⚠️ 以下所有内容总结都来自于 大语言模型的能力,如有错误,仅供参考,谨慎使用
🔴 请注意:千万不要用于严肃的学术场景,只能用于论文阅读前的初筛!
💗 如果您觉得我们的项目对您有帮助 ChatPaperFree ,还请您给我们一些鼓励!⭐️ HuggingFace免费体验
2025-01-04 更新
Double-Flow GAN model for the reconstruction of perceived faces from brain activities
Authors:Zihao Wang, Jing Zhao, Xuetong Ding, Hui Zhang
Face plays an important role in humans visual perception, and reconstructing perceived faces from brain activities is challenging because of its difficulty in extracting high-level features and maintaining consistency of multiple face attributes, such as expression, identity, gender, etc. In this study, we proposed a novel reconstruction framework, which we called Double-Flow GAN, that can enhance the capability of discriminator and handle imbalances in images from certain domains that are too easy for generators. We also designed a pretraining process that uses features extracted from images as conditions for making it possible to pretrain the conditional reconstruction model from fMRI in a larger pure image dataset. Moreover, we developed a simple pretrained model for fMRI alignment to alleviate the problem of cross-subject reconstruction due to the variations of brain structure among different subjects. We conducted experiments by using our proposed method and traditional reconstruction models. Results showed that the proposed method is significant at accurately reconstructing multiple face attributes, outperforms the previous reconstruction models, and exhibited state-of-the-art reconstruction abilities.
面部在人类视觉感知中扮演着重要角色,而从脑活动中重建感知的面部具有挑战性,因为很难提取高级特征并保持多个面部属性的一致性,例如表情、身份、性别等。本研究提出了一种新型重建框架,我们称之为Double-Flow GAN,它可以增强鉴别器的能力,并处理某些领域图像中的不平衡问题,这些问题对于生成器来说太容易了。我们还设计了一个预训练过程,该过程使用从图像中提取的特征作为条件,使得能够在更大的纯图像数据集上从fMRI进行条件重建模型的预训练成为可能。此外,我们开发了一个简单的预训练模型用于fMRI对齐,以减轻由于不同受试者之间的大脑结构变化导致的跨主体重建问题。我们通过使用所提出的方法和传统重建模型进行了实验。结果表明,所提出的方法在准确重建多个面部属性方面表现显著,优于以前的重建模型,并展现了最先进的重建能力。
论文及项目相关链接
摘要
本文研究了面部重建的问题,提出了一种新的重建框架——Double-Flow GAN。该框架能提高鉴别器的性能,并处理特定领域图像中的不平衡问题。设计了一种预训练过程,使用从图像中提取的特征作为条件,使得可以在更大的纯图像数据集上预训练条件重建模型。此外,开发了一种简单的预训练模型用于fMRI对齐,以缓解由于不同受试者之间的大脑结构差异导致的跨主体重建问题。实验表明,该方法在准确重建多个面部特征方面表现优异,优于传统的重建模型,并展现出最先进的重建能力。
关键见解
- Double-Flow GAN框架能提高鉴别器的性能,并处理图像中的不平衡问题。
- 设计了一种预训练过程,使用图像特征作为条件,以便在更大的图像数据集上预训练条件重建模型。
- 开发了一种简单的预训练模型用于fMRI数据对齐,以减轻跨主体重建时的差异问题。
- 该方法可以准确重建多个面部特征,如表情、身份、性别等。
- 该方法在传统重建模型上表现出优越性,具有最先进的重建能力。
- 通过实验验证了该方法的有效性和优越性。
点此查看论文截图
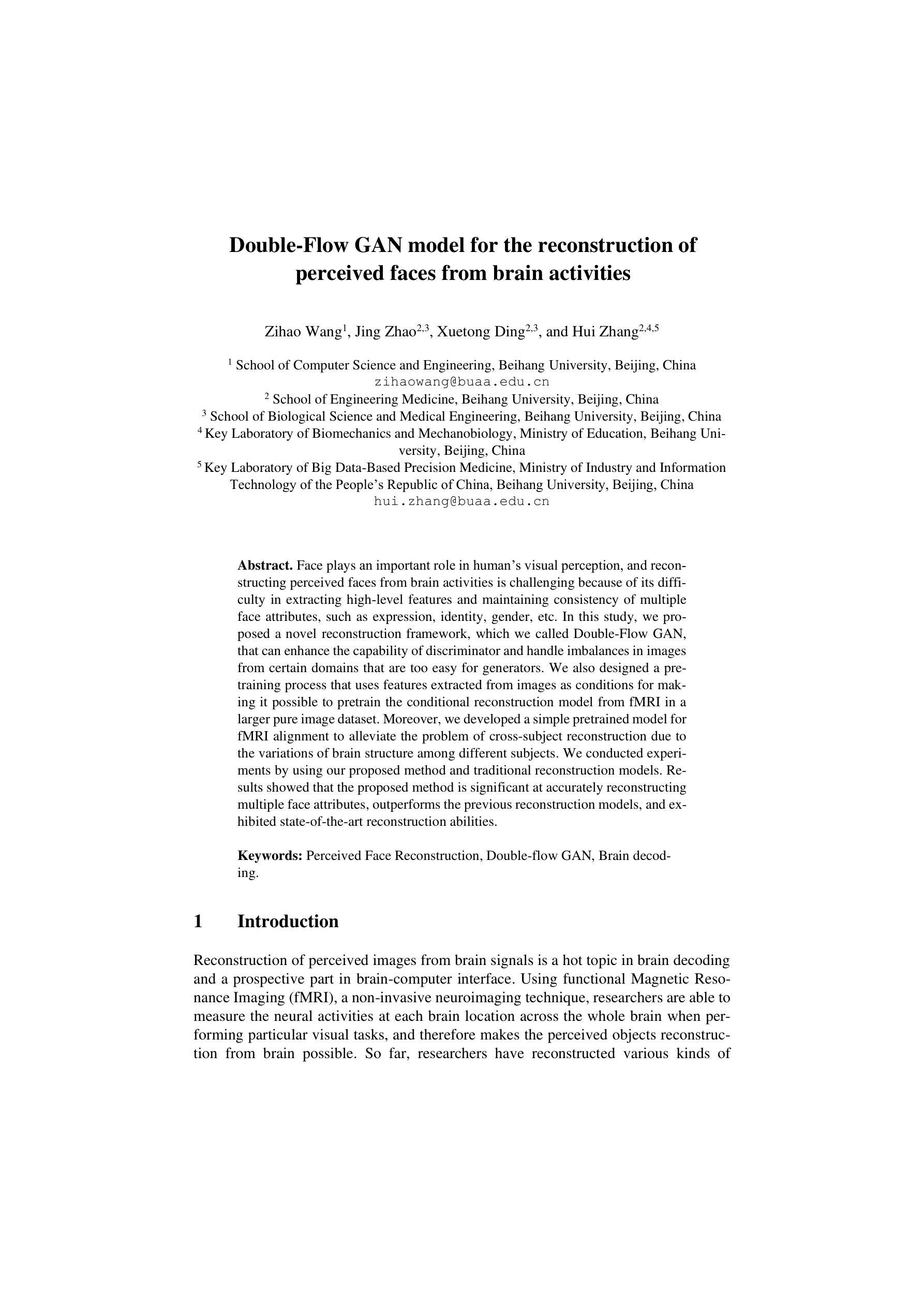
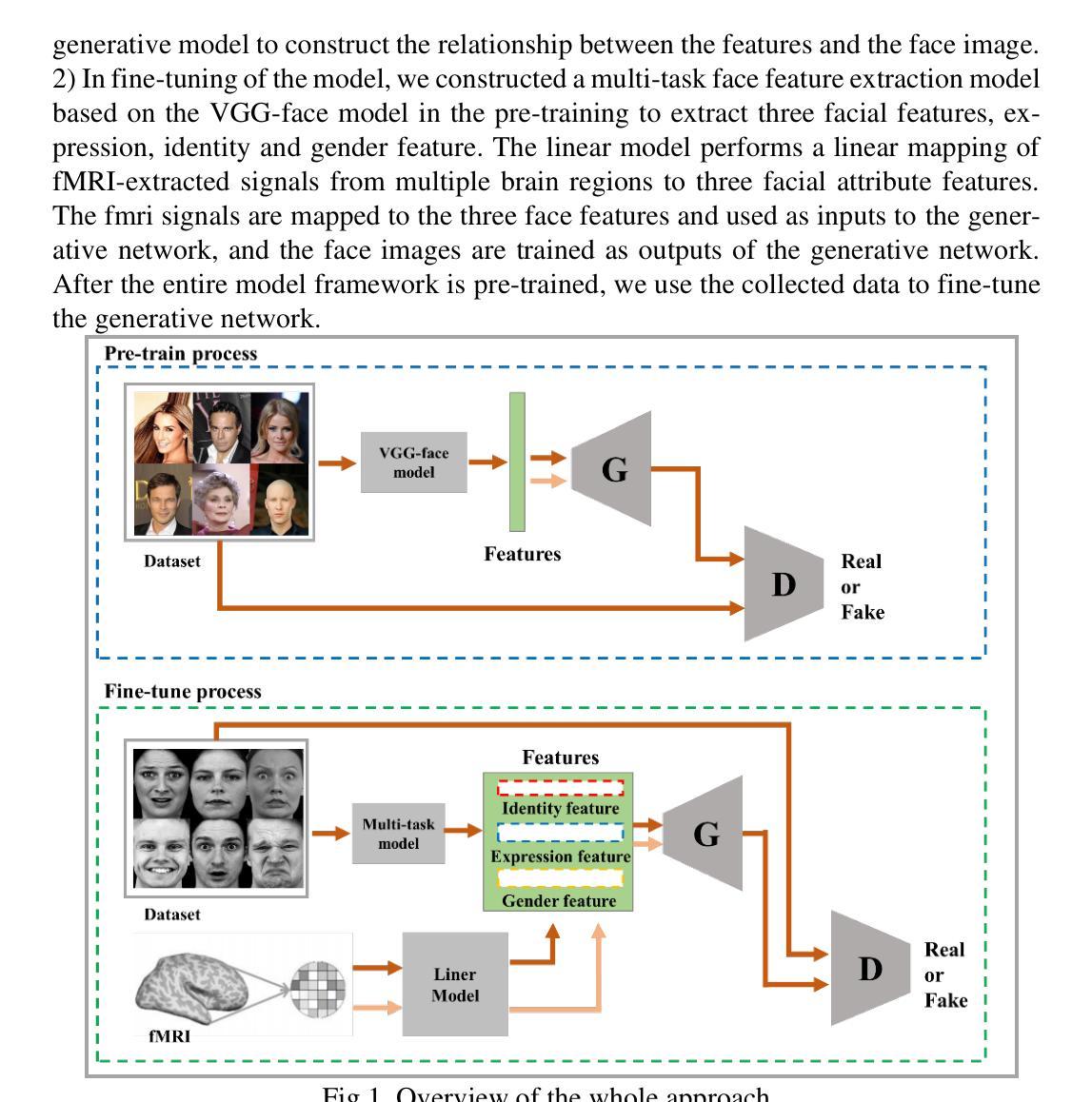
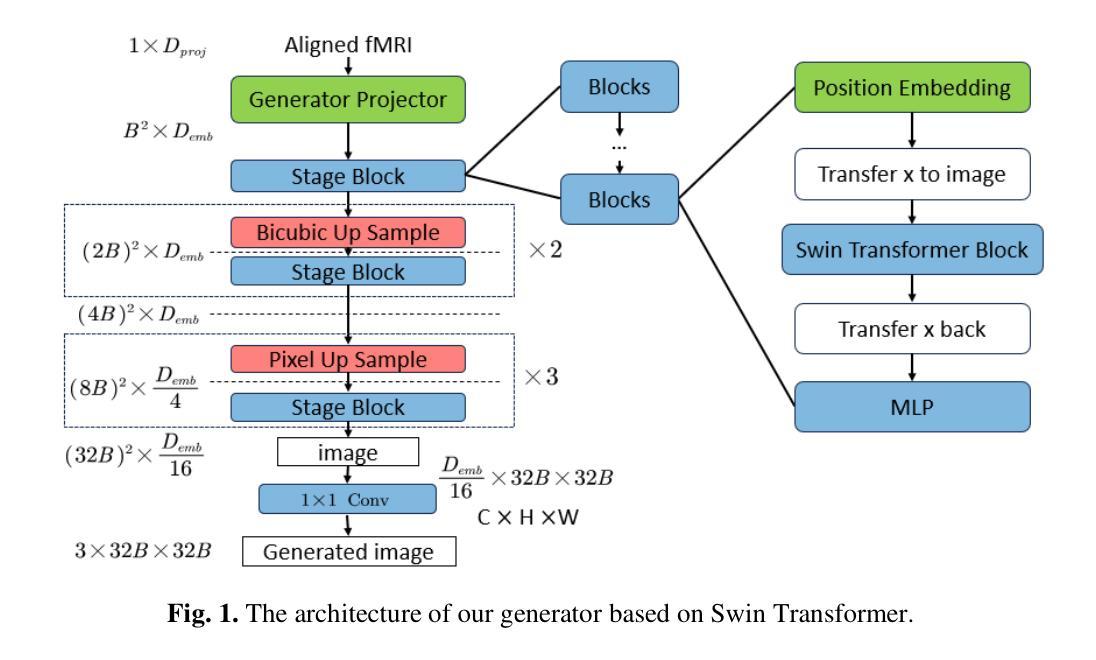