⚠️ 以下所有内容总结都来自于 大语言模型的能力,如有错误,仅供参考,谨慎使用
🔴 请注意:千万不要用于严肃的学术场景,只能用于论文阅读前的初筛!
💗 如果您觉得我们的项目对您有帮助 ChatPaperFree ,还请您给我们一些鼓励!⭐️ HuggingFace免费体验
2025-01-04 更新
SSR-Speech: Towards Stable, Safe and Robust Zero-shot Text-based Speech Editing and Synthesis
Authors:Helin Wang, Meng Yu, Jiarui Hai, Chen Chen, Yuchen Hu, Rilin Chen, Najim Dehak, Dong Yu
In this paper, we introduce SSR-Speech, a neural codec autoregressive model designed for stable, safe, and robust zero-shot textbased speech editing and text-to-speech synthesis. SSR-Speech is built on a Transformer decoder and incorporates classifier-free guidance to enhance the stability of the generation process. A watermark Encodec is proposed to embed frame-level watermarks into the edited regions of the speech so that which parts were edited can be detected. In addition, the waveform reconstruction leverages the original unedited speech segments, providing superior recovery compared to the Encodec model. Our approach achieves state-of-the-art performance in the RealEdit speech editing task and the LibriTTS text-to-speech task, surpassing previous methods. Furthermore, SSR-Speech excels in multi-span speech editing and also demonstrates remarkable robustness to background sounds. The source code and demos are released.
在本文中,我们介绍了SSR-Speech,这是一种为稳定、安全、稳健的零样本文本语音编辑和文本到语音合成设计的神经网络编解码器自回归模型。SSR-Speech基于Transformer解码器构建,并引入了无分类器引导技术以增强生成过程的稳定性。我们还提出了一种水印Encodec,可将水印嵌入语音的编辑区域,以便检测哪些部分已被编辑。此外,波形重建利用原始未编辑的语音片段,与Encodec模型相比,提供了更出色的恢复能力。我们的方法在实际编辑语音任务和LibriTTS文本到语音任务中实现了最先进的性能表现,超越了以前的方法。此外,SSR-Speech在多跨度语音编辑方面表现出色,并且对背景声音表现出了令人印象深刻的稳健性。源代码和演示程序已发布。
论文及项目相关链接
PDF ICASSP 2025
Summary
SSR-Speech模型是一种基于神经的编码解码自回归模型,设计用于稳定、安全、稳健的零步文本语音编辑和文本到语音合成。该模型使用Transformer解码器构建,并引入无分类器引导以增强生成过程的稳定性。提出的水印Encodec可将帧级水印嵌入语音的编辑区域,以便检测哪些部分已被编辑。此外,波形重建利用原始未编辑的语音片段,提供了比Encodec模型更出色的恢复效果。SSR-Speech在RealEdit语音编辑任务和LibriTTS文本到语音任务上达到了最新技术水平,超越了以前的方法。该模型在多跨度语音编辑方面表现出色,并对背景声音显示出惊人的稳健性。已发布源代码和演示。
Key Takeaways
- SSR-Speech是一个基于神经的编码解码自回归模型,用于稳定、安全、稳健的文本语音编辑和文本到语音合成。
- 该模型使用Transformer解码器和无分类器引导,增强了生成过程的稳定性。
- SSR-Speech通过水印Encodec嵌入帧级水印,能检测语音中哪些部分已被编辑。
- 波形重建利用原始未编辑的语音片段,提供高质量恢复效果。
- SSR-Speech在RealEdit和LibriTTS任务上表现优秀,超越先前方法。
- 模型在多跨度语音编辑方面有出色表现,并对背景声音保持稳定。
点此查看论文截图
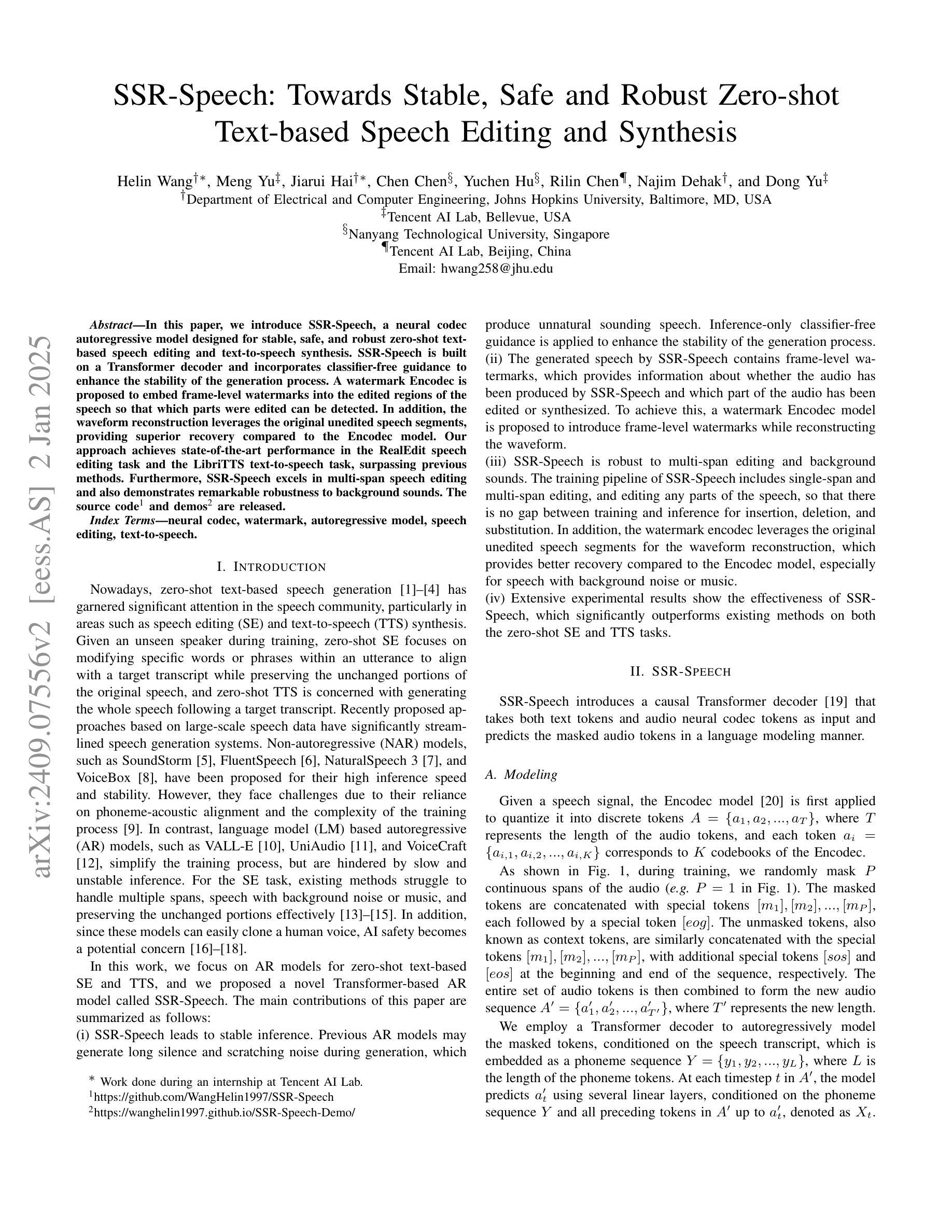
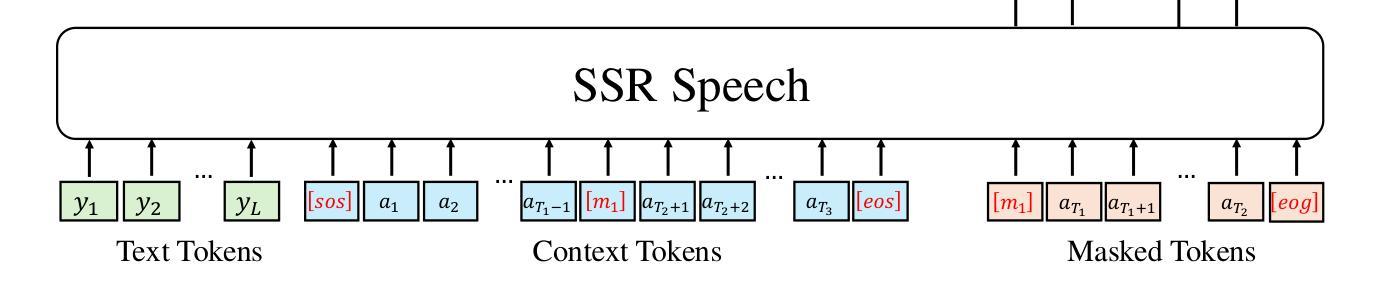
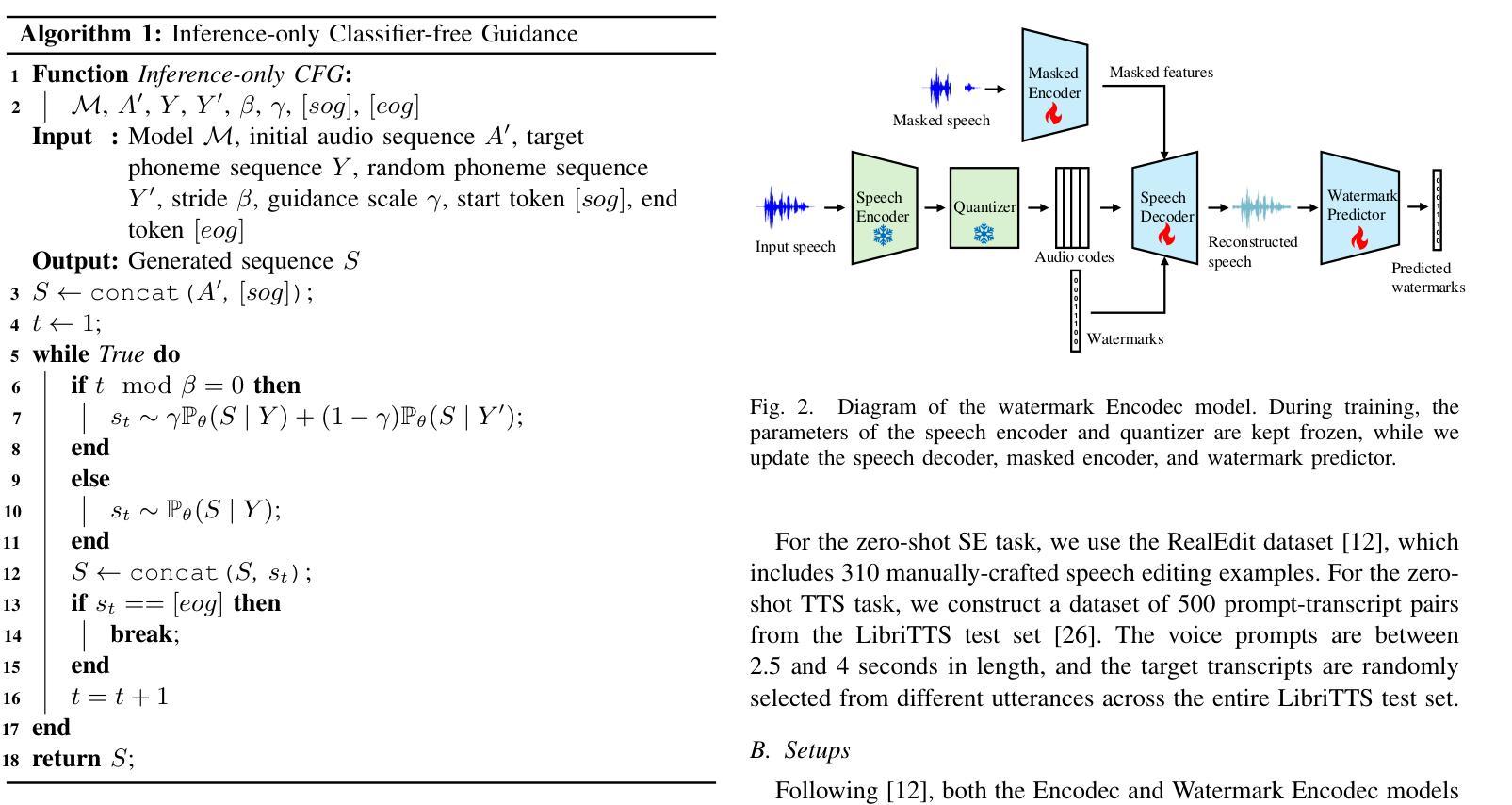
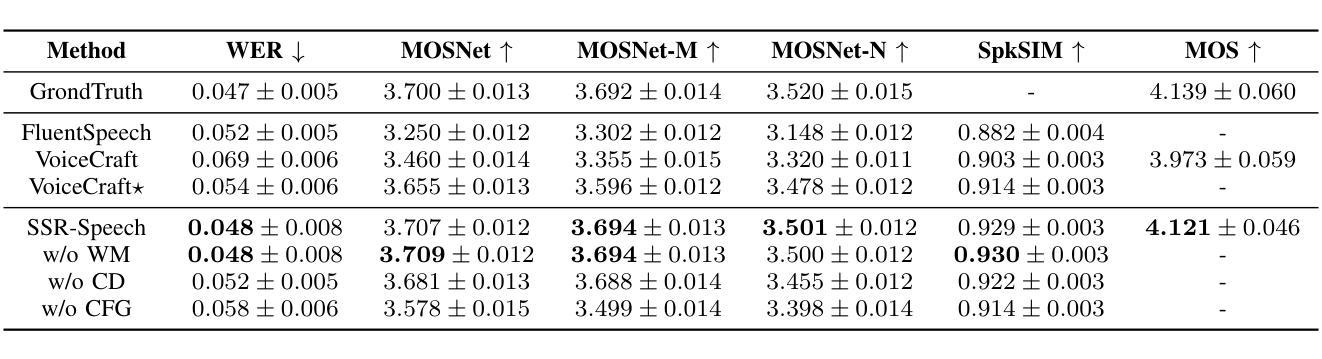
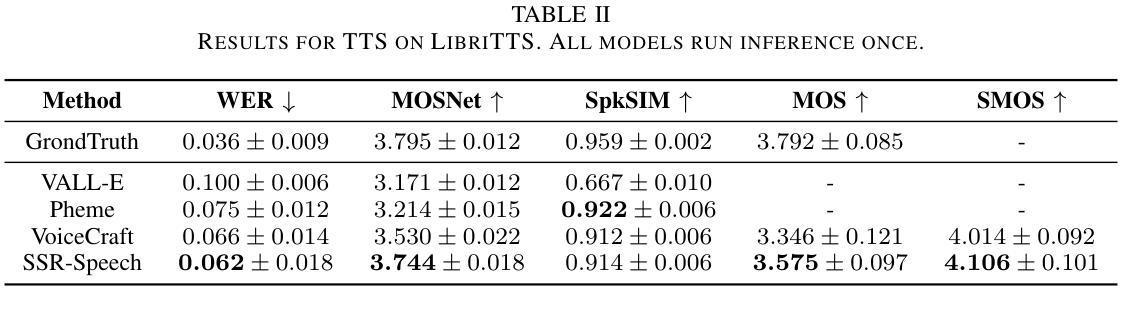
Multi-Scale Accent Modeling and Disentangling for Multi-Speaker Multi-Accent Text-to-Speech Synthesis
Authors:Xuehao Zhou, Mingyang Zhang, Yi Zhou, Zhizheng Wu, Haizhou Li
Generating speech across different accents while preserving speaker identity is crucial for various real-world applications. However, accurately and independently modeling both speaker and accent characteristics in text-to-speech (TTS) systems is challenging due to the complex variations of accents and the inherent entanglement between speaker and accent identities. In this paper, we propose a novel approach for multi-speaker multi-accent TTS synthesis that aims to synthesize speech for multiple speakers, each with various accents. Our approach employs a multi-scale accent modeling strategy to address accent variations on different levels. Specifically, we introduce both global (utterance level) and local (phoneme level) accent modeling to capture overall accent characteristics within an utterance and fine-grained accent variations across phonemes, respectively. To enable independent control of speakers and accents, we use the speaker embedding to represent speaker identity and achieve speaker-independent accent control through speaker disentanglement within the multi-scale accent modeling. Additionally, we present a local accent prediction model that enables our system to generate accented speech directly from phoneme inputs. We conduct extensive experiments on an English accented speech corpus. Experimental results demonstrate that our proposed system outperforms baseline systems in terms of speech quality and accent rendering for generating multi-speaker multi-accent speech. Ablation studies further validate the effectiveness of different components in our proposed system.
在不同口音中生成语音的同时保持说话人身份,对于各种实际应用至关重要。然而,由于口音的复杂变化和说话人与口音身份之间的固有纠缠,在文本到语音(TTS)系统中准确且独立地建模说话人和口音特征是充满挑战的。在本文中,我们提出了一种针对多说话人多口音的TTS合成的新方法,旨在合成多个说话人的语音,每个说话人具有不同的口音。我们的方法采用多尺度口音建模策略,以处理不同层次的口音变化。具体来说,我们引入了全局(句子级别)和局部(音素级别)的口音建模,以捕捉句子中的整体口音特征,并分别捕捉音素之间的细微口音变化。为了实现说话人和口音的独立控制,我们使用说话人嵌入来表示说话人身份,并通过多尺度口音建模中的说话人解耦来实现与说话人无关的口音控制。此外,我们提出了一个局部口音预测模型,使我们的系统能够直接从音素输入生成带口音的语音。我们在英语口音语音语料库上进行了大量实验。实验结果表明,我们提出的系统在生成多说话人多口音的语音方面,在语音质量和口音呈现方面优于基线系统。消融研究进一步验证了我们的系统中不同组件的有效性。
论文及项目相关链接
Summary
本文提出一种多发言人、多口音的TTS合成方法,旨在合成多个发言人带有不同口音的语音。采用多尺度口音建模策略应对不同层次的口音变化,并引入全局和局部口音建模,分别捕捉话语中的整体口音特征和音素间的细微变化。通过运用发言人嵌入技术实现独立控制发言人和口音,提出本地口音预测模型,直接从音素输入生成带口音的语音。实验结果表明,该系统在生成多发言人、多口音的语音方面优于基准系统。
Key Takeaways
- 提出了一种多发言人、多口音的TTS合成方法,能合成带有不同口音的多个发言人的语音。
- 采用多尺度口音建模策略,应对不同层次的口音变化。
- 引入全局和局部口音建模,分别捕捉整体口音特征和音素间的细微变化。
- 通过运用发言人嵌入技术,实现了独立控制发言人和口音。
- 提出了一个本地口音预测模型,能够直接从音素输入生成带口音的语音。
- 在英语口音语音库上进行了大量实验,结果表明该系统在语音质量和口音表现上优于基准系统。
点此查看论文截图
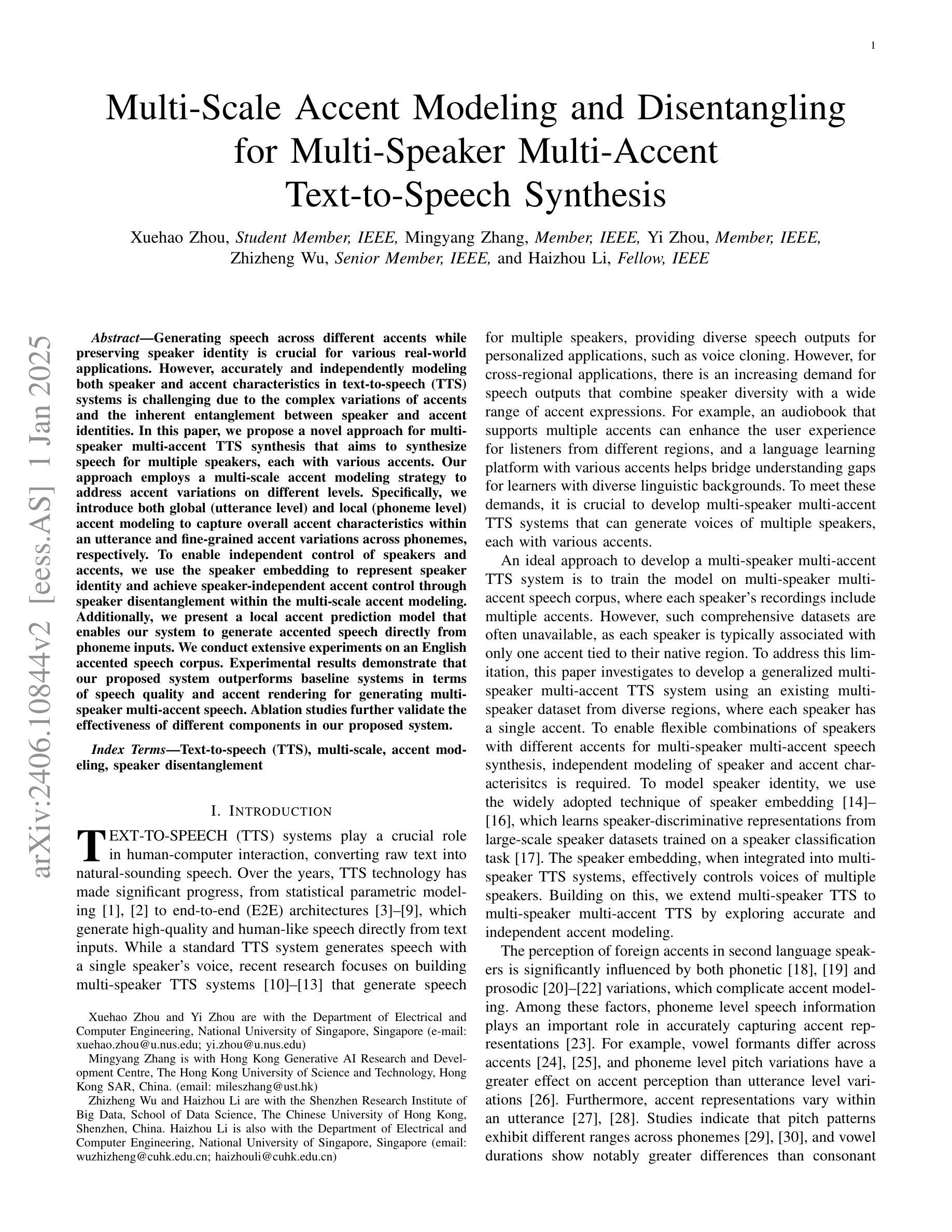
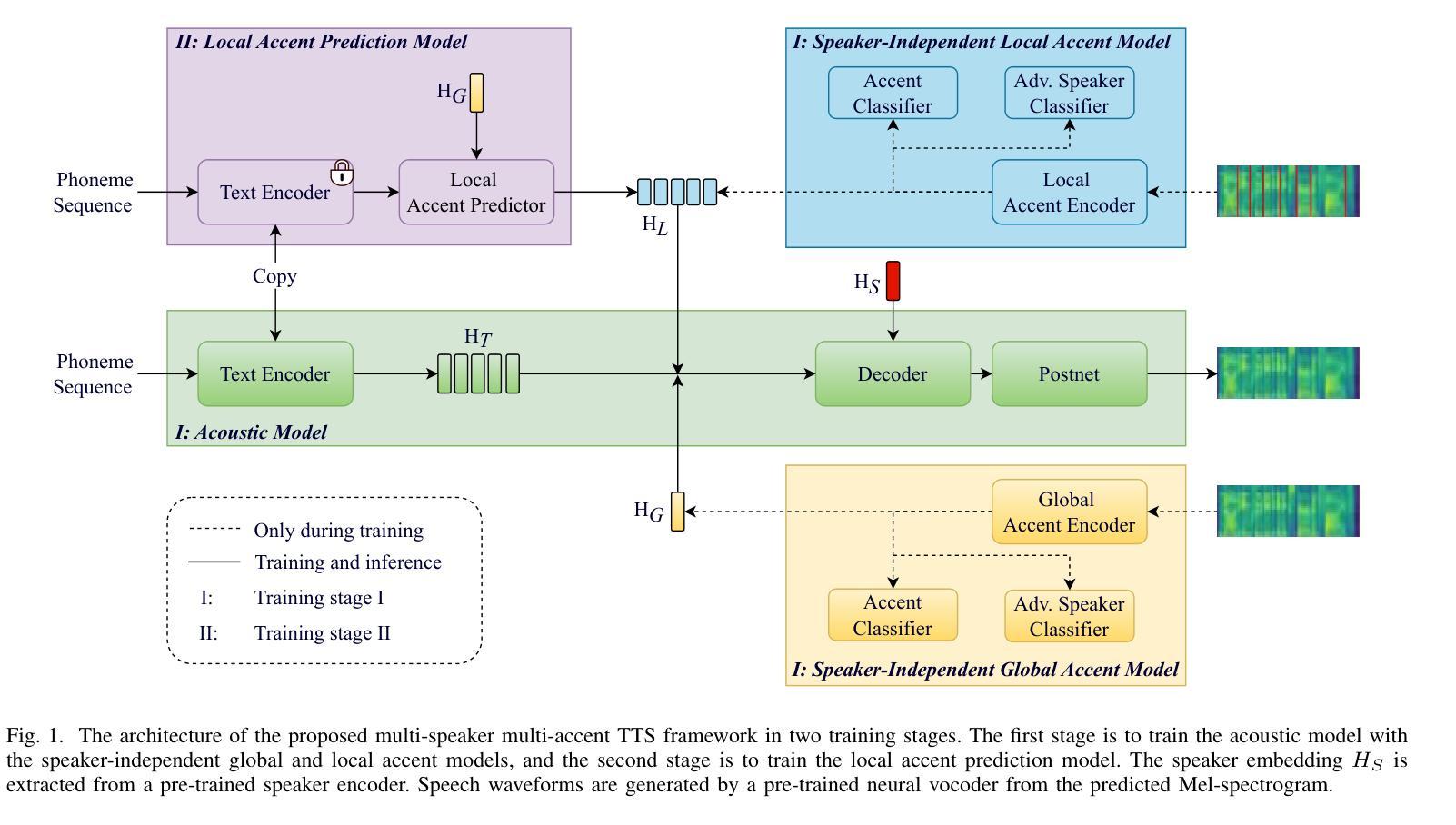
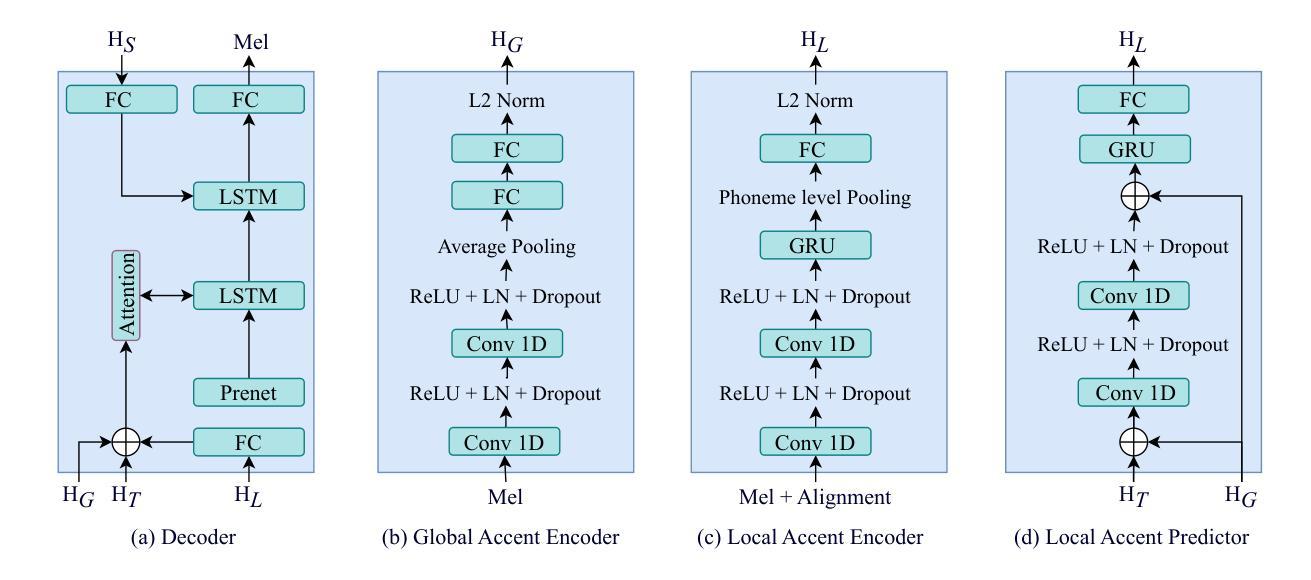