⚠️ 以下所有内容总结都来自于 大语言模型的能力,如有错误,仅供参考,谨慎使用
🔴 请注意:千万不要用于严肃的学术场景,只能用于论文阅读前的初筛!
💗 如果您觉得我们的项目对您有帮助 ChatPaperFree ,还请您给我们一些鼓励!⭐️ HuggingFace免费体验
2025-01-06 更新
VideoAnydoor: High-fidelity Video Object Insertion with Precise Motion Control
Authors:Yuanpeng Tu, Hao Luo, Xi Chen, Sihui Ji, Xiang Bai, Hengshuang Zhao
Despite significant advancements in video generation, inserting a given object into videos remains a challenging task. The difficulty lies in preserving the appearance details of the reference object and accurately modeling coherent motions at the same time. In this paper, we propose VideoAnydoor, a zero-shot video object insertion framework with high-fidelity detail preservation and precise motion control. Starting from a text-to-video model, we utilize an ID extractor to inject the global identity and leverage a box sequence to control the overall motion. To preserve the detailed appearance and meanwhile support fine-grained motion control, we design a pixel warper. It takes the reference image with arbitrary key-points and the corresponding key-point trajectories as inputs. It warps the pixel details according to the trajectories and fuses the warped features with the diffusion U-Net, thus improving detail preservation and supporting users in manipulating the motion trajectories. In addition, we propose a training strategy involving both videos and static images with a reweight reconstruction loss to enhance insertion quality. VideoAnydoor demonstrates significant superiority over existing methods and naturally supports various downstream applications (e.g., talking head generation, video virtual try-on, multi-region editing) without task-specific fine-tuning.
尽管视频生成领域已经取得了重大进展,但在视频中插入给定对象仍然是一项具有挑战性的任务。难点在于同时保留参考对象的外貌细节并准确模拟连贯的运动。在本文中,我们提出了VideoAnydoor,一个零样本视频对象插入框架,具有高保真细节保留和精确运动控制的特点。我们从文本到视频模型出发,使用ID提取器注入全局身份,并利用盒子序列控制整体运动。为了保留细节外观的同时支持精细的运动控制,我们设计了一个像素扭曲器。它以带有任意关键点的参考图像和相应的关键点轨迹作为输入。它根据轨迹扭曲像素细节,并将扭曲的特征与扩散U-Net融合,从而改善细节保留并支持用户操作运动轨迹。此外,我们提出了一种涉及视频和静态图像的训练策略,采用加权重建损失来提高插入质量。VideoAnydoor相较于现有方法表现出显著的优势,无需特定任务的微调即可自然支持各种下游应用(例如谈话头生成、视频虚拟试穿、多区域编辑)。
论文及项目相关链接
PDF Method for object insertion in videos
Summary
本文提出了一种名为VideoAnydoor的零样本视频物体插入框架,具有高度的细节保真度和精确的运动控制。该框架以文本转视频模型为基础,通过ID提取器注入全局身份并利用序列框控制整体运动。为保留详细外观并支持精细运动控制,设计了一个像素扭曲器,能够根据轨迹扭曲像素细节并与扩散U-Net融合。此外,提出了一种涉及视频和静态图像的训练策略,使用重新加权重建损失以提高插入质量。VideoAnydoor相较于现有方法具有显著优势,并自然支持各种下游应用,如说话人头部生成、视频虚拟试穿、多区域编辑等,无需特定任务微调。
Key Takeaways
- VideoAnydoor是一个零样本视频物体插入框架,具有细节保真度和精确运动控制。
- 框架基于文本转视频模型,通过ID提取器注入全局身份。
- 像素扭曲器设计用于保留详细外观并支持精细运动控制,根据轨迹扭曲像素细节。
- 框架利用序列框控制整体运动,并与扩散U-Net融合以提升性能。
- 训练策略涉及视频和静态图像,采用重新加权重建损失以提高插入质量。
- VideoAnydoor显著优于现有方法,支持多种下游应用,如说话人头部生成、视频虚拟试穿、多区域编辑。
点此查看论文截图
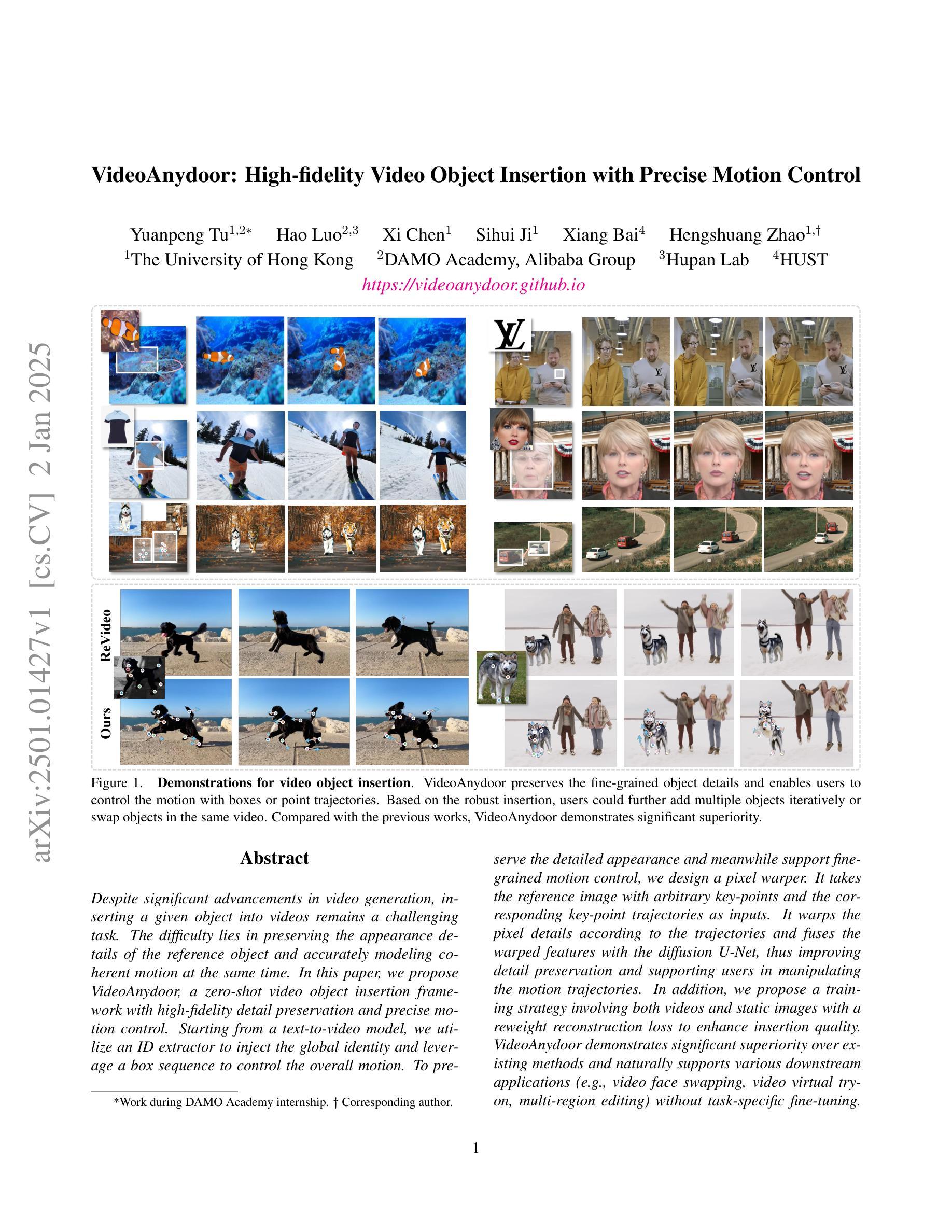
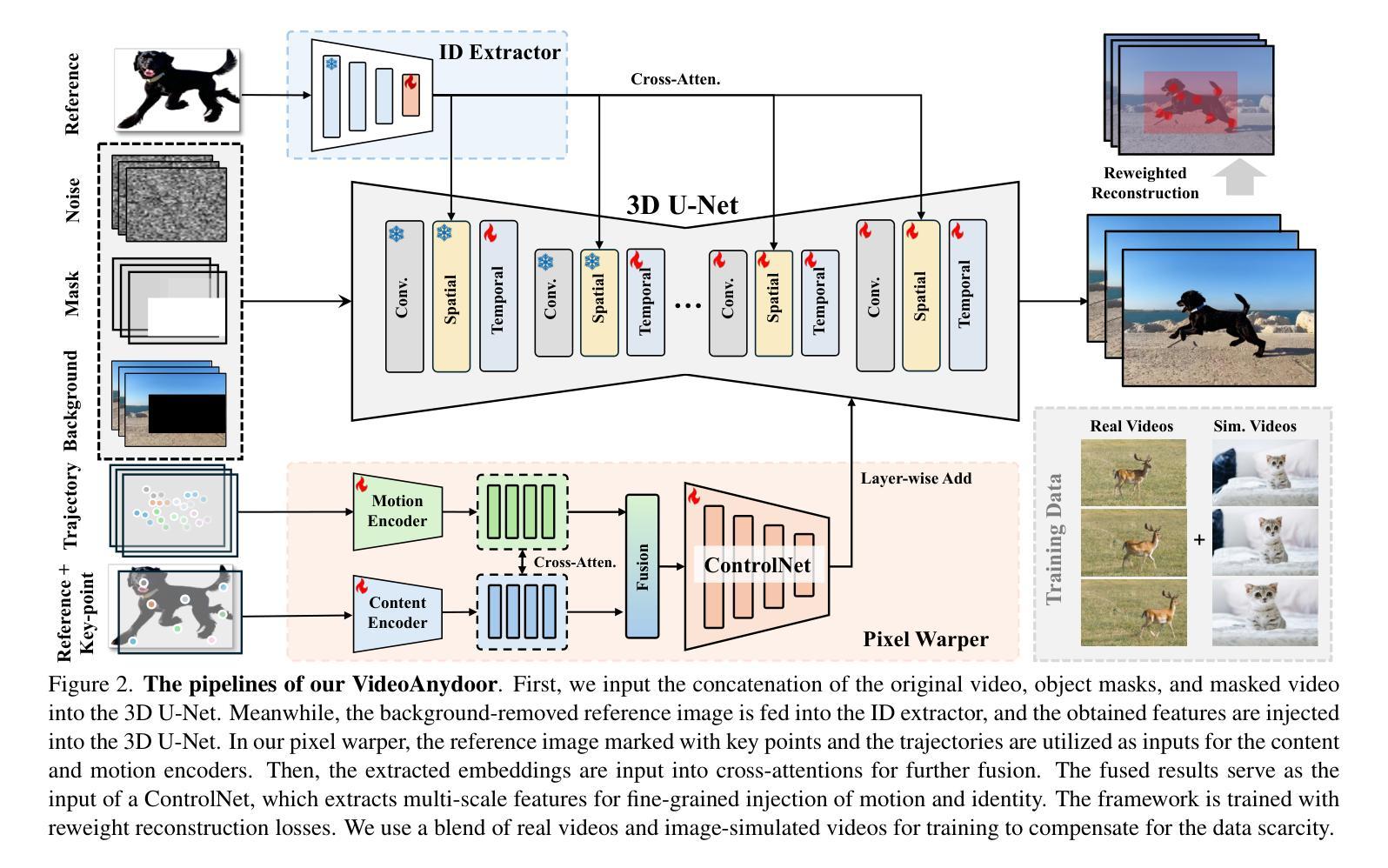
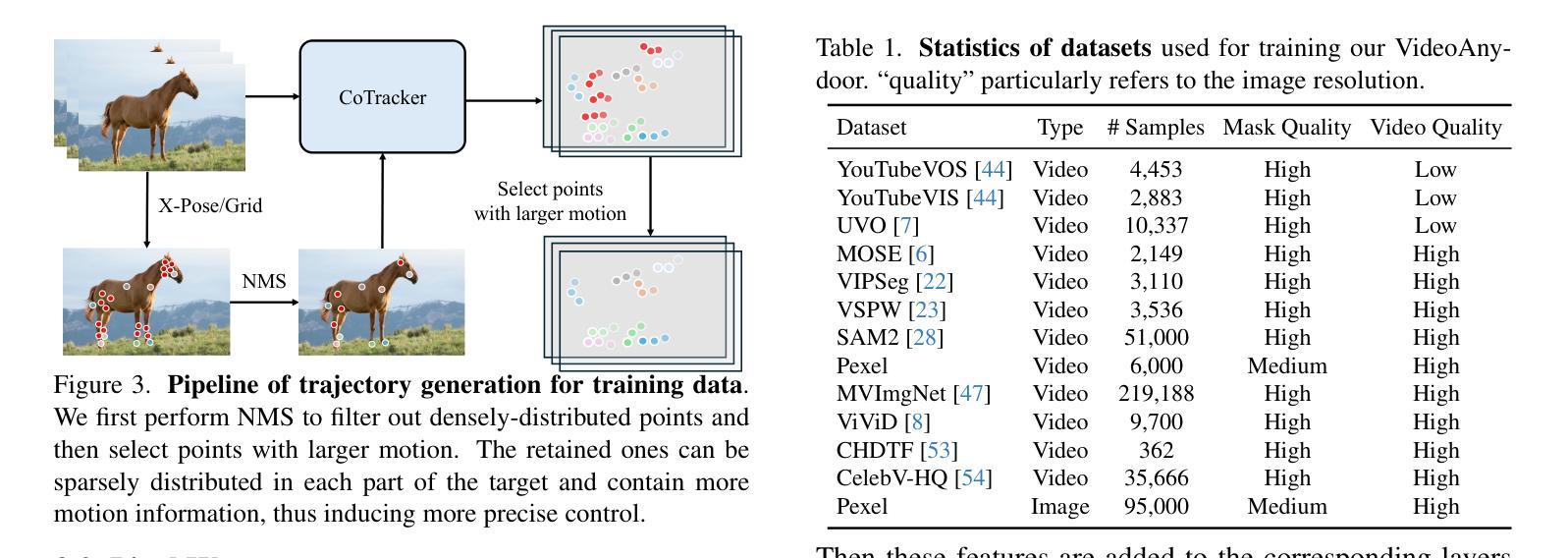
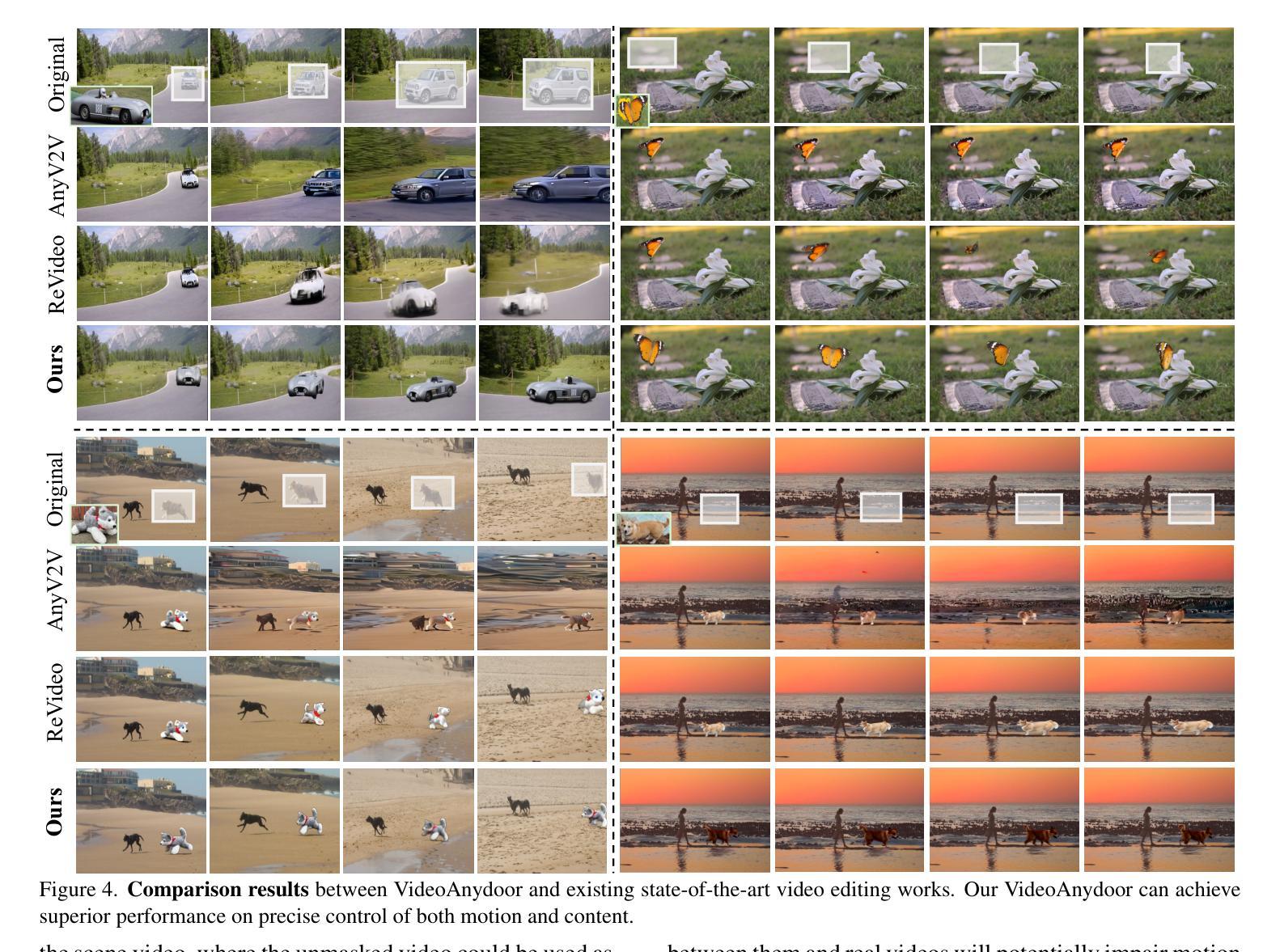
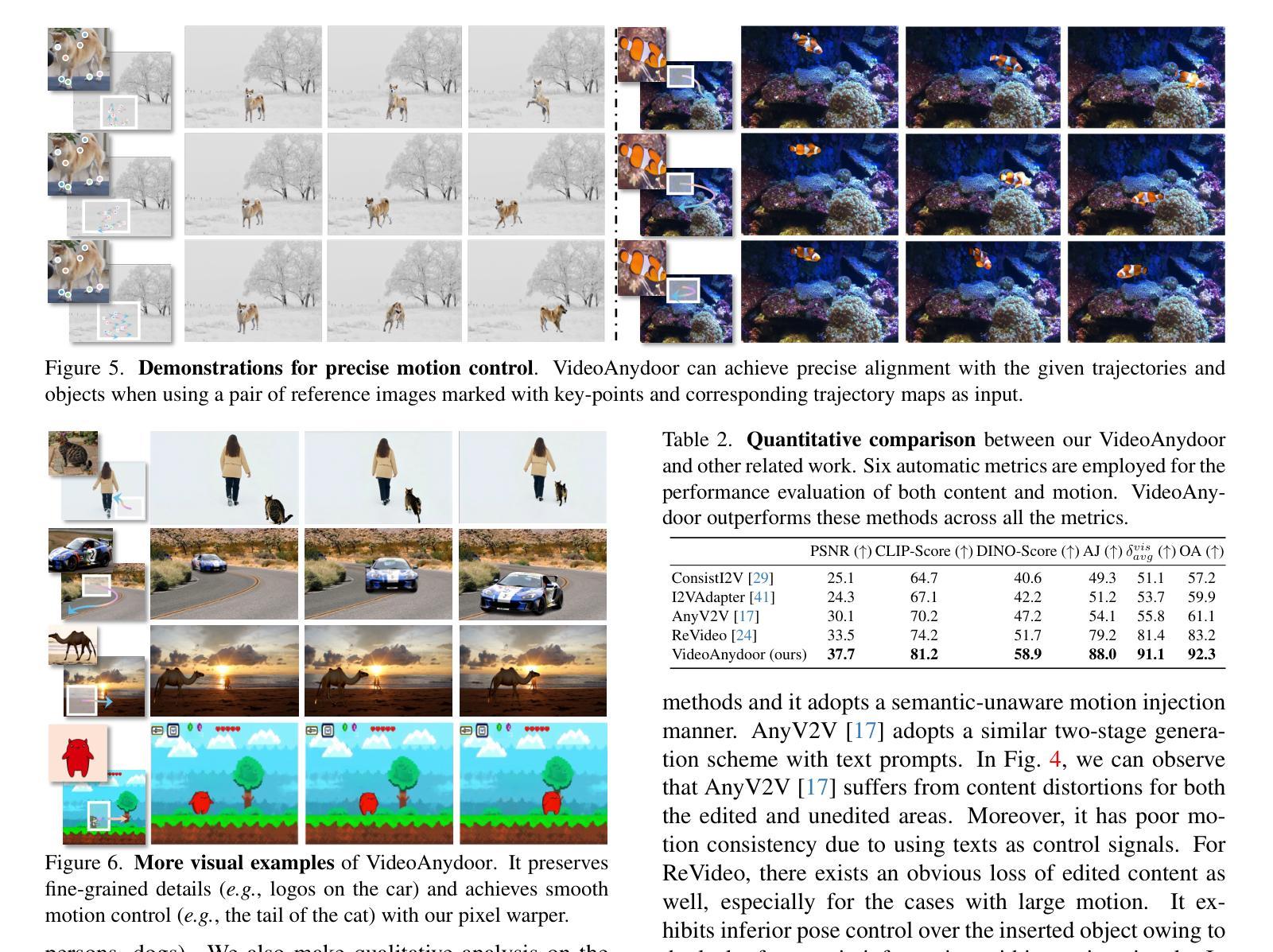