⚠️ 以下所有内容总结都来自于 大语言模型的能力,如有错误,仅供参考,谨慎使用
🔴 请注意:千万不要用于严肃的学术场景,只能用于论文阅读前的初筛!
💗 如果您觉得我们的项目对您有帮助 ChatPaperFree ,还请您给我们一些鼓励!⭐️ HuggingFace免费体验
2025-01-07 更新
Reading to Listen at the Cocktail Party: Multi-Modal Speech Separation
Authors:Akam Rahimi, Triantafyllos Afouras, Andrew Zisserman
The goal of this paper is speech separation and enhancement in multi-speaker and noisy environments using a combination of different modalities. Previous works have shown good performance when conditioning on temporal or static visual evidence such as synchronised lip movements or face identity. In this paper, we present a unified framework for multi-modal speech separation and enhancement based on synchronous or asynchronous cues. To that end we make the following contributions: (i) we design a modern Transformer-based architecture tailored to fuse different modalities to solve the speech separation task in the raw waveform domain; (ii) we propose conditioning on the textual content of a sentence alone or in combination with visual information; (iii) we demonstrate the robustness of our model to audio-visual synchronisation offsets; and, (iv) we obtain state-of-the-art performance on the well-established benchmark datasets LRS2 and LRS3.
本文的目标是在多说话人和嘈杂的环境中进行语音分离和增强,方法是将不同的模态结合起来。以前的工作在基于时间或静态的视觉证据(如同步的唇部动作或面部身份)时表现出良好的性能。在本文中,我们提出了基于同步或异步线索的多模态语音分离和增强的统一框架。为此,我们做出了以下贡献:(i)我们设计了一种基于现代Transformer的架构,该架构能够融合不同的模态,以解决原始波形域中的语音分离任务;(ii)我们提出以句子中的文本内容或结合视觉信息进行条件处理;(iii)我们证明了我们的模型对视听同步偏移的鲁棒性;(iv)我们在成熟的LRS2和LRS3基准数据集上获得了最先进的性能。
论文及项目相关链接
Summary
多语种融合模态语音分离增强方法的研究。该论文提出一种基于同步或异步线索的多模态语音分离和增强统一框架,利用不同模态的组合,在原始波形域解决语音分离任务。论文贡献包括设计基于Transformer的架构融合不同模态信息,提出基于句子文本内容或结合视觉信息的条件化模型,展示模型对音视频同步偏移的鲁棒性,并在LRS2和LRS3基准数据集上获得最佳性能。
Key Takeaways
- 该论文研究多语种环境下的语音分离与增强技术。
- 论文提出了一种多模态语音分离和增强的统一框架。
- 该框架可基于同步或异步线索进行工作。
- 研究者设计了一种基于Transformer的架构来解决语音分离任务,该架构能够融合不同的模态信息。
- 论文探索了将句子文本内容作为条件化模型的可能性,也可结合视觉信息使用。
- 论文中的模型对音视频同步偏移具有鲁棒性。
点此查看论文截图
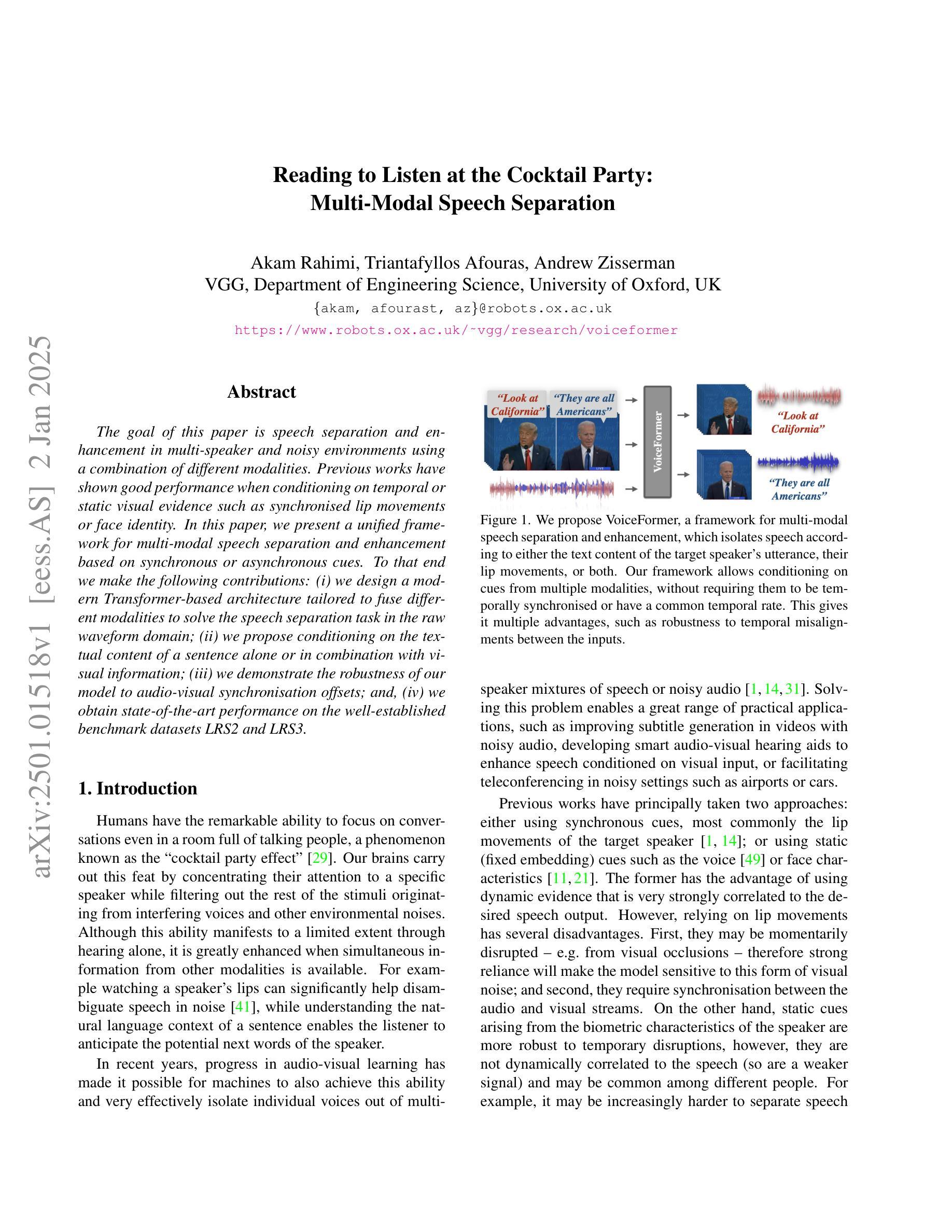
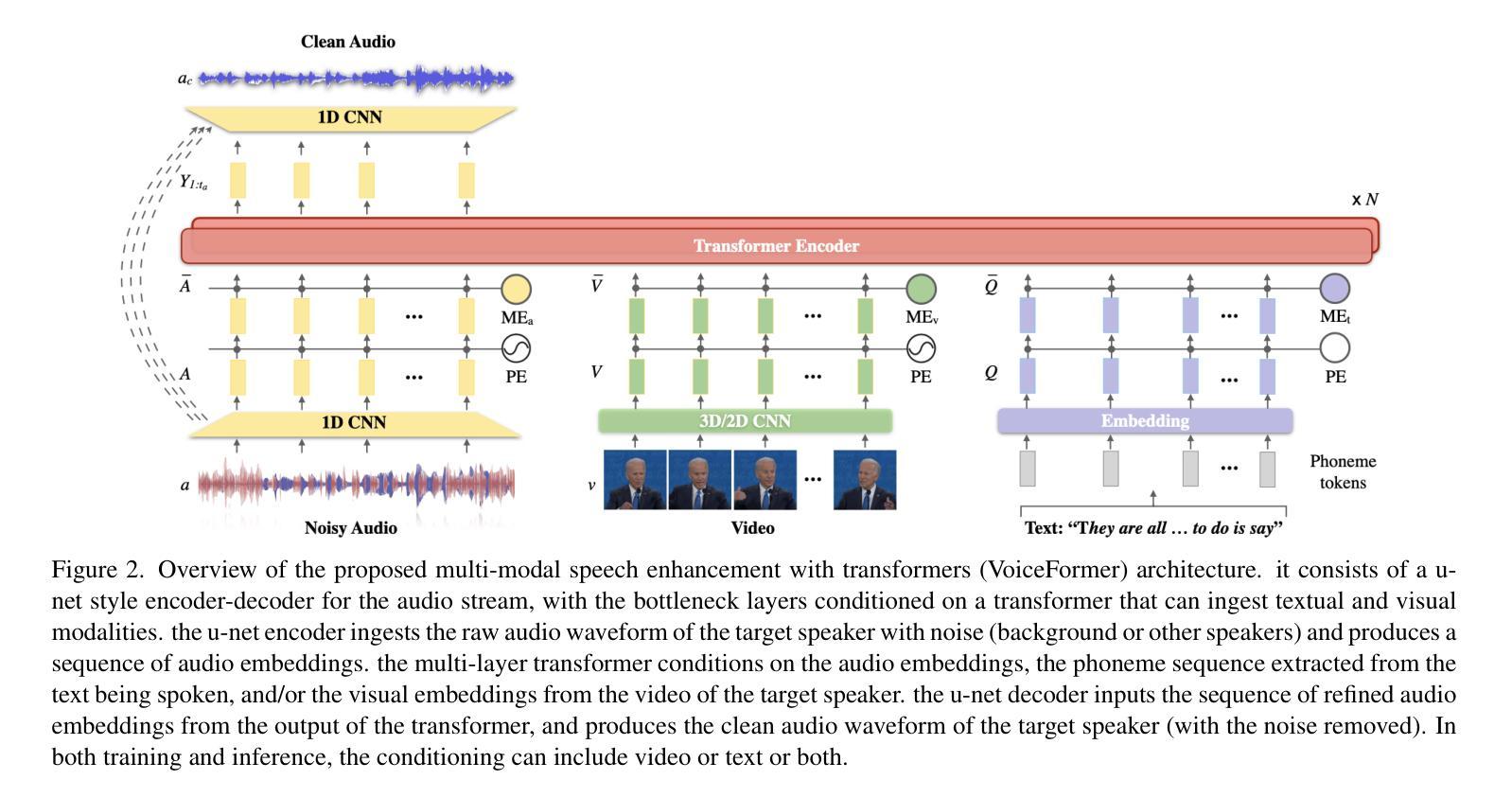
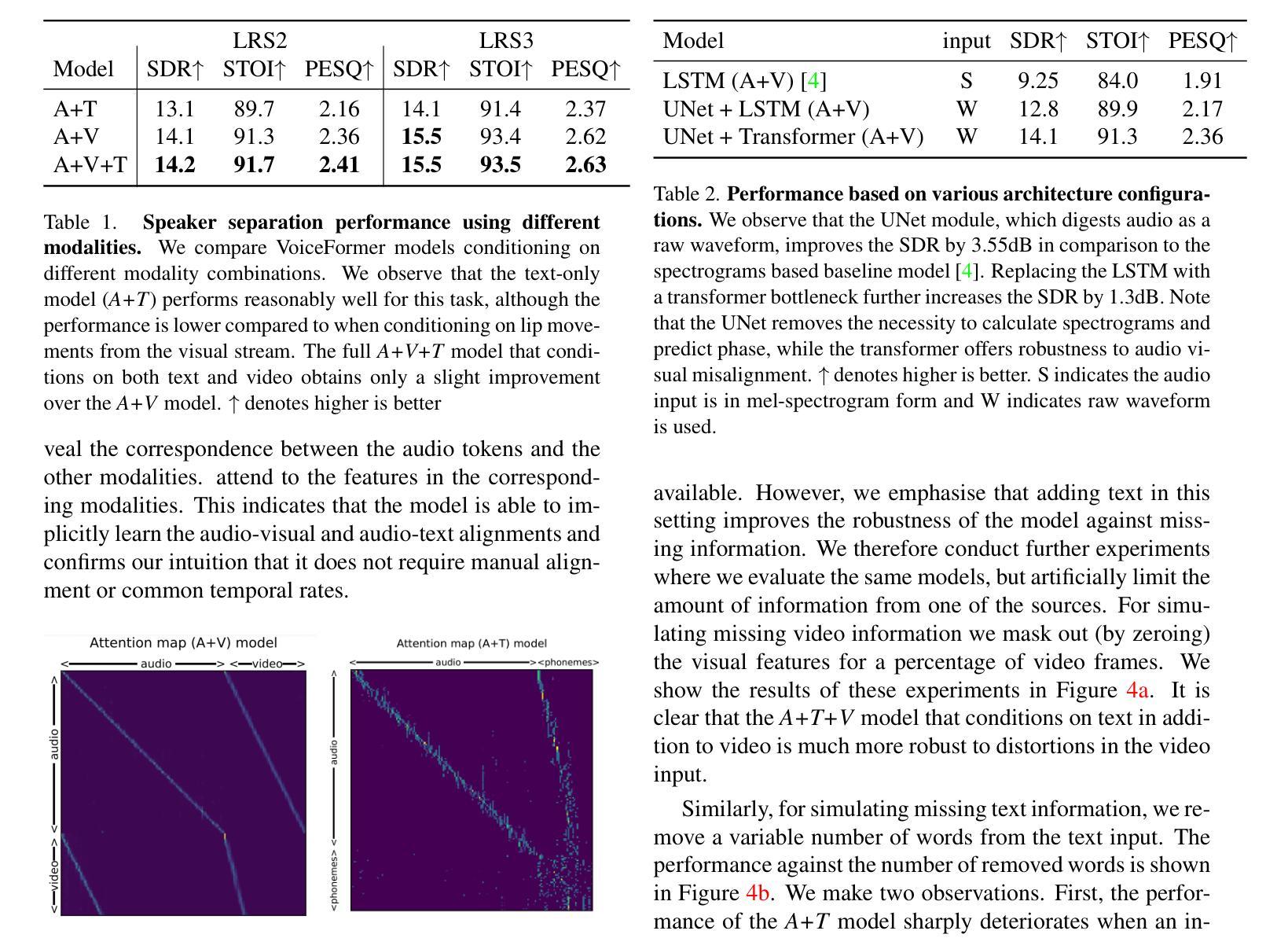
Learning Chemical Reaction Representation with Reactant-Product Alignment
Authors:Kaipeng Zeng, Xianbin Liu, Yu Zhang, Xiaokang Yang, Yaohui Jin, Yanyan Xu
Organic synthesis stands as a cornerstone of the chemical industry. The development of robust machine learning models to support tasks associated with organic reactions is of significant interest. However, current methods rely on hand-crafted features or direct adaptations of model architectures from other domains, which lack feasibility as data scales increase or ignore the rich chemical information inherent in reactions. To address these issues, this paper introduces RAlign, a novel chemical reaction representation learning model for various organic reaction-related tasks. By integrating atomic correspondence between reactants and products, our model discerns the molecular transformations that occur during the reaction, thereby enhancing comprehension of the reaction mechanism. We have designed an adapter structure to incorporate reaction conditions into the chemical reaction representation, allowing the model to handle various reaction conditions and to adapt to various datasets and downstream tasks. Additionally, we introduce a reaction-center-aware attention mechanism that enables the model to concentrate on key functional groups, thereby generating potent representations for chemical reactions. Our model has been evaluated on a range of downstream tasks. Experimental results indicate that our model markedly outperforms existing chemical reaction representation learning architectures on most of the datasets. We plan to open-source the code contingent upon the acceptance of the paper.
有机合成是化学工业的基石。开发强大的机器学习模型来支持与有机反应相关的任务具有极大的兴趣。然而,当前的方法依赖于手工特征或使用其他领域模型架构的直接适应,这在数据规模增加时缺乏可行性,或者忽略了反应中固有的丰富化学信息。为了解决这些问题,本文介绍了RAlign,这是一种用于各种有机反应相关任务的新型化学反应表征学习模型。通过整合反应物和产物之间的原子对应关系,我们的模型能够识别反应过程中发生的分子转化,从而提高对反应机制的理解。我们设计了一种适配器结构,将反应条件融入化学反应表征中,使模型能够处理各种反应条件,并适应各种数据集和下游任务。此外,我们还引入了一种反应中心感知注意力机制,使模型能够关注关键官能团,从而为化学反应生成有效的表征。我们的模型在多种下游任务上进行了评估。实验结果表明,在大多数数据集上,我们的模型显著优于现有的化学反应表征学习架构。我们计划根据论文的接受情况开源代码。
论文及项目相关链接
Summary
有机合成是化学工业的核心。针对有机反应相关任务,开发强大的机器学习模型具有重要意义。然而,当前方法依赖于手工特征或模型架构的直接适应,随着数据规模的增加,这些方法缺乏可行性或忽略了反应中的丰富化学信息。本文引入RAlign模型,这是一种新型化学反应表征学习模型,用于各种有机反应相关任务。通过整合反应物和产物之间的原子对应关系,该模型能够区分反应中发生的分子转化,从而提高对反应机制的理解。此外,我们还设计了适应反应条件的适配器结构,使模型能够处理各种反应条件并适应不同的数据集和下游任务。我们还引入了一种反应中心注意力机制,使模型能够关注关键官能团,从而生成有效的化学反应表征。实验结果表明,该模型在大多数数据集上显著优于现有的化学反应表征学习架构。
Key Takeaways
- 有机合成是化学工业的核心,开发用于支持有机反应相关任务的机器学习模型至关重要。
- 当前方法在处理大规模数据时存在局限性,无法充分利用反应中的丰富化学信息。
- RAlign模型是一种新型化学反应表征学习模型,通过整合反应物和产物之间的原子对应关系来提高对反应机制的理解。
- RAlign模型设计了适配器结构,以处理各种反应条件并适应不同的数据集和下游任务。
- 反应中心注意力机制使模型能够关注关键官能团,生成有效的化学反应表征。
- 实验结果表明,RAlign模型在多个数据集上显著优于现有模型。
点此查看论文截图
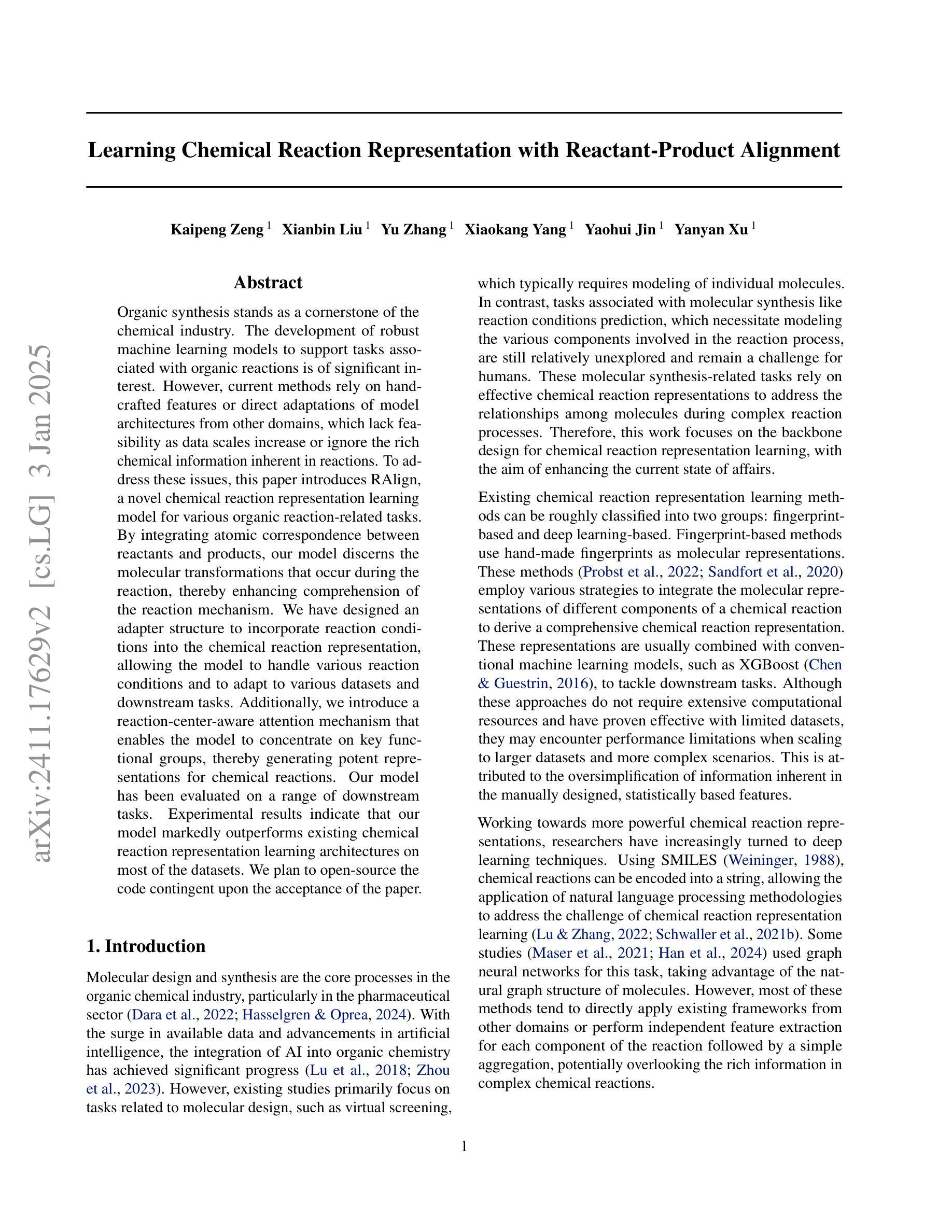
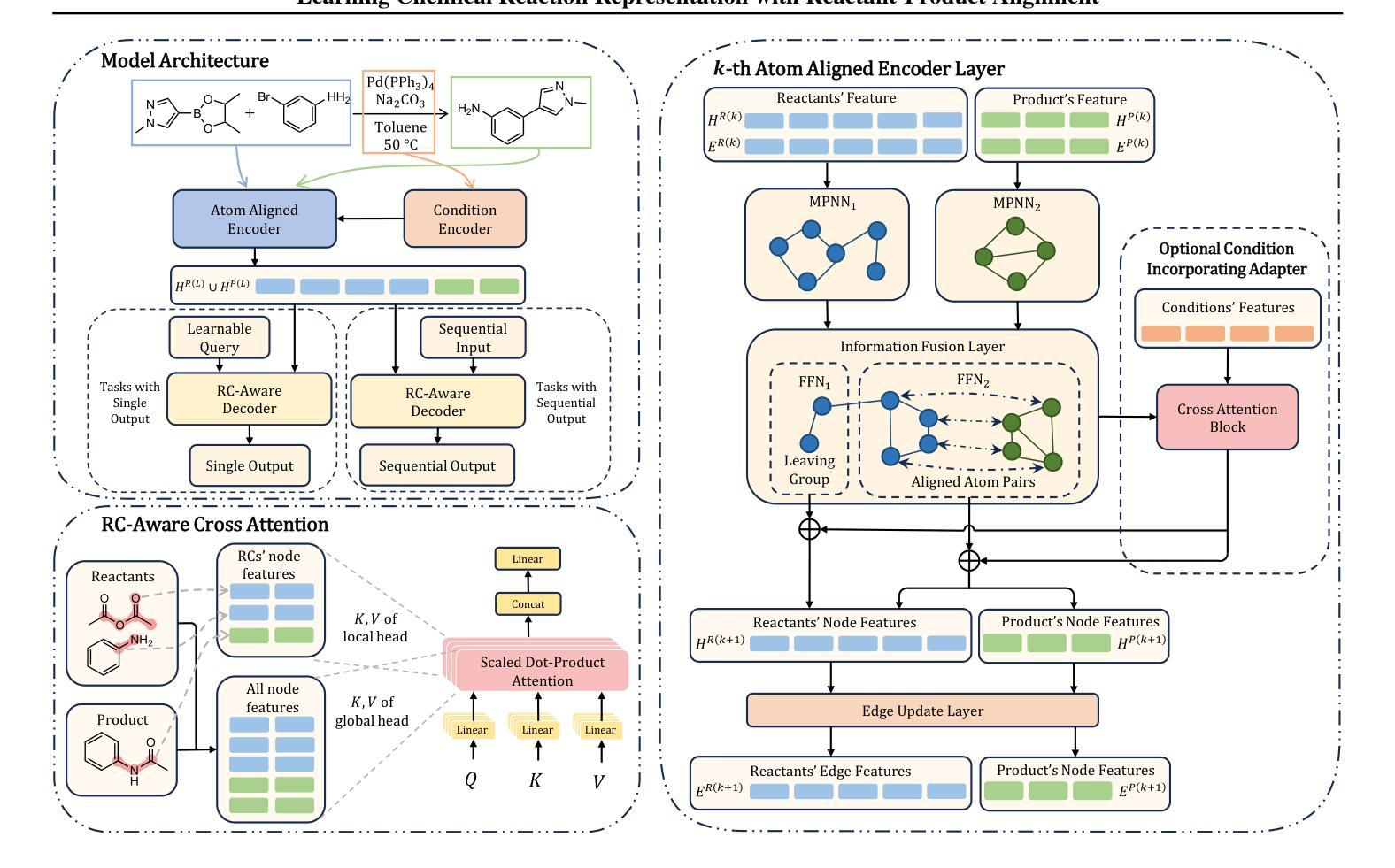