⚠️ 以下所有内容总结都来自于 大语言模型的能力,如有错误,仅供参考,谨慎使用
🔴 请注意:千万不要用于严肃的学术场景,只能用于论文阅读前的初筛!
💗 如果您觉得我们的项目对您有帮助 ChatPaperFree ,还请您给我们一些鼓励!⭐️ HuggingFace免费体验
2025-01-04 更新
Prometheus: 3D-Aware Latent Diffusion Models for Feed-Forward Text-to-3D Scene Generation
Authors:Yuanbo Yang, Jiahao Shao, Xinyang Li, Yujun Shen, Andreas Geiger, Yiyi Liao
In this work, we introduce Prometheus, a 3D-aware latent diffusion model for text-to-3D generation at both object and scene levels in seconds. We formulate 3D scene generation as multi-view, feed-forward, pixel-aligned 3D Gaussian generation within the latent diffusion paradigm. To ensure generalizability, we build our model upon pre-trained text-to-image generation model with only minimal adjustments, and further train it using a large number of images from both single-view and multi-view datasets. Furthermore, we introduce an RGB-D latent space into 3D Gaussian generation to disentangle appearance and geometry information, enabling efficient feed-forward generation of 3D Gaussians with better fidelity and geometry. Extensive experimental results demonstrate the effectiveness of our method in both feed-forward 3D Gaussian reconstruction and text-to-3D generation. Project page: https://freemty.github.io/project-prometheus/
在这项工作中,我们介绍了Prometheus,这是一个3D感知潜在扩散模型,可在对象和场景级别实现文本到3D的即时生成。我们将3D场景生成公式化为潜在扩散范式内的多视角、前馈、像素对齐的3D高斯生成。为了确保模型的通用性,我们在预训练的文本到图像生成模型的基础上进行构建,只需进行微小的调整,并使用大量来自单视角和多视角数据集的图片进行进一步训练。此外,我们将RGB-D潜在空间引入3D高斯生成中,以分离外观和几何信息,实现高效的前馈3D高斯生成,具有更高的保真度和几何形状。大量的实验结果证明了我们的方法在前馈3D高斯重建和文本到3D生成中的有效性。项目页面:https://freemty.github.io/project-prometheus/
论文及项目相关链接
Summary
本工作介绍了Prometheus,一个用于文本到三维物体与场景生成的3D感知潜在扩散模型。其将三维场景生成公式化为多视角、前馈、像素对齐的潜在扩散模型内的三维高斯生成。模型基于预训练的文本到图像生成模型构建,仅作微小调整,并使用大量单视角和多视角图像数据集进行训练。引入RGB-D潜在空间到三维高斯生成中,以分离外观和几何信息,实现高效的前馈三维高斯生成,提高保真度和几何性能。
Key Takeaways
- Prometheus是一个用于文本到三维物体和场景生成的3D感知潜在扩散模型。
- 该模型将三维场景生成看作是多视角、前馈、像素对齐的三维高斯生成问题。
- 模型基于预训练的文本到图像生成模型构建,具有良好的泛化能力。
- 通过引入RGB-D潜在空间,模型能够更有效地分离并处理外观和几何信息。
- 模型使用大量单视角和多视角图像数据集进行训练,提高了生成的三维模型的保真度和几何性能。
- 实验结果表明,该模型在三维高斯重建和文本到三维生成方面均表现出良好的效果。
点此查看论文截图
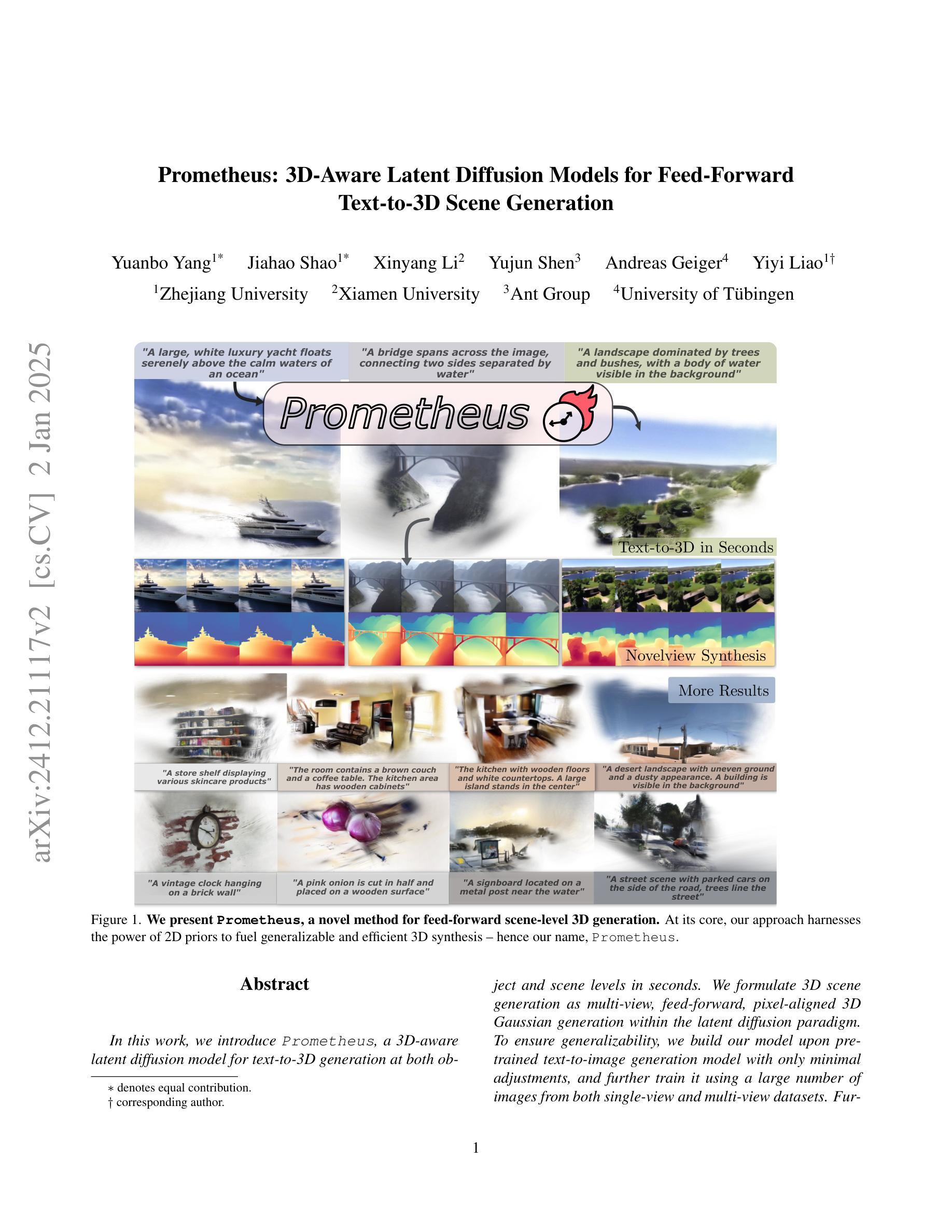
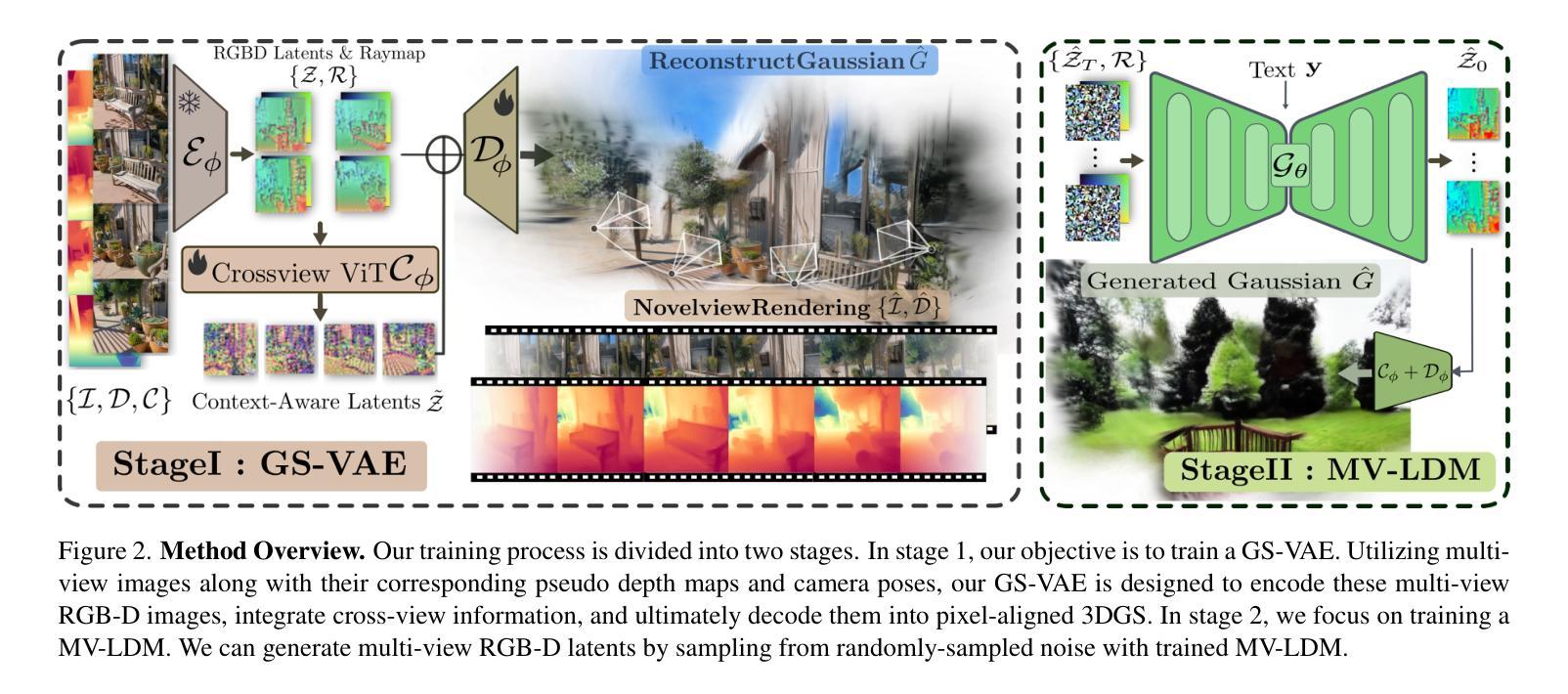
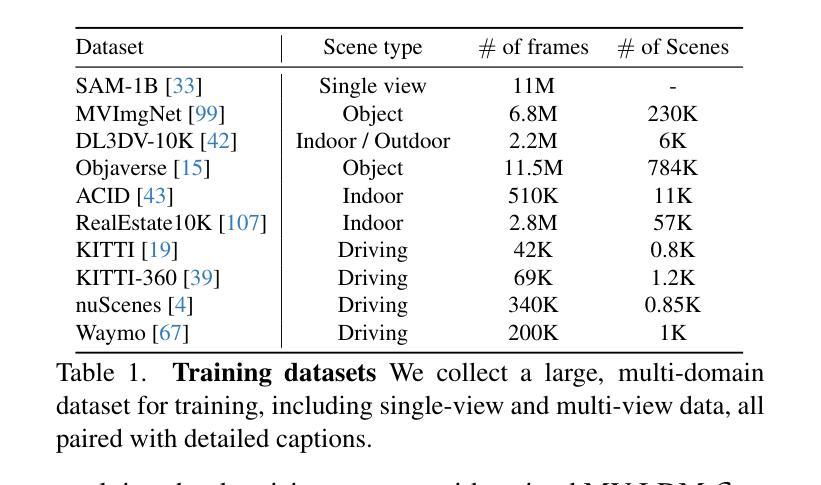
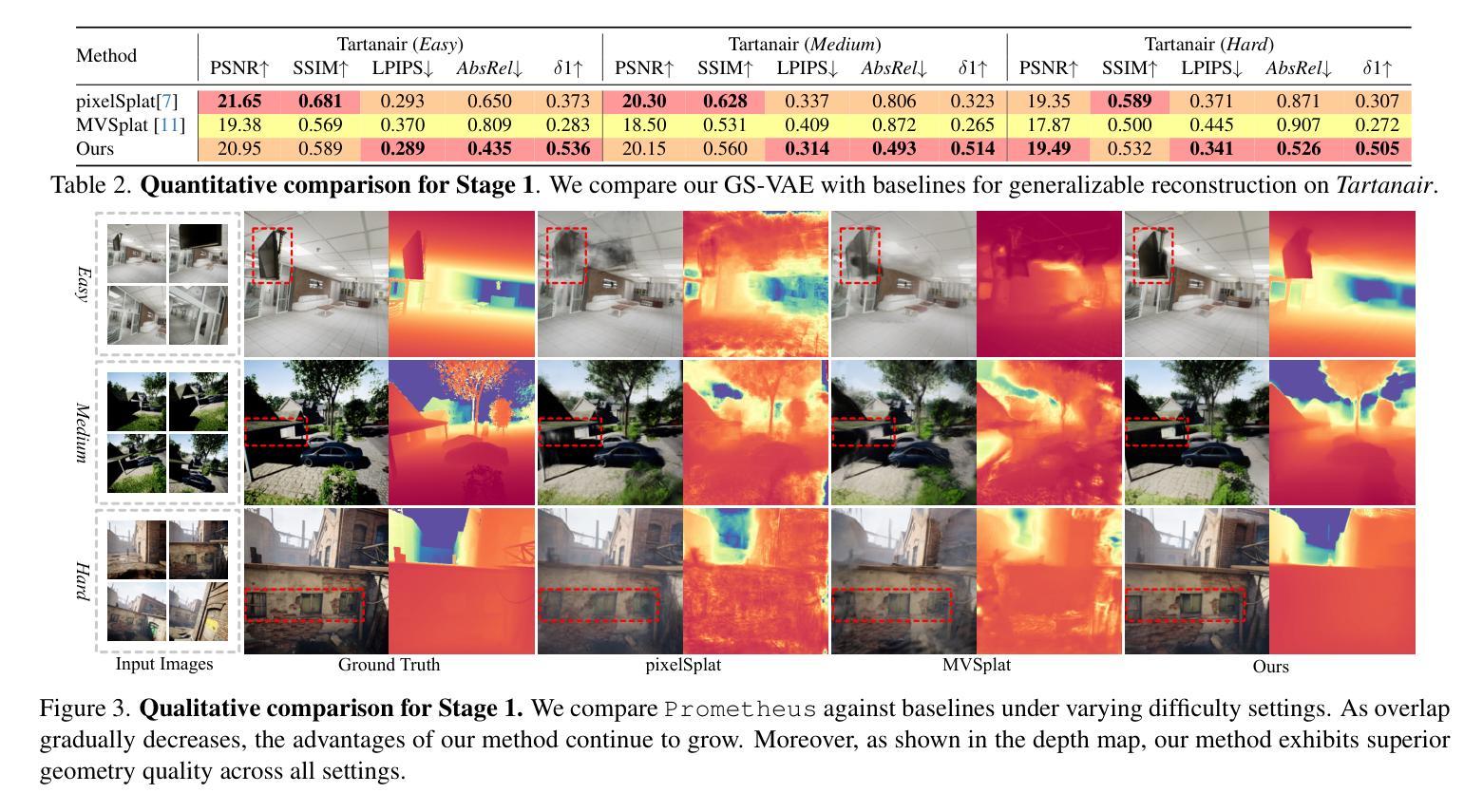
AugGS: Self-augmented Gaussians with Structural Masks for Sparse-view 3D Reconstruction
Authors:Bi’an Du, Lingbei Meng, Wei Hu
Sparse-view 3D reconstruction is a major challenge in computer vision, aiming to create complete three-dimensional models from limited viewing angles. Key obstacles include: 1) a small number of input images with inconsistent information; 2) dependence on input image quality; and 3) large model parameter sizes. To tackle these issues, we propose a self-augmented two-stage Gaussian splatting framework enhanced with structural masks for sparse-view 3D reconstruction. Initially, our method generates a basic 3D Gaussian representation from sparse inputs and renders multi-view images. We then fine-tune a pre-trained 2D diffusion model to enhance these images, using them as augmented data to further optimize the 3D Gaussians. Additionally, a structural masking strategy during training enhances the model’s robustness to sparse inputs and noise. Experiments on benchmarks like MipNeRF360, OmniObject3D, and OpenIllumination demonstrate that our approach achieves state-of-the-art performance in perceptual quality and multi-view consistency with sparse inputs.
稀疏视角下的三维重建是计算机视觉领域的一大挑战,其目标是从有限的视角创建完整的三维模型。主要障碍包括:1)输入图像数量少且信息不一致;2)依赖于输入图像的质量;以及3)模型参数尺寸大。为了解决这些问题,我们提出了一种增强型两阶段高斯飞溅框架,结合结构掩膜用于稀疏视角下的三维重建。首先,我们的方法从稀疏输入生成基本的三维高斯表示,并呈现多视角图像。然后,我们微调预训练的二维扩散模型以增强这些图像,将其作为增强数据进一步优化三维高斯。此外,在训练过程中采用结构掩膜策略,增强了模型对稀疏输入和噪声的鲁棒性。在MipNeRF360、OmniObject3D和OpenIllumination等基准测试上的实验表明,我们的方法在感知质量和多视角一致性方面达到了最先进的性能,且适用于稀疏输入。
论文及项目相关链接
Summary
本文提出一种基于自增强两阶段高斯贴图框架的稀疏视角3D重建方法,通过结构掩膜增强技术应对关键挑战。首先,该方法从稀疏输入生成基本3D高斯表示并渲染多视角图像。接着,利用预训练的2D扩散模型进行微调以优化图像,并使用这些增强图像进一步优化3D高斯表示。此外,训练过程中的结构掩蔽策略提高了模型对稀疏输入和噪声的鲁棒性。实验结果表明,该方法在MipNeRF360、OmniObject3D和OpenIllumination等基准测试上实现了最先进的性能。
Key Takeaways
- 本文主要介绍了针对稀疏视角3D重建的挑战及现有问题。
- 提出了一种基于自增强两阶段高斯贴图框架的解决方法,涉及基本3D高斯表示的生成和多视角图像的渲染。
- 利用预训练的2D扩散模型进行微调以增强图像质量,进一步优化3D高斯表示。
- 引入了结构掩膜增强技术,提高了模型对稀疏输入和噪声的鲁棒性。
- 在多个基准测试上实现了先进的性能,包括MipNeRF360、OmniObject3D和OpenIllumination等数据集。
点此查看论文截图
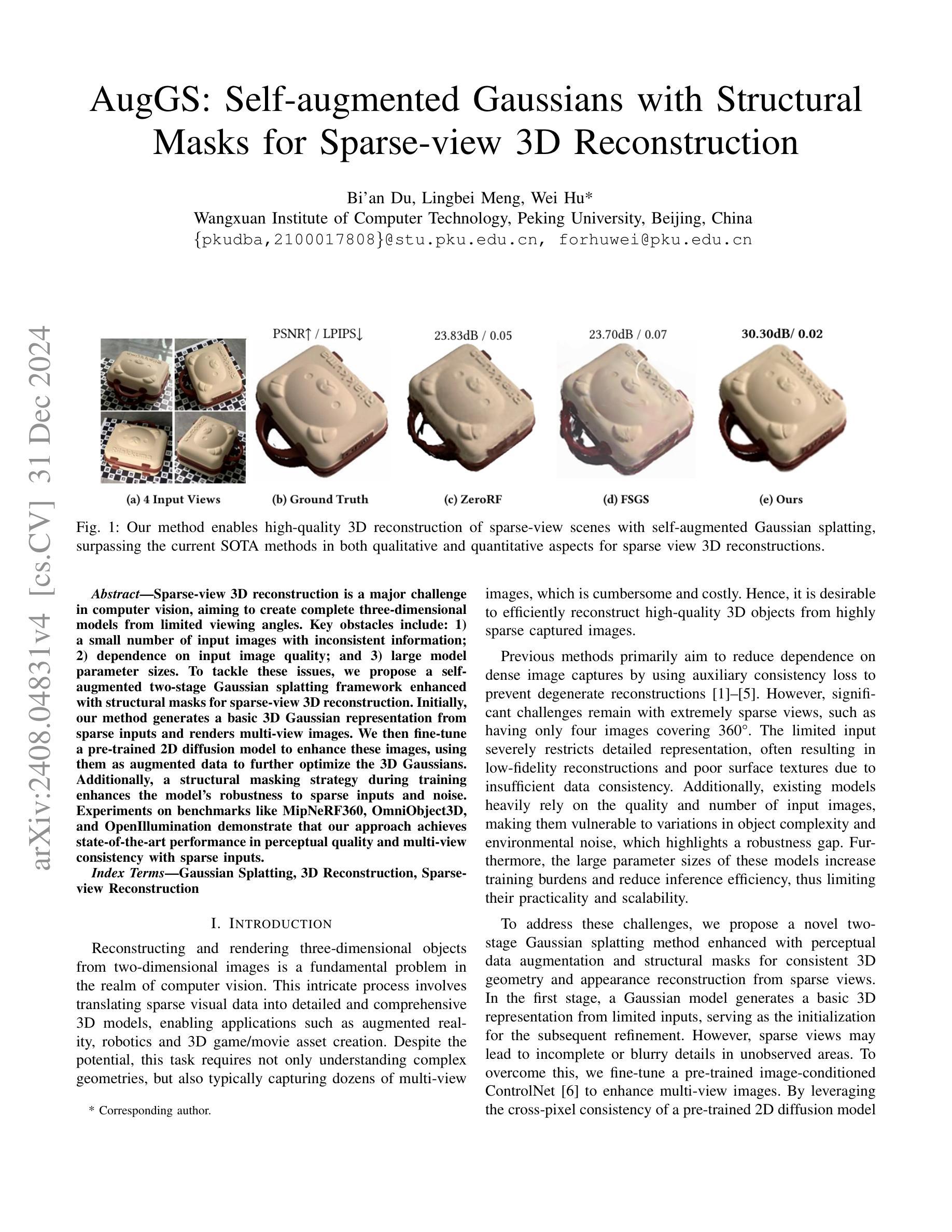
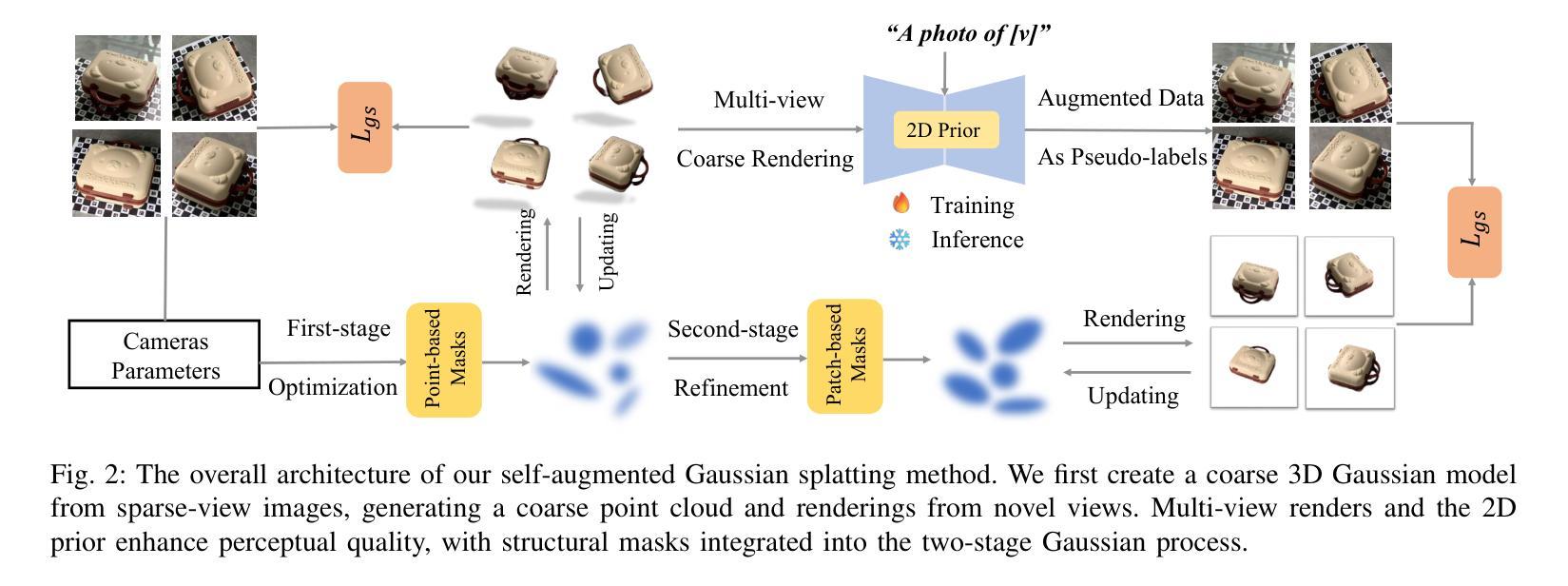
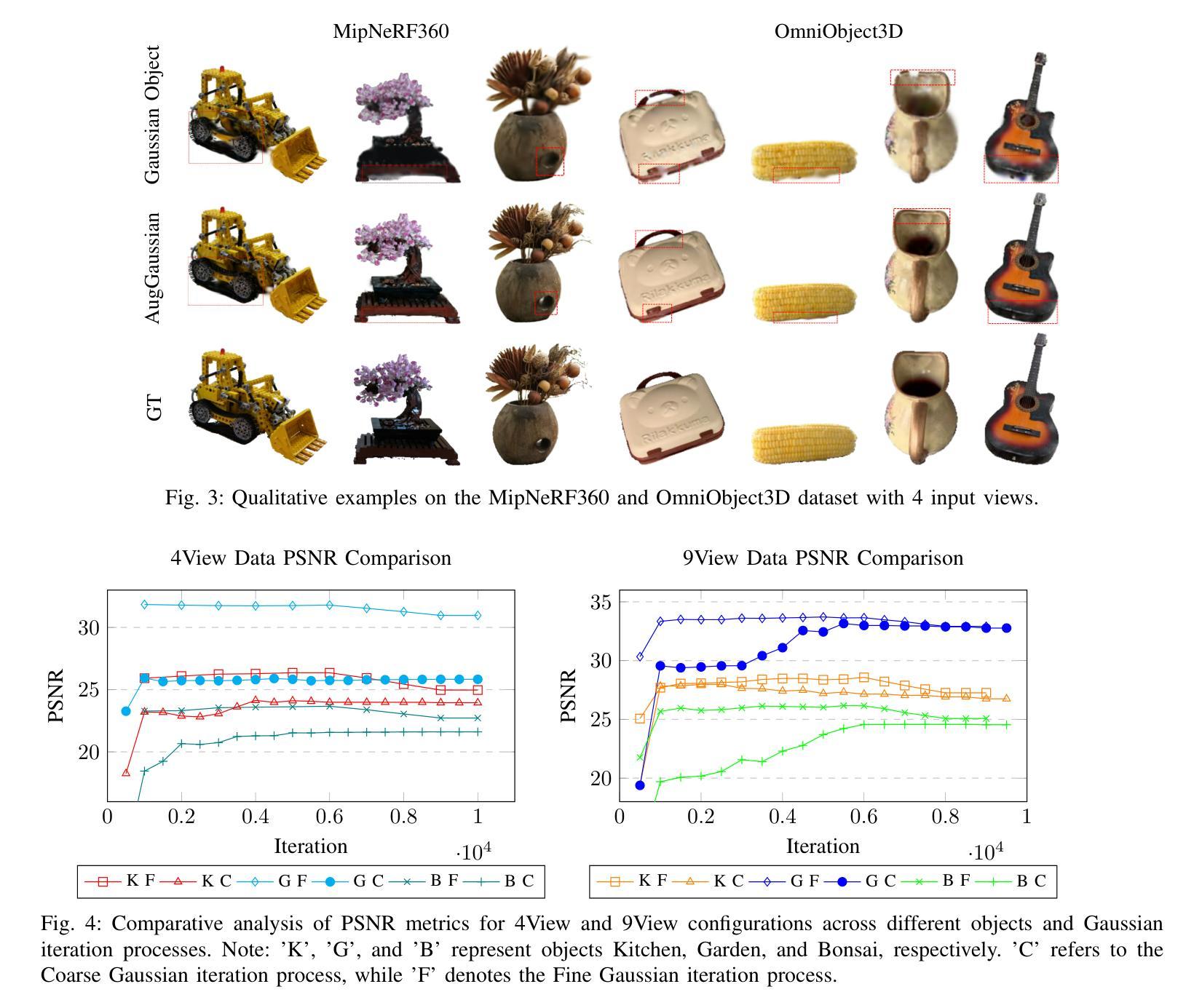
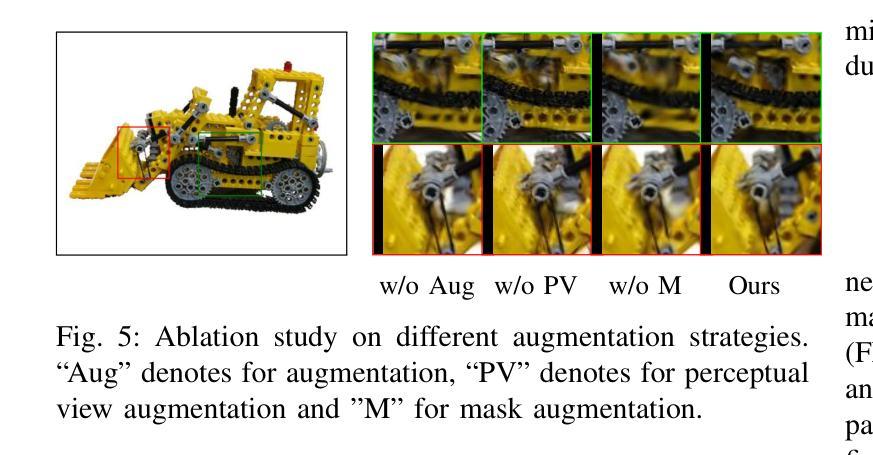
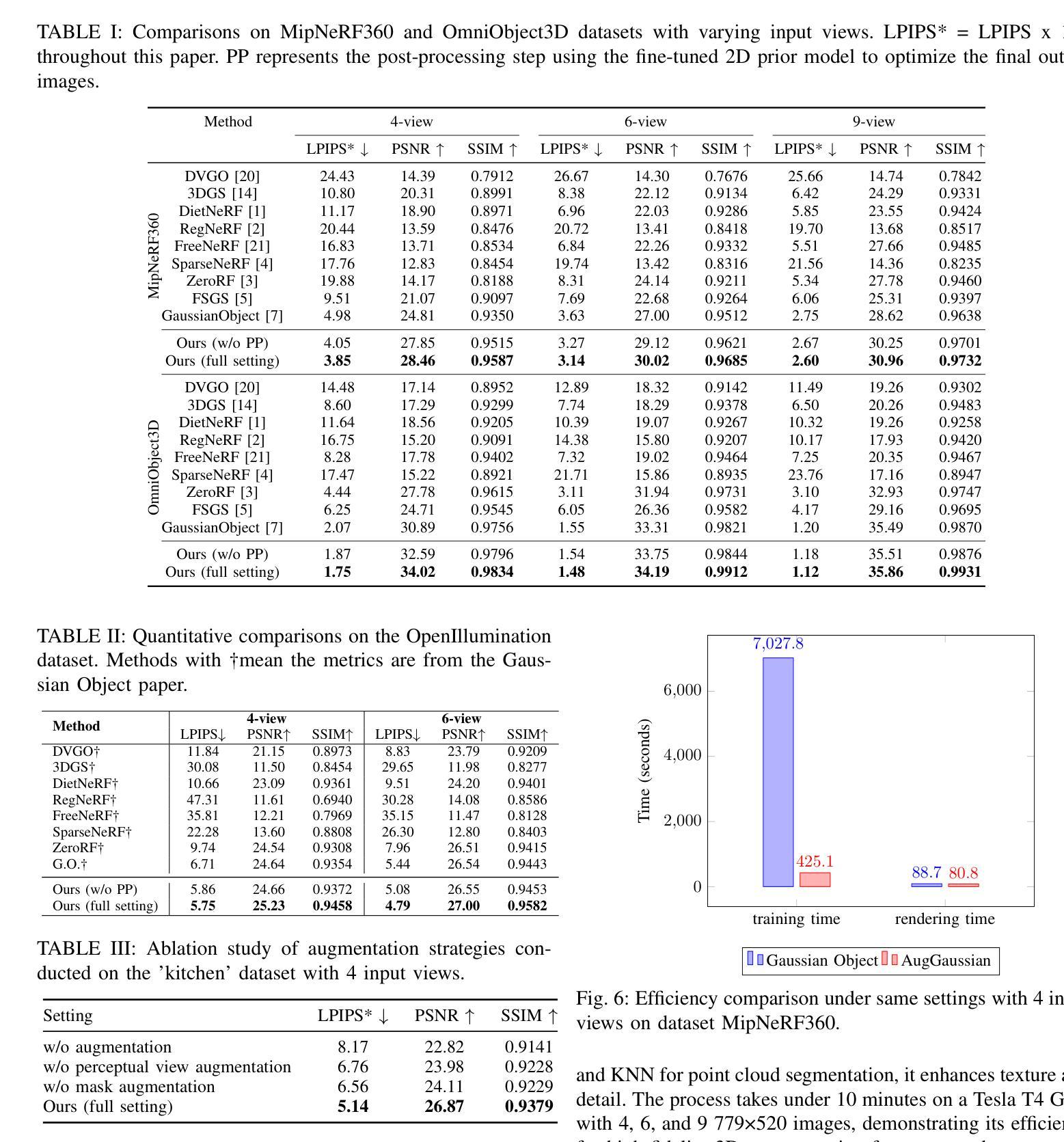
GFlow: Recovering 4D World from Monocular Video
Authors:Shizun Wang, Xingyi Yang, Qiuhong Shen, Zhenxiang Jiang, Xinchao Wang
Recovering 4D world from monocular video is a crucial yet challenging task. Conventional methods usually rely on the assumptions of multi-view videos, known camera parameters, or static scenes. In this paper, we relax all these constraints and tackle a highly ambitious but practical task: With only one monocular video without camera parameters, we aim to recover the dynamic 3D world alongside the camera poses. To solve this, we introduce GFlow, a new framework that utilizes only 2D priors (depth and optical flow) to lift a video to a 4D scene, as a flow of 3D Gaussians through space and time. GFlow starts by segmenting the video into still and moving parts, then alternates between optimizing camera poses and the dynamics of the 3D Gaussian points. This method ensures consistency among adjacent points and smooth transitions between frames. Since dynamic scenes always continually introduce new visual content, we present prior-driven initialization and pixel-wise densification strategy for Gaussian points to integrate new content. By combining all those techniques, GFlow transcends the boundaries of 4D recovery from causal videos; it naturally enables tracking of points and segmentation of moving objects across frames. Additionally, GFlow estimates the camera poses for each frame, enabling novel view synthesis by changing camera pose. This capability facilitates extensive scene-level or object-level editing, highlighting GFlow’s versatility and effectiveness. Visit our project page at: https://littlepure2333.github.io/GFlow
从单目视频中恢复四维世界是一项至关重要且具有挑战性的任务。传统方法通常依赖于多视角视频的假设、已知的相机参数或静态场景。在本文中,我们放宽了所有这些约束,并解决了一项雄心勃勃但实用的任务:仅使用没有相机参数的单个单目视频,我们旨在恢复动态的3D世界以及相机姿态。为解决此问题,我们引入了GFlow,这是一个新的框架,它仅利用2D先验(深度和光流)将视频提升到4D场景,作为时空中的3D高斯流。GFlow首先将视频分割成静态和动态部分,然后在优化相机姿态和3D高斯点的动力学之间交替进行。这种方法确保了相邻点之间的一致性以及帧之间的平滑过渡。由于动态场景会持续引入新的视觉内容,我们为高斯点呈现先验驱动初始化和像素级细化策略以集成新内容。通过结合所有这些技术,GFlow超越了从因果视频进行4D恢复的界限;它自然地实现了跨帧的点跟踪和移动对象分割。此外,GFlow估计每帧的相机姿态,通过改变相机姿态来实现新型视图合成。这种能力促进了场景级别或对象级别的广泛编辑,突出了GFlow的通用性和有效性。请访问我们的项目页面:https://littlepure2333.github.io/GFlow
论文及项目相关链接
PDF AAAI 2025. Project page: https://littlepure2333.github.io/GFlow
Summary
本文提出了一种新的方法GFlow,能够从单目视频中恢复动态的3D世界并估算相机姿态。该方法利用2D先验知识(深度和光流)将视频提升到4D场景,通过空间中时间的3D高斯流动来实现。GFlow能够处理动态场景,通过优化相机姿态和3D高斯点的动态性,确保相邻点之间的连贯性和帧之间的平滑过渡。该项目还实现了因果视频下的4D恢复突破,能跟踪点和分割移动物体,并估算每帧的相机姿态,为场景或对象级别的编辑提供了便利。
Key Takeaways
- GFlow框架能从单目视频中恢复动态的3D世界,无需多视角视频、已知相机参数或静态场景假设。
- GFlow利用2D先验知识(深度和光流)将视频提升到4D场景,通过空间中时间的3D高斯流动实现。
- GFlow通过优化相机姿态和3D高斯点的动态性,确保处理的连贯性和帧间过渡的平滑性。
- 针对动态场景,GFlow采用先验驱动初始化和像素级加密策略整合新的内容。
- GFlow突破了从因果视频进行4D恢复的界限,能实现跨帧的点跟踪和移动物体分割。
- GFlow能估算每帧的相机姿态,便于新型视图合成,支持场景或对象级别的编辑。
点此查看论文截图
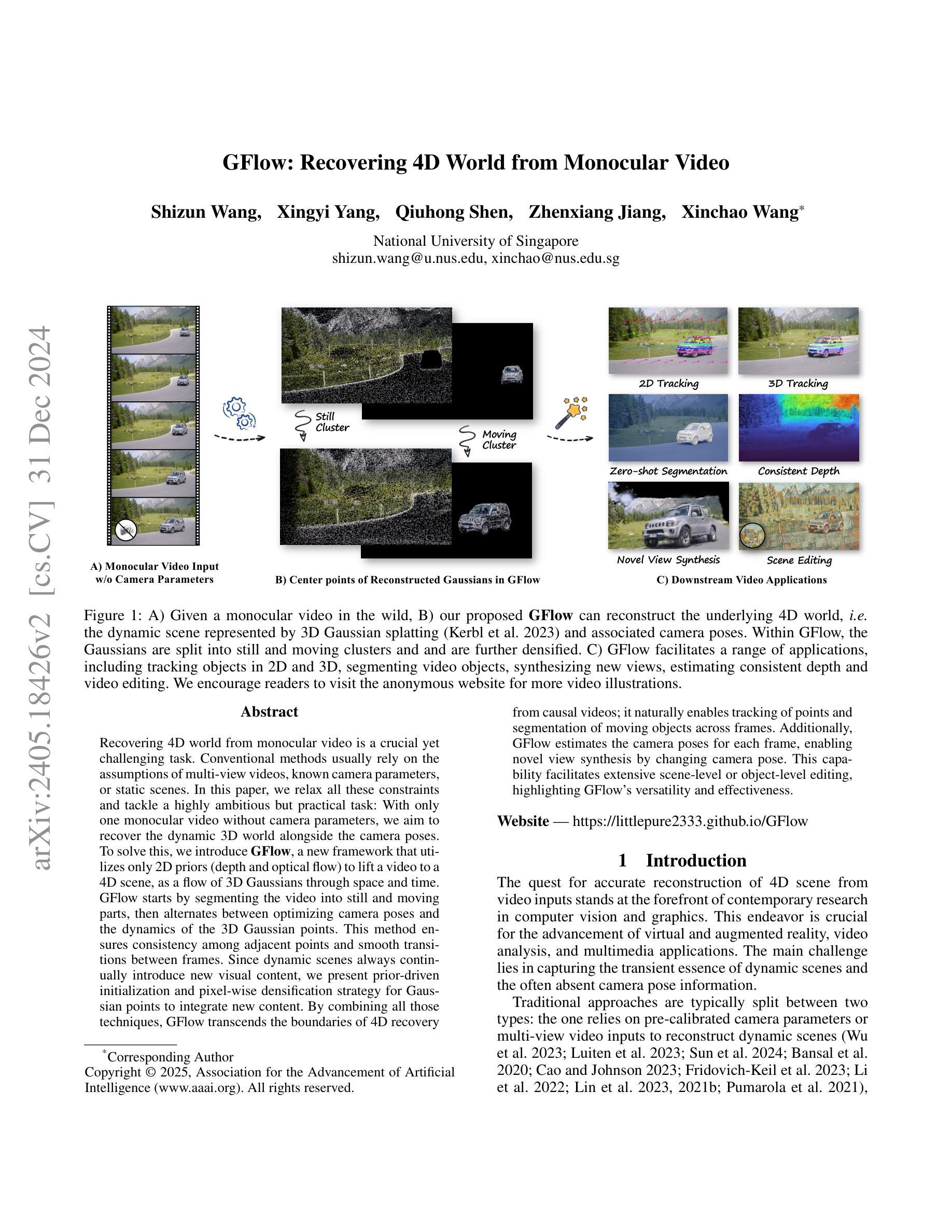
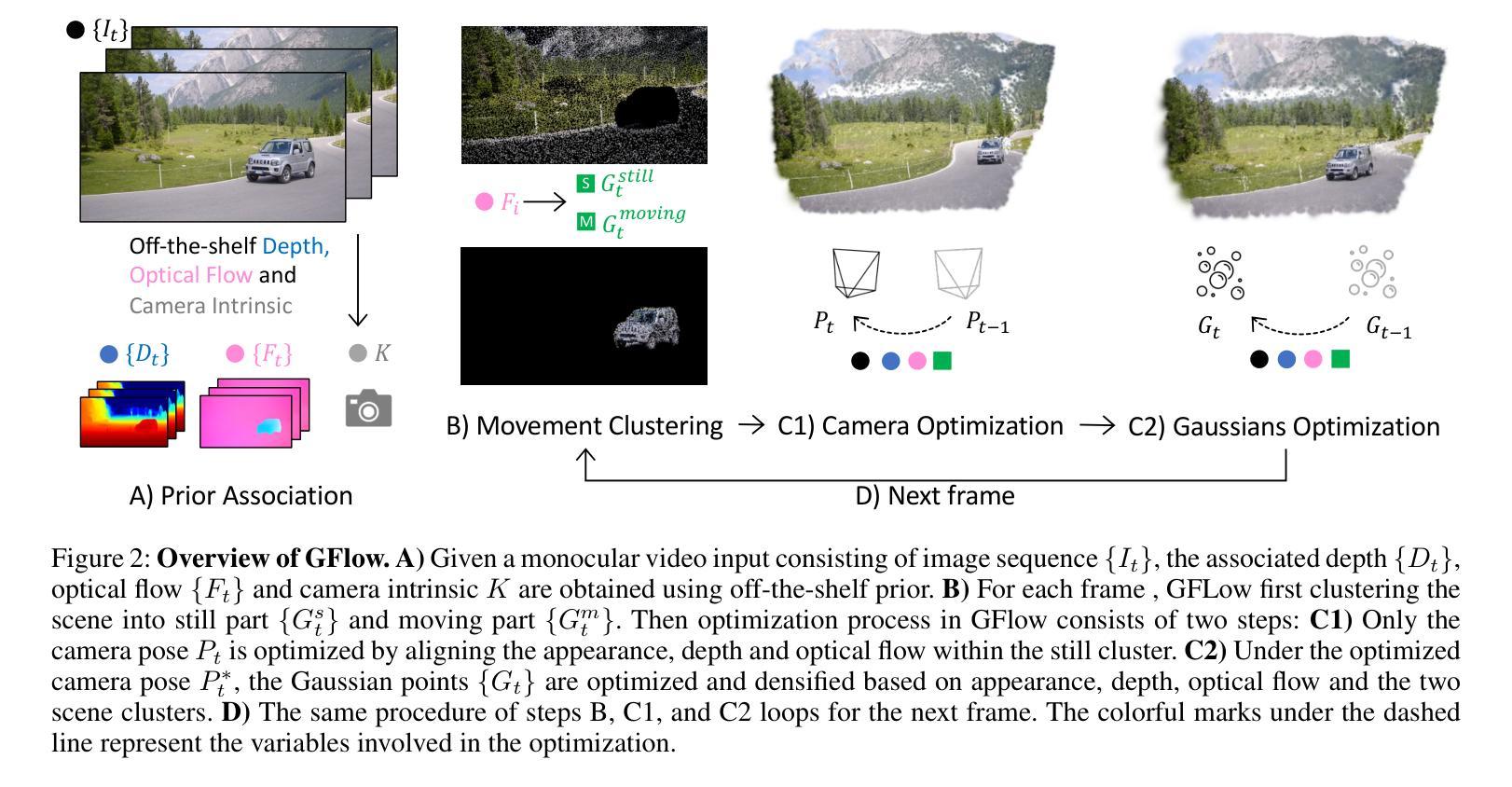
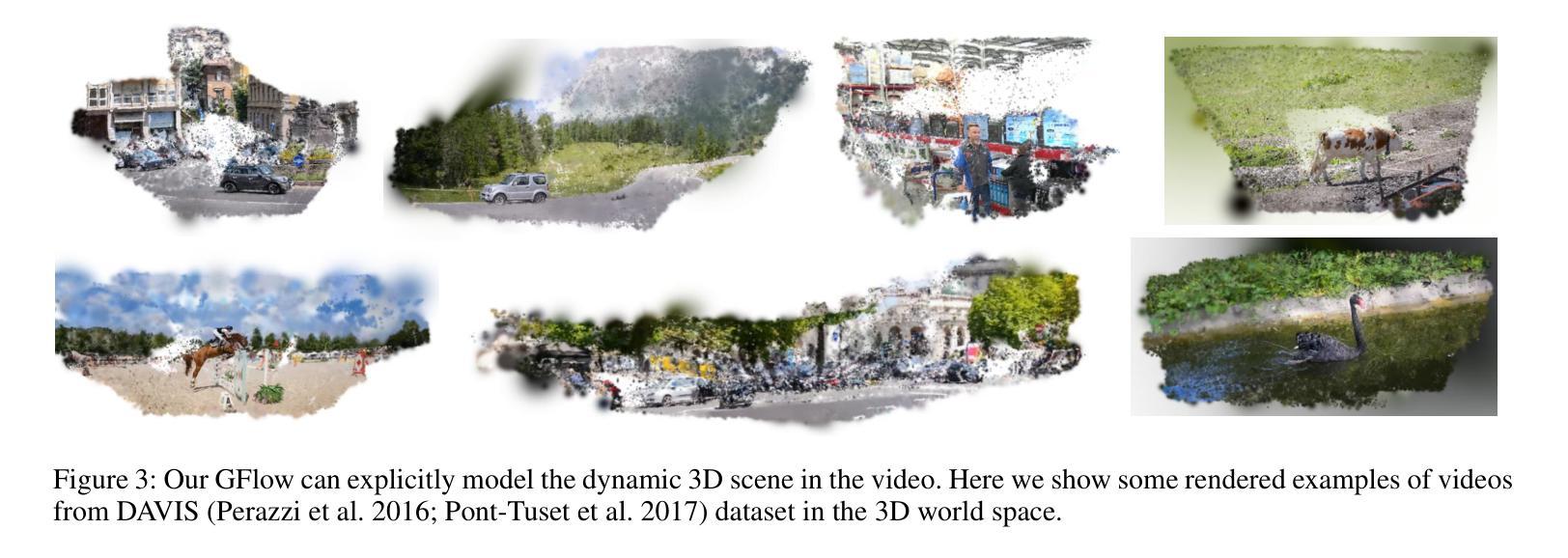
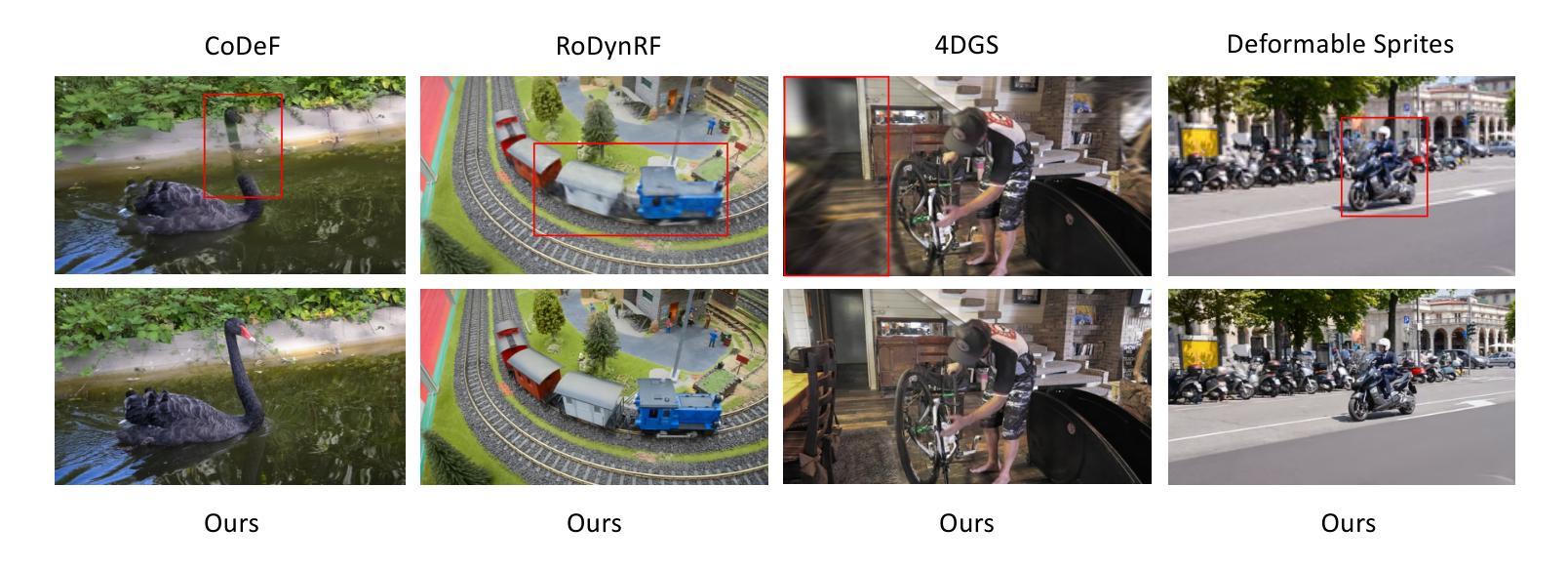