⚠️ 以下所有内容总结都来自于 大语言模型的能力,如有错误,仅供参考,谨慎使用
🔴 请注意:千万不要用于严肃的学术场景,只能用于论文阅读前的初筛!
💗 如果您觉得我们的项目对您有帮助 ChatPaperFree ,还请您给我们一些鼓励!⭐️ HuggingFace免费体验
2025-01-07 更新
AVATAR: Adversarial Autoencoders with Autoregressive Refinement for Time Series Generation
Authors:MohammadReza EskandariNasab, Shah Muhammad Hamdi, Soukaina Filali Boubrahimi
Data augmentation can significantly enhance the performance of machine learning tasks by addressing data scarcity and improving generalization. However, generating time series data presents unique challenges. A model must not only learn a probability distribution that reflects the real data distribution but also capture the conditional distribution at each time step to preserve the inherent temporal dependencies. To address these challenges, we introduce AVATAR, a framework that combines Adversarial Autoencoders (AAE) with Autoregressive Learning to achieve both objectives. Specifically, our technique integrates the autoencoder with a supervisor and introduces a novel supervised loss to assist the decoder in learning the temporal dynamics of time series data. Additionally, we propose another innovative loss function, termed distribution loss, to guide the encoder in more efficiently aligning the aggregated posterior of the autoencoder’s latent representation with a prior Gaussian distribution. Furthermore, our framework employs a joint training mechanism to simultaneously train all networks using a combined loss, thereby fulfilling the dual objectives of time series generation. We evaluate our technique across a variety of time series datasets with diverse characteristics. Our experiments demonstrate significant improvements in both the quality and practical utility of the generated data, as assessed by various qualitative and quantitative metrics.
数据增强可以通过解决数据稀缺问题和提高泛化能力来显著提高机器学习任务的性能。然而,生成时间序列数据存在独特的挑战。模型不仅必须学习反映真实数据分布的概率分布,还必须捕获每个时间步长的条件分布,以保持内在的临时依赖关系。为了解决这些挑战,我们引入了AVATAR框架,该框架结合了对抗性自编码器(AAE)和自回归学习来实现这两个目标。具体来说,我们的技术将自编码器与监督者相结合,并引入了一种新型监督损失,以帮助解码器学习时间序列数据的临时动态。此外,我们提出了另一种创新的损失函数,称为分布损失,以指导编码器更有效地使自编码器的潜在表示的聚合后验与先验高斯分布对齐。此外,我们的框架采用联合训练机制,使用组合损失同时训练所有网络,从而实现时间序列生成的两个目标。我们在具有不同特征的各种时间序列数据集上评估了我们的技术。实验表明,在各种定性和定量指标评估下,生成数据的质量和实用性都得到了显著提高。
论文及项目相关链接
PDF This work has been accepted to the SDM 2025 on December 20, 2024
Summary
数据增强能显著提升机器学习任务性能,通过解决数据稀缺性和提高泛化能力。针对时间序列数据的生成存在独特挑战,需要模型不仅学习真实数据分布的概率分布,还要捕捉每一步的条件分布以保持内在的临时依赖性。为此,我们引入了AVATAR框架,结合对抗自编码器(AAE)和自回归学习实现这两个目标。该框架使用创新性的损失函数引导编码器更高效地使自编码器的潜在表示的聚合后验与先验高斯分布对齐。通过联合训练机制同时训练所有网络,实现时间序列数据生成的双目标。实验表明,该技术在各种时间序列数据集上显著提高了生成数据的质量和实用性。
Key Takeaways
- 数据增强提升机器学习性能,通过解决数据稀缺和增强泛化能力。
- 生成时间序列数据存在独特挑战,需捕捉临时依赖性。
- AVATAR框架结合对抗自编码器和自回归学习应对这些挑战。
- 引入创新性的损失函数以辅助解码器学习时间序列数据的临时动态。
- 编码器通过新的损失函数更有效地对齐潜在表示的聚合后验与先验高斯分布。
- 使用联合训练机制同时实现时间序列生成的双目标。
点此查看论文截图
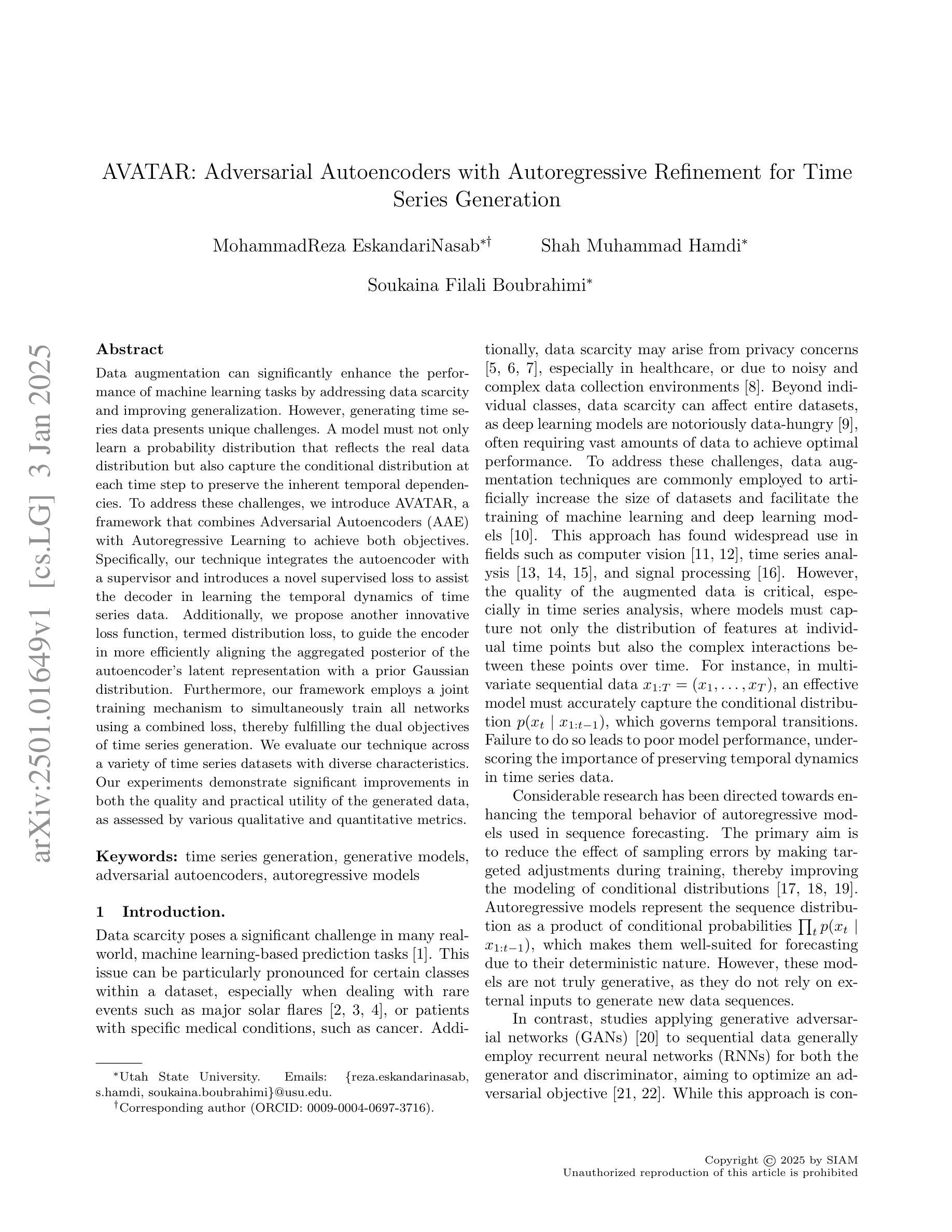
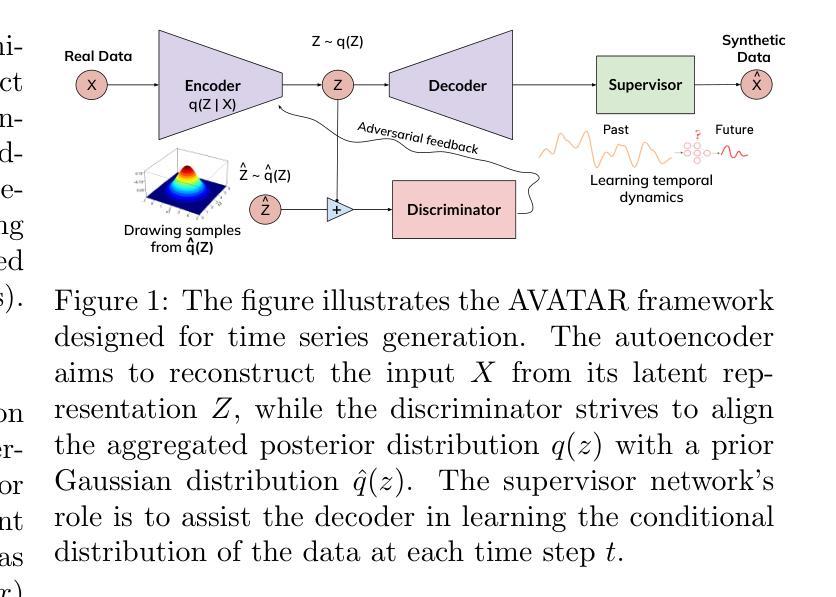