⚠️ 以下所有内容总结都来自于 大语言模型的能力,如有错误,仅供参考,谨慎使用
🔴 请注意:千万不要用于严肃的学术场景,只能用于论文阅读前的初筛!
💗 如果您觉得我们的项目对您有帮助 ChatPaperFree ,还请您给我们一些鼓励!⭐️ HuggingFace免费体验
2025-01-06 更新
ProjectedEx: Enhancing Generation in Explainable AI for Prostate Cancer
Authors:Xuyin Qi, Zeyu Zhang, Aaron Berliano Handoko, Huazhan Zheng, Mingxi Chen, Ta Duc Huy, Vu Minh Hieu Phan, Lei Zhang, Linqi Cheng, Shiyu Jiang, Zhiwei Zhang, Zhibin Liao, Yang Zhao, Minh-Son To
Prostate cancer, a growing global health concern, necessitates precise diagnostic tools, with Magnetic Resonance Imaging (MRI) offering high-resolution soft tissue imaging that significantly enhances diagnostic accuracy. Recent advancements in explainable AI and representation learning have significantly improved prostate cancer diagnosis by enabling automated and precise lesion classification. However, existing explainable AI methods, particularly those based on frameworks like generative adversarial networks (GANs), are predominantly developed for natural image generation, and their application to medical imaging often leads to suboptimal performance due to the unique characteristics and complexity of medical image. To address these challenges, our paper introduces three key contributions. First, we propose ProjectedEx, a generative framework that provides interpretable, multi-attribute explanations, effectively linking medical image features to classifier decisions. Second, we enhance the encoder module by incorporating feature pyramids, which enables multiscale feedback to refine the latent space and improves the quality of generated explanations. Additionally, we conduct comprehensive experiments on both the generator and classifier, demonstrating the clinical relevance and effectiveness of ProjectedEx in enhancing interpretability and supporting the adoption of AI in medical settings. Code will be released at https://github.com/Richardqiyi/ProjectedEx
前列腺癌是日益增长的全球健康问题,需要精确的诊断工具。磁共振成像(MRI)提供高分辨率的软组织成像,显著提高了诊断的准确性。最近,解释性人工智能和表示学习的进步通过实现自动化和精确的病变分类,显著提高了前列腺癌的诊断水平。然而,现有的解释性人工智能方法,特别是基于生成对抗网络(GANs)等框架的方法,主要是为自然图像生成而开发的,由于其独特的特点和复杂性,它们在医学成像中的应用往往导致性能不佳。为了应对这些挑战,我们的论文提出了三个关键贡献。首先,我们提出了ProjectedEx,这是一个生成框架,提供可解释的多属性解释,有效地将医学图像特征与分类器决策联系起来。其次,我们通过融入特征金字塔增强了编码器模块,这启用了多尺度反馈来优化潜在空间,提高了生成解释的质量。此外,我们对生成器和分类器都进行了全面的实验,证明了ProjectedEx在临床相关性和有效性方面的优势,提高了可解释性,支持在医疗环境中采用人工智能。代码将在https://github.com/Richardqiyi/ProjectedEx公布。
论文及项目相关链接
Summary
前列腺癌诊断领域亟待精准工具,而磁共振成像(MRI)能生成高分辨软组织图像提高诊断准确度。新的解释性人工智能与表示学习技术提高了前列腺癌诊断的准确性。但现有的解释性人工智能方法,特别是基于生成对抗网络(GANs)的方法主要应用于自然图像生成,应用于医学成像时性能往往不佳。本研究提出ProjectedEx框架,为医学图像特征提供可解释的多属性解释,连接医学图像特征与分类器决策。同时,通过引入特征金字塔增强编码器模块,实现多尺度反馈以优化潜在空间并提高解释质量。本研究通过实验验证了ProjectedEx的临床相关性和有效性,提高了人工智能在医疗环境中的可解释性和采用度。
Key Takeaways
- 前列腺癌的诊断需要精确的工具,而MRI提供高解析度的软组织图像增强了诊断的准确性。
- 解释性人工智能与表示学习技术改进了前列腺癌的诊断过程。
- 当前解释性人工智能方法在医学成像应用中性能受限,主要挑战在于医学图像的独特特性和复杂性。
- 本研究引入ProjectedEx框架,有效连接医学图像特征与分类器决策,提供可解释的多属性解释。
- 通过引入特征金字塔增强编码器模块,实现多尺度反馈,优化潜在空间并提高解释质量。
- ProjectedEx的临床相关性和有效性通过实验验证。
点此查看论文截图
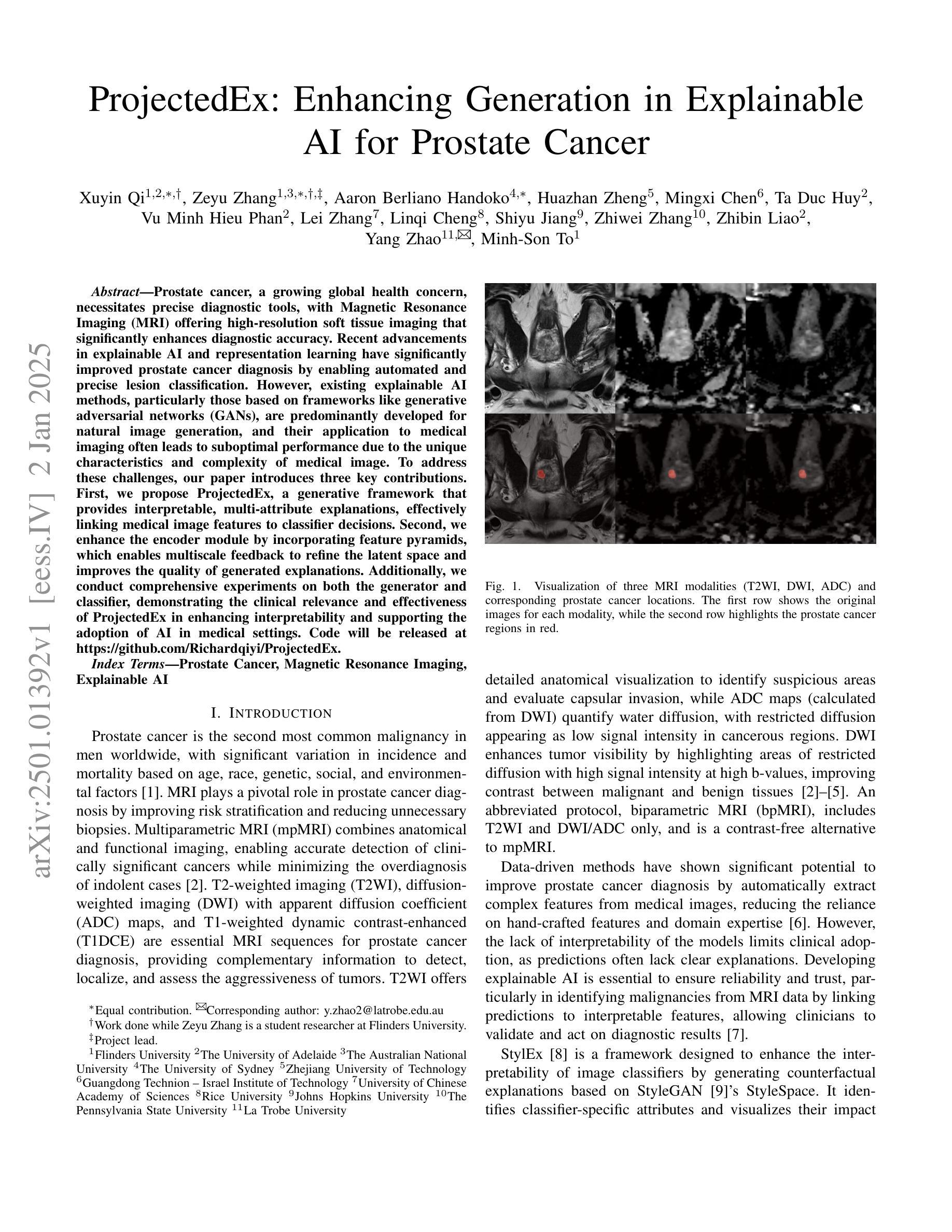
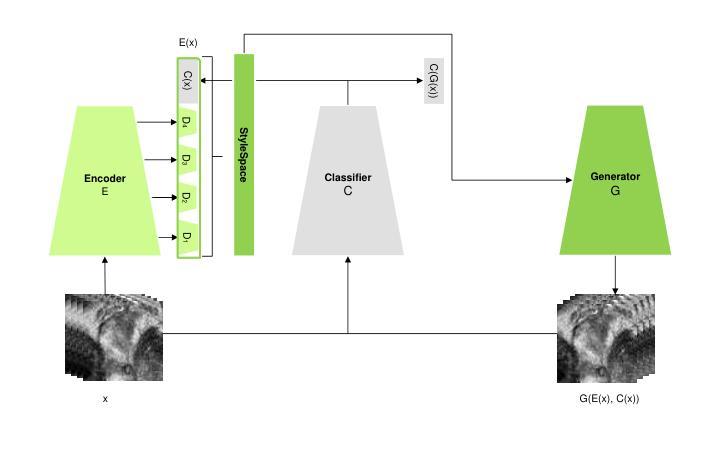
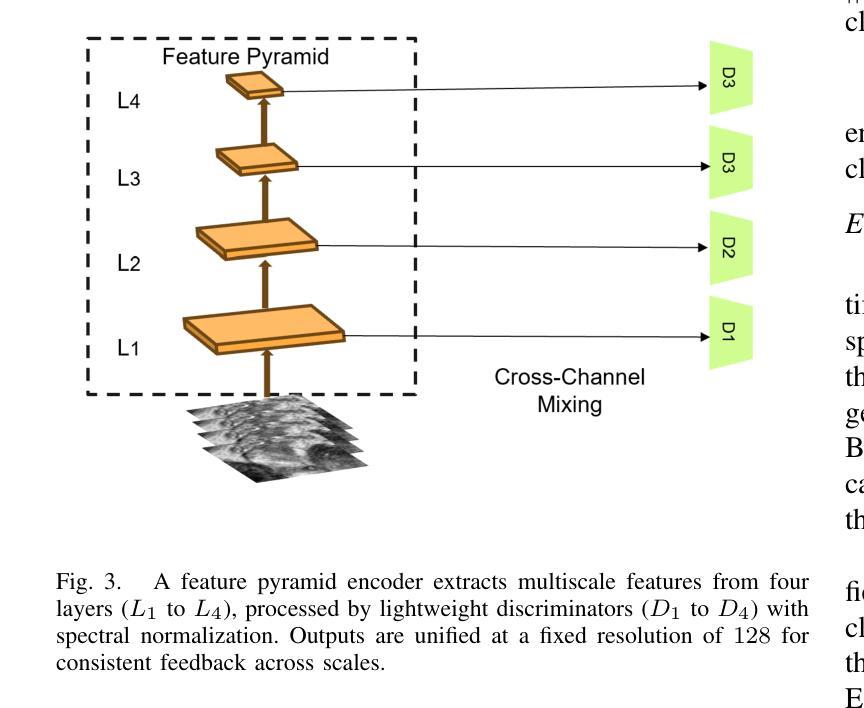
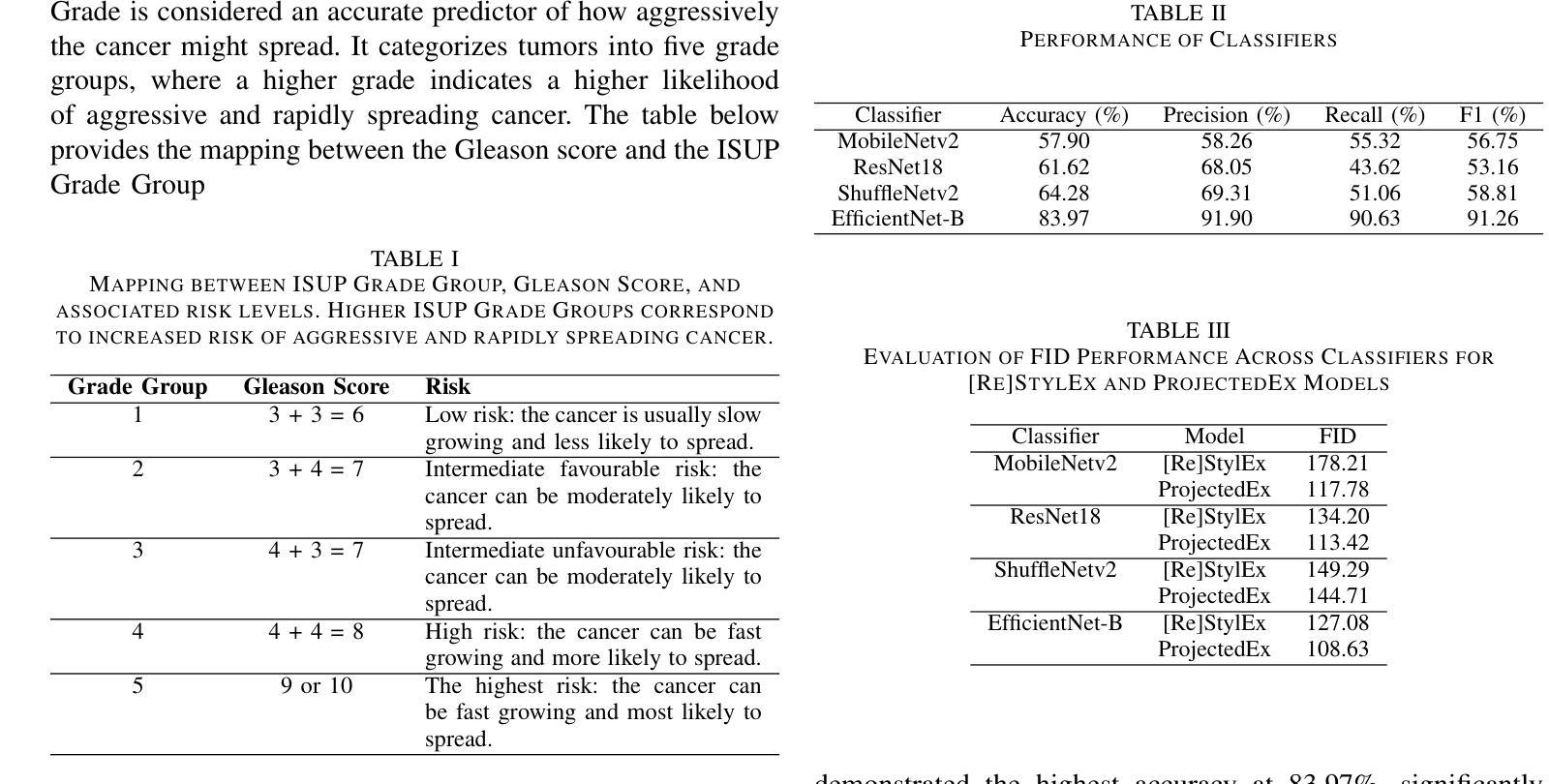
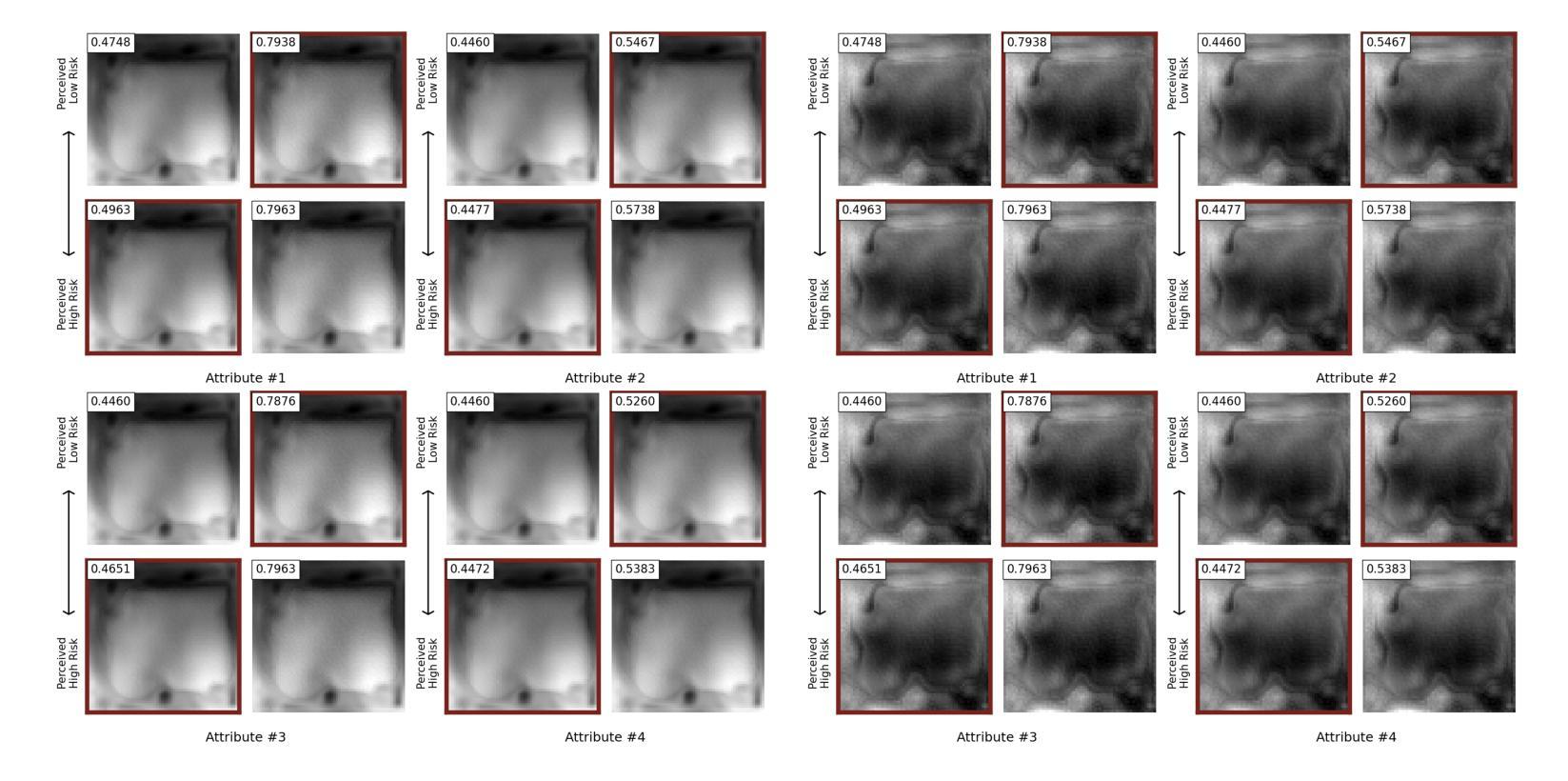
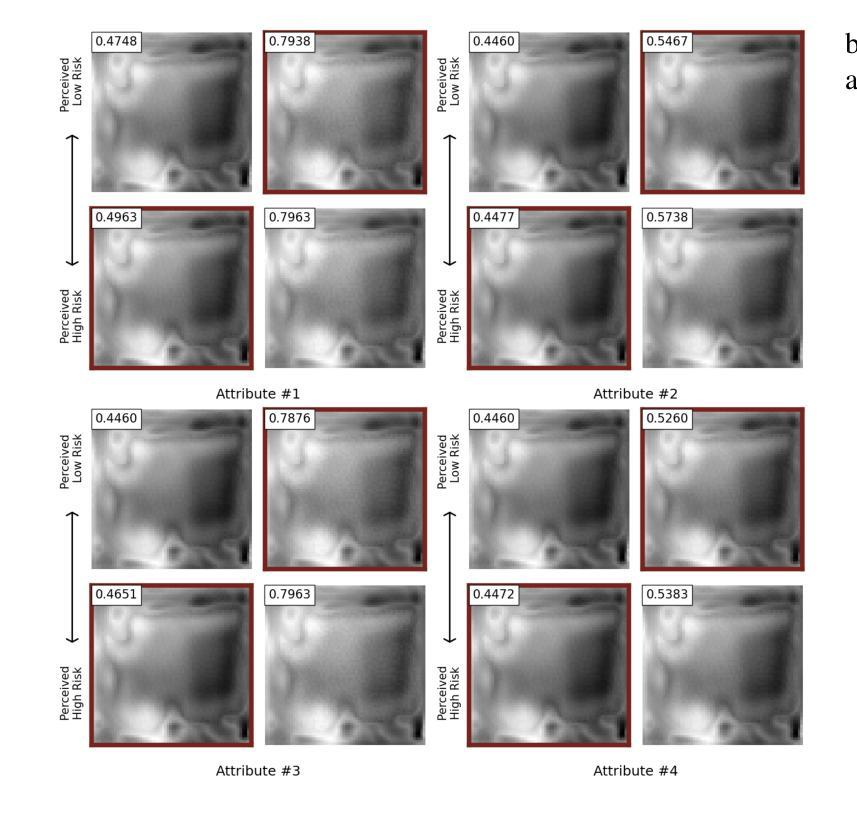
Iris Recognition for Infants
Authors:Rasel Ahmed Bhuiyan, Mateusz Trokielewicz, Piotr Maciejewicz, Sherri Bucher, Adam Czajka
Non-invasive, efficient, physical token-less, accurate and stable identification methods for newborns may prevent baby swapping at birth, limit baby abductions and improve post-natal health monitoring across geographies, within the context of both the formal (i.e., hospitals) and informal (i.e., humanitarian and fragile settings) health sectors. This paper explores the feasibility of application iris recognition to build biometric identifiers for 4-6 week old infants. We (a) collected near infrared (NIR) iris images from 17 infants using a specially-designed NIR iris sensor; (b) evaluated six iris recognition methods to assess readiness of the state-of-the-art iris recognition to be applied to newborns and infants; (c) proposed a new segmentation model that correctly detects iris texture within infants iris images, and coupled it with several iris texture encoding approaches to offer, to the first of our knowledge, a fully-operational infant iris recognition system; and, (d) trained a StyleGAN-based model to synthesize iris images mimicking samples acquired from infants to deliver to the research community privacy-safe infant iris images. The proposed system, incorporating the specially-designed iris sensor and segmenter, and applied to the collected infant iris samples, achieved Equal Error Rate (EER) of 3% and Area Under ROC Curve (AUC) of 99%, compared to EER$\geq$20% and AUC$\leq$88% obtained for state of the art adult iris recognition systems. This suggests that it may be feasible to design methods that succesfully extract biometric features from infant irises.
本文探讨了将虹膜识别技术应用于构建新生儿(4-6周)生物识别技术的可行性。我们的研究内容包括:(a)使用专门设计的近红外虹膜传感器采集了来自17名新生儿的近红外虹膜图像;(b)评估了六种虹膜识别方法,以评估当前先进的虹膜识别技术应用于新生儿和婴儿的成熟度;(c)提出了一种新的分割模型,能够正确检测婴儿虹膜图像中的纹理,并与几种虹膜纹理编码方法相结合,据我们所知,首次实现了一个全面运作的婴儿虹膜识别系统;(d)训练了一种基于StyleGAN的模型,用于合成模拟从婴儿身上获取的虹膜图像样本,为学术界提供安全的婴儿虹膜图像样本。所提出的系统结合了专门设计的虹膜传感器和分割器,并应用于收集的婴儿虹膜样本,其误识率(EER)达到3%,ROC曲线下面积(AUC)达到99%,相比之下当前先进的成人虹膜识别系统的EER达到或超过20%,AUC为或低于88%。这表明设计能够从婴儿虹膜中提取生物特征的方法可能是可行的。这一技术的实际应用可能有助于预防新生儿出生时的抱错事件、减少婴儿被拐的情况并改善不同地区的产后健康监测。这对于正规(例如医院)和非正规(例如人道主义和脆弱的环境)医疗领域都有重要意义。
论文及项目相关链接
Summary
本文探索了将虹膜识别技术应用于新生儿生物识别领域,研究内容包括采集新生儿的虹膜图像、评估多种虹膜识别方法、提出新的分割模型以及合成婴儿虹膜图像等。研究结果表该明针对婴儿的虹膜识别系统具有可行性,相较于现有成人虹膜识别系统性能更佳。有望用于预防新生儿调换、减少婴儿拐带事件,并提升产后地理区域的健康监测水平。
Key Takeaways
- 论文探索了将虹膜识别技术应用于新生儿生物识别领域,旨在预防婴儿调换和减少拐带事件。
- 通过采集新生儿的虹膜图像,评估了多种虹膜识别方法的适用性。
- 提出了一种新的分割模型,能够准确检测婴儿虹膜纹理,与多种虹膜纹理编码方法相结合,构建了婴儿虹膜识别系统。
- 利用StyleGAN模型合成模拟婴儿虹膜图像,为研究领域提供隐私安全的样本。
- 设计的婴儿虹膜识别系统取得了良好的效果,Equal Error Rate(EER)达到3%,Area Under ROC Curve(AUC)达到99%。
- 与现有成人虹膜识别系统相比,婴儿虹膜识别系统的性能更佳。
点此查看论文截图
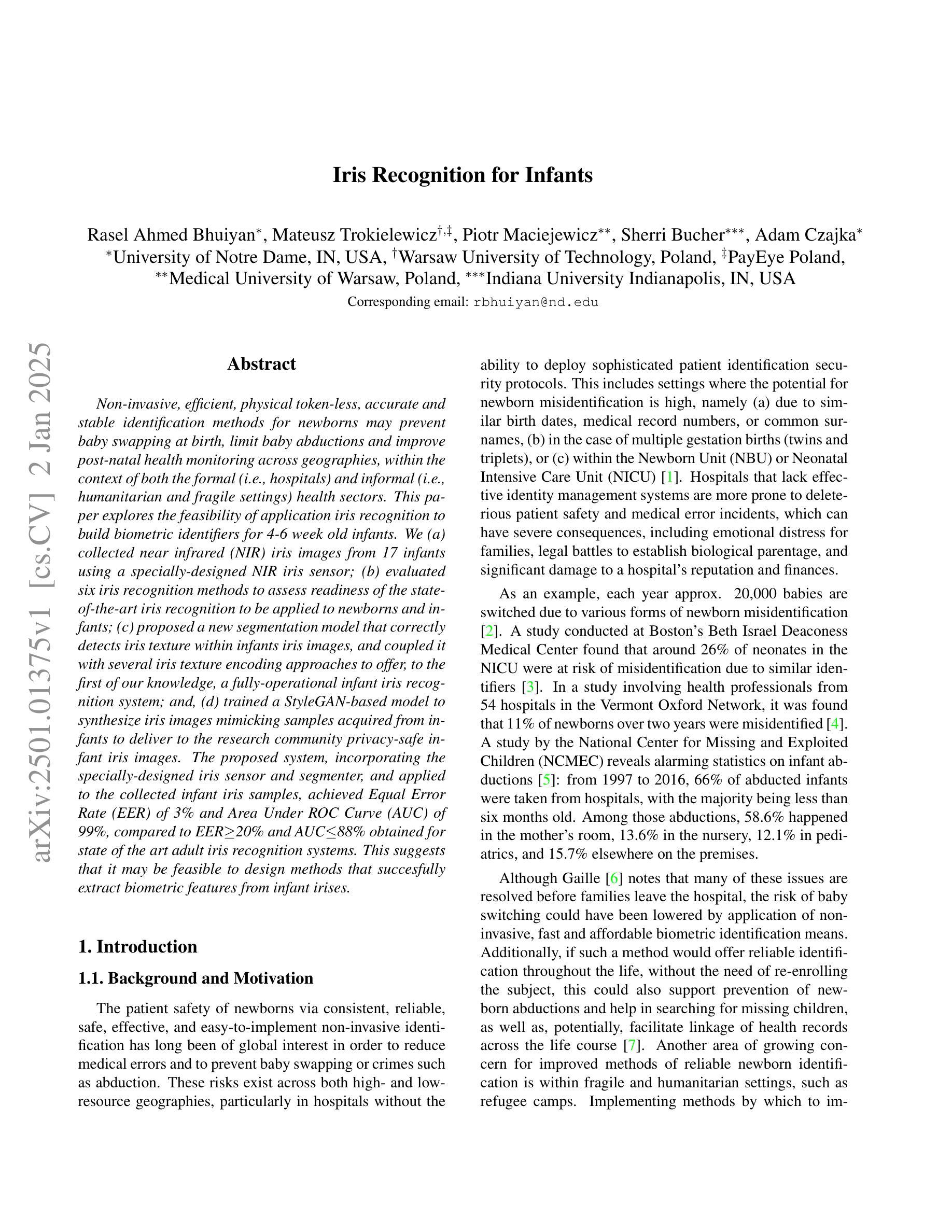
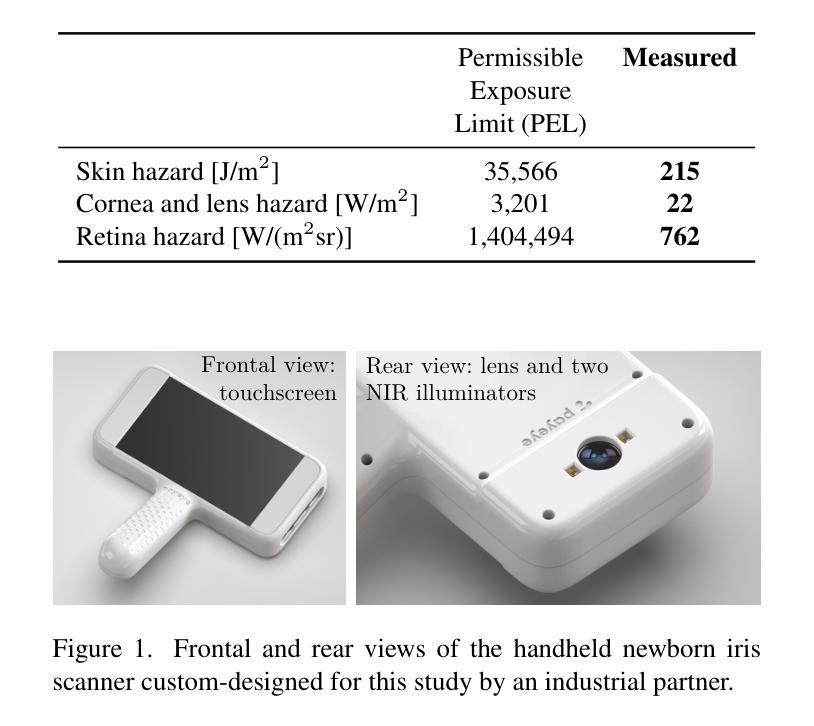
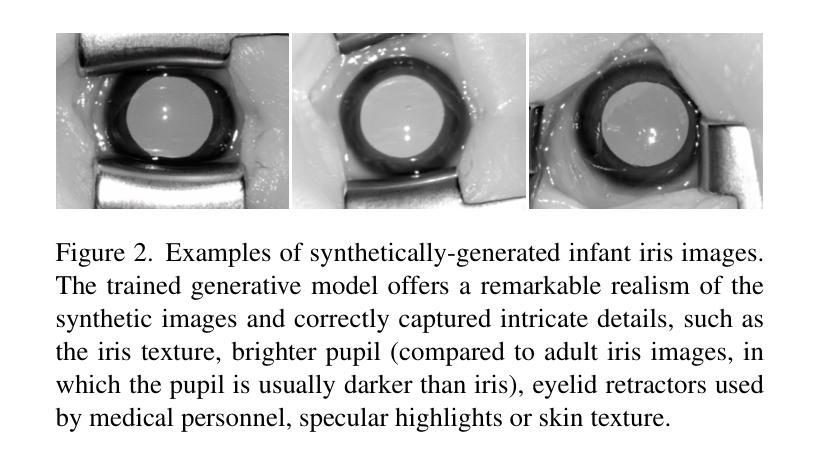
Text-to-Image GAN with Pretrained Representations
Authors:Xiaozhou You, Jian Zhang
Generating desired images conditioned on given text descriptions has received lots of attention. Recently, diffusion models and autoregressive models have demonstrated their outstanding expressivity and gradually replaced GAN as the favored architectures for text-to-image synthesis. However, they still face some obstacles: slow inference speed and expensive training costs. To achieve more powerful and faster text-to-image synthesis under complex scenes, we propose TIGER, a text-to-image GAN with pretrained representations. To be specific, we propose a vision-empowered discriminator and a high-capacity generator. (i) The vision-empowered discriminator absorbs the complex scene understanding ability and the domain generalization ability from pretrained vision models to enhance model performance. Unlike previous works, we explore stacking multiple pretrained models in our discriminator to collect multiple different representations. (ii) The high-capacity generator aims to achieve effective text-image fusion while increasing the model capacity. The high-capacity generator consists of multiple novel high-capacity fusion blocks (HFBlock). And the HFBlock contains several deep fusion modules and a global fusion module, which play different roles to benefit our model. Extensive experiments demonstrate the outstanding performance of our proposed TIGER both on standard and zero-shot text-to-image synthesis tasks. On the standard text-to-image synthesis task, TIGER achieves state-of-the-art performance on two challenging datasets, which obtain a new FID 5.48 (COCO) and 9.38 (CUB). On the zero-shot text-to-image synthesis task, we achieve comparable performance with fewer model parameters, smaller training data size and faster inference speed. Additionally, more experiments and analyses are conducted in the Supplementary Material.
基于给定的文本描述生成所需的图像已经引起了广泛关注。最近,扩散模型和自回归模型表现出了出色的表现力,并逐渐成为文本到图像合成的首选架构,逐渐取代了GAN。然而,它们仍然面临一些障碍:推理速度慢和训练成本高。为了实现更复杂场景下的更强大、更快的文本到图像合成,我们提出了TIGER,这是一种具有预训练表示的文本到图像GAN。具体来说,我们提出了一个视觉赋能判别器和一个高容量生成器。(一)视觉赋能判别器吸收了预训练视觉模型的复杂场景理解能力和领域泛化能力,以提高模型性能。与以前的工作不同,我们探索在判别器中堆叠多个预训练模型,以收集多种不同的表示。(二)高容量生成器的目标是实现有效的文本图像融合,同时增加模型容量。高容量生成器由多个新的高容量融合块(HFBlock)组成。HFBlock包含多个深度融合模块和一个全局融合模块,它们扮演着不同的角色来使我们的模型受益。大量实验表明,我们提出的TIGER在标准文本到图像合成任务和零样本文本到图像合成任务上都表现出卓越的性能。在标准文本到图像合成任务上,TIGER在两个具有挑战性的数据集上达到了最先进的性能,获得了新的FID 5.48(COCO)和9.38(CUB)。在零样本文本到图像合成任务上,我们的性能相当,但使用了更少的模型参数、更小的训练数据集和更快的推理速度。此外,更多的实验和分析将在补充材料中进行。
论文及项目相关链接
Summary
提出一种基于预训练表征的文本到图像生成对抗网络(TIGER)。包括一个视觉赋能判别器和高容量生成器。判别器从预训练视觉模型中吸收复杂场景理解能力和领域泛化能力,生成器旨在实现有效的文本图像融合并增加模型容量。在标准和非文本驱动的场景下,TIGER均展现出卓越性能。
Key Takeaways
- TIGER是一个结合预训练表征的文本到图像生成对抗网络。
- 视觉赋能判别器利用预训练视觉模型来提升复杂场景的理解和泛化能力。
- 高容量生成器旨在实现文本与图像的有效融合,同时增加模型容量。
- TIGER在标准和非文本驱动的场景下的文本到图像合成任务中均表现出卓越性能。
- 在COCO和CUB数据集上,TIGER实现了最先进的性能,新的FID分别为5.48和9.38。
- 在零样本文本到图像合成任务中,TIGER在模型参数、训练数据大小和推理速度方面表现出优势。
点此查看论文截图
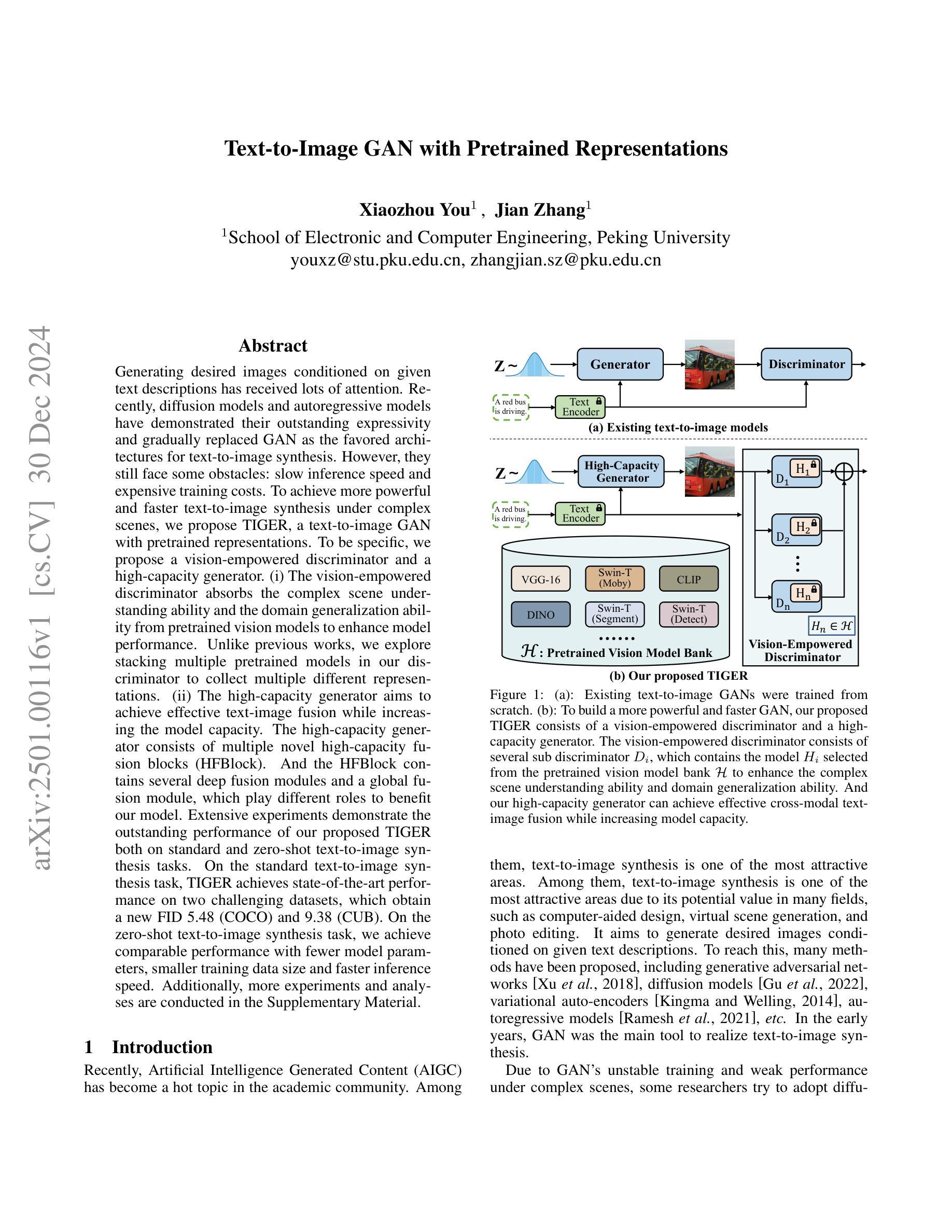
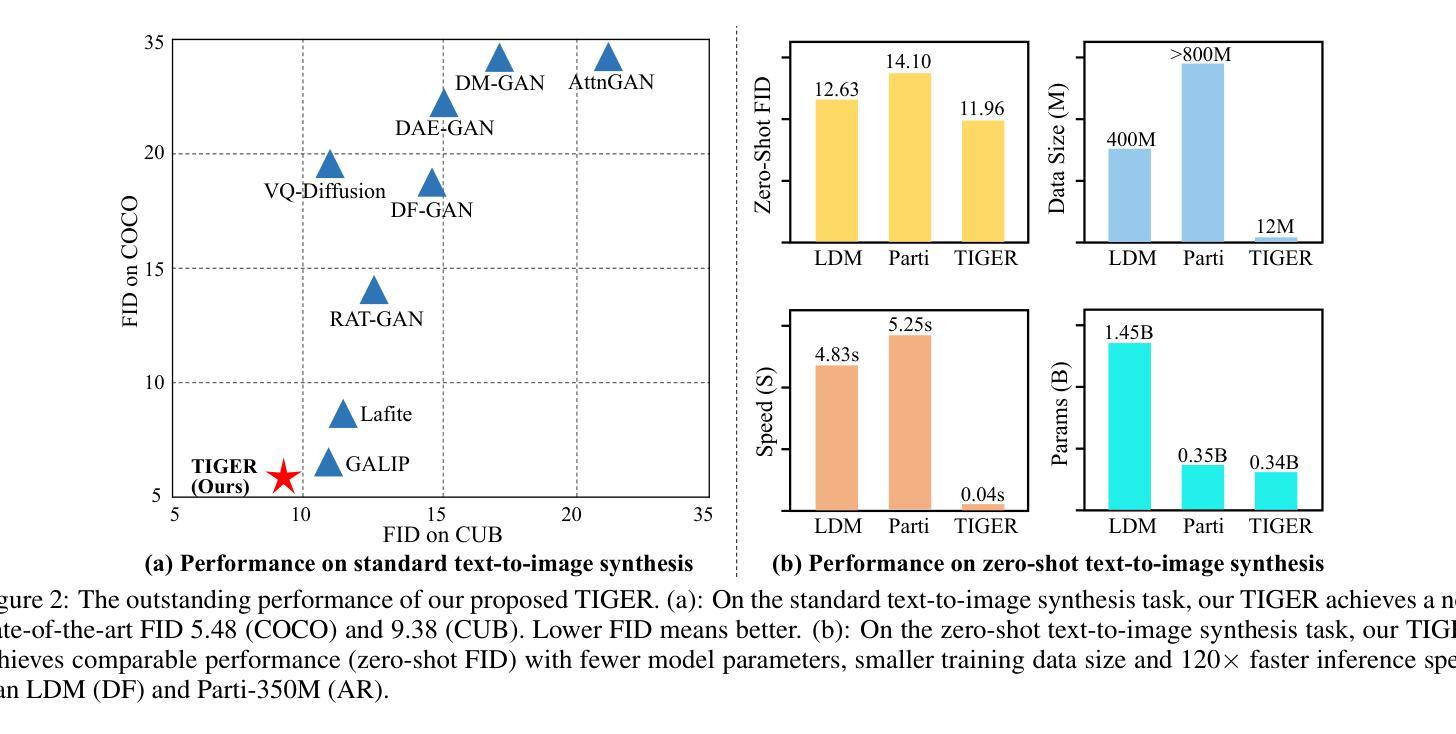
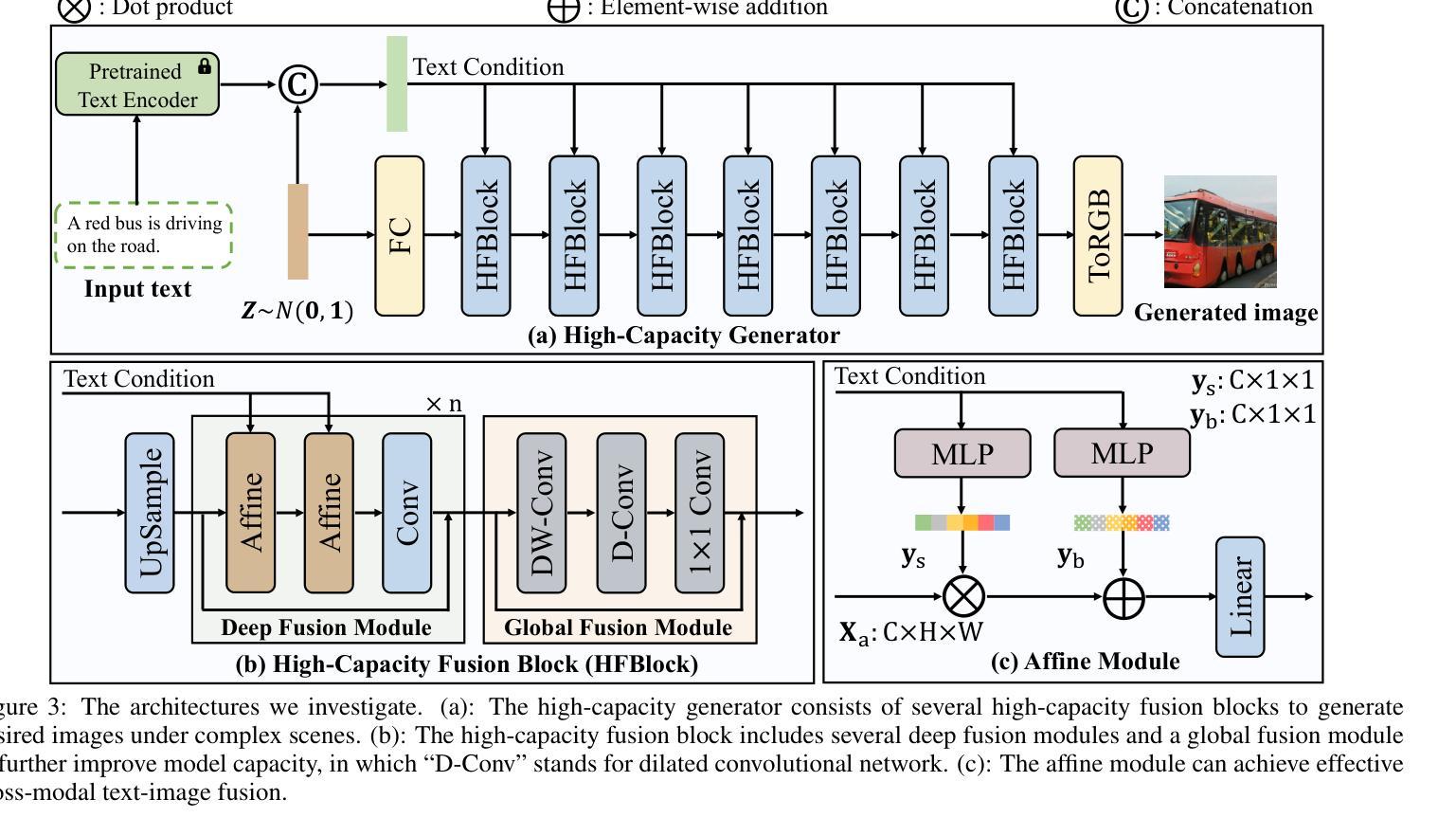
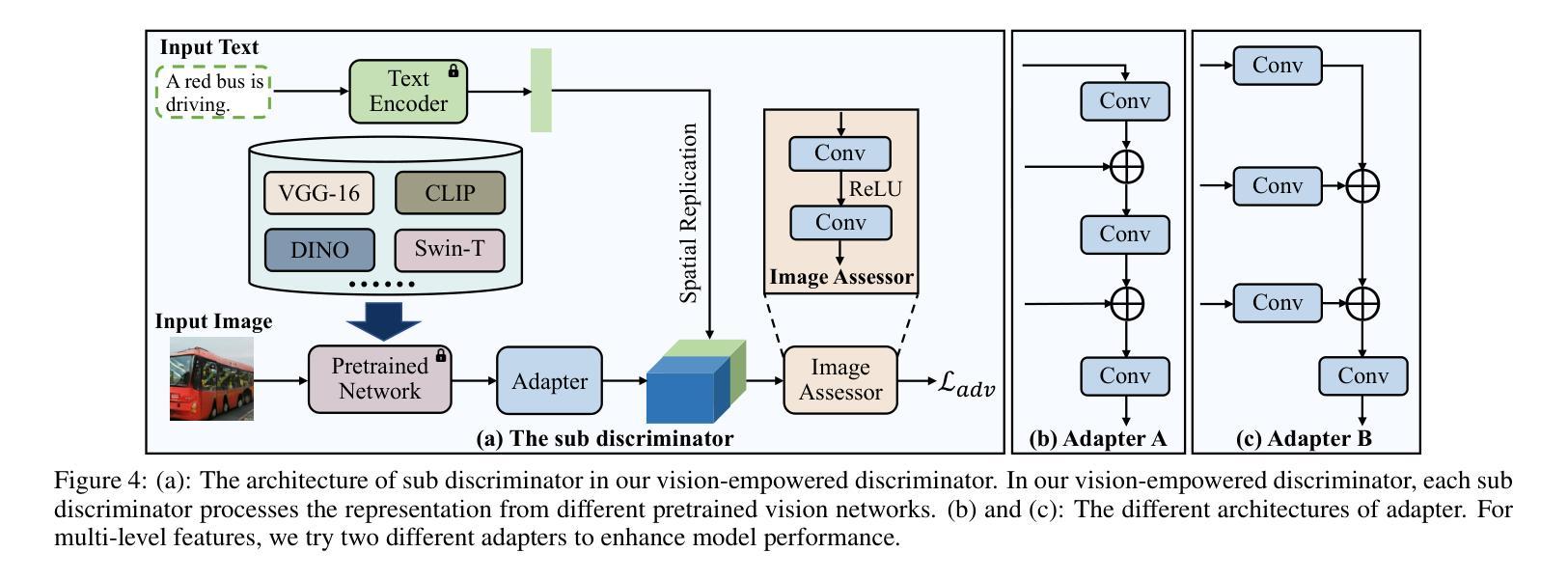
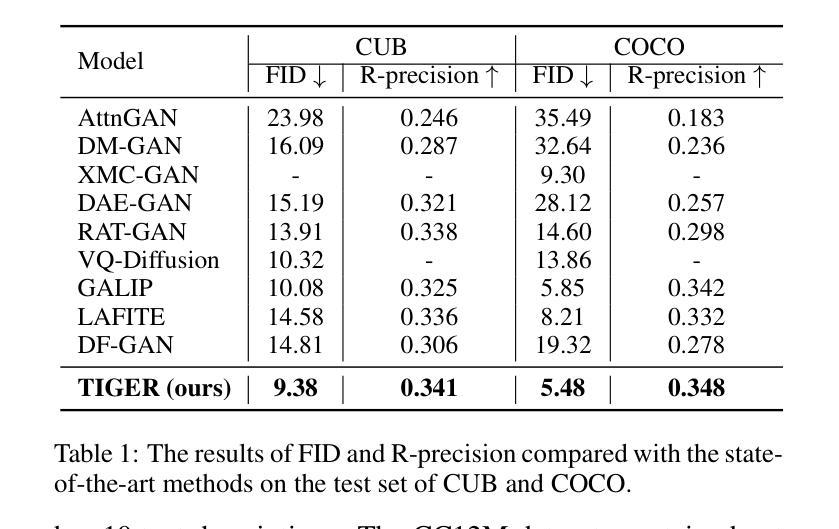
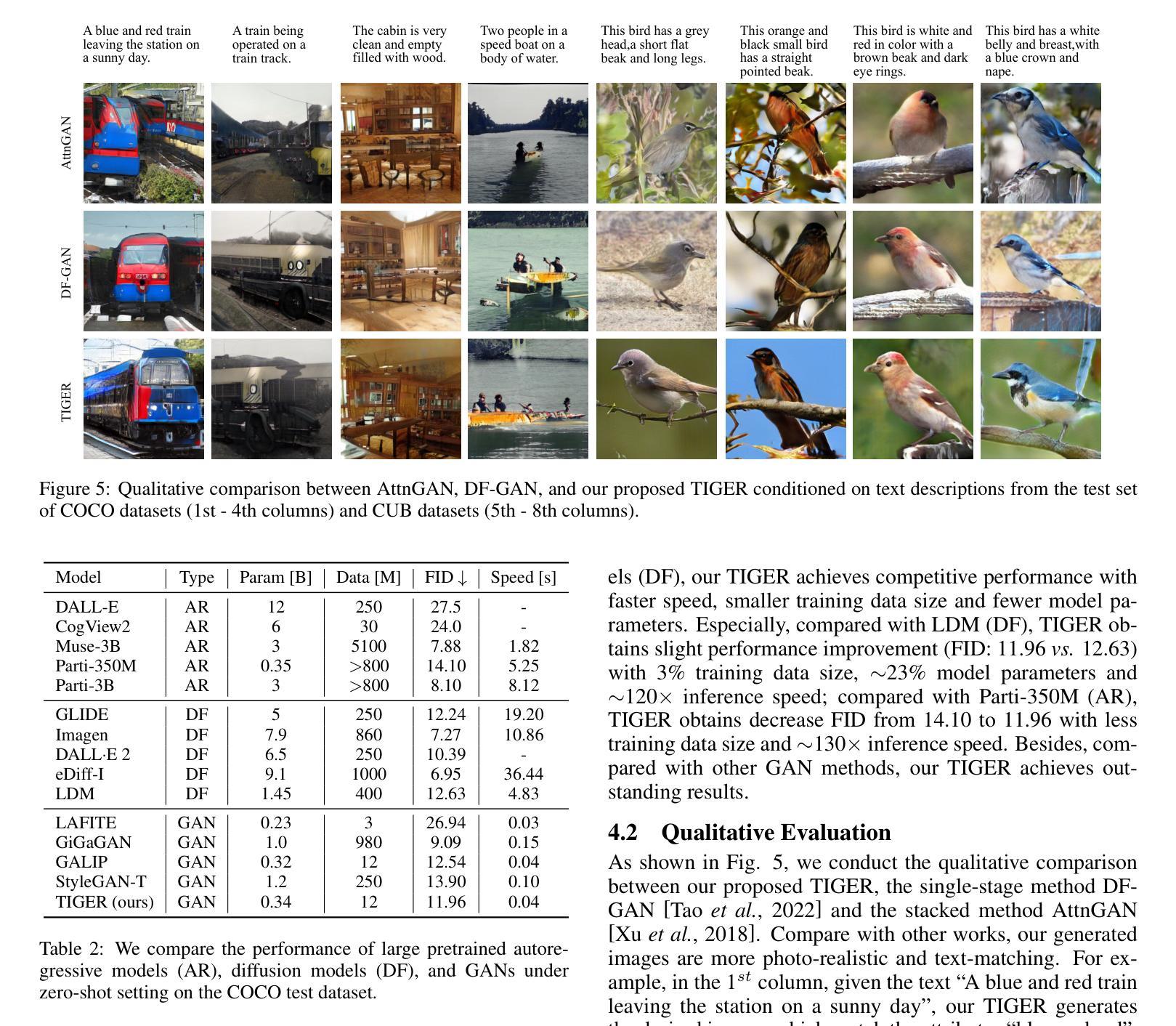