⚠️ 以下所有内容总结都来自于 大语言模型的能力,如有错误,仅供参考,谨慎使用
🔴 请注意:千万不要用于严肃的学术场景,只能用于论文阅读前的初筛!
💗 如果您觉得我们的项目对您有帮助 ChatPaperFree ,还请您给我们一些鼓励!⭐️ HuggingFace免费体验
2025-01-07 更新
HLV-1K: A Large-scale Hour-Long Video Benchmark for Time-Specific Long Video Understanding
Authors:Heqing Zou, Tianze Luo, Guiyang Xie, Victor, Zhang, Fengmao Lv, Guangcong Wang, Junyang Chen, Zhuochen Wang, Hansheng Zhang, Huaijian Zhang
Multimodal large language models have become a popular topic in deep visual understanding due to many promising real-world applications. However, hour-long video understanding, spanning over one hour and containing tens of thousands of visual frames, remains under-explored because of 1) challenging long-term video analyses, 2) inefficient large-model approaches, and 3) lack of large-scale benchmark datasets. Among them, in this paper, we focus on building a large-scale hour-long long video benchmark, HLV-1K, designed to evaluate long video understanding models. HLV-1K comprises 1009 hour-long videos with 14,847 high-quality question answering (QA) and multi-choice question asnwering (MCQA) pairs with time-aware query and diverse annotations, covering frame-level, within-event-level, cross-event-level, and long-term reasoning tasks. We evaluate our benchmark using existing state-of-the-art methods and demonstrate its value for testing deep long video understanding capabilities at different levels and for various tasks. This includes promoting future long video understanding tasks at a granular level, such as deep understanding of long live videos, meeting recordings, and movies.
多模态大型语言模型由于许多有前途的实景应用而成为了深度视觉理解领域的热门话题。然而,长达一小时的视频理解,由于其涵盖超过一小时的内容并包含数万个视频帧,仍然存在许多未被探索的领域,这主要是因为1)长期视频分析的挑战,2)低效的大型模型方法,以及3)缺乏大规模基准数据集。本文中,我们重点关注构建大规模长达一小时的视频基准数据集HLV-1K,旨在评估长视频理解模型。HLV-1K包含1009个长达一小时的视频,以及包含时间感知查询和多样化注释的14847个高质量问答(QA)和多项选择问答(MCQA)对,涵盖帧级、事件内级、跨事件级和长期推理任务。我们使用现有的最新方法对基准数据集进行评估,并证明了其在不同层次和不同任务上测试深度长视频理解能力的价值。这将有助于推动未来在细微层次上的长视频理解任务,例如对长直播视频、会议记录和电影等的深度理解。
论文及项目相关链接
Summary
多媒体长视频理解领域近年来受到广泛关注,因为存在许多具有潜力的现实应用。然而,关于超过一小时、包含成千上万视觉帧的长视频的理解仍然是研究领域的难点,面临长期视频分析挑战、大型模型方法效率低下以及缺乏大规模基准数据集的问题。本文重点介绍构建了一个大规模一小时长视频基准数据集HLV-1K,旨在评估长视频理解模型。该数据集包含高质量的问题回答和多项选择题对,覆盖不同级别的任务,并展示了其在测试深度长视频理解能力方面的价值。
Key Takeaways
- 多模态大型语言模型在深度视觉理解中受到关注,但对一小时以上的视频理解仍面临挑战。
- 本文构建了大规模一小时长视频基准数据集HLV-1K,用于评估长视频理解模型。
- HLV-1K包含高质量的问题回答和多项选择题对,涵盖不同级别的任务,如帧级别、事件内级别、跨事件级别和长期推理任务。
- 数据集的设计促进了未来对长视频理解的深入研究,如直播视频、会议记录、电影等的深度理解。
- 数据集能用于测试深度长视频理解能力在不同级别的能力。
点此查看论文截图
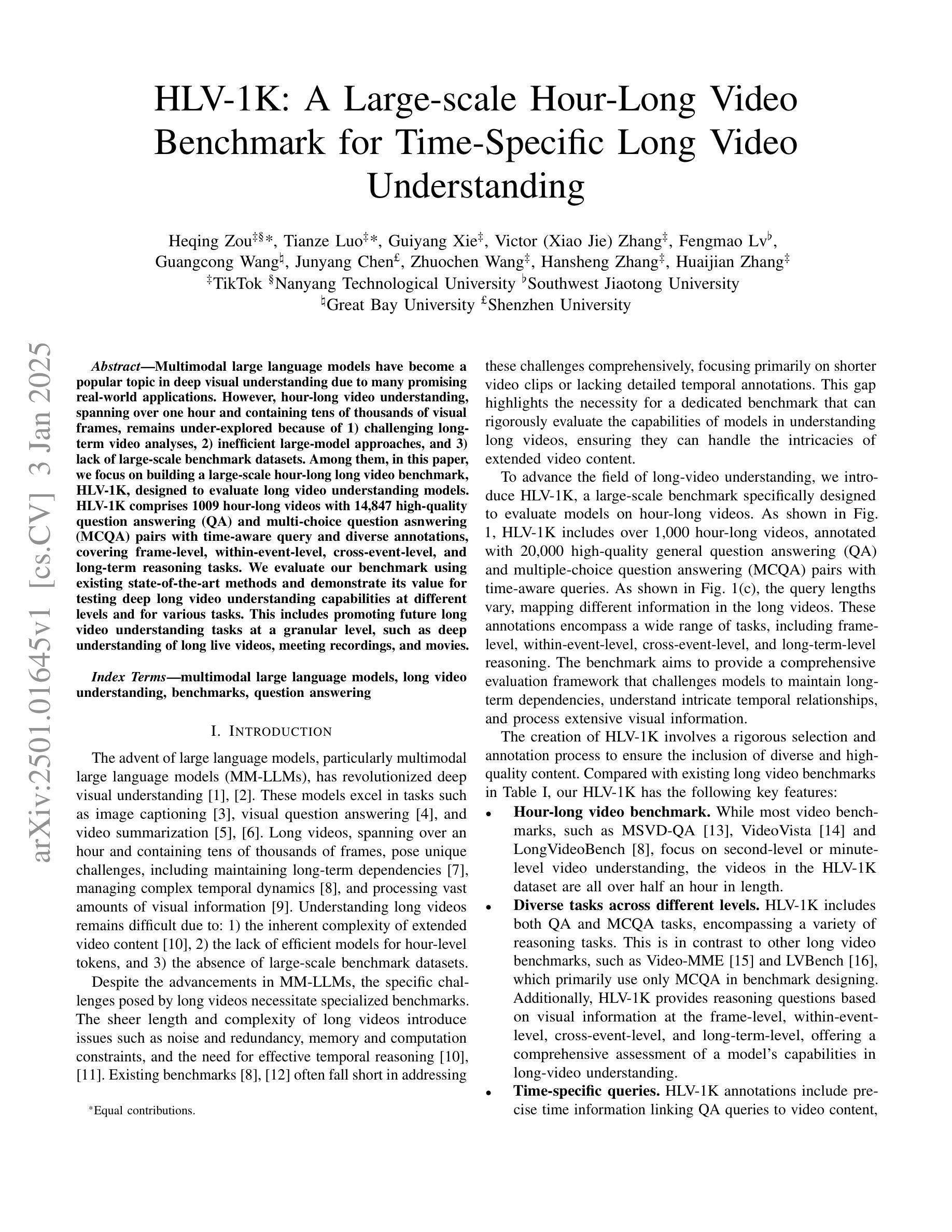
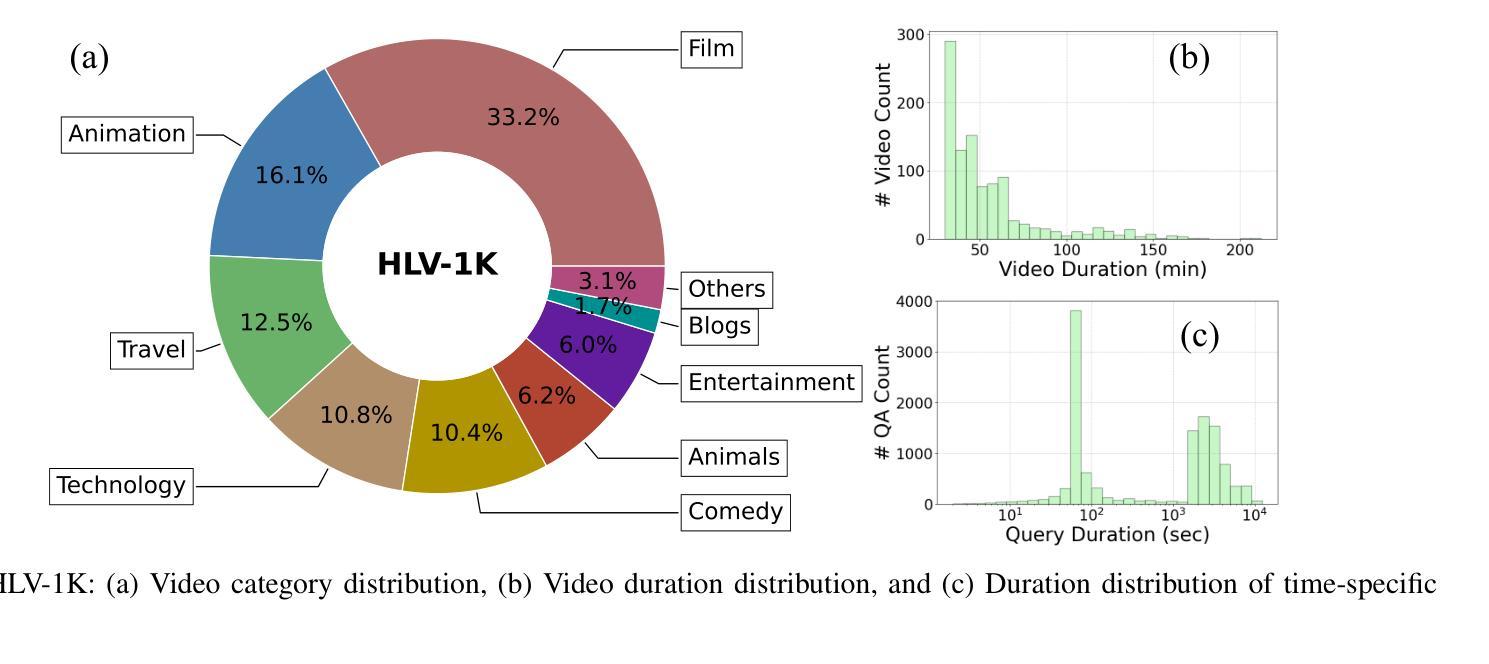
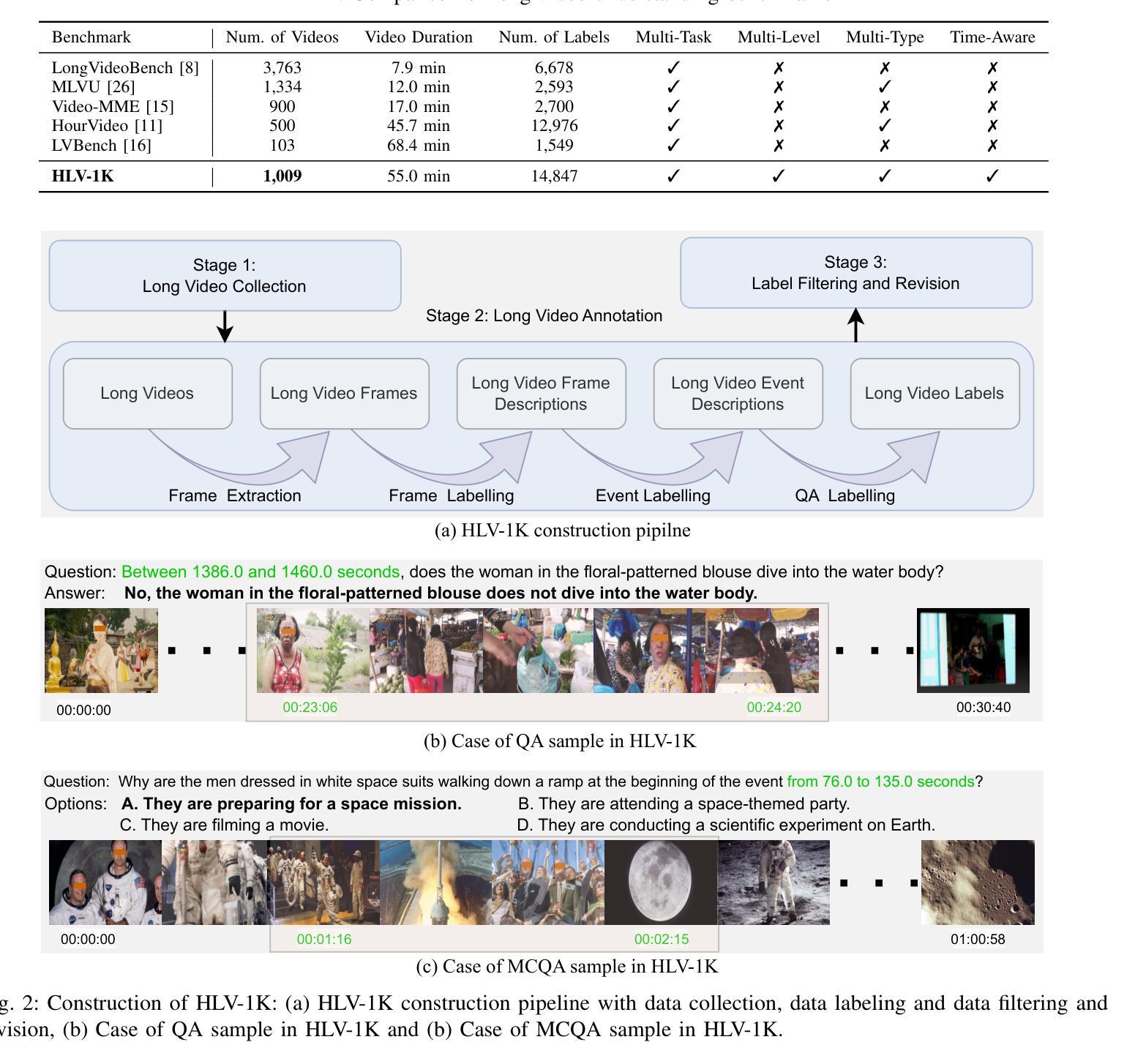
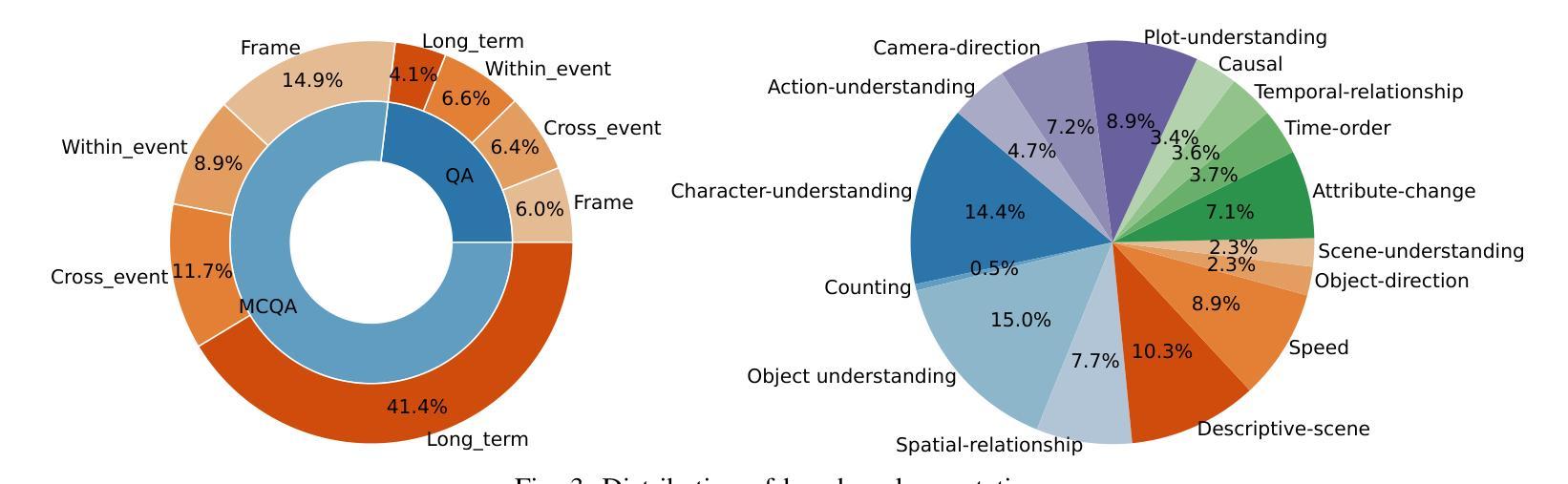
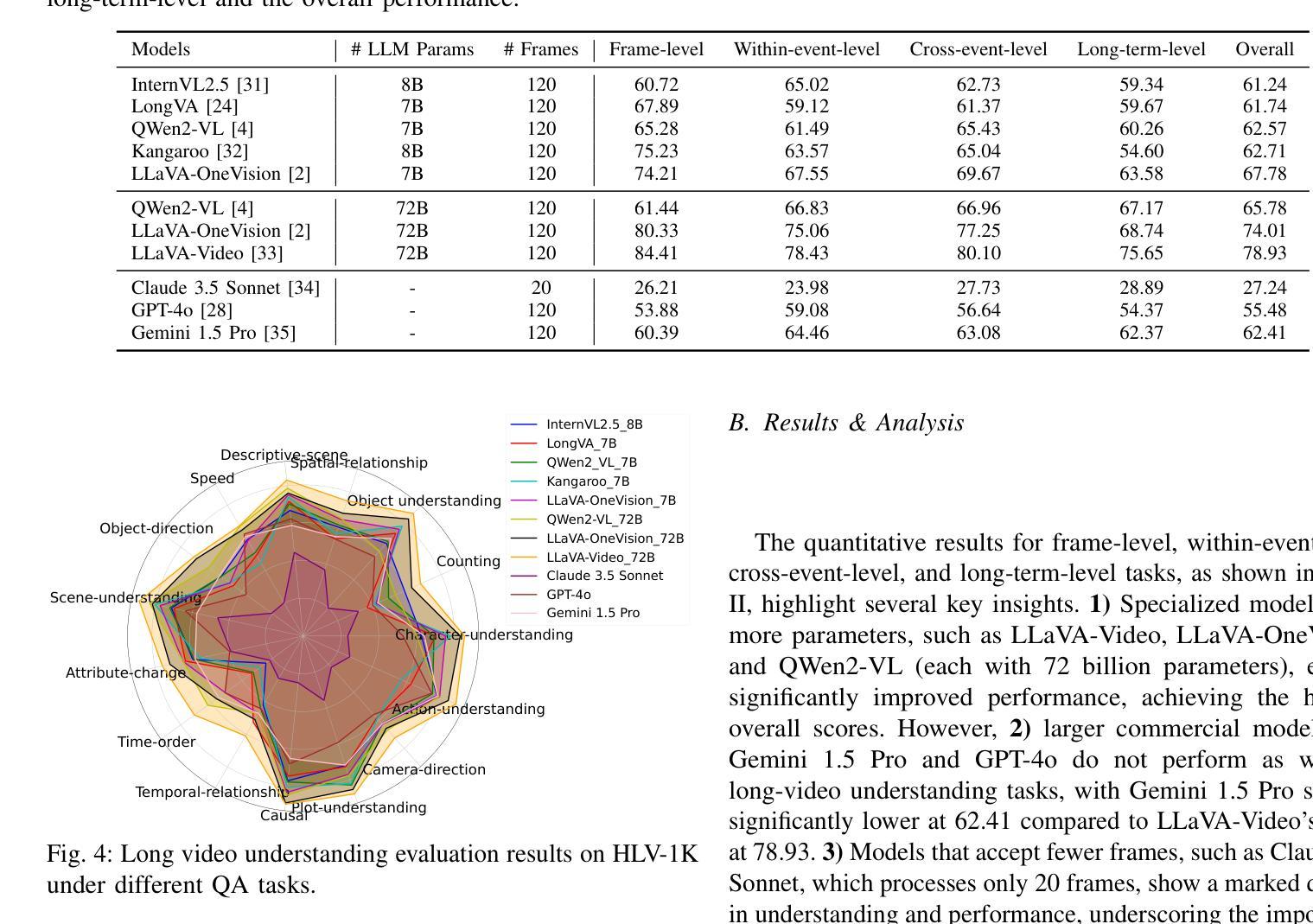
GPT4Scene: Understand 3D Scenes from Videos with Vision-Language Models
Authors:Zhangyang Qi, Zhixiong Zhang, Ye Fang, Jiaqi Wang, Hengshuang Zhao
In recent years, 2D Vision-Language Models (VLMs) have made significant strides in image-text understanding tasks. However, their performance in 3D spatial comprehension, which is critical for embodied intelligence, remains limited. Recent advances have leveraged 3D point clouds and multi-view images as inputs, yielding promising results. However, we propose exploring a purely vision-based solution inspired by human perception, which merely relies on visual cues for 3D spatial understanding. This paper empirically investigates the limitations of VLMs in 3D spatial knowledge, revealing that their primary shortcoming lies in the lack of global-local correspondence between the scene and individual frames. To address this, we introduce GPT4Scene, a novel visual prompting paradigm in VLM training and inference that helps build the global-local relationship, significantly improving the 3D spatial understanding of indoor scenes. Specifically, GPT4Scene constructs a 3D Bird’s Eye View (BEV) image from the video and marks consistent object IDs across both frames and the BEV image. The model then inputs the concatenated BEV image and video frames with markers. In zero-shot evaluations, GPT4Scene improves performance over closed-source VLMs like GPT-4o. Additionally, we prepare a processed video dataset consisting of 165K text annotation to fine-tune open-source VLMs, achieving state-of-the-art performance on all 3D understanding tasks. Surprisingly, after training with the GPT4Scene paradigm, VLMs consistently improve during inference, even without visual prompting and BEV image as explicit correspondence. It demonstrates that the proposed paradigm helps VLMs develop an intrinsic ability to understand 3D scenes, which paves the way for a noninvasive approach to extending pre-trained VLMs for 3D scene understanding.
近年来,二维视觉语言模型(VLMs)在图文理解任务中取得了显著进展。然而,它们在三维空间理解方面的表现,对于体现智能至关重要,仍然有限。最近的进展利用三维点云和多视角图像作为输入,取得了有前景的结果。然而,我们提出探索一种纯基于视觉的解决方案,该方案受到人类感知的启发,仅依赖视觉线索进行三维空间理解。
论文及项目相关链接
PDF Project page: https://gpt4scene.github.io/
摘要
近年来,二维视觉语言模型(VLMs)在图像文本理解任务中取得了显著进展。然而,它们在三维空间理解方面的表现仍然有限,这对于智能实体至关重要。尽管已有研究利用三维点云和多视角图像作为输入取得了有前景的结果,但本文提出了一个纯粹基于视觉的解决方案,该方案借鉴人类感知机制,仅依靠视觉线索进行三维空间理解。本文实证研究了VLMs在三维空间知识方面的局限性,发现其主要缺点在于场景与个体帧之间缺乏全局局部对应关系。为解决这一问题,我们提出了GPT4Scene,这是一种新型视觉提示范式,用于VLM训练和推理,有助于建立全局局部关系,显著提高对室内场景的三维空间理解能力。GPT4Scene通过构建视频的三维鸟瞰图(BEV)图像,并在帧和BEV图像中标记一致的对象ID来实现这一目标。然后模型将带有标记的BEV图像和视频帧作为输入。在零样本评估中,GPT4Scene改进了封闭源VLMs(如GPT-4o)的性能。此外,我们还准备了一个包含16.5万文本注释的处理过的视频数据集,以微调开源VLMs,在所有三维理解任务上实现最佳性能。令人惊讶的是,经过GPT4Scene范式训练后,即使在不需要视觉提示和BEV图像作为明确对应的情况下,VLMs在推理过程中的表现也持续提高。这表明所提出的范式有助于VLMs发展内在理解三维场景的能力,为扩展预训练VLMs进行三维场景理解提供了非侵入性方法的途径。
要点提炼
- 2D VLMs在图像文本理解任务中取得显著进展,但在关键的三维空间理解方面存在局限性。
- 现有研究尝试利用三维点云和多视角图像作为输入来提升性能。
- 本文提出GPT4Scene方法,一个基于视觉的解决方法,旨在建立场景与帧之间的全局局部关系。
- GPT4Scene通过构建三维鸟瞰图(BEV)图像和标记对象ID来实现这一关系。
- GPT4Scene能提高零样本评估中的性能并改进封闭源VLMs的表现。
- 使用处理过的视频数据集微调开源VLMs后,在所有三维理解任务上实现最佳性能。
点此查看论文截图
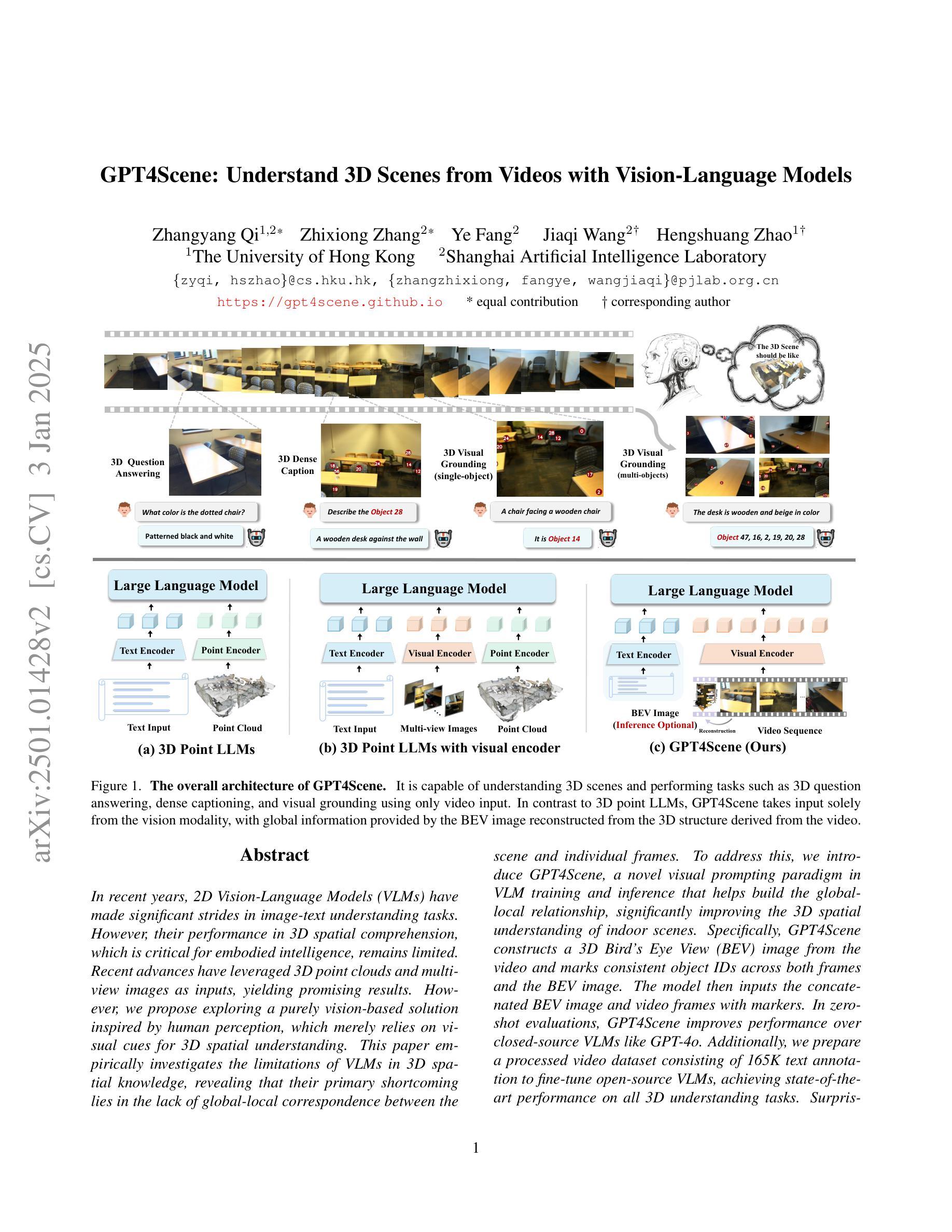
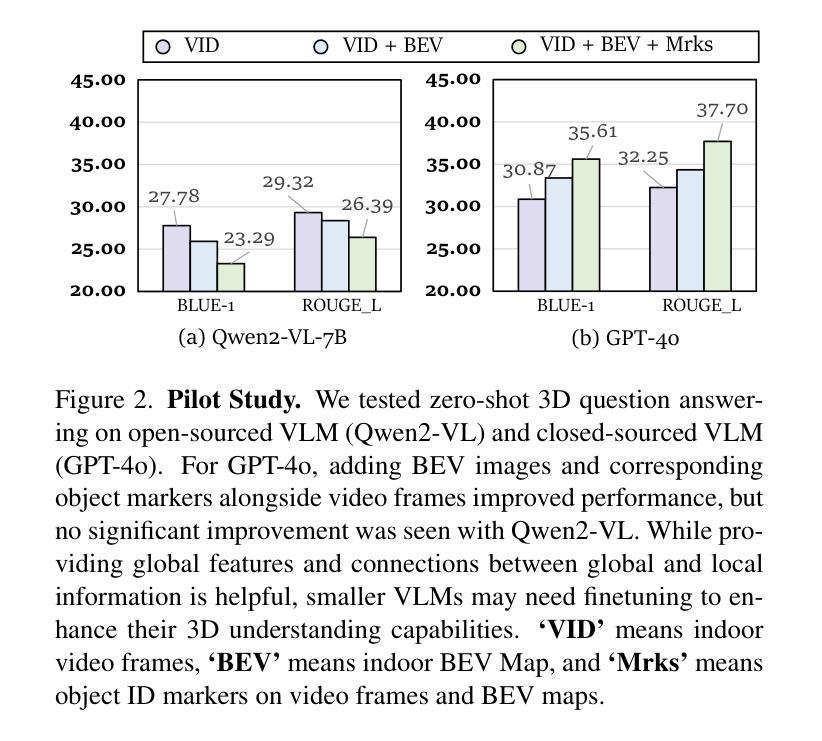
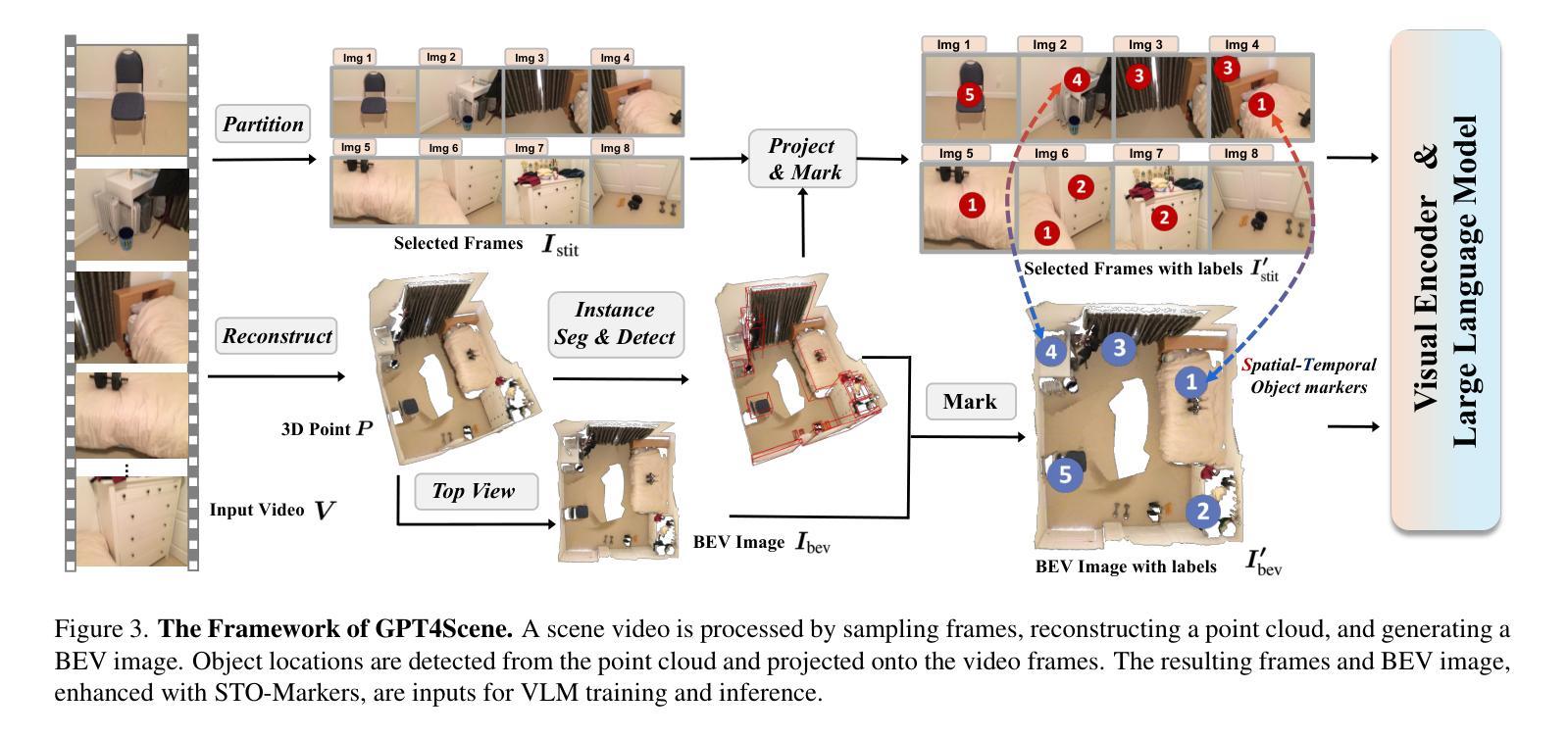
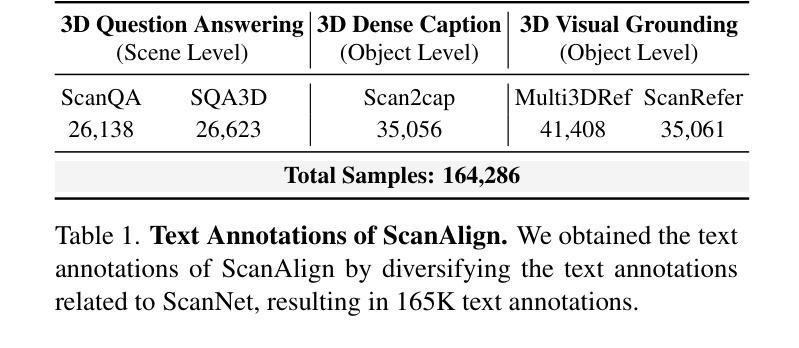
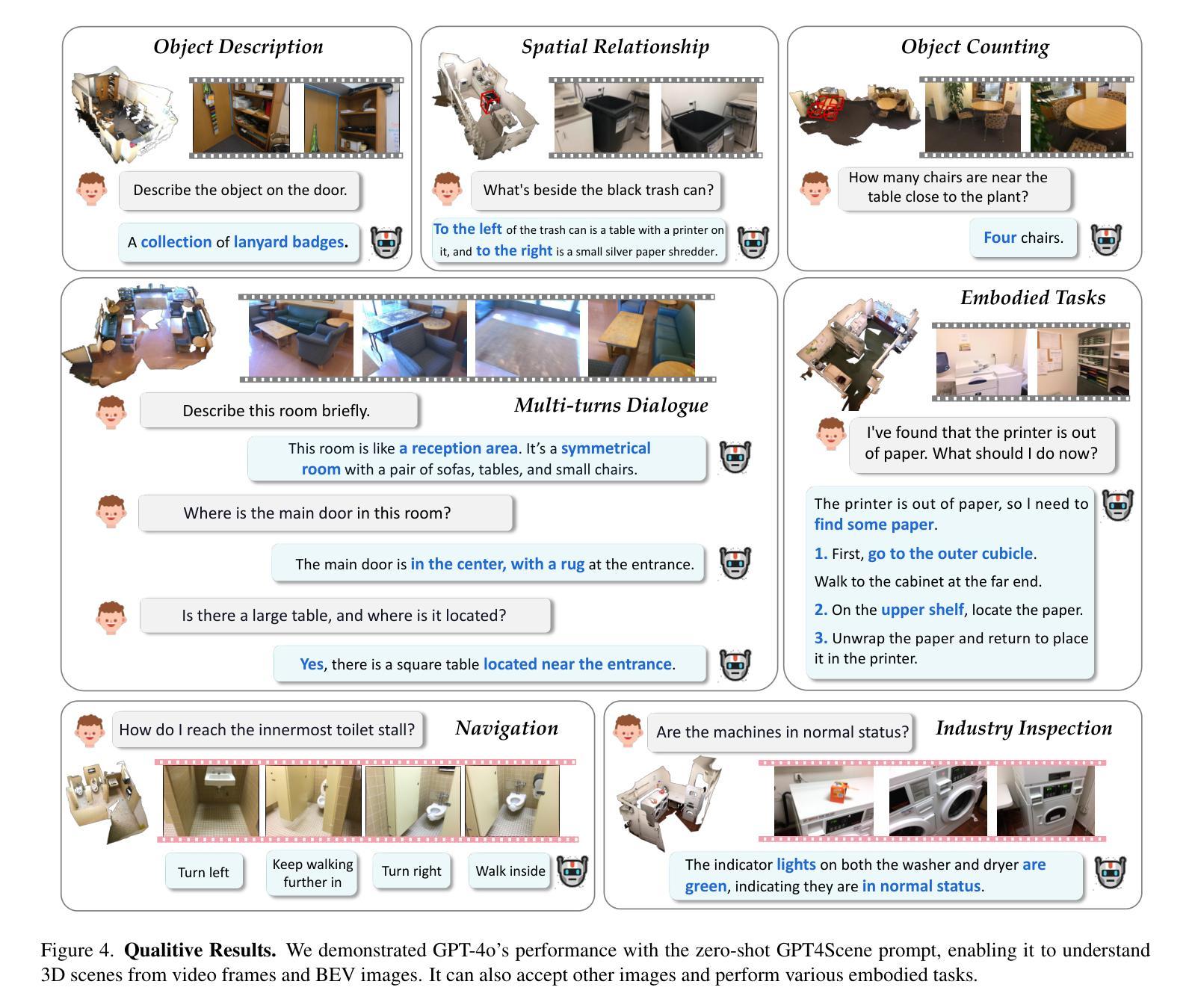