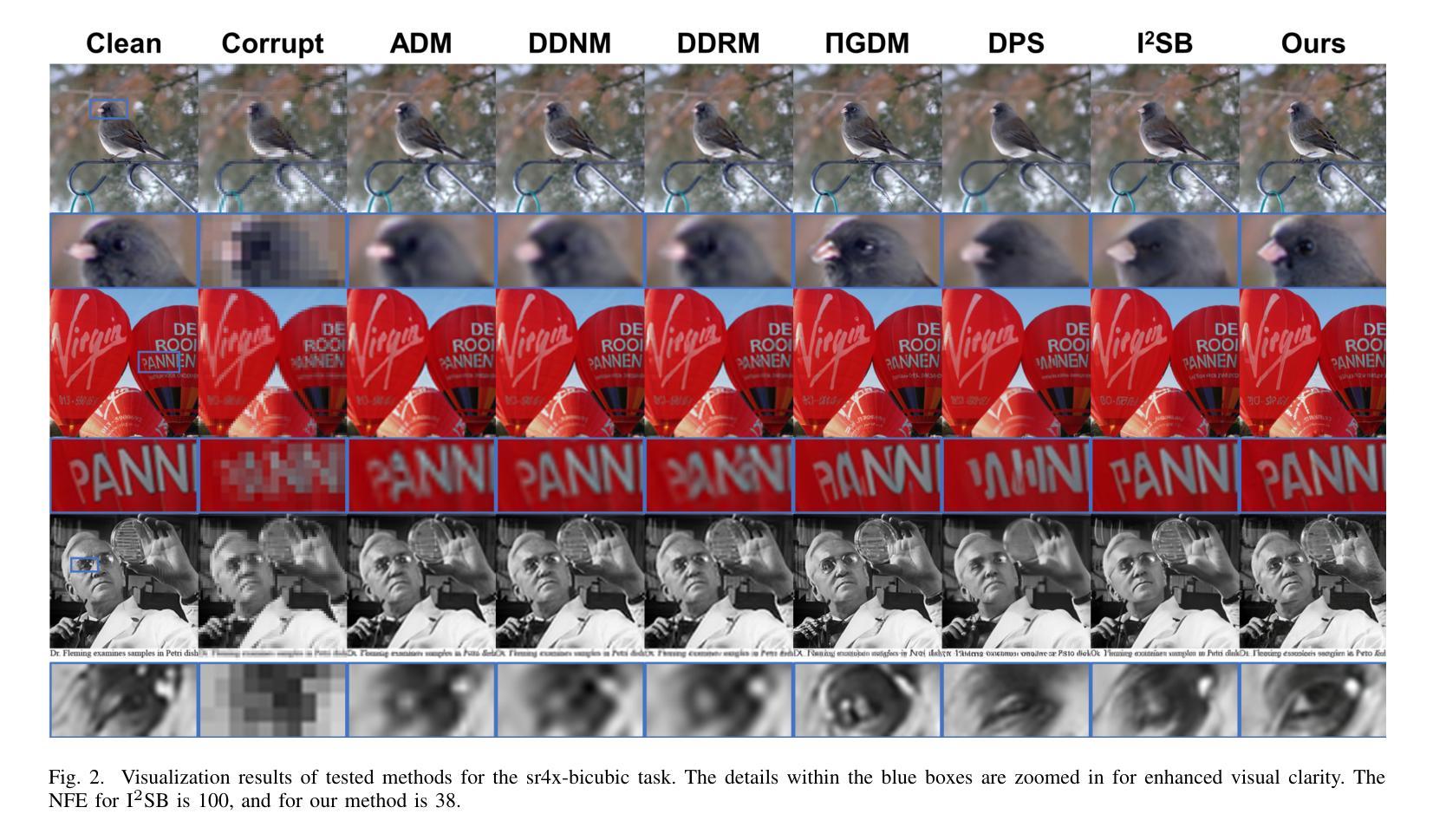
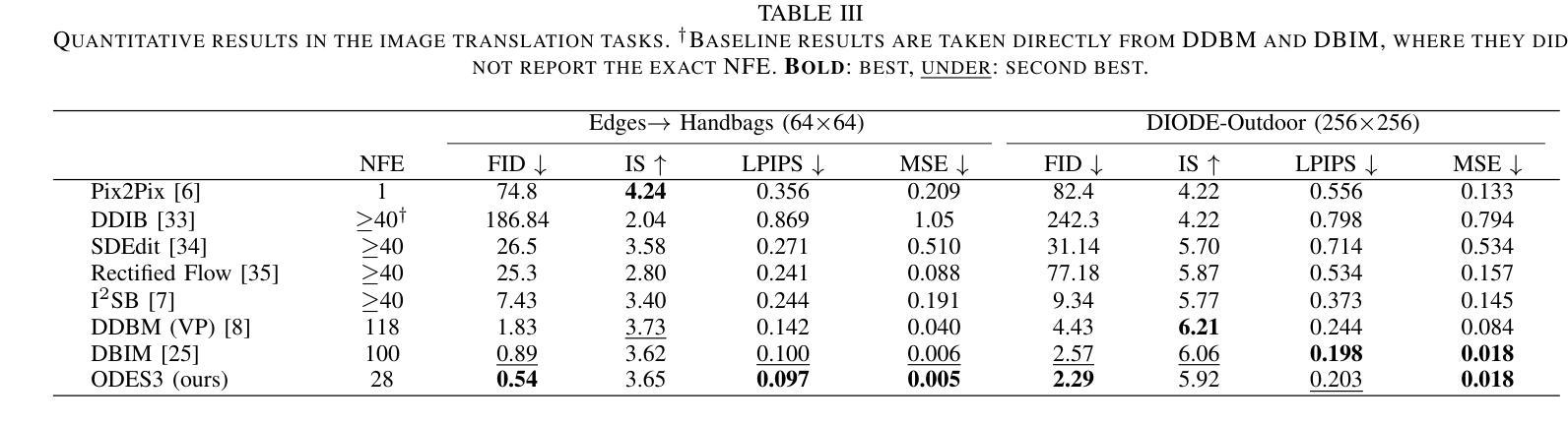
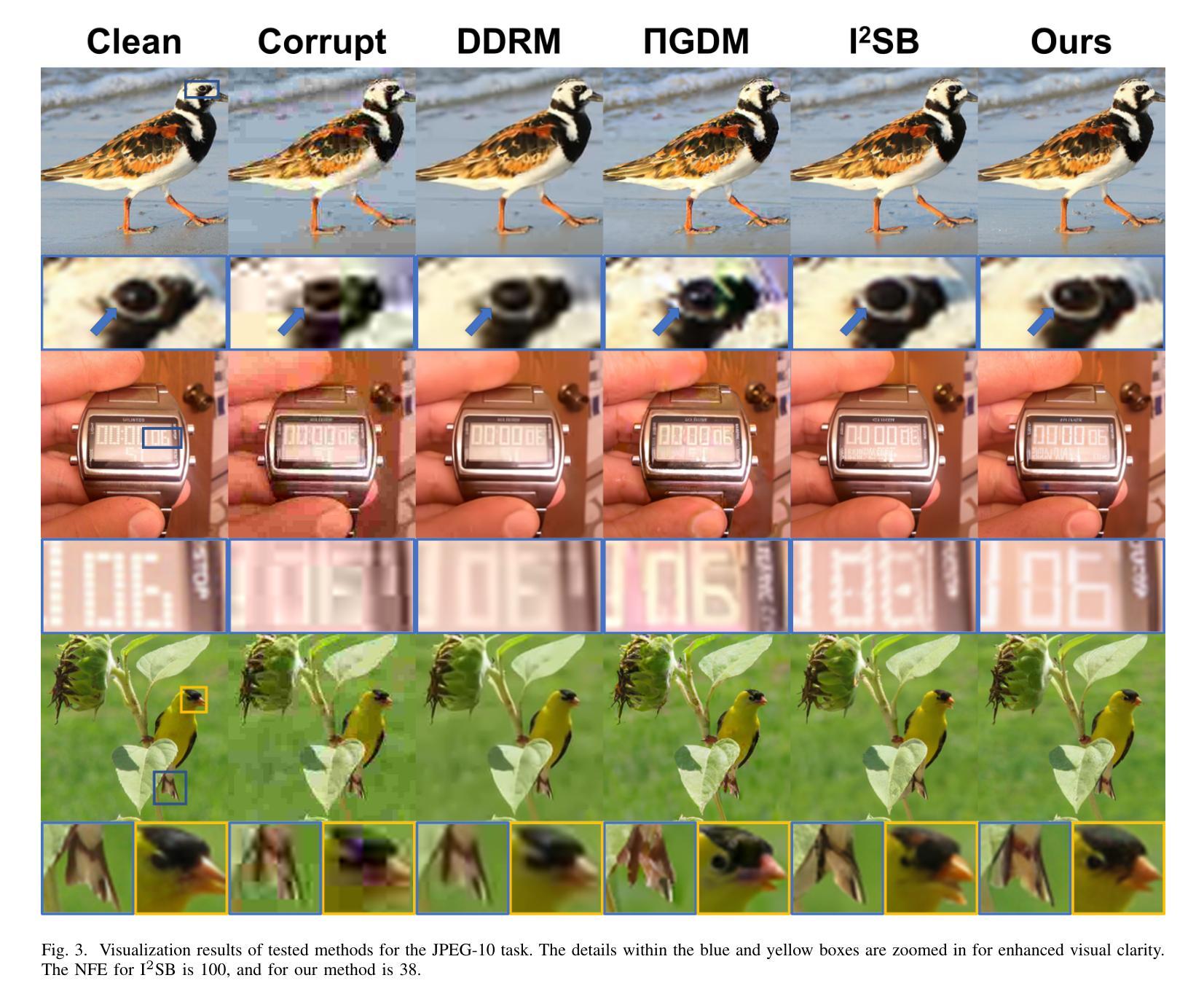
CAD-GPT: Synthesising CAD Construction Sequence with Spatial Reasoning-Enhanced Multimodal LLMs
Authors:Siyu Wang, Cailian Chen, Xinyi Le, Qimin Xu, Lei Xu, Yanzhou Zhang, Jie Yang
Computer-aided design (CAD) significantly enhances the efficiency, accuracy, and innovation of design processes by enabling precise 2D and 3D modeling, extensive analysis, and optimization. Existing methods for creating CAD models rely on latent vectors or point clouds, which are difficult to obtain and costly to store. Recent advances in Multimodal Large Language Models (MLLMs) have inspired researchers to use natural language instructions and images for CAD model construction. However, these models still struggle with inferring accurate 3D spatial location and orientation, leading to inaccuracies in determining the spatial 3D starting points and extrusion directions for constructing geometries. This work introduces CAD-GPT, a CAD synthesis method with spatial reasoning-enhanced MLLM that takes either a single image or a textual description as input. To achieve precise spatial inference, our approach introduces a 3D Modeling Spatial Mechanism. This method maps 3D spatial positions and 3D sketch plane rotation angles into a 1D linguistic feature space using a specialized spatial unfolding mechanism, while discretizing 2D sketch coordinates into an appropriate planar space to enable precise determination of spatial starting position, sketch orientation, and 2D sketch coordinate translations. Extensive experiments demonstrate that CAD-GPT consistently outperforms existing state-of-the-art methods in CAD model synthesis, both quantitatively and qualitatively.
计算机辅助设计(CAD)通过实现精确的2D和3D建模、全面的分析和优化,显著提高了设计过程的效率、准确性和创新性。现有的创建CAD模型的方法依赖于潜在向量或点云,这些难以获得且成本高昂,不利于存储。最近多模态大型语言模型(MLLMs)的进步启发研究人员使用自然语言指令和图像进行CAD模型构建。然而,这些模型在推断精确的3D空间位置和方向时仍面临困难,导致在确定构建几何体的空间3D起点和挤压方向时出现不准确。本研究介绍了CAD-GPT,这是一种带有空间推理增强型MLLM的CAD合成方法,它可以将单张图像或文本描述作为输入。为了精确实现空间推断,我们的方法引入了一种3D建模空间机制。该方法利用专用的空间展开机制将3D空间位置和3D草图平面旋转角度映射到一维语言特征空间中,同时将2D草图坐标离散化到适当的平面空间内,以实现空间起始位置、草图方向和2D草图坐标转换的精确确定。大量实验表明,无论是在数量上还是在质量上,CAD-GPT在CAD模型合成方面均持续超越现有先进技术。
论文及项目相关链接
Summary
CAD-GPT是一种结合多模态大型语言模型(MLLM)的计算机辅助设计(CAD)合成方法,通过引入3D建模空间机制,能够精确进行空间推理,实现从单一图像或文本描述生成CAD模型。该方法在合成CAD模型方面表现出卓越性能,超越现有技术。
Key Takeaways
- CAD设计通过使精确的2D和3D建模、广泛的分析和优化,显著提高了设计效率、准确性和创新性。
- 现有的CAD模型创建方法主要依赖难以获取和存储成本高昂的潜在向量或点云。
- 多模态大型语言模型(MLLM)的最新进展激发了使用自然语言指令和图像进行CAD模型构建的研究。
- CAD-GPT是一种新型的CAD合成方法,结合了空间推理增强的MLLM,可接受单一图像或文本描述作为输入来创建CAD模型。
- CAD-GPT通过引入3D建模空间机制,实现了精确的空间推理,将3D空间位置和旋转角度映射到1D语言特征空间。
- 该方法通过将2D草图坐标离散化到适当的平面空间,能够精确确定空间起始位置、草图方向和2D草图坐标转换。
点此查看论文截图
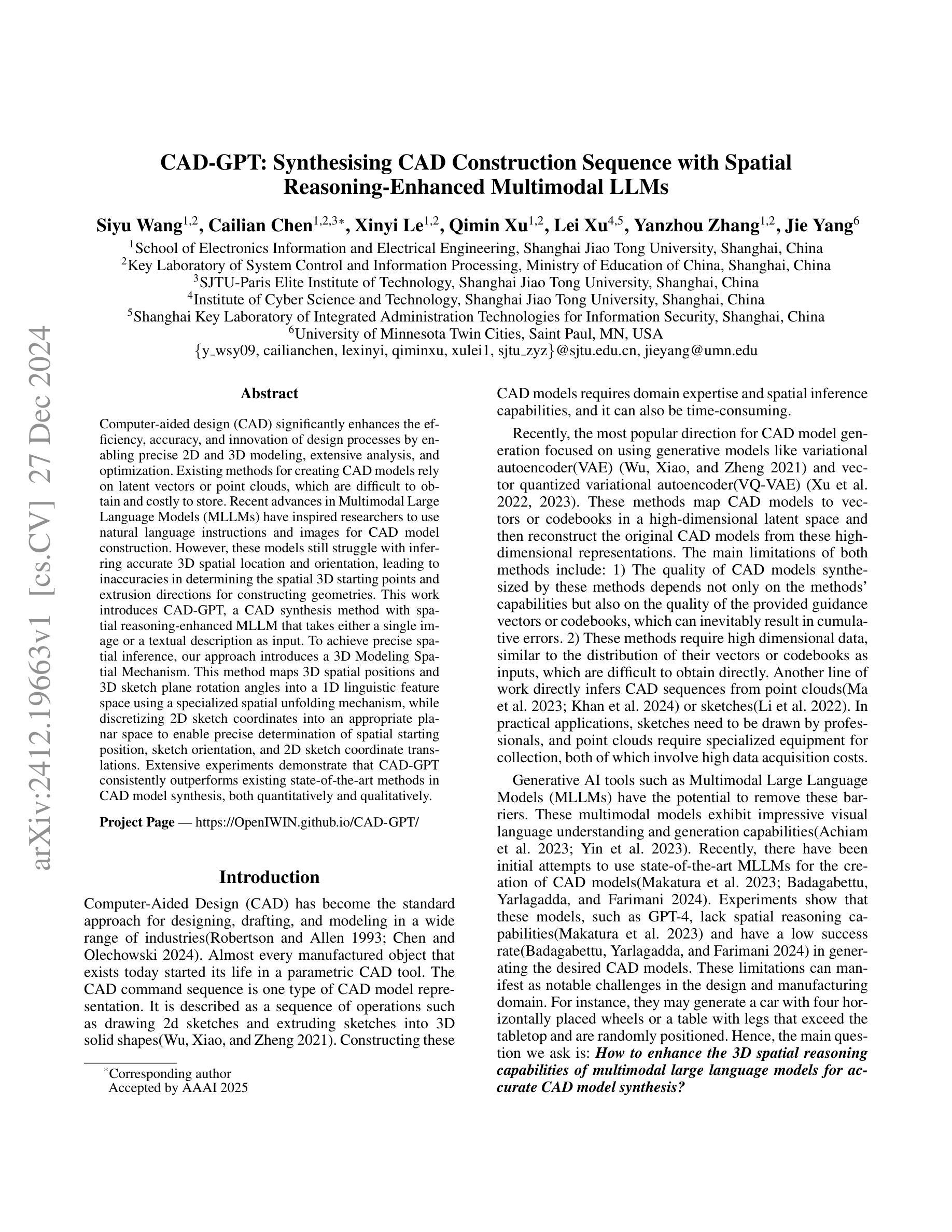
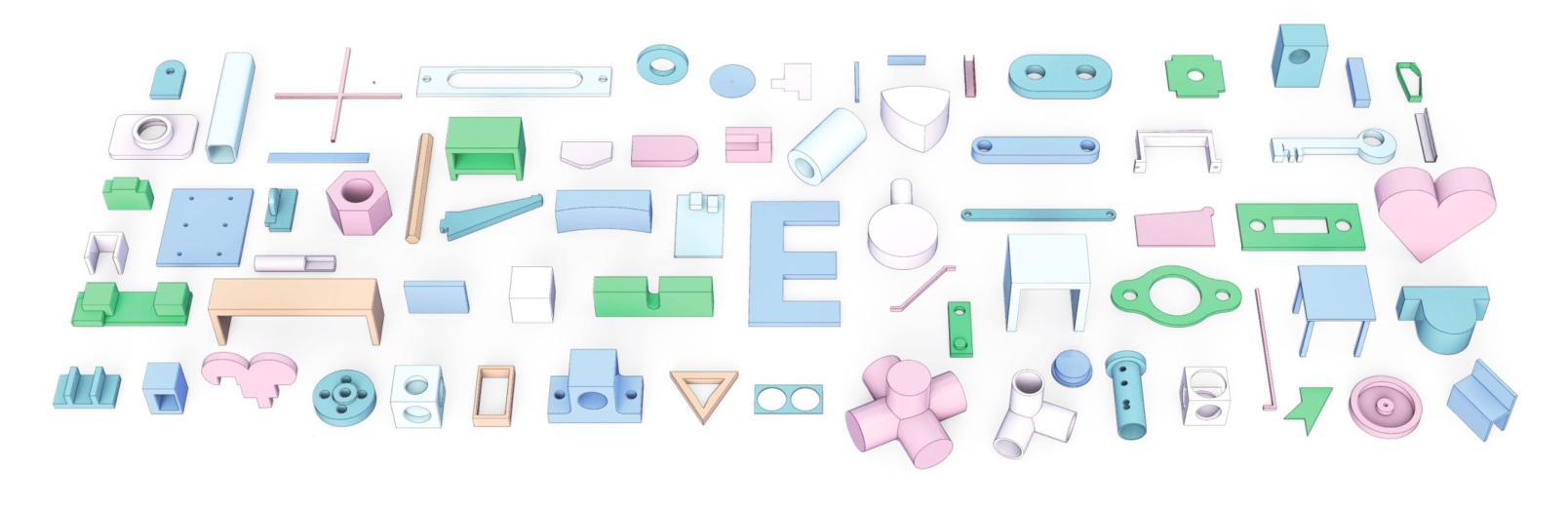
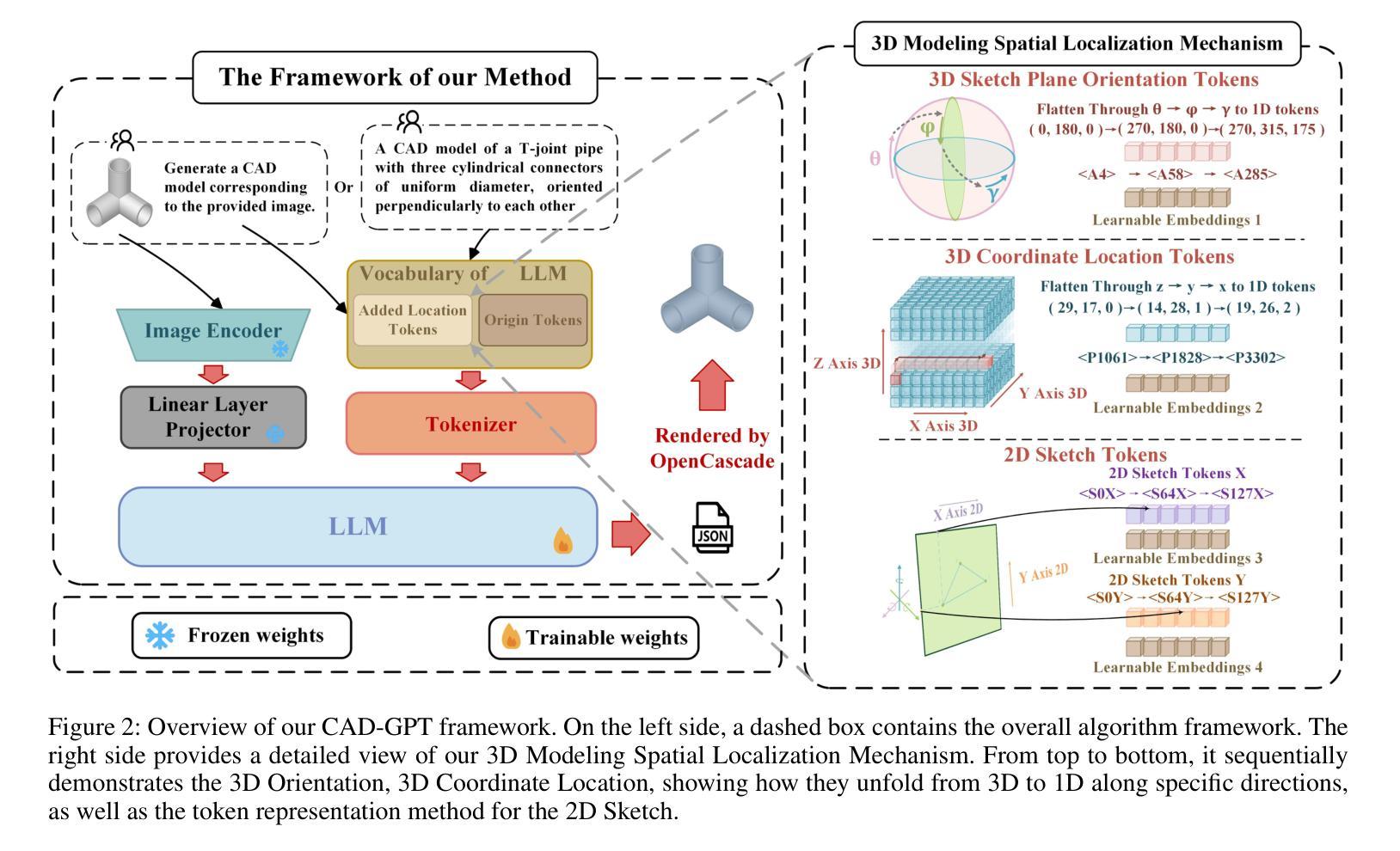
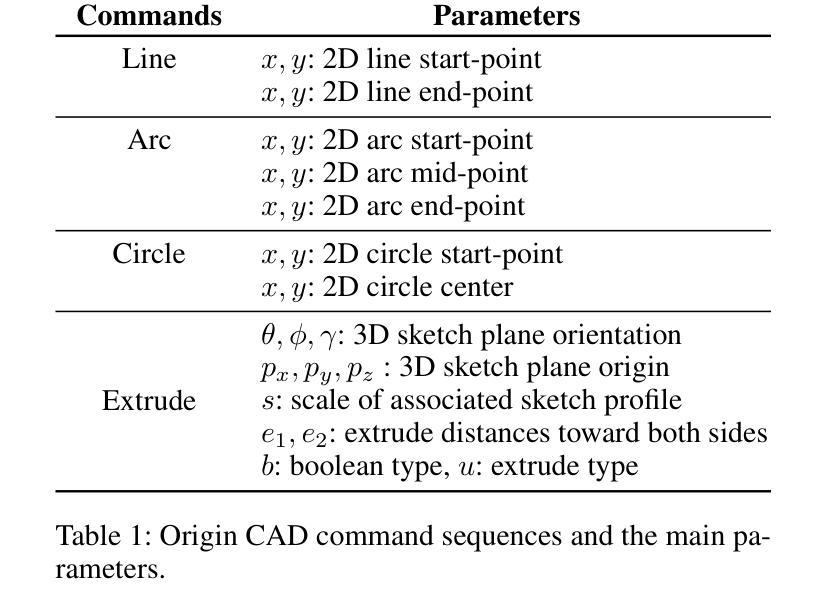
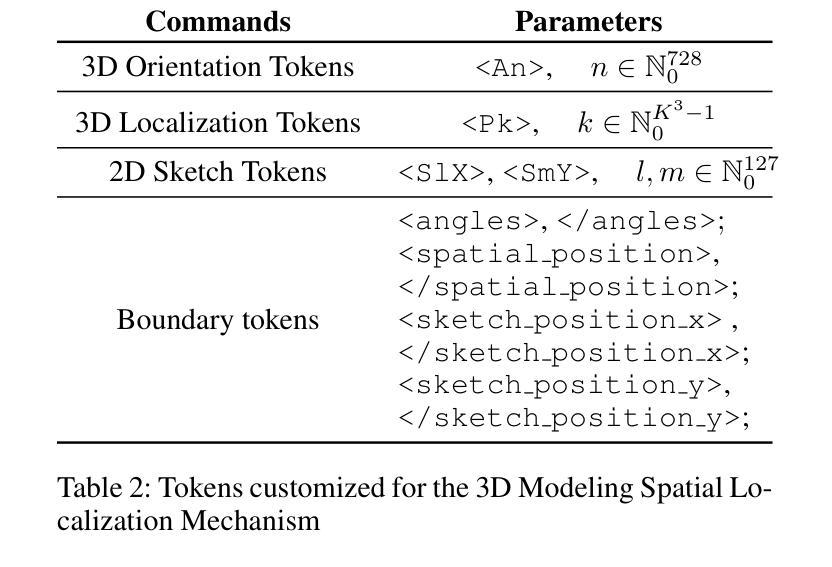
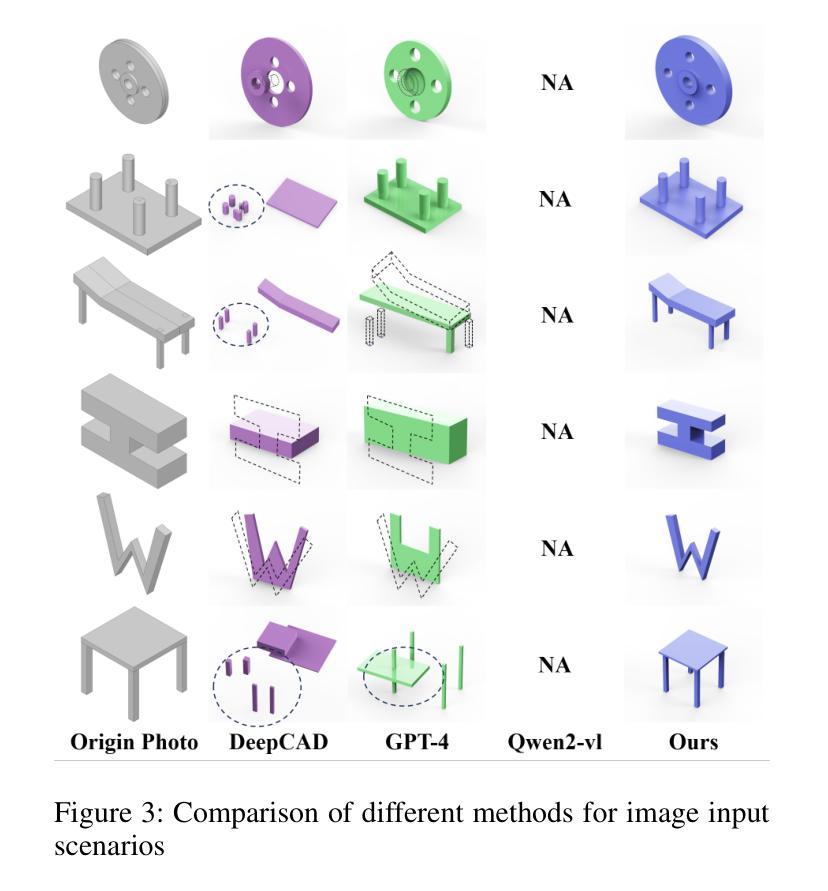
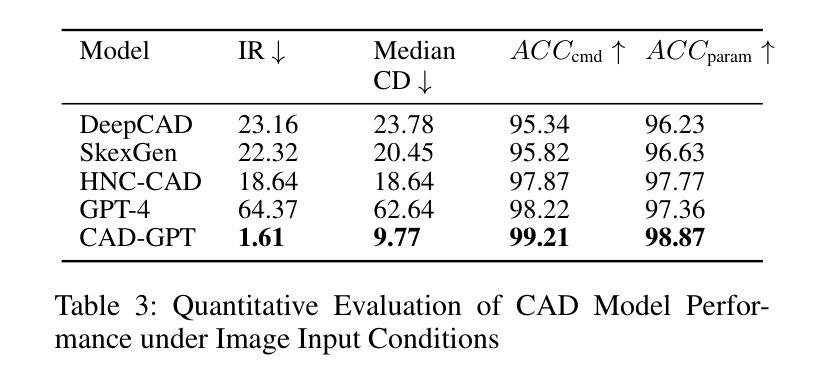
Structural Similarity in Deep Features: Image Quality Assessment Robust to Geometrically Disparate Reference
Authors:Keke Zhang, Weiling Chen, Tiesong Zhao, Zhou Wang
Image Quality Assessment (IQA) with references plays an important role in optimizing and evaluating computer vision tasks. Traditional methods assume that all pixels of the reference and test images are fully aligned. Such Aligned-Reference IQA (AR-IQA) approaches fail to address many real-world problems with various geometric deformations between the two images. Although significant effort has been made to attack Geometrically-Disparate-Reference IQA (GDR-IQA) problem, it has been addressed in a task-dependent fashion, for example, by dedicated designs for image super-resolution and retargeting, or by assuming the geometric distortions to be small that can be countered by translation-robust filters or by explicit image registrations. Here we rethink this problem and propose a unified, non-training-based Deep Structural Similarity (DeepSSIM) approach to address the above problems in a single framework, which assesses structural similarity of deep features in a simple but efficient way and uses an attention calibration strategy to alleviate attention deviation. The proposed method, without application-specific design, achieves state-of-the-art performance on AR-IQA datasets and meanwhile shows strong robustness to various GDR-IQA test cases. Interestingly, our test also shows the effectiveness of DeepSSIM as an optimization tool for training image super-resolution, enhancement and restoration, implying an even wider generalizability. \footnote{Source code will be made public after the review is completed.
图像质量评估(IQA)在优化和评估计算机视觉任务中起着重要作用。传统的方法假设参考图像和测试图像的所有像素完全对齐。这种对齐参考的IQA(AR-IQA)方法无法解决两个图像之间存在各种几何变形等现实世界的许多问题。尽管已经付出了巨大努力来解决几何分散参考IQA(GDR-IQA)问题,但它仍采用依赖于任务的方式解决,例如针对图像超分辨率和重新定位进行设计,或者假设几何畸变可以通过平移鲁棒滤波器或显式图像注册来抵消。在这里我们重新思考这个问题,提出了一个统一的、非基于训练的深度结构相似性(DeepSSIM)方法来解决上述问题在一个单一的框架内,它通过简单有效的方式评估深度特征的结构相似性,并使用注意力校准策略来缓解注意力偏差。该方法无需针对特定应用进行设计,即可在AR-IQA数据集上实现最先进的性能,同时在各种GDR-IQA测试用例中表现出强大的鲁棒性。有趣的是,我们的测试还显示了DeepSSIM作为图像超分辨率、增强和恢复的训练优化工具的有效性,这暗示了其更广泛的通用性。注:源代码将在审查完成后公开。
论文及项目相关链接
Summary
基于深度结构相似性(DeepSSIM)的图像质量评估方法重新考虑了几何失真图像质量评估问题。此方法无需训练,通过评估深度特征的结构相似性,并采用注意力校准策略减轻注意力偏差,在统一框架内解决对齐参考图像质量评估(AR-IQA)和几何离散参考图像质量评估(GDR-IQA)问题。该方法具有出色的性能,并且在多种GDR-IQA测试案例中表现出强大的稳健性。此外,DeepSSIM作为图像超分辨率、增强和修复的优化工具也表现出有效性,显示出更广泛的通用性。
Key Takeaways
- 传统图像质量评估方法假定参考和测试图像的所有像素完全对齐,但在现实世界中存在几何变形问题。
- 几何离散参考图像质量评估(GDR-IQA)问题虽已有研究,但仍以任务依赖的方式解决,需要针对特定任务设计解决方案。
- 论文提出了一种基于深度结构相似性(DeepSSIM)的统一方法来解决上述问题,无需特定应用设计。
- DeepSSIM通过评估深度特征的结构相似性并采用注意力校准策略来减轻注意力偏差。
- 该方法在AR-IQA数据集上实现了卓越性能,同时在多种GDR-IQA测试案例中表现出强大的稳健性。
- DeepSSIM作为图像超分辨率、增强和修复的优化工具的有效性得到了验证。
- 该方法暗示了DeepSSIM更广泛的通用性。
点此查看论文截图
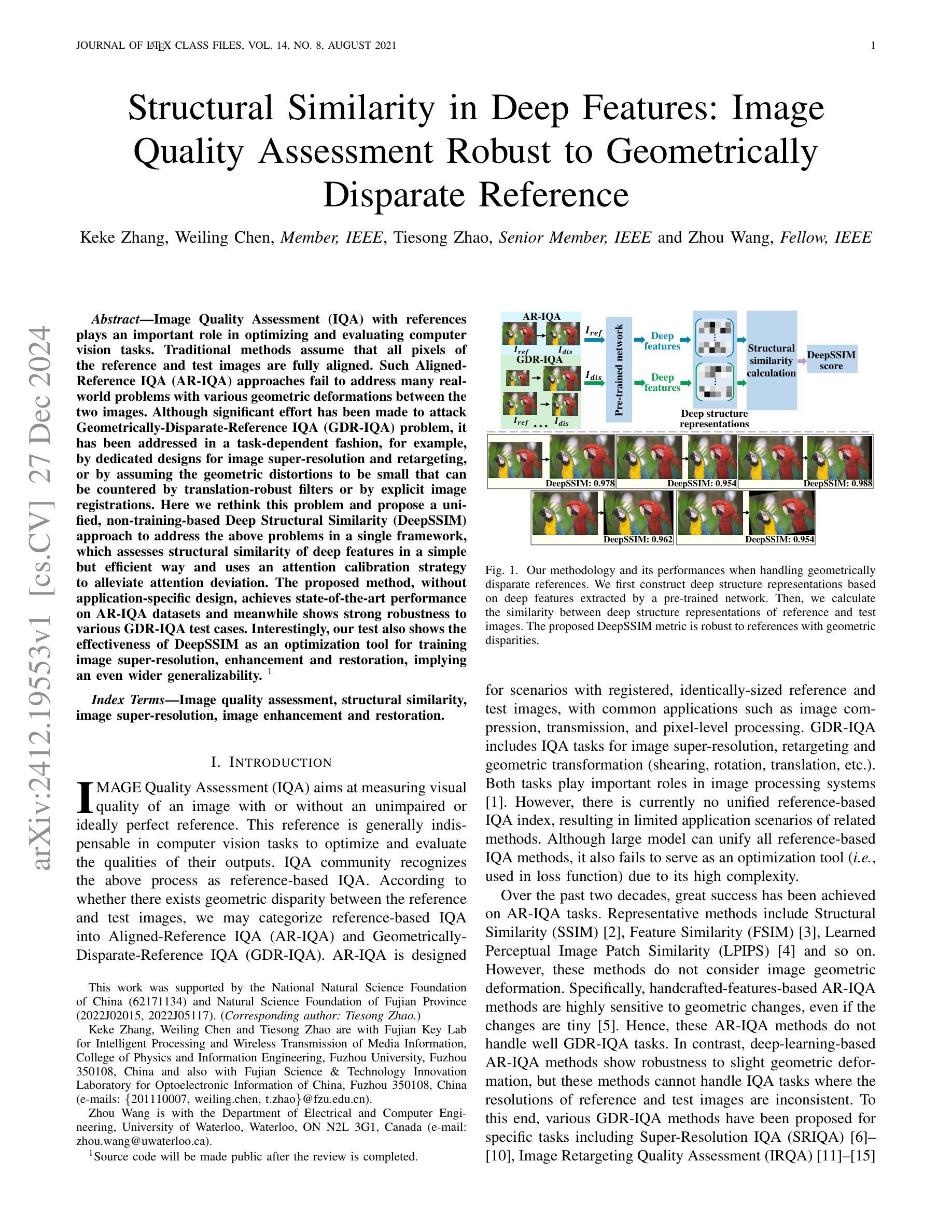
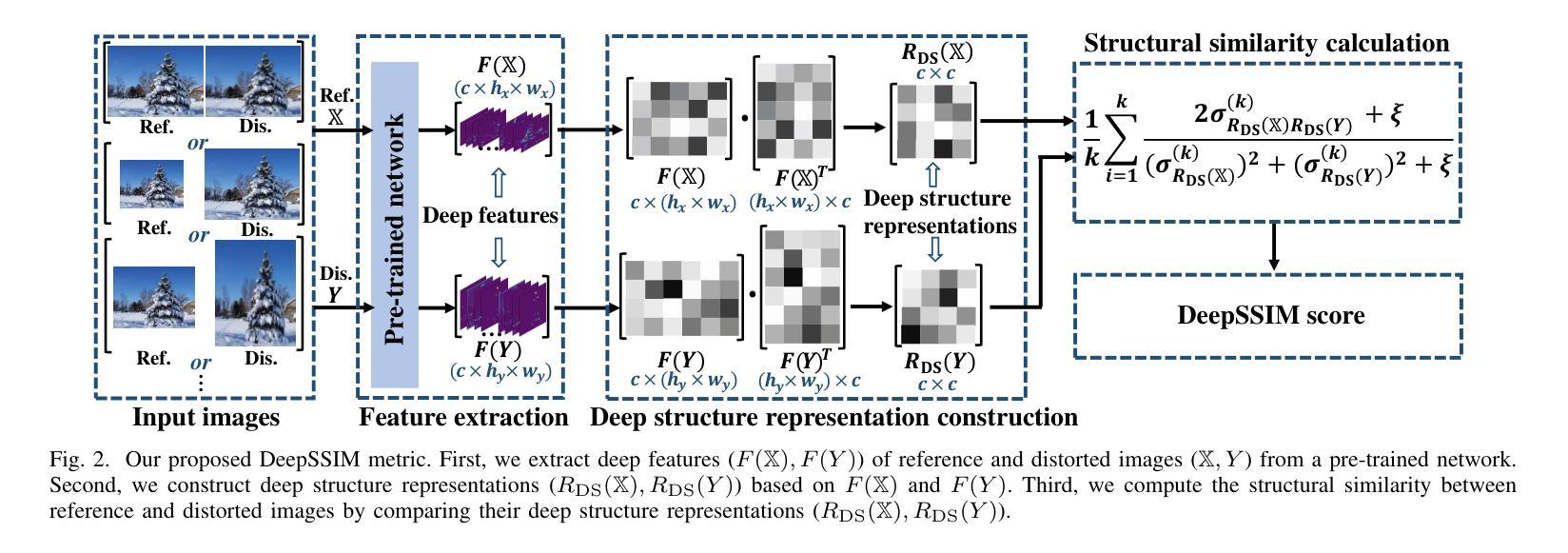
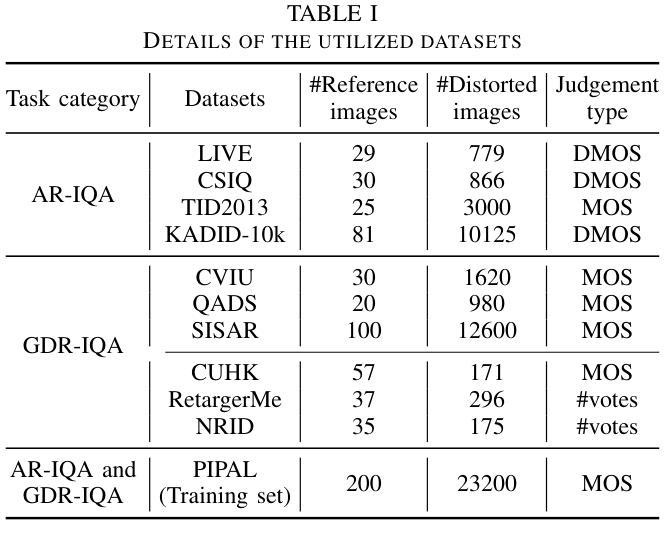
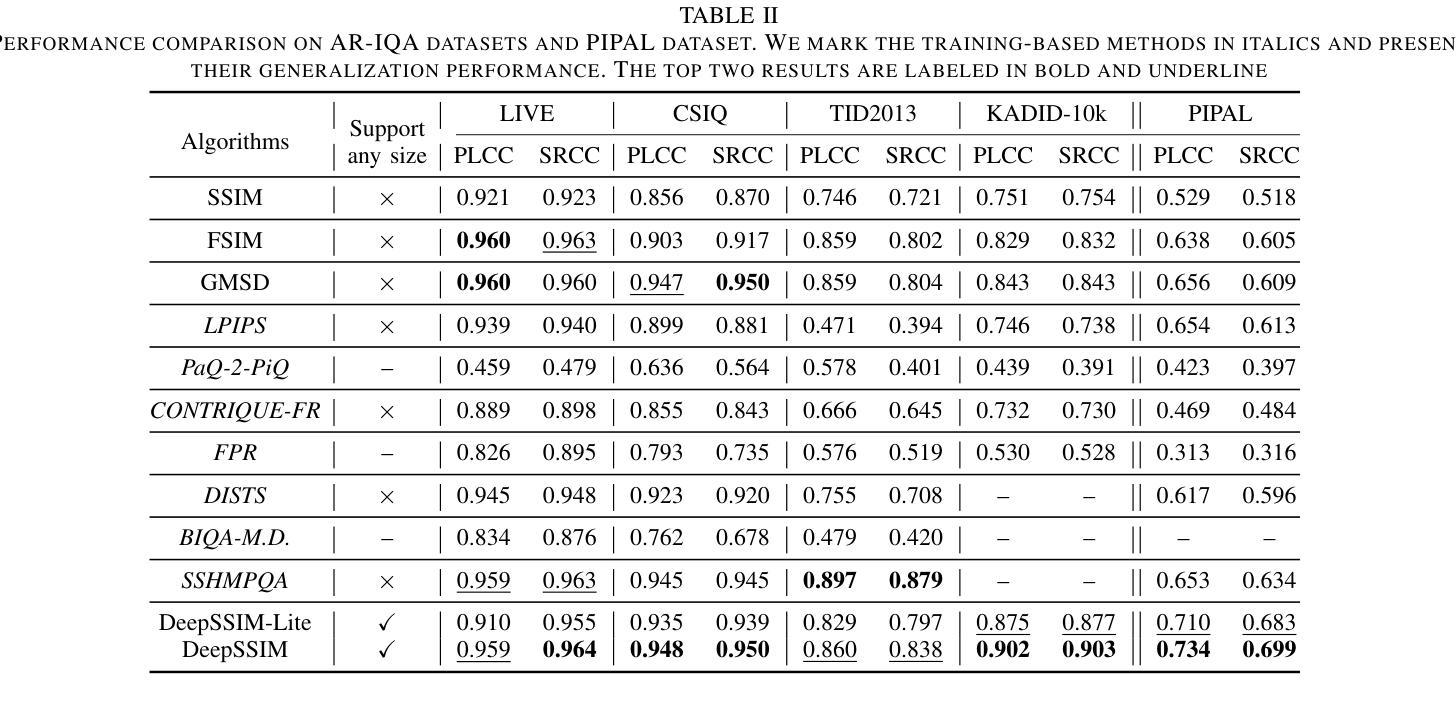
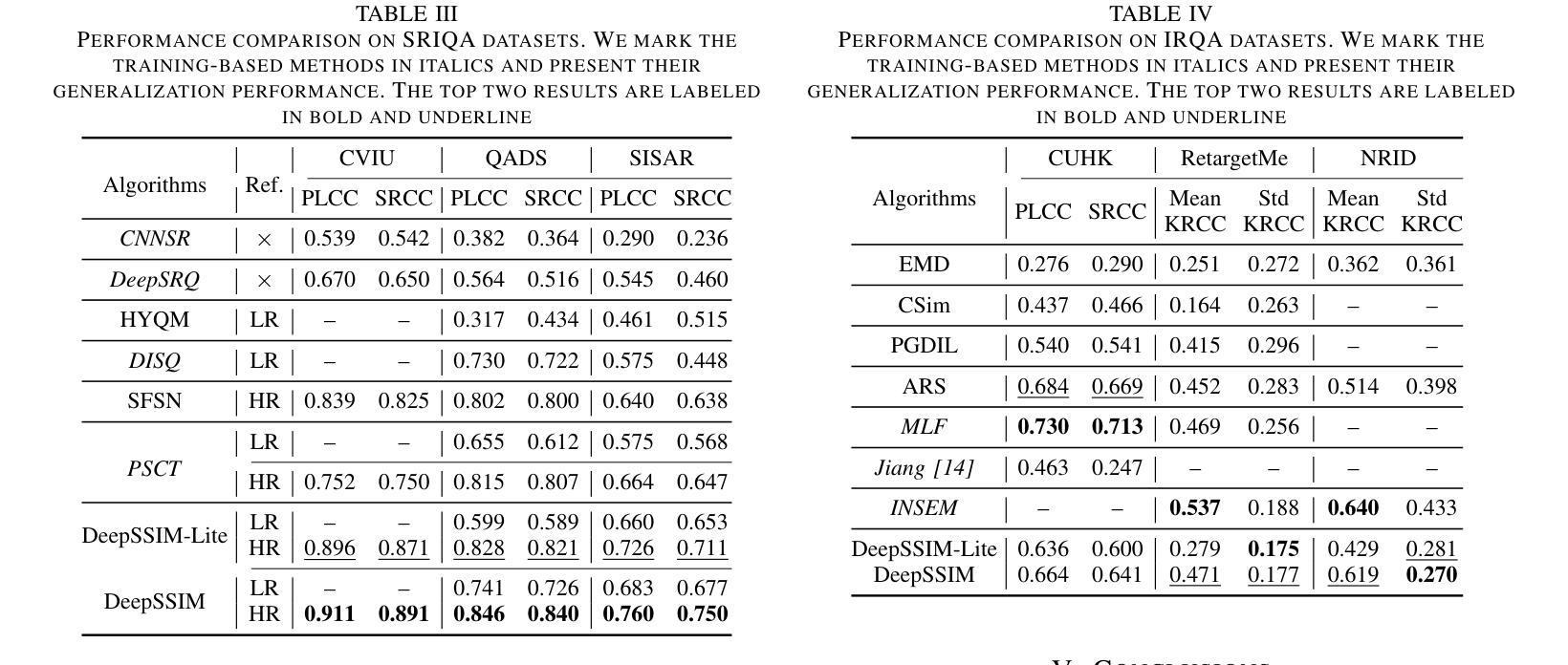
NijiGAN: Transform What You See into Anime with Contrastive Semi-Supervised Learning and Neural Ordinary Differential Equations
Authors:Kevin Putra Santoso, Anny Yuniarti, Dwiyasa Nakula, Dimas Prihady Setyawan, Adam Haidar Azizi, Jeany Aurellia P. Dewati, Farah Dhia Fadhila, Maria T. Elvara Bumbungan
Generative AI has transformed the animation industry. Several models have been developed for image-to-image translation, particularly focusing on converting real-world images into anime through unpaired translation. Scenimefy, a notable approach utilizing contrastive learning, achieves high fidelity anime scene translation by addressing limited paired data through semi-supervised training. However, it faces limitations due to its reliance on paired data from a fine-tuned StyleGAN in the anime domain, often producing low-quality datasets. Additionally, Scenimefy’s high parameter architecture presents opportunities for computational optimization. This research introduces NijiGAN, a novel model incorporating Neural Ordinary Differential Equations (NeuralODEs), which offer unique advantages in continuous transformation modeling compared to traditional residual networks. NijiGAN successfully transforms real-world scenes into high fidelity anime visuals using half of Scenimefy’s parameters. It employs pseudo-paired data generated through Scenimefy for supervised training, eliminating dependence on low-quality paired data and improving the training process. Our comprehensive evaluation includes ablation studies, qualitative, and quantitative analysis comparing NijiGAN to similar models. The testing results demonstrate that NijiGAN produces higher-quality images compared to AnimeGAN, as evidenced by a Mean Opinion Score (MOS) of 2.192, it surpasses AnimeGAN’s MOS of 2.160. Furthermore, our model achieved a Frechet Inception Distance (FID) score of 58.71, outperforming Scenimefy’s FID score of 60.32. These results demonstrate that NijiGAN achieves competitive performance against existing state-of-the-arts, especially Scenimefy as the baseline model.
生成式人工智能已经彻底改变了动画产业。已经开发了几种图像到图像的翻译模型,特别专注于通过非配对翻译将现实世界图像转化为动漫。Scenimefy是一种利用对比学习的方法,通过半监督训练解决配对数据有限的问题,实现了高保真动漫场景翻译。然而,它依赖于精细调整的StyleGAN在动漫领域的配对数据,因此存在局限性,常产生低质量数据集。此外,Scenimefy的高参数架构还存在计算优化的机会。本研究引入了NijiGAN,这是一个结合神经常微分方程(NeuralODEs)的新模型,与传统的残差网络相比,它在连续变换建模方面具有独特优势。NijiGAN成功地将现实场景转化为高保真动漫视觉,使用的是Scenimefy一半的参数。它采用通过Scenimefy生成伪配对数据进行监督训练,消除了对低质量配对数据的依赖,改进了训练过程。我们的综合评估包括消融研究、定性和定量分析,比较了NijiGAN与类似模型的表现。测试结果表明,NijiGAN产生的图像质量高于AnimeGAN,平均意见得分(MOS)为2.192,超过了AnimeGAN的MOS得分2.160。此外,我们的模型达到了Frechet Inception Distance(FID)得分58.71,超越了Scenimefy的FID得分60.32。这些结果表明,NijiGAN在现有先进技术中表现出竞争力,尤其是作为基线模型的Scenimefy。
论文及项目相关链接
Summary
生成式人工智能已经改变了动画产业。新的模型如Scenimefy和NijiGAN的发展,推动了图像到图像的转换技术,特别是在将现实世界图像转化为动漫图像方面取得了显著进展。Scenimefy通过对比学习实现高质量动漫场景翻译,但它依赖精细调整的StyleGAN动漫领域的配对数据,存在质量不稳定的问题。NijiGAN则采用神经网络常微分方程进行连续变换建模,以较少的参数成功转化真实场景为高质量动漫图像,并摆脱了对低质量配对数据的依赖。测试结果显示,NijiGAN在图像质量和性能上超过了现有的先进技术,特别是相对于基线模型Scenimefy有更好的表现。
**Key Takeaways**
1. 生成式人工智能对动画产业产生了重大影响。
2. Scenimefy利用对比学习实现高质量动漫场景翻译,但依赖配对数据存在质量问题。
3. NijiGAN采用神经网络常微分方程建模,参数更少且转化效果优异。
4. NijiGAN摆脱了对低质量配对数据的依赖,通过伪配对数据进行监督训练。
5. NijiGAN图像质量高于AnimeGAN,Mean Opinion Score(MOS)为2.192。
6. NijiGAN的Frechet Inception Distance(FID)得分为58.71,优于Scenimefy。
点此查看论文截图
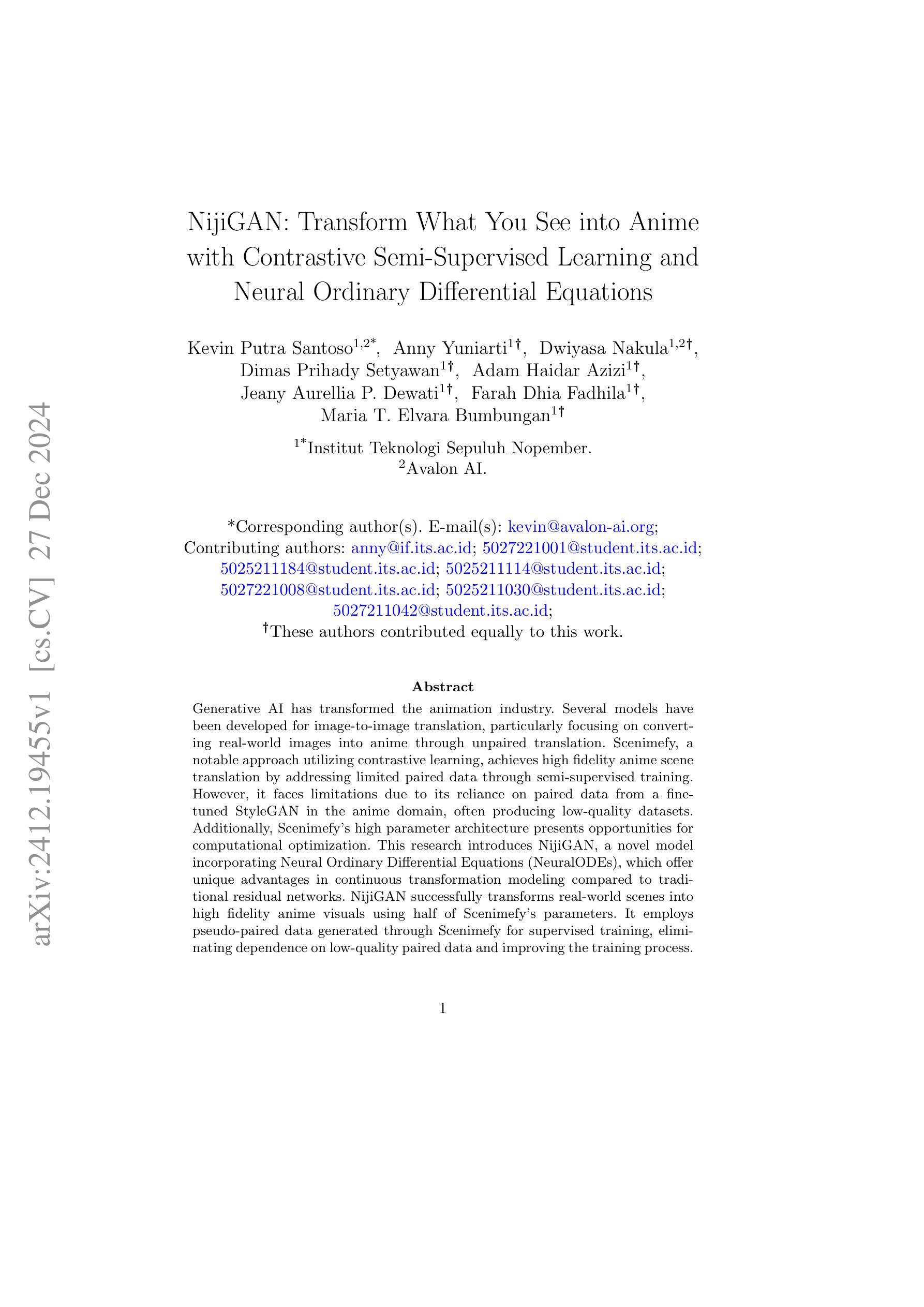
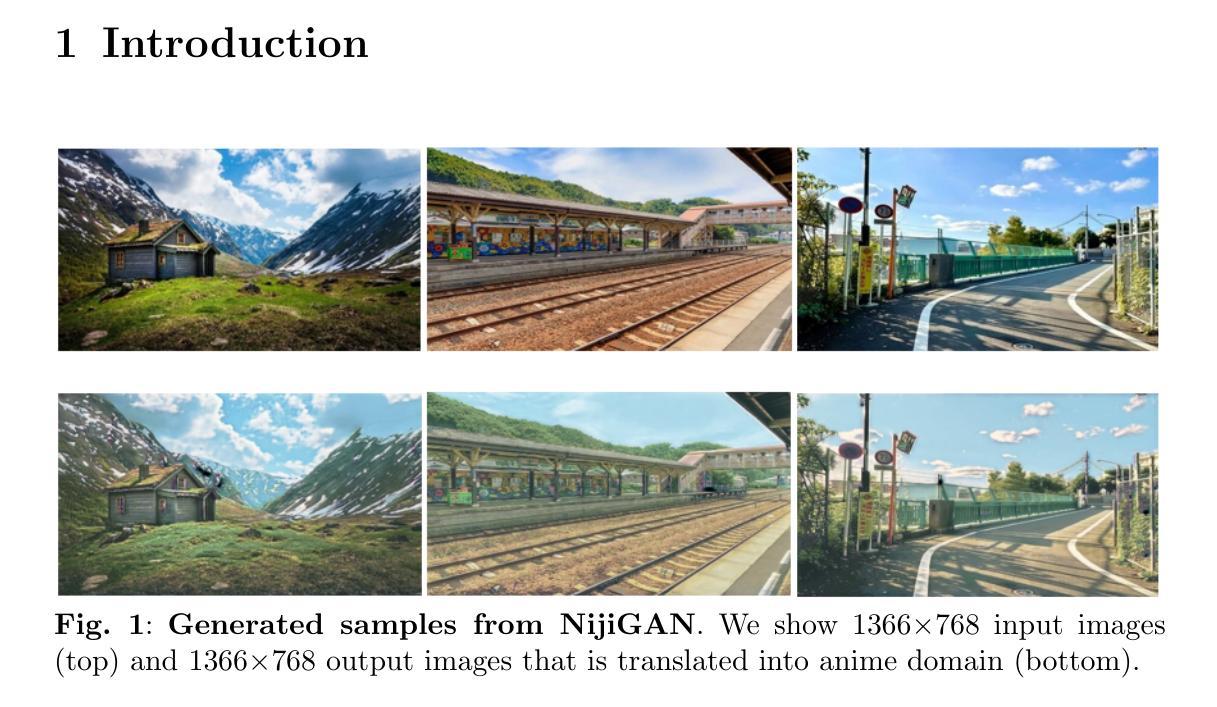
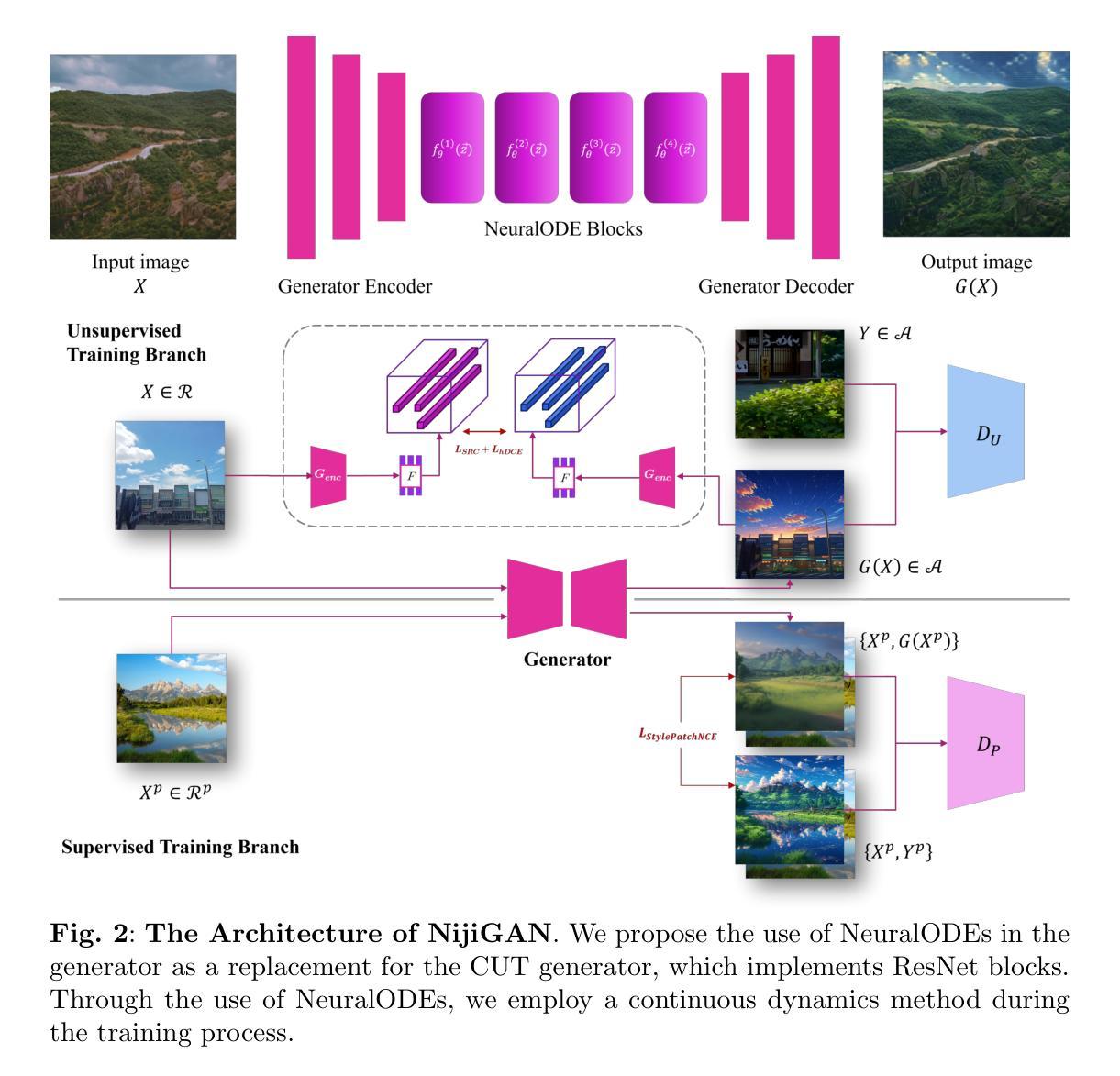
VoiceDiT: Dual-Condition Diffusion Transformer for Environment-Aware Speech Synthesis
Authors:Jaemin Jung, Junseok Ahn, Chaeyoung Jung, Tan Dat Nguyen, Youngjoon Jang, Joon Son Chung
We present VoiceDiT, a multi-modal generative model for producing environment-aware speech and audio from text and visual prompts. While aligning speech with text is crucial for intelligible speech, achieving this alignment in noisy conditions remains a significant and underexplored challenge in the field. To address this, we present a novel audio generation pipeline named VoiceDiT. This pipeline includes three key components: (1) the creation of a large-scale synthetic speech dataset for pre-training and a refined real-world speech dataset for fine-tuning, (2) the Dual-DiT, a model designed to efficiently preserve aligned speech information while accurately reflecting environmental conditions, and (3) a diffusion-based Image-to-Audio Translator that allows the model to bridge the gap between audio and image, facilitating the generation of environmental sound that aligns with the multi-modal prompts. Extensive experimental results demonstrate that VoiceDiT outperforms previous models on real-world datasets, showcasing significant improvements in both audio quality and modality integration.
我们提出了VoiceDiT,这是一种多模态生成模型,可以从文本和视觉提示生成环境感知的语音和音频。虽然将语音与文本对齐对于可理解的语音至关重要,但在嘈杂的条件下实现这种对齐仍然是该领域一个重大且尚未被充分研究的挑战。为了解决这一问题,我们提出了一种名为VoiceDiT的新型音频生成管道。该管道包括三个关键组件:(1)创建用于预训练的大规模合成语音数据集和用于精细调整的真实世界语音数据集;(2)Dual-DiT模型,该模型旨在高效保留对齐的语音信息,同时准确反映环境状况;(3)基于扩散的图像到音频翻译器,使模型能够在音频和图像之间建立桥梁,便于生成与环境声音相匹配的多媒体提示。大量的实验结果表明,在真实世界的数据集上,VoiceDiT优于之前的模型,在音频质量和模态集成方面都取得了显著的改进。
论文及项目相关链接
PDF Accepted to ICASSP 2025
Summary
本文介绍了VoiceDiT,一种多模态生成模型,可以从文本和视觉提示产生环境感知的语音和音频。该模型针对噪声环境下的语音与文本对齐问题,提出了名为VoiceDiT的新型音频生成流程。该流程包括三个关键组件:大规模合成语音数据集用于预训练和精细调整的真实世界语音数据集、用于高效保存对齐语音信息的双DiT模型,以及基于扩散的图像到音频翻译器,可缩短音频和图像之间的差距,促进与环境声音的多模态提示对齐的生成。实验结果表明,VoiceDiT在真实世界数据集上的表现优于先前模型,在音频质量和模态集成方面都有显著提高。
Key Takeaways
- VoiceDiT是一种多模态生成模型,可以从文本和视觉提示产生环境感知的语音和音频。
- 针对噪声环境下的语音与文本对齐问题,提出了名为VoiceDiT的音频生成流程。
- VoiceDiT包括三个关键组件:大规模合成语音数据集、双DiT模型和基于扩散的图像到音频翻译器。
- 双DiT模型能够高效保存对齐语音信息,并准确反映环境状况。
- 图像到音频翻译器能够缩短音频和图像之间的差距,促进环境声音的多模态生成。
- VoiceDiT在真实世界数据集上的表现优于先前模型。
点此查看论文截图
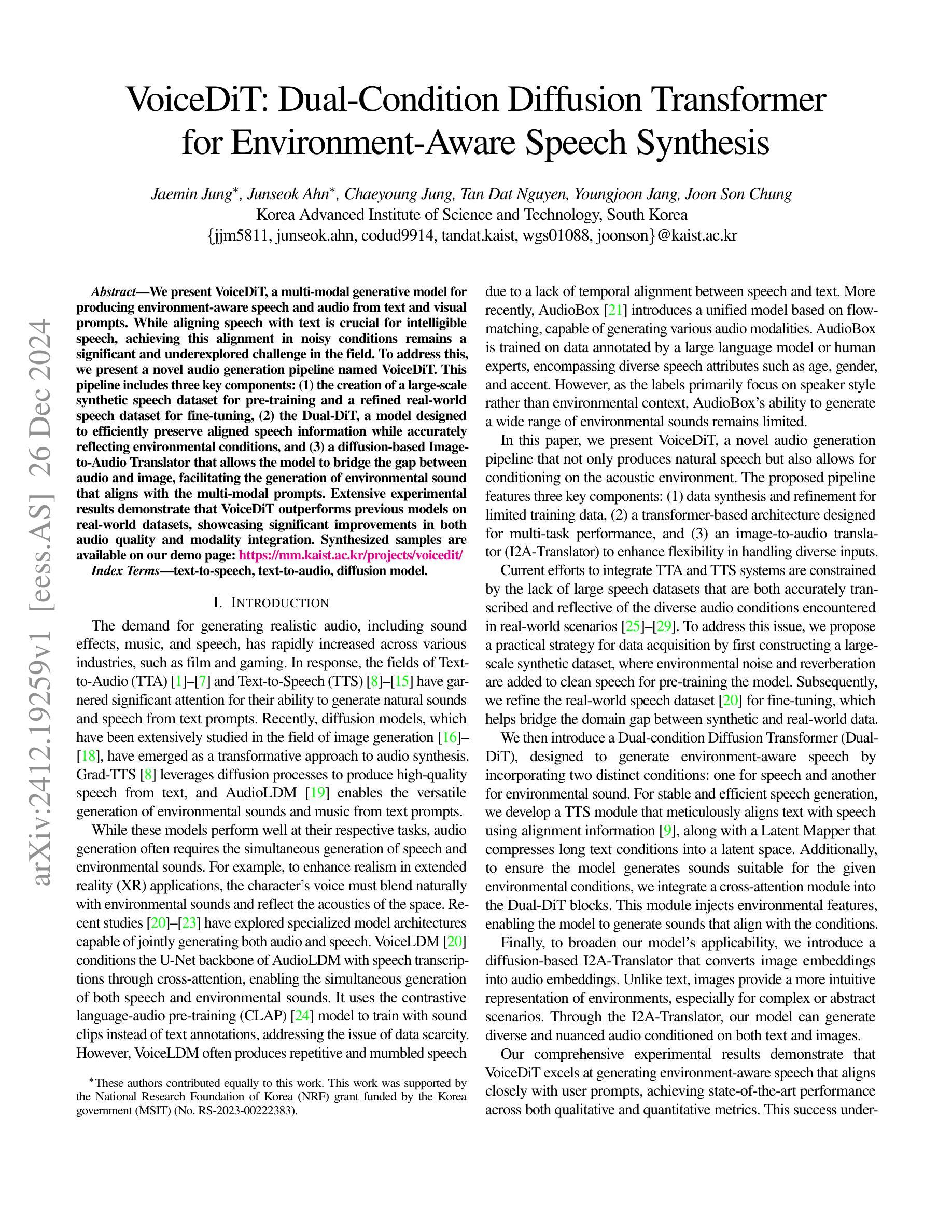
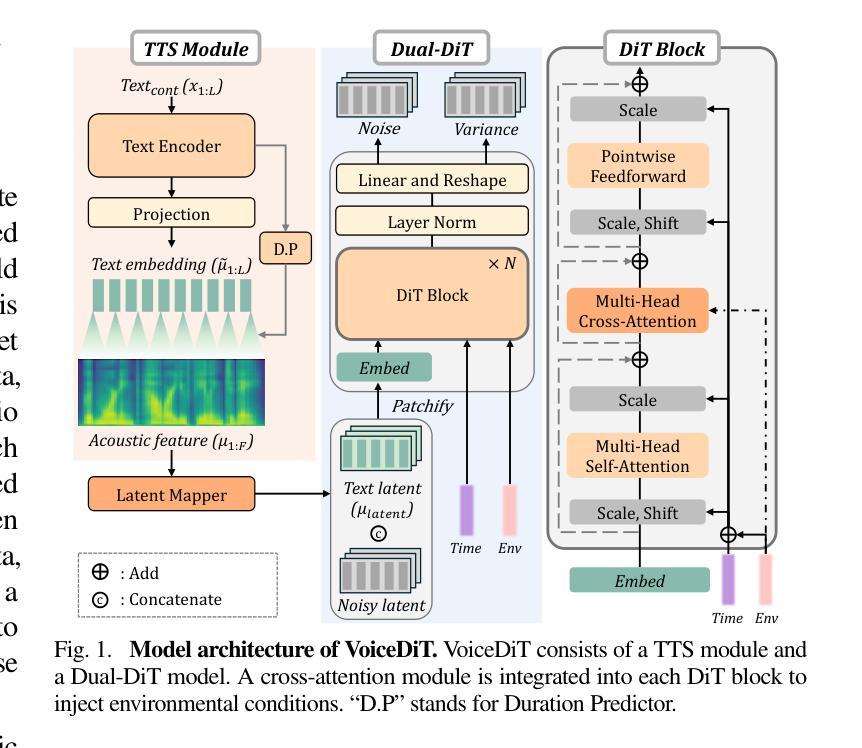
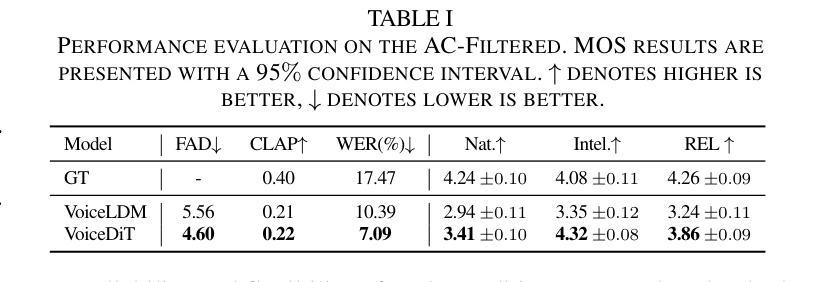
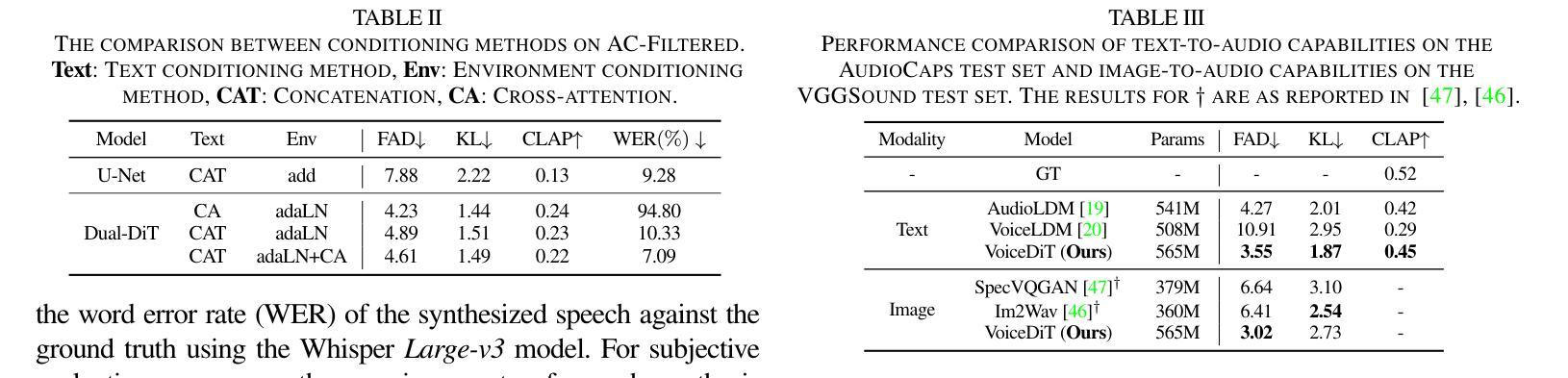