⚠️ 以下所有内容总结都来自于 大语言模型的能力,如有错误,仅供参考,谨慎使用
🔴 请注意:千万不要用于严肃的学术场景,只能用于论文阅读前的初筛!
💗 如果您觉得我们的项目对您有帮助 ChatPaperFree ,还请您给我们一些鼓励!⭐️ HuggingFace免费体验
2025-01-06 更新
Test-time Controllable Image Generation by Explicit Spatial Constraint Enforcement
Authors:Z. Zhang, B. Liu, J. Bao, L. Chen, S. Zhu, J. Yu
Recent text-to-image generation favors various forms of spatial conditions, e.g., masks, bounding boxes, and key points. However, the majority of the prior art requires form-specific annotations to fine-tune the original model, leading to poor test-time generalizability. Meanwhile, existing training-free methods work well only with simplified prompts and spatial conditions. In this work, we propose a novel yet generic test-time controllable generation method that aims at natural text prompts and complex conditions. Specifically, we decouple spatial conditions into semantic and geometric conditions and then enforce their consistency during the image-generation process individually. As for the former, we target bridging the gap between the semantic condition and text prompts, as well as the gap between such condition and the attention map from diffusion models. To achieve this, we propose to first complete the prompt w.r.t. semantic condition, and then remove the negative impact of distracting prompt words by measuring their statistics in attention maps as well as distances in word space w.r.t. this condition. To further cope with the complex geometric conditions, we introduce a geometric transform module, in which Region-of-Interests will be identified in attention maps and further used to translate category-wise latents w.r.t. geometric condition. More importantly, we propose a diffusion-based latents-refill method to explicitly remove the impact of latents at the RoI, reducing the artifacts on generated images. Experiments on Coco-stuff dataset showcase 30$%$ relative boost compared to SOTA training-free methods on layout consistency evaluation metrics.
近期文本到图像生成更加偏向于各种空间条件的形式,例如掩膜、边界框和关键点。然而,大多数先前技术需要特定形式的标注来微调原始模型,导致测试时通用性较差。同时,现有的无训练方法仅对简单的提示和空间条件有效。在这项工作中,我们提出了一种新型且通用的测试时可控生成方法,旨在处理自然语言文本提示和复杂条件。具体来说,我们将空间条件解耦为语义条件和几何条件,然后在图像生成过程中分别强制执行它们的一致性。针对前者,我们的目标是弥合语义条件和文本提示之间的鸿沟,以及这样的条件与扩散模型的注意力图之间的鸿沟。为了实现这一点,我们提出首先根据语义条件完成提示,然后通过测量注意力图中的统计数据和与此条件相关的词空间中的距离来消除令人分心提示词的不利影响。为了进一步应对复杂的几何条件,我们引入了一个几何变换模块,该模块将在注意力图中识别感兴趣区域,并进一步用于根据几何条件转换类别潜在变量。更重要的是,我们提出了一种基于扩散的潜在变量填充方法,以显式消除感兴趣区域内潜在变量的影响,从而减少生成图像上的伪影。在Coco-stuff数据集上的实验显示,与最新的无训练方法相比,在布局一致性评估指标上相对提升了30%。
论文及项目相关链接
Summary
本文提出了一种新型的测试时可控生成方法,该方法针对自然文本提示和复杂条件进行图像生成。通过解耦空间条件为语义和几何条件,并在图像生成过程中分别执行它们的一致性。使用语义条件完成提示并消除干扰提示词的负面影响,同时通过注意力图和词空间距离测量实现。为应对复杂的几何条件,引入几何变换模块,识别关注区域并翻译与几何条件相关的类别潜在特征。提出基于扩散的潜在特征填充方法,明确消除关注区域潜在特征的影响,减少生成图像的瑕疵。在Coco-stuff数据集上的实验显示,与最新的无训练方法相比,布局一致性评估指标相对提高了30%。
Key Takeaways
- 提出了新型的测试时可控生成方法,适用于自然文本提示和复杂条件。
- 通过解耦空间条件为语义和几何条件,提升图像生成的灵活性。
- 语义条件下完成提示,并通过注意力图和词空间距离测量消除干扰提示词的负面影响。
- 引入几何变换模块应对复杂几何条件,识别关注区域并翻译相关类别潜在特征。
- 提出基于扩散的潜在特征填充方法,减少生成图像的瑕疵。
- 在Coco-stuff数据集上的实验显示,该方法在布局一致性评估指标上相对提高了30%。
点此查看论文截图
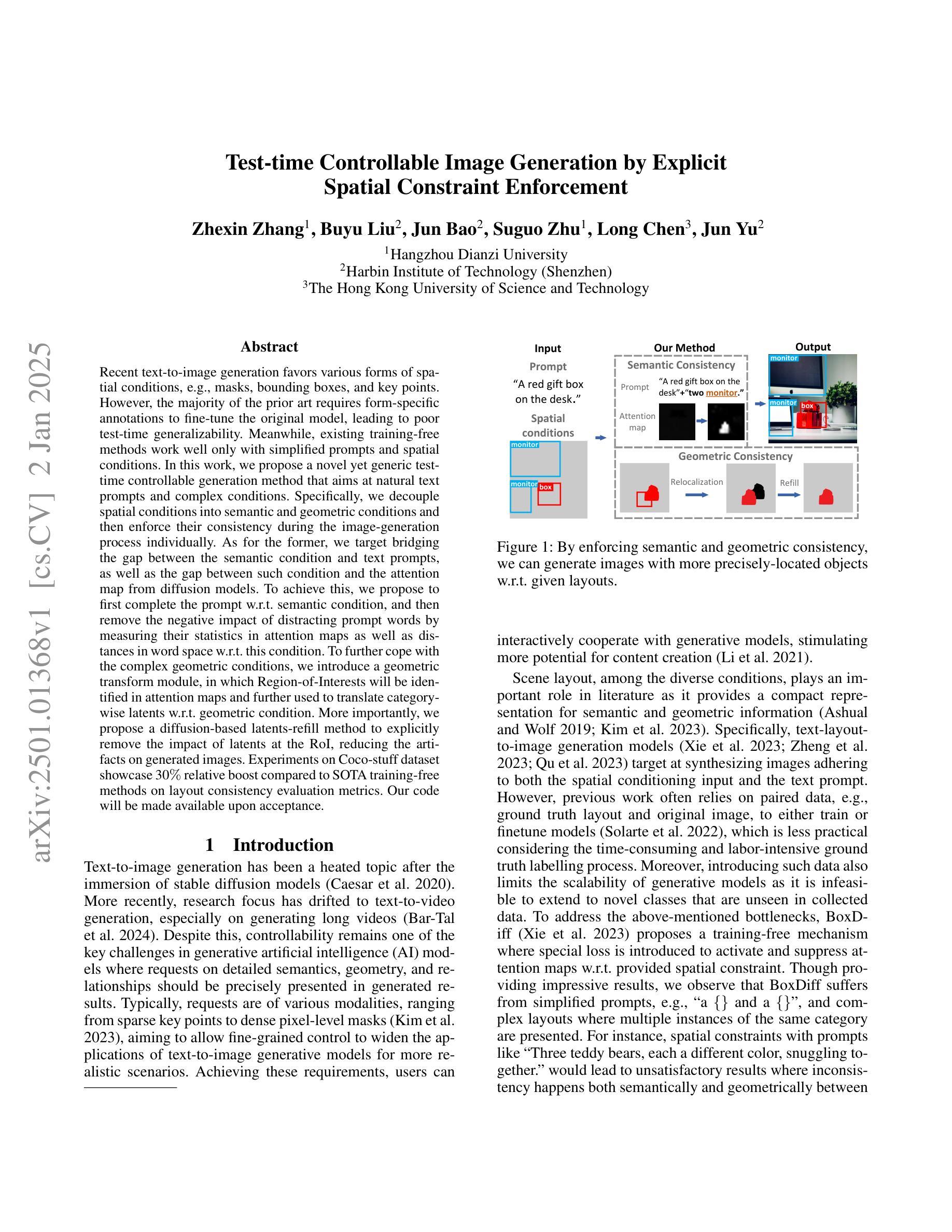
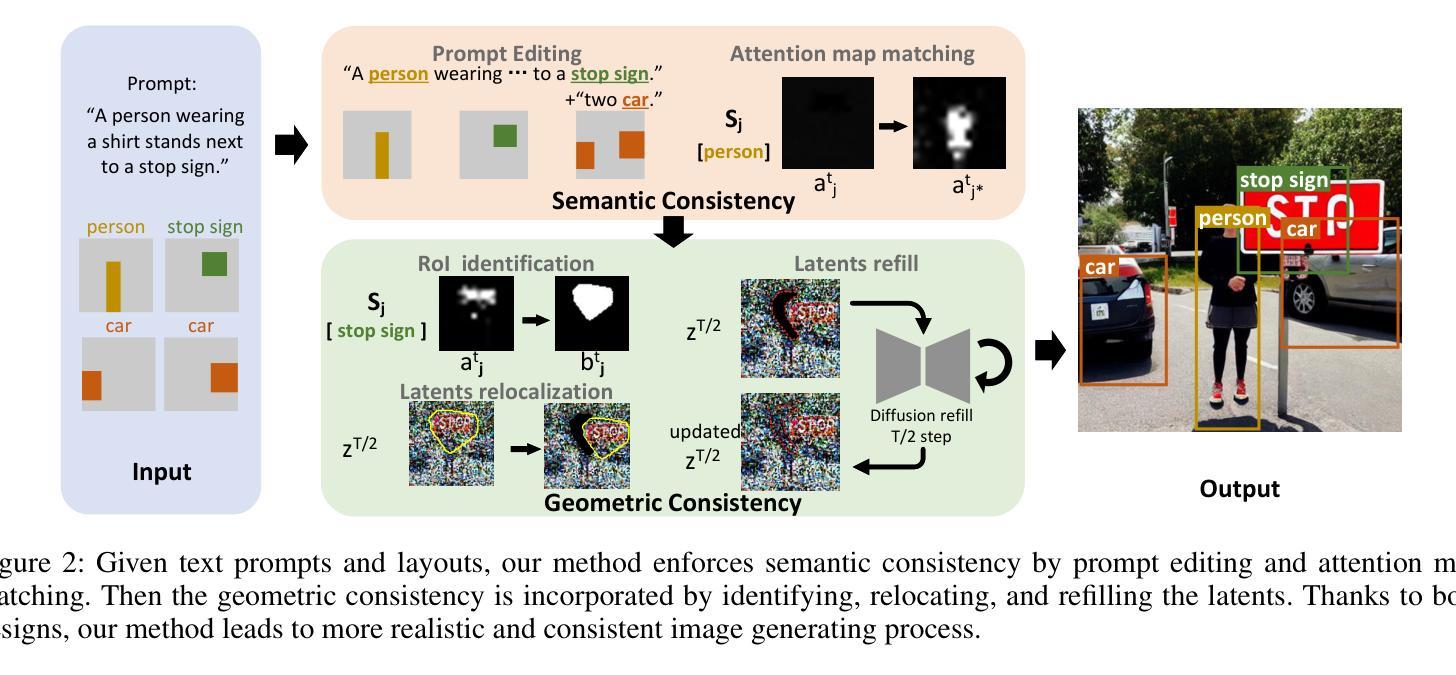
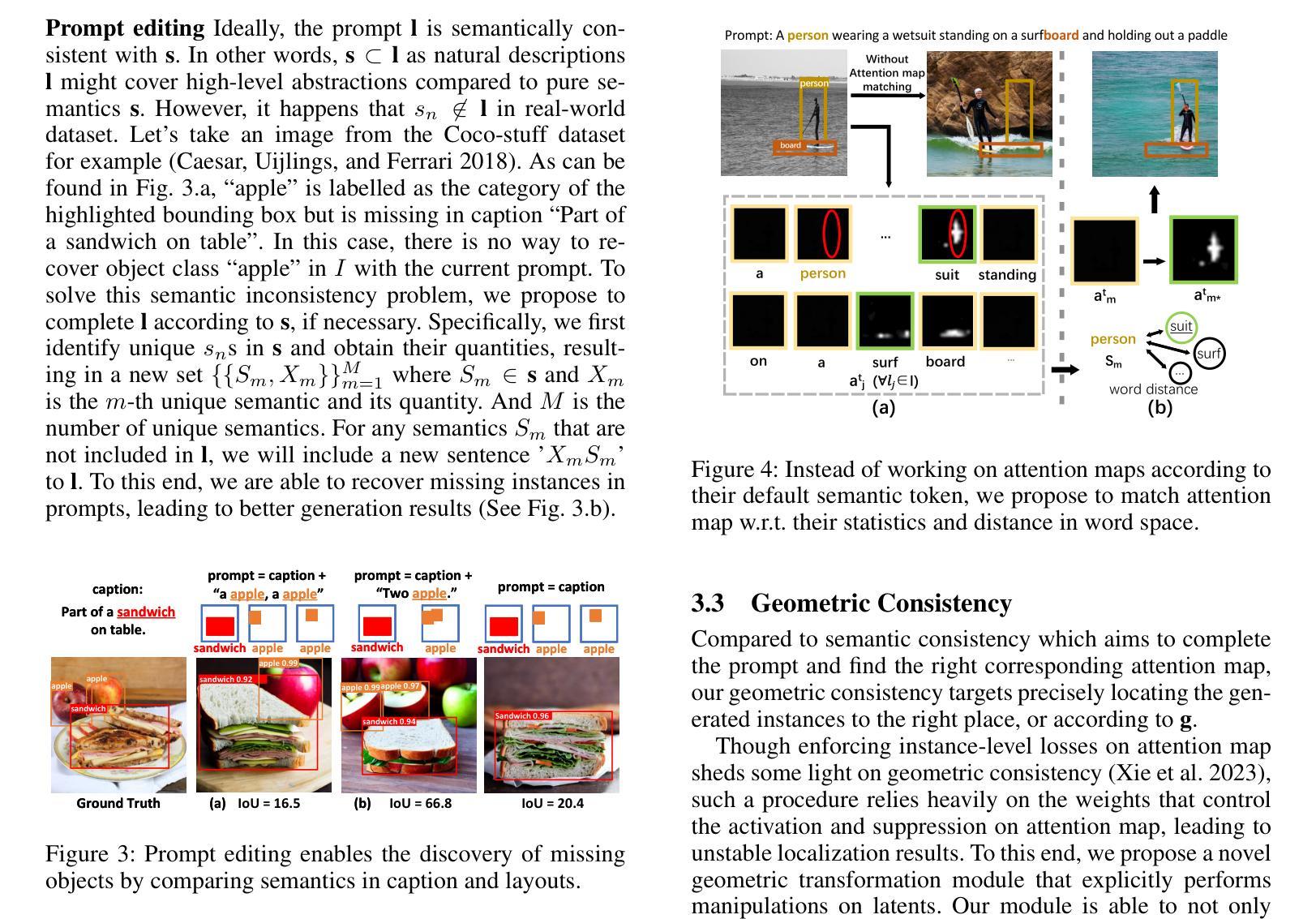
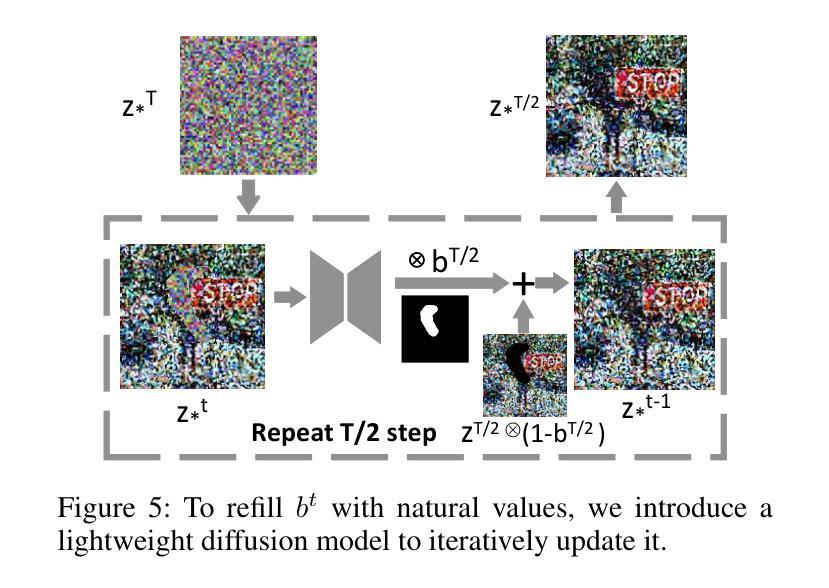
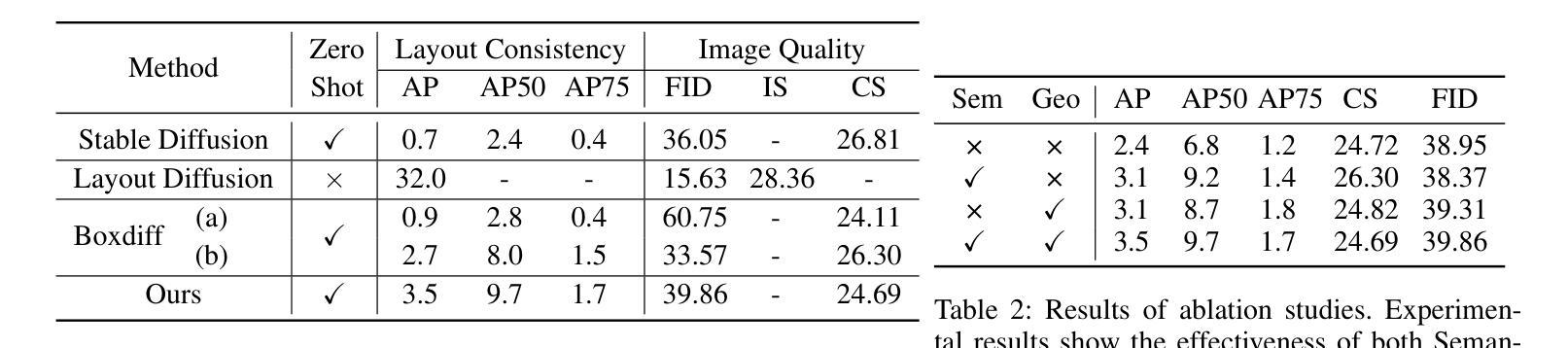
Conditional Consistency Guided Image Translation and Enhancement
Authors:A. V. Subramanyam, Amil Bhagat, Milind Jain
Consistency models have emerged as a promising alternative to diffusion models, offering high-quality generative capabilities through single-step sample generation. However, their application to multi-domain image translation tasks, such as cross-modal translation and low-light image enhancement remains largely unexplored. In this paper, we introduce Conditional Consistency Models (CCMs) for multi-domain image translation by incorporating additional conditional inputs. We implement these modifications by introducing task-specific conditional inputs that guide the denoising process, ensuring that the generated outputs retain structural and contextual information from the corresponding input domain. We evaluate CCMs on 10 different datasets demonstrating their effectiveness in producing high-quality translated images across multiple domains. Code is available at https://github.com/amilbhagat/Conditional-Consistency-Models.
一致性模型已成为扩散模型的一个前景可观的替代方案,它通过单步采样生成提供高质量生成能力。然而,它们在多域图像翻译任务(如跨模态翻译和低光图像增强)中的应用仍然很少被探索。在本文中,我们通过引入额外的条件输入,引入了用于多域图像翻译的条件一致性模型(CCM)。我们通过引入任务特定的条件输入来实现这些修改,指导去噪过程,确保生成的输出保留来自相应输入域的结构和上下文信息。我们在10个不同的数据集上评估了CCM,证明了它们在多个领域生成高质量翻译图像的有效性。代码可在https://github.com/amilbhagat/Conditional-Consistency-Models获得。
论文及项目相关链接
PDF 6 pages, 5 figures, 4 tables, ICME conference 2025
Summary
该研究提出了一种新的模型——条件一致性模型(CCM),旨在通过引入条件输入来解决多领域图像翻译问题。该模型能够在单步采样生成中实现高质量图像翻译,且在多个数据集上的表现得到了验证。模型可以确保生成的输出保留输入领域的结构和上下文信息。相关代码已公开在GitHub上。
Key Takeaways
- 条件一致性模型(CCM)被提出用于多领域图像翻译,通过引入额外的条件输入实现高质量生成。
- CCM模型能够实现单步采样生成,提高了生成效率。
- CCM模型在结构保留和上下文信息保留方面表现出色,确保生成的图像与输入领域相关。
- CCM模型在多个数据集上的表现得到了验证,展现了广泛的应用前景。
- 该模型的应用范围包括跨模态翻译和低光图像增强等。
- 模型的相关代码已经公开,便于其他研究者进行进一步的研究和实验。
点此查看论文截图
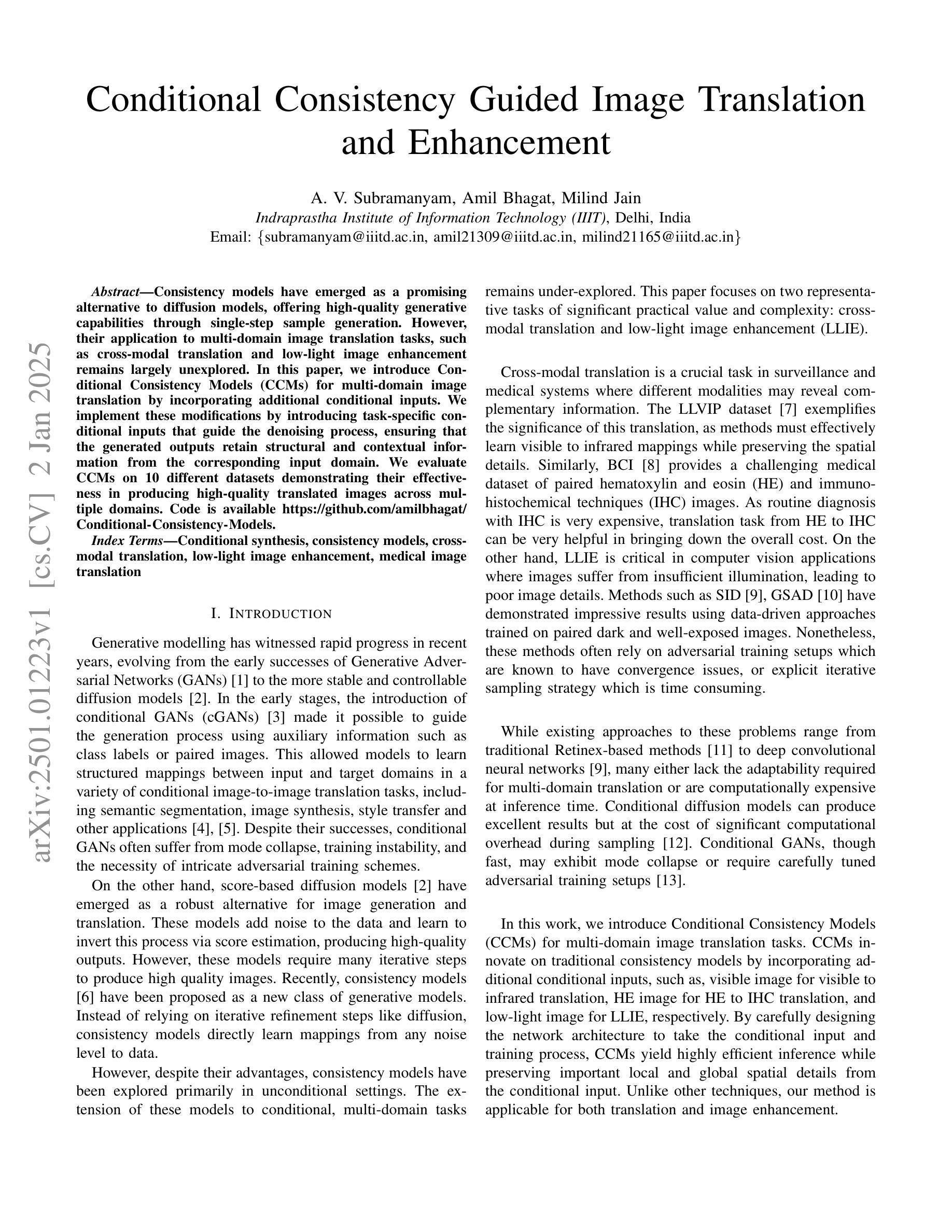
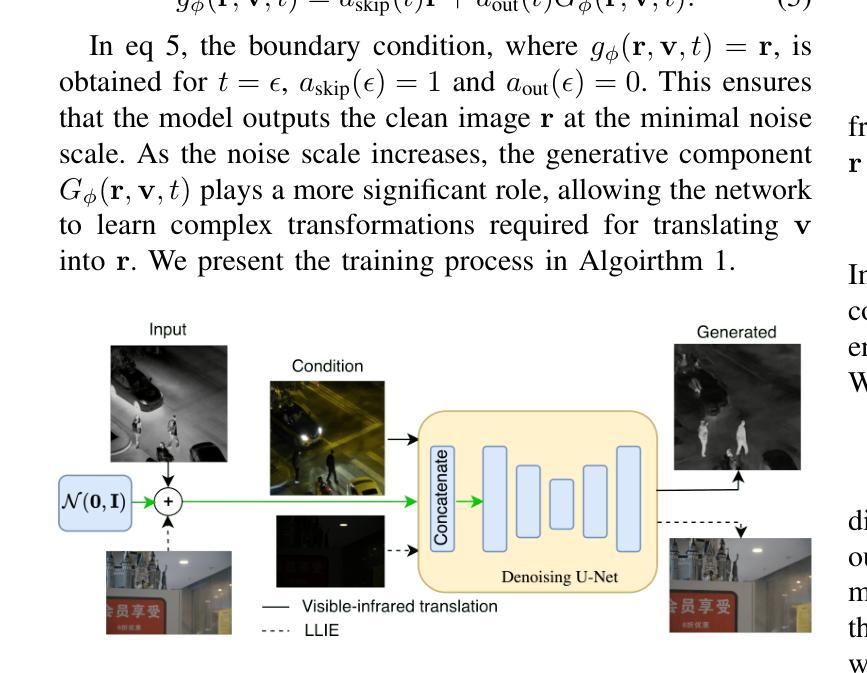
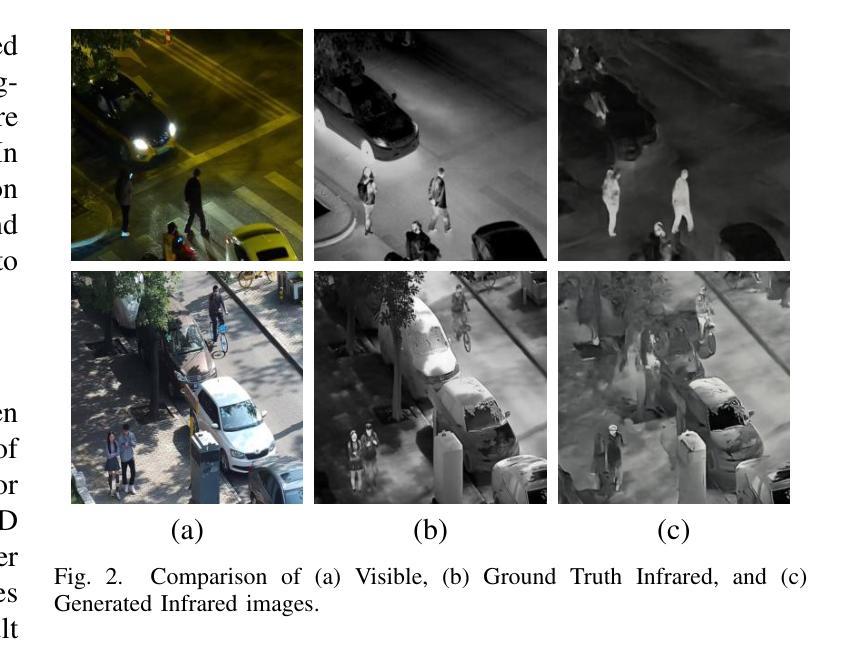
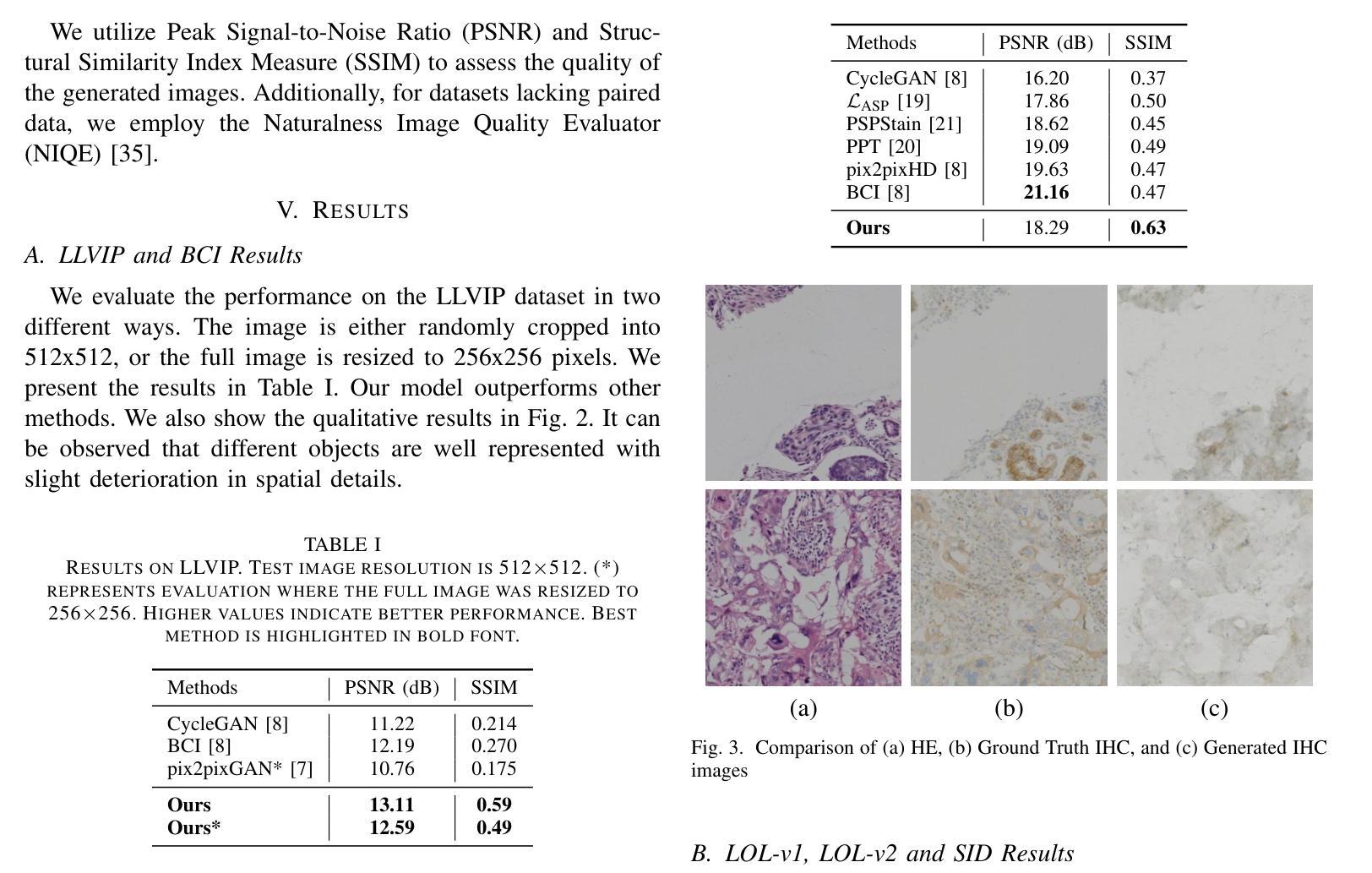
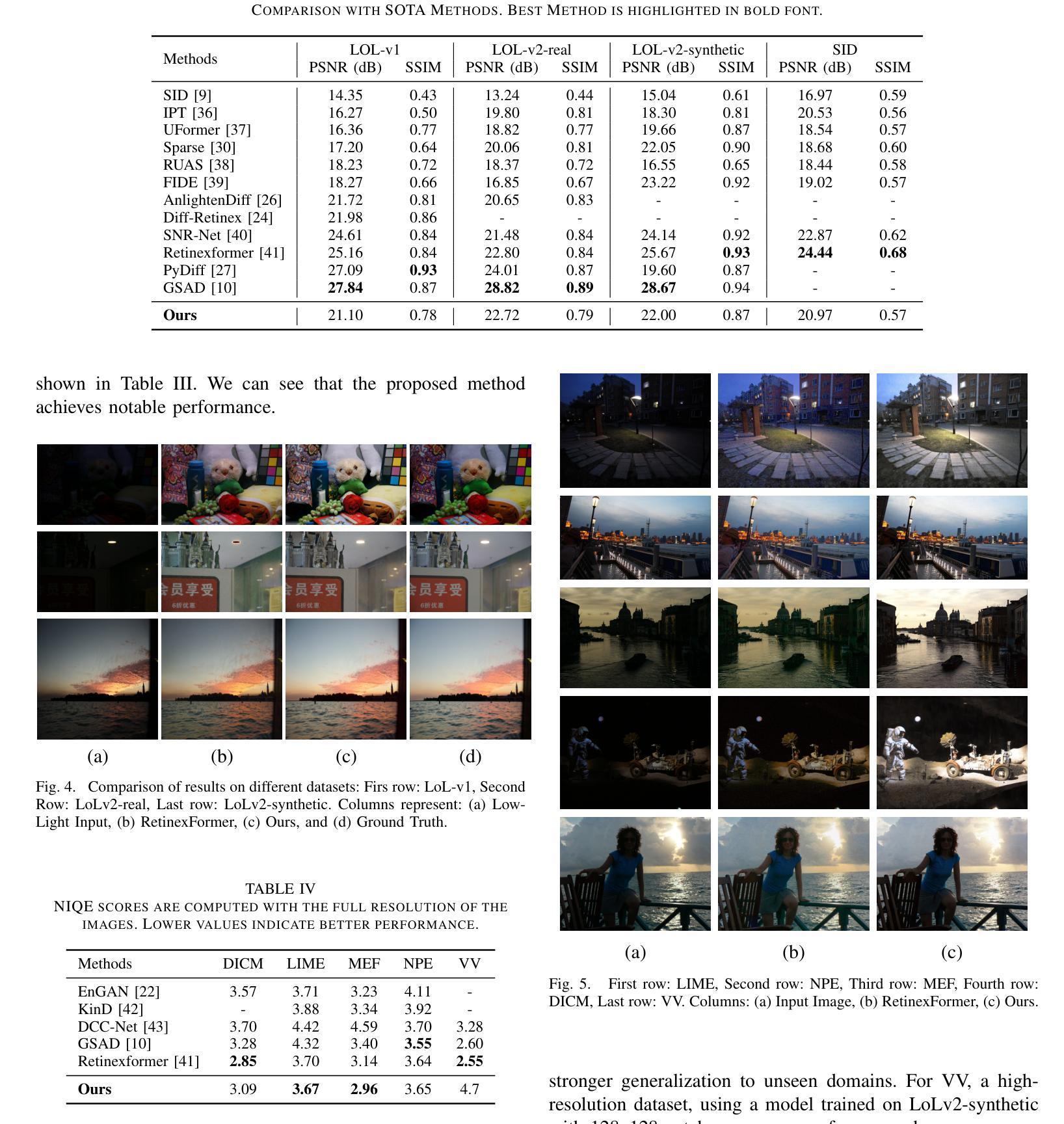