⚠️ 以下所有内容总结都来自于 大语言模型的能力,如有错误,仅供参考,谨慎使用
🔴 请注意:千万不要用于严肃的学术场景,只能用于论文阅读前的初筛!
💗 如果您觉得我们的项目对您有帮助 ChatPaperFree ,还请您给我们一些鼓励!⭐️ HuggingFace免费体验
2025-01-04 更新
ARNet: Self-Supervised FG-SBIR with Unified Sample Feature Alignment and Multi-Scale Token Recycling
Authors:Jianan Jiang, Hao Tang, Zhilin Jiang, Weiren Yu, Di Wu
Fine-Grained Sketch-Based Image Retrieval (FG-SBIR) aims to minimize the distance between sketches and corresponding images in the embedding space. However, scalability is hindered by the growing complexity of solutions, mainly due to the abstract nature of fine-grained sketches. In this paper, we propose an effective approach to narrow the gap between the two domains. It mainly facilitates unified mutual information sharing both intra- and inter-samples, rather than treating them as a single feature alignment problem between modalities. Specifically, our approach includes: (i) Employing dual weight-sharing networks to optimize alignment within the sketch and image domain, which also effectively mitigates model learning saturation issues. (ii) Introducing an objective optimization function based on contrastive loss to enhance the model’s ability to align features in both intra- and inter-samples. (iii) Presenting a self-supervised Multi-Scale Token Recycling (MSTR) Module featured by recycling discarded patch tokens in multi-scale features, further enhancing representation capability and retrieval performance. Our framework achieves excellent results on CNN- and ViT-based backbones. Extensive experiments demonstrate its superiority over existing methods. We also introduce Cloths-V1, the first professional fashion sketch-image dataset, utilized to validate our method and will be beneficial for other applications.
细粒度草图基于图像检索(FG-SBIR)旨在最小化嵌入空间中草图与对应图像之间的距离。然而,随着解决方案的复杂性增长,可扩展性受到阻碍,这主要是由于细粒度草图的抽象性质。在本文中,我们提出了一种有效的方法来缩小两个领域之间的差距。它主要促进了跨内样本和跨样本的统一信息共享,而不是将它们视为不同模态之间的单一特征对齐问题。具体来说,我们的方法包括:(i)采用双权重共享网络来优化草图域和图像域内的对齐,这也可以有效地缓解模型学习饱和问题。(ii)引入基于对比损失的客观优化函数,以提高模型在内部和外部样本中对齐特征的能力。(iii)提出了一种具有多尺度特征中丢弃的补丁令牌回收功能的自监督多尺度令牌回收(MSTR)模块,进一步提高表示能力和检索性能。我们的框架在基于CNN和ViT的主干网络上取得了优异的结果。大量实验证明了其优于现有方法。我们还介绍了Cloths-V1,这是第一个专业时尚草图图像数据集,用于验证我们的方法,并将对其他应用有益。
论文及项目相关链接
PDF Accepted by the 39th Annual AAAI Conference on Artificial Intelligence (AAAI-25)
Summary
本文提出了一种针对细粒度草图基于图像检索(FG-SBIR)的有效方法,旨在缩小草图与图像领域之间的差距。该方法通过统一跨样本和样本内的信息共享,而非将其视为单一特征对齐问题,来解决模型的复杂性和抽象性挑战。该方法包括使用双权重共享网络优化草图与图像领域内的对齐,引入基于对比损失的优化函数,以及提出自我监督的多尺度令牌回收模块。该方法在CNN和ViT骨干网络上均取得了优异的结果。同时,本文还介绍了首个专业时尚草图图像数据集Cloths-V1,用于验证方法的有效性,并将对其他应用产生积极影响。
Key Takeaways
- 本文旨在缩小细粒度草图与图像在嵌入空间中的距离,解决FG-SBIR的域差距问题。
- 提出了一种统一的信息共享方法,跨样本和样本内实现信息共享。
- 使用了双权重共享网络来优化草图与图像领域内的对齐,并解决了模型学习饱和问题。
- 引入了基于对比损失的优化函数,增强了模型在跨样本和样本内的特征对齐能力。
- 提出了自我监督的多尺度令牌回收模块,通过回收丢弃的补丁令牌增强表示能力和检索性能。
- 在CNN和ViT骨干网络上进行了广泛实验,证明了该方法的优越性。
点此查看论文截图
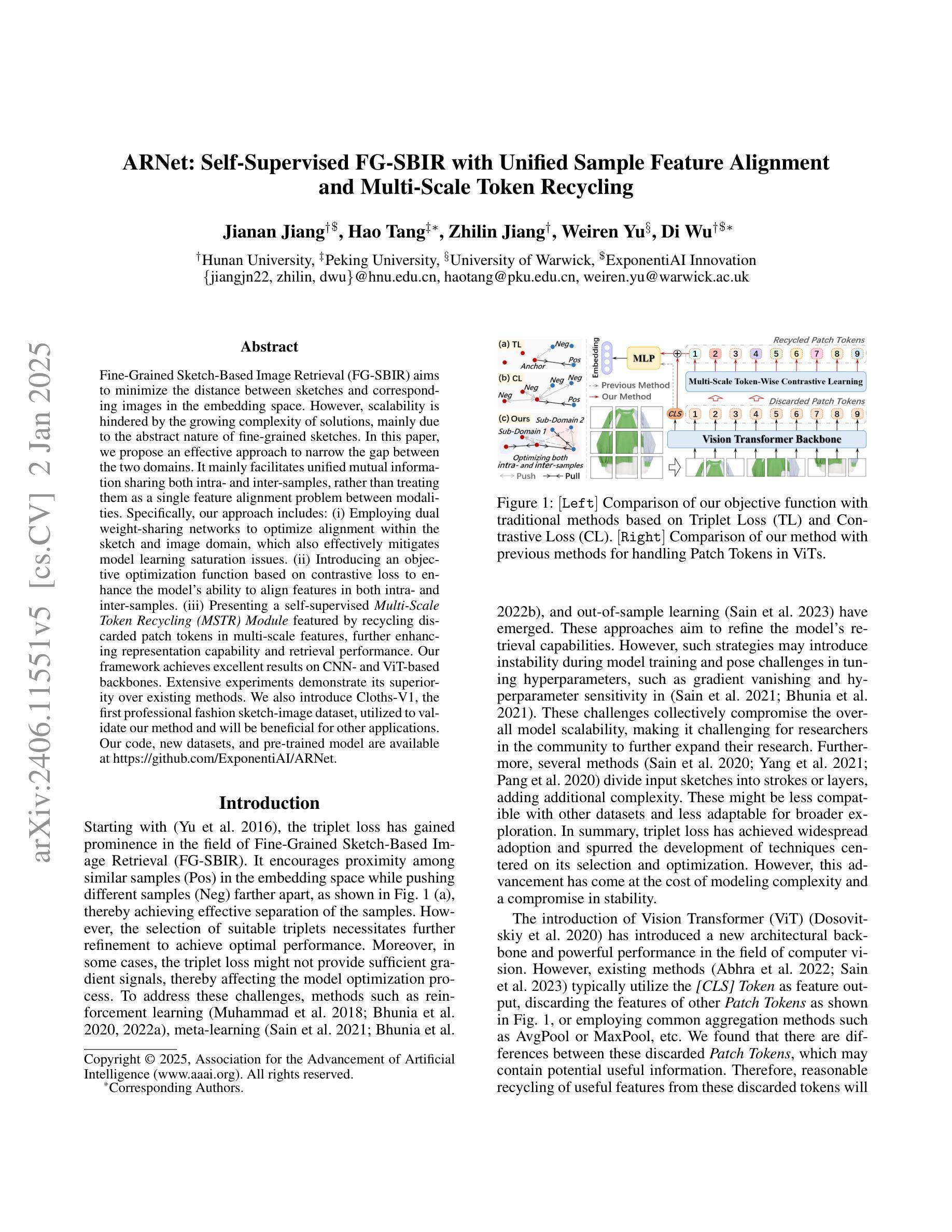
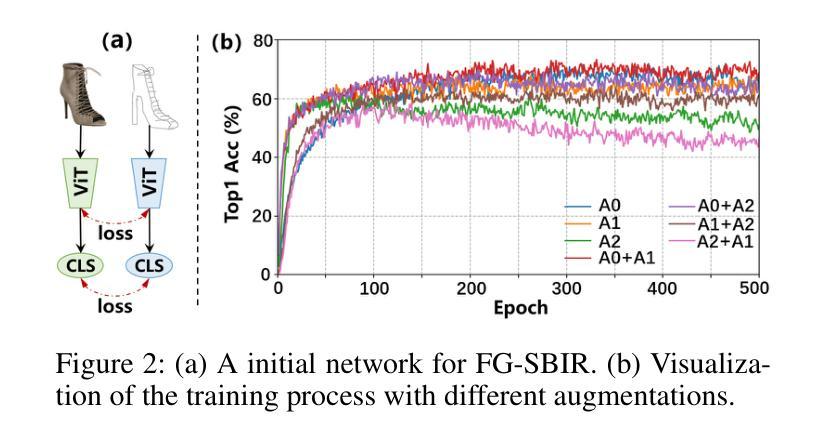
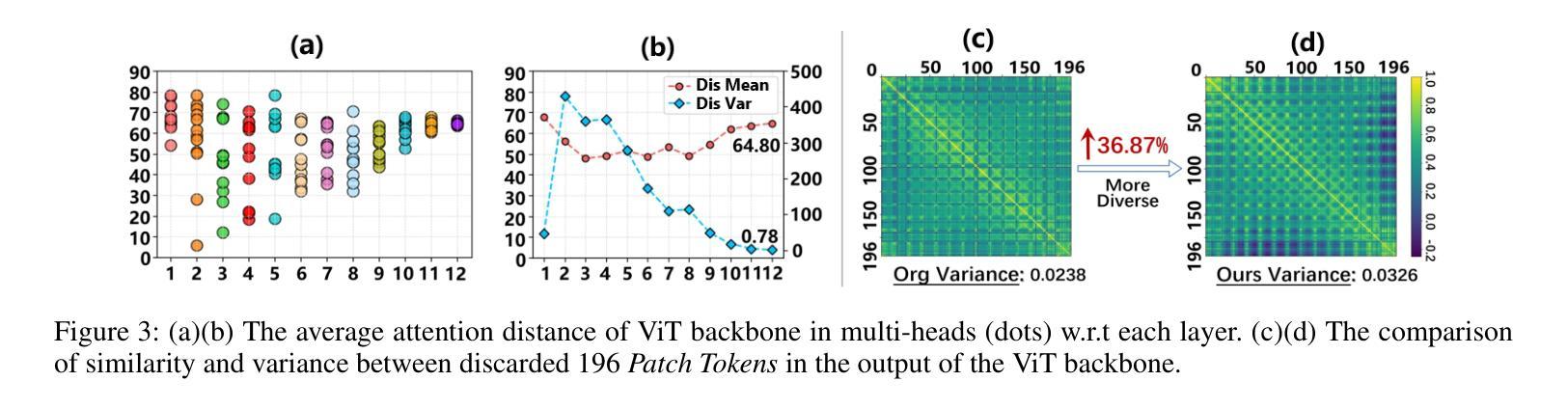
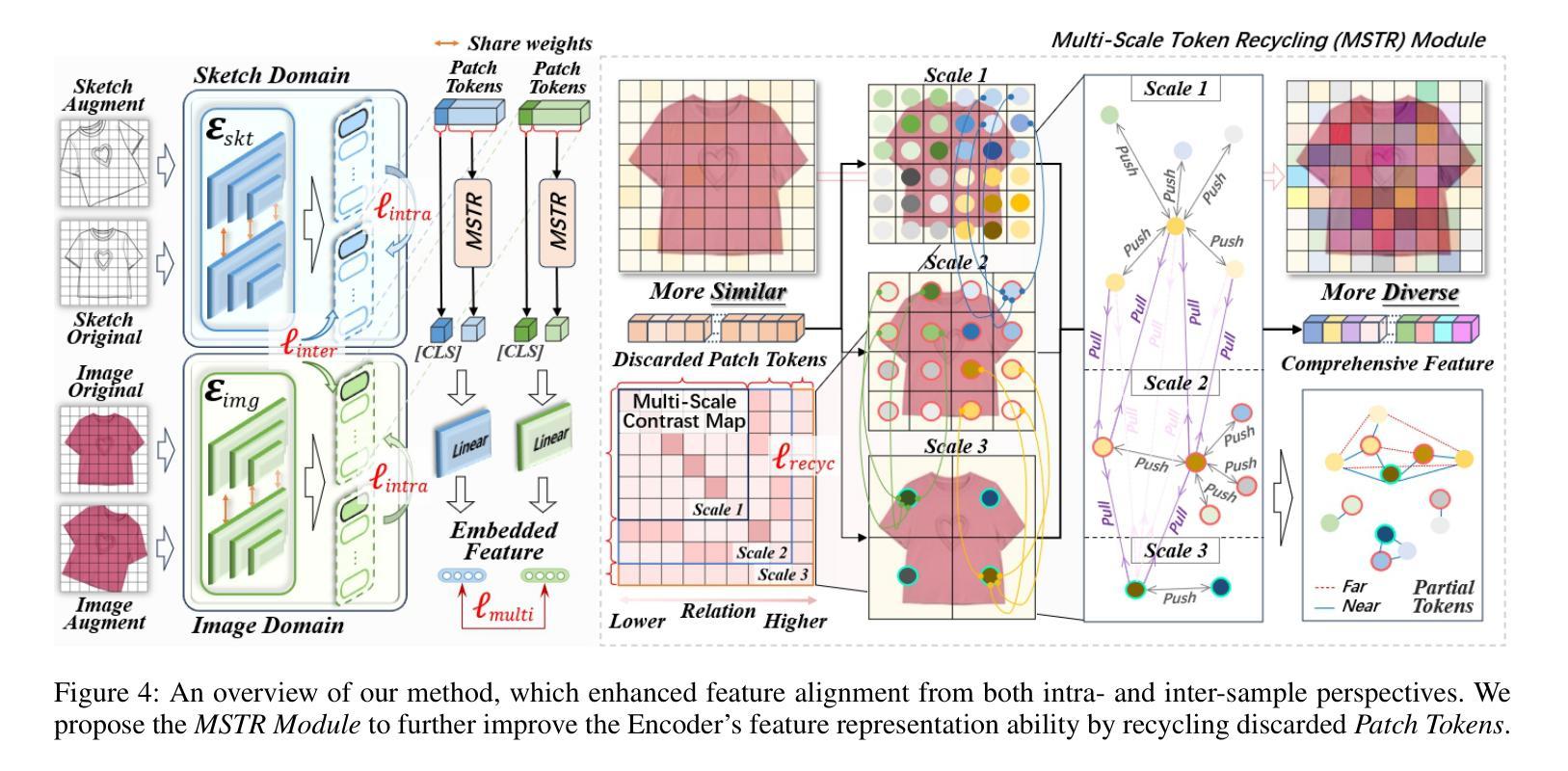
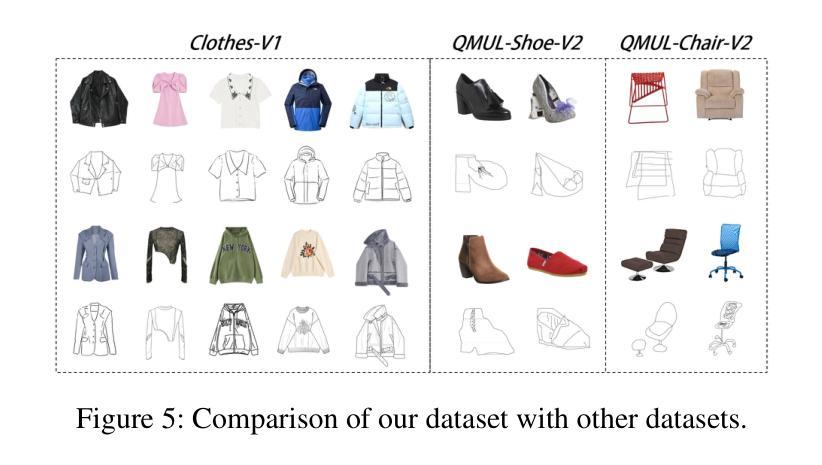
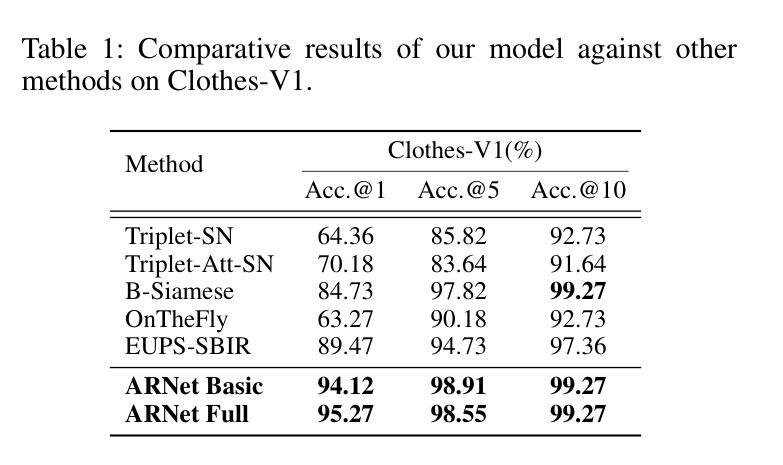
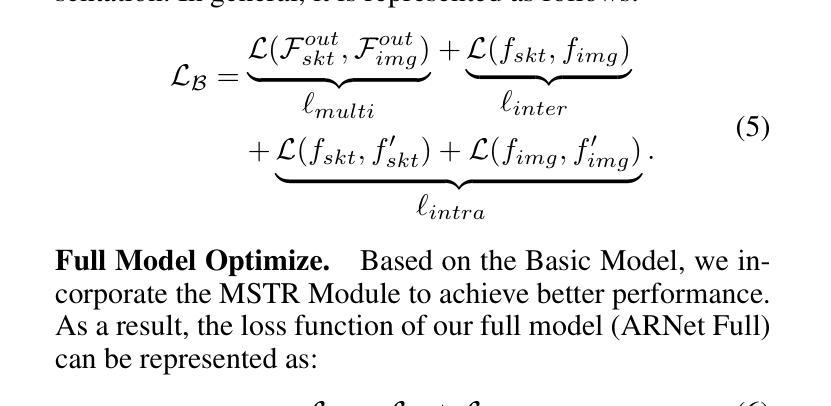
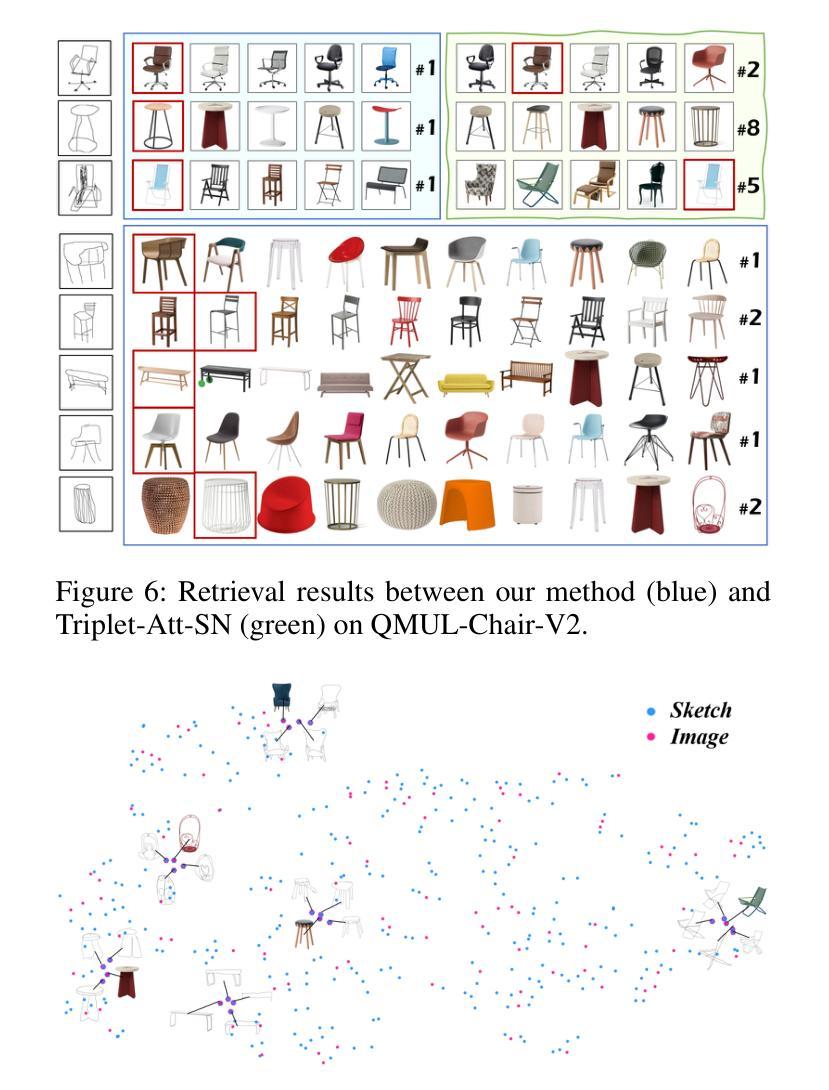
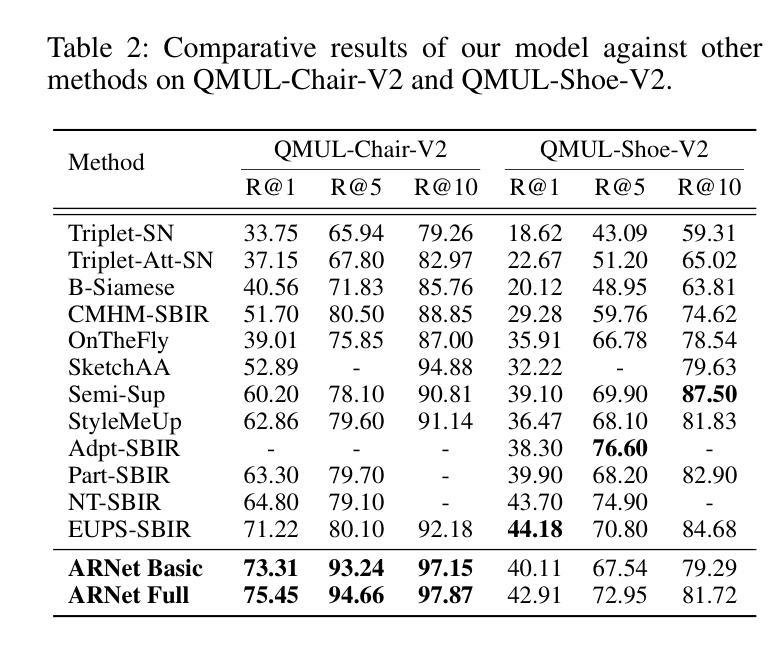