⚠️ 以下所有内容总结都来自于 大语言模型的能力,如有错误,仅供参考,谨慎使用
🔴 请注意:千万不要用于严肃的学术场景,只能用于论文阅读前的初筛!
💗 如果您觉得我们的项目对您有帮助 ChatPaperFree ,还请您给我们一些鼓励!⭐️ HuggingFace免费体验
2025-01-08 更新
Auto-CARD: Efficient and Robust Codec Avatar Driving for Real-time Mobile Telepresence
Authors:Yonggan Fu, Yuecheng Li, Chenghui Li, Jason Saragih, Peizhao Zhang, Xiaoliang Dai, Yingyan Celine Lin
Real-time and robust photorealistic avatars for telepresence in AR/VR have been highly desired for enabling immersive photorealistic telepresence. However, there still exists one key bottleneck: the considerable computational expense needed to accurately infer facial expressions captured from headset-mounted cameras with a quality level that can match the realism of the avatar’s human appearance. To this end, we propose a framework called Auto-CARD, which for the first time enables real-time and robust driving of Codec Avatars when exclusively using merely on-device computing resources. This is achieved by minimizing two sources of redundancy. First, we develop a dedicated neural architecture search technique called AVE-NAS for avatar encoding in AR/VR, which explicitly boosts both the searched architectures’ robustness in the presence of extreme facial expressions and hardware friendliness on fast evolving AR/VR headsets. Second, we leverage the temporal redundancy in consecutively captured images during continuous rendering and develop a mechanism dubbed LATEX to skip the computation of redundant frames. Specifically, we first identify an opportunity from the linearity of the latent space derived by the avatar decoder and then propose to perform adaptive latent extrapolation for redundant frames. For evaluation, we demonstrate the efficacy of our Auto-CARD framework in real-time Codec Avatar driving settings, where we achieve a 5.05x speed-up on Meta Quest 2 while maintaining a comparable or even better animation quality than state-of-the-art avatar encoder designs.
在增强现实(AR)和虚拟现实(VR)中实现沉浸式逼真远程存在的一个重要需求是实时且强大的逼真虚拟化身。然而,仍存在一个关键瓶颈:为了从头戴式相机中准确推断面部表情,并实现与虚拟化身逼真的人类外观相匹配的画质水平,需要大量的计算开销。为此,我们提出了一个名为Auto-CARD的框架,该框架首次实现仅使用设备本身的计算资源来实时驱动高质量虚拟化身。这是通过最小化两个冗余来源来实现的。首先,我们开发了一种专门用于AR/VR虚拟化身编码的神经网络架构搜索技术,称为AVE-NAS,该技术不仅提高了在极端面部表情下的搜索架构稳健性,还提高了对快速演变的AR/VR头戴设备的硬件友好性。其次,我们利用连续渲染过程中连续捕获图像的时空冗余性,并开发了一种称为LATEX的机制来跳过冗余帧的计算。具体来说,我们首先识别由虚拟化身解码器推导出的潜在空间的线性特征,然后提出对冗余帧进行自适应潜在外推。为了评估效果,我们在实时编码化身驱动环境中展示了Auto-CARD框架的有效性。在Meta Quest 2上,我们在保持或甚至超越当前最先进的化身编码器设计动画质量的同时,实现了5.05倍的加速效果。
论文及项目相关链接
PDF Accepted by CVPR 2023
Summary
实时且稳健的光栅化化身技术对于增强现实和虚拟现实中的沉浸式体验至关重要。然而,目前仍存在关键瓶颈,即从头戴式相机捕捉到的面部表情推断所需的大量计算资源。我们提出了一种名为Auto-CARD的框架,它首次实现了仅使用设备本身的计算能力驱动实时且稳健的编解码化身。通过最小化冗余信息的两个来源来实现这一目标。首先,我们开发了一种专门针对AR/VR编解码化身的神经网络架构搜索技术AVE-NAS,该技术提高了搜索到的架构在面对极端面部表情时的稳健性以及硬件友好性。其次,我们利用连续渲染过程中连续图像的时间冗余性,提出了一种名为LATEX的机制来跳过冗余帧的计算。我们在实时编解码化身驱动环境中验证了Auto-CARD框架的有效性,在Meta Quest 2上实现了5.05倍的速度提升,同时保持了甚至优于当前最先进化身编码器设计的动画质量。
Key Takeaways
- 实现实时且稳健的光栅化化身技术的挑战在于准确推断头戴式相机捕捉到的面部表情所需的大量计算资源。
- Auto-CARD框架首次实现了仅使用设备本身的计算能力来驱动实时且稳健的编解码化身。
- 通过最小化冗余信息来源实现这一目标,包括通过AVE-NAS技术提高架构稳健性和硬件友好性,以及利用连续图像的时间冗余性来跳过冗余帧的计算。
点此查看论文截图
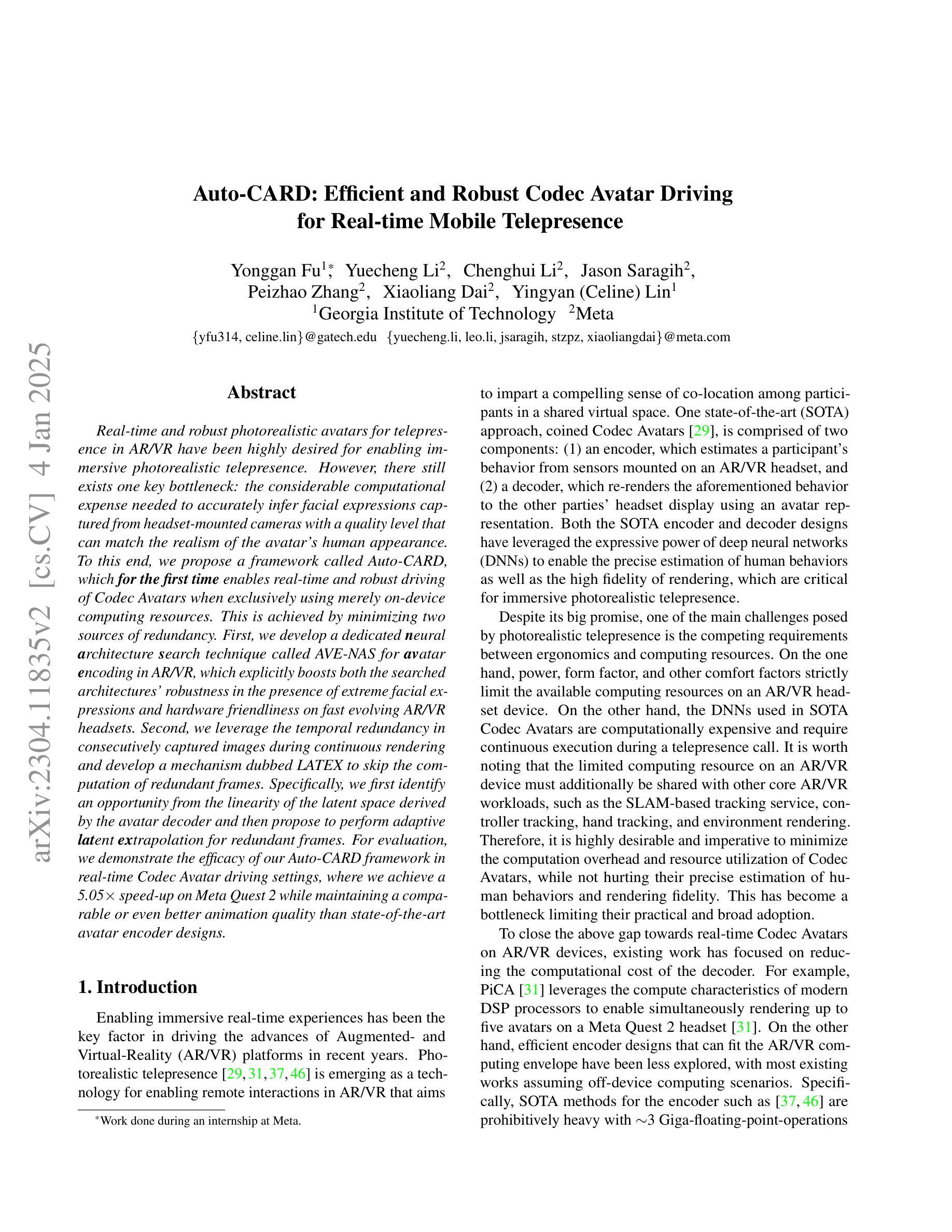
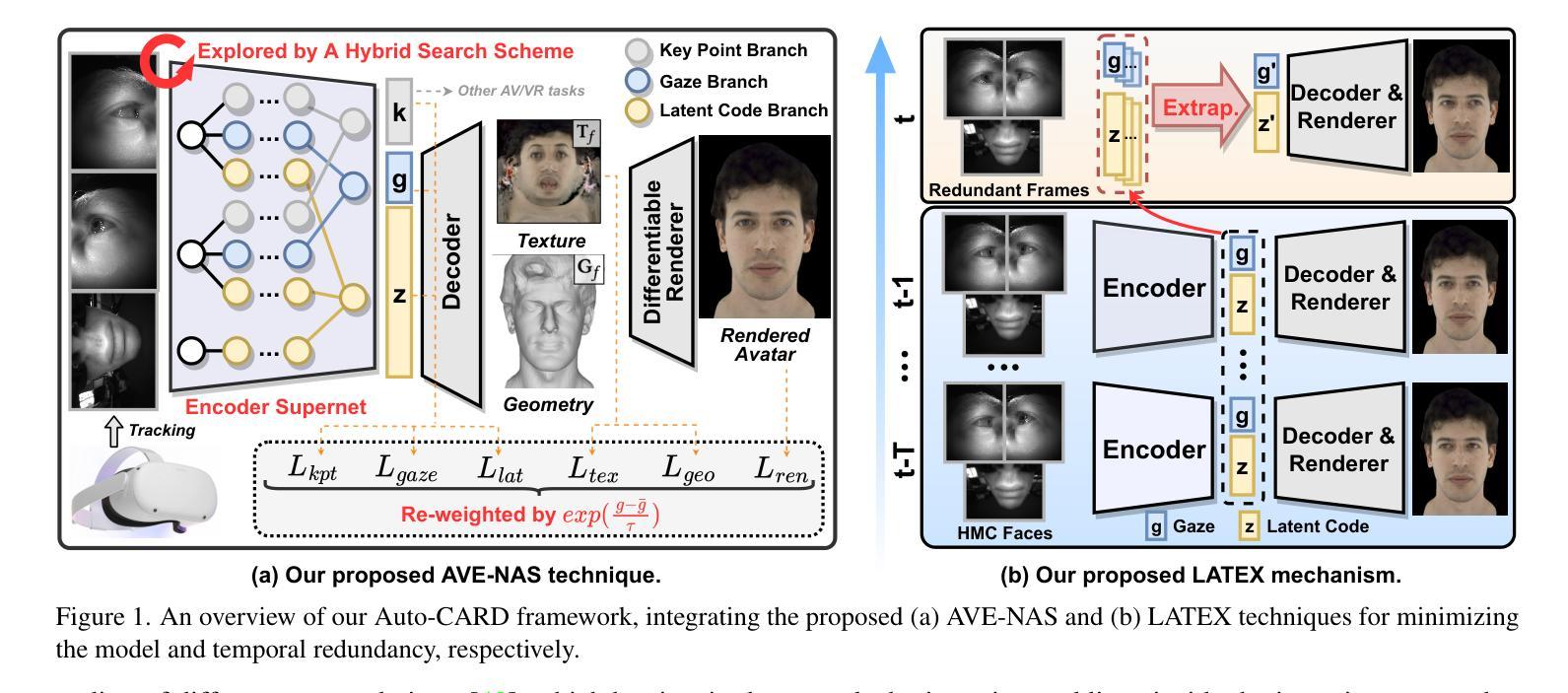
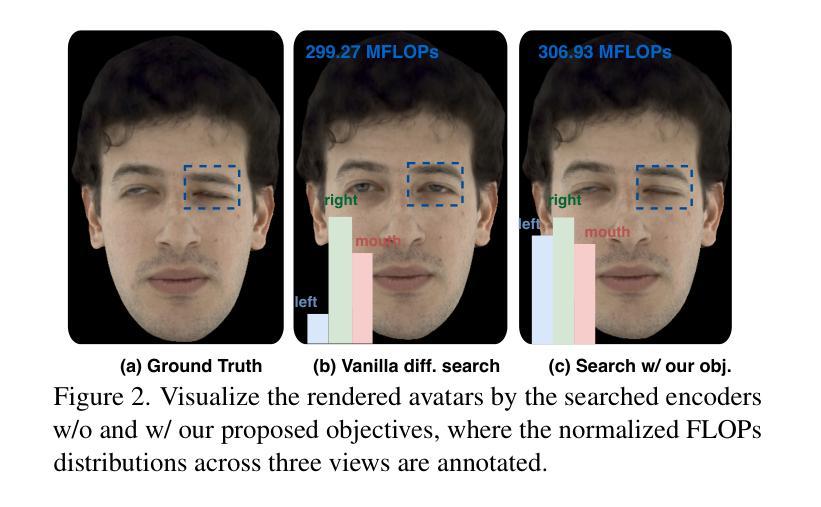
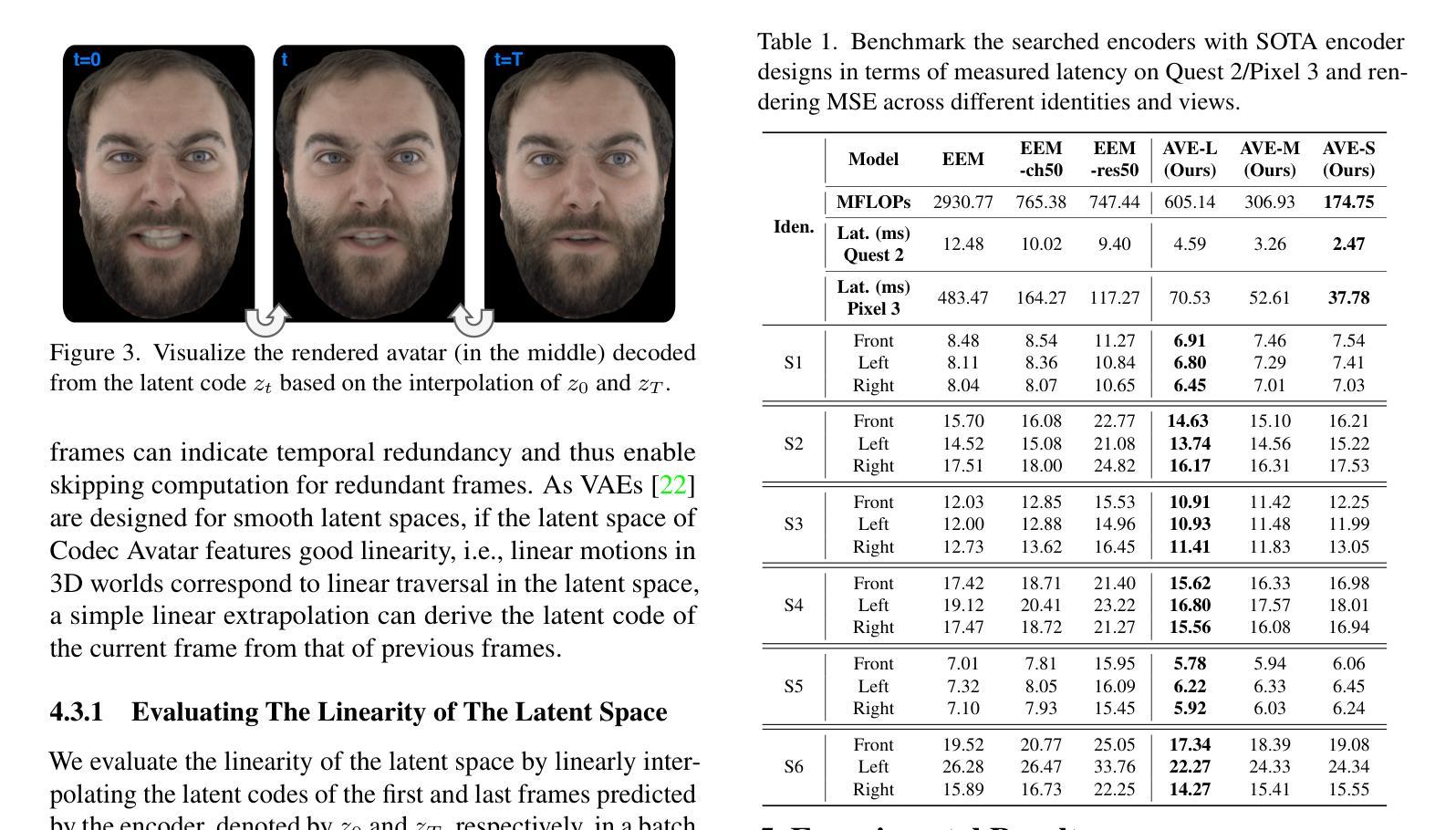