⚠️ 以下所有内容总结都来自于 大语言模型的能力,如有错误,仅供参考,谨慎使用
🔴 请注意:千万不要用于严肃的学术场景,只能用于论文阅读前的初筛!
💗 如果您觉得我们的项目对您有帮助 ChatPaperFree ,还请您给我们一些鼓励!⭐️ HuggingFace免费体验
2025-01-08 更新
STAR: Spatial-Temporal Augmentation with Text-to-Video Models for Real-World Video Super-Resolution
Authors:Rui Xie, Yinhong Liu, Penghao Zhou, Chen Zhao, Jun Zhou, Kai Zhang, Zhenyu Zhang, Jian Yang, Zhenheng Yang, Ying Tai
Image diffusion models have been adapted for real-world video super-resolution to tackle over-smoothing issues in GAN-based methods. However, these models struggle to maintain temporal consistency, as they are trained on static images, limiting their ability to capture temporal dynamics effectively. Integrating text-to-video (T2V) models into video super-resolution for improved temporal modeling is straightforward. However, two key challenges remain: artifacts introduced by complex degradations in real-world scenarios, and compromised fidelity due to the strong generative capacity of powerful T2V models (\textit{e.g.}, CogVideoX-5B). To enhance the spatio-temporal quality of restored videos, we introduce\textbf{\name} (\textbf{S}patial-\textbf{T}emporal \textbf{A}ugmentation with T2V models for \textbf{R}eal-world video super-resolution), a novel approach that leverages T2V models for real-world video super-resolution, achieving realistic spatial details and robust temporal consistency. Specifically, we introduce a Local Information Enhancement Module (LIEM) before the global attention block to enrich local details and mitigate degradation artifacts. Moreover, we propose a Dynamic Frequency (DF) Loss to reinforce fidelity, guiding the model to focus on different frequency components across diffusion steps. Extensive experiments demonstrate\textbf{\name}~outperforms state-of-the-art methods on both synthetic and real-world datasets.
图像扩散模型已被适应于现实世界的视频超分辨率以解决基于GAN的方法中的过度平滑问题。然而,这些模型在维持时间一致性方面遇到困难,因为它们是在静态图像上训练的,这限制了它们有效捕捉时间动态的能力。将文本到视频(T2V)模型集成到视频超分辨率中以改进时间建模是直接的。然而,还有两个主要挑战:现实世界场景中复杂退化引起的伪影,以及由于强大的T2V模型(例如CogVideoX-5B)的强大生成能力而导致的保真度降低。为了增强恢复视频的时空质量,我们引入了名为STAR的新方法(利用T2V模型进行现实视频超分辨率的空间时间增强)。该方法通过引入局部信息增强模块(LIEM)来丰富局部细节并减轻退化伪影。此外,我们提出了一种动态频率(DF)损失来加强保真度,引导模型在不同的扩散步骤中关注不同的频率分量。大量实验表明,STAR在合成和真实世界数据集上都优于现有方法。
论文及项目相关链接
摘要
针对基于GAN的图像扩散模型在处理真实世界视频超分辨率时的过平滑问题,本文提出了一种新的方法——利用文本到视频(T2V)模型进行真实世界视频超分辨率的空间时间增强技术(STAR)。通过引入局部信息增强模块(LIEM)和动态频率(DF)损失,STAR能够增强局部细节,减轻退化伪影,同时强化保真度,使模型在扩散步骤中关注不同的频率成分。实验表明,STAR在合成和真实世界数据集上均优于现有方法。
关键见解
- 图像扩散模型已应用于真实世界视频超分辨率,以解决GAN方法中的过平滑问题。
- 这些模型在保持时间一致性方面遇到困难,因为它们基于静态图像进行训练,难以有效捕捉时间动态。
- 集成文本到视频(T2V)模型以改进时间建模是直接的,但面临复杂退化引入的伪影和强大T2V模型对保真度的妥协等挑战。
- 提出的STAR方法利用T2V模型进行真实世界视频超分辨率,实现真实空间细节和稳健的时间一致性。
- 通过引入局部信息增强模块(LIEM),STAR能够丰富局部细节并减轻退化伪影。
- 动态频率(DF)损失的引入强化了保真度,引导模型在扩散步骤中关注不同的频率成分。
点此查看论文截图
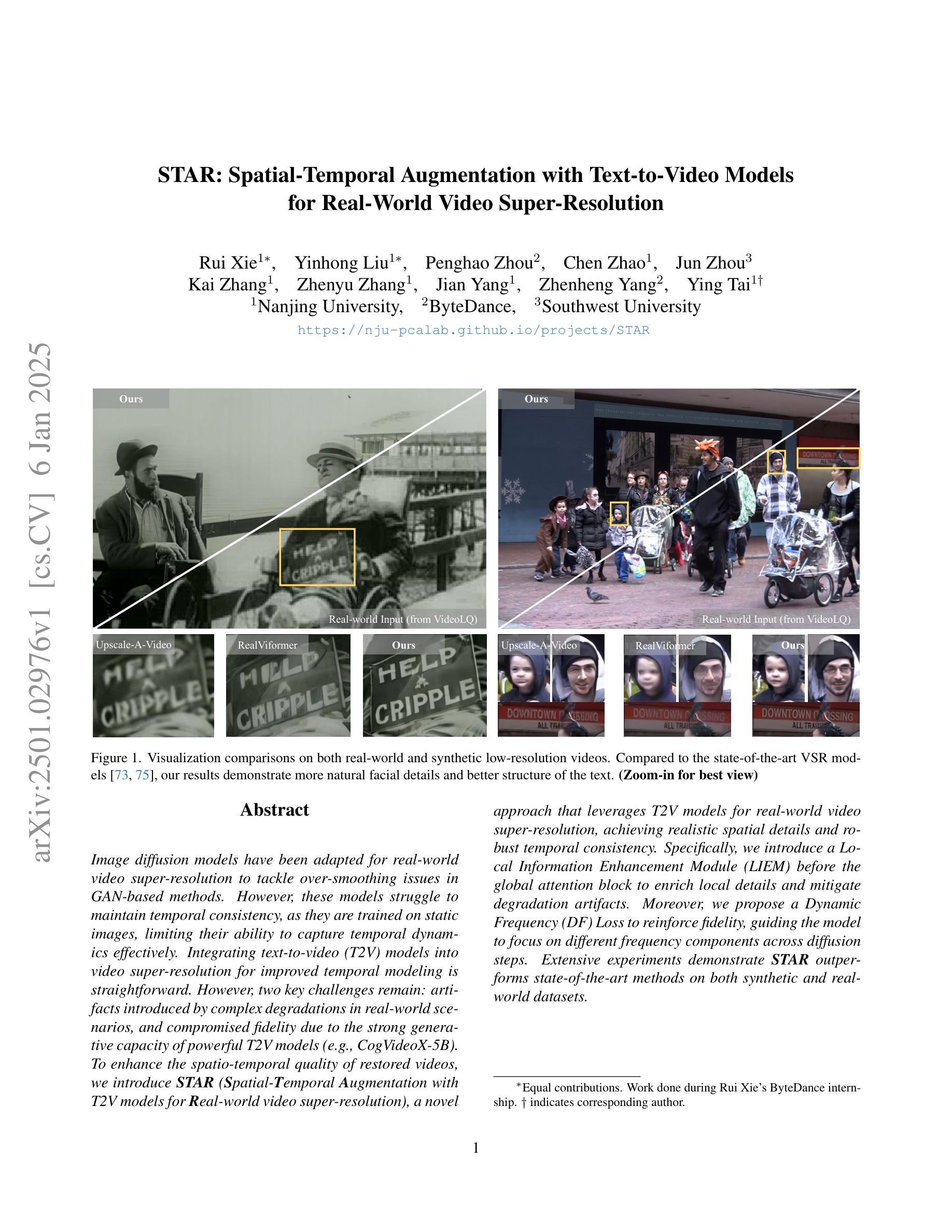
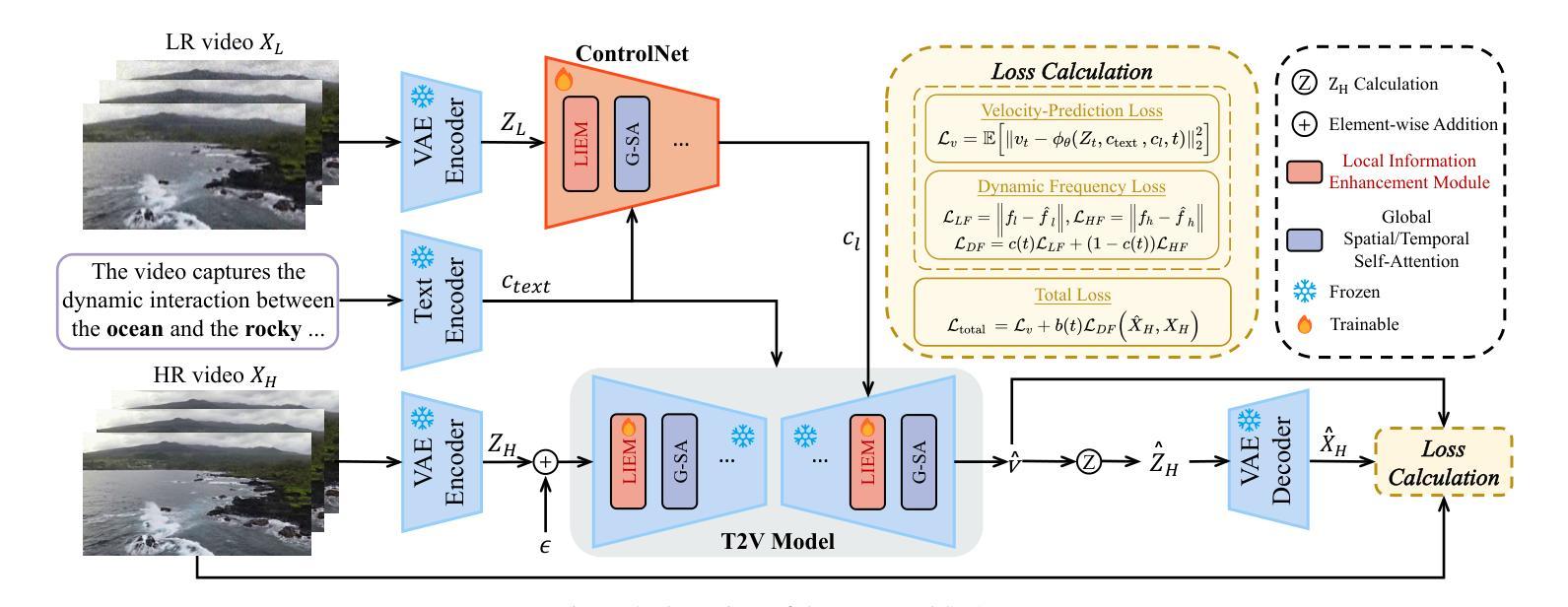
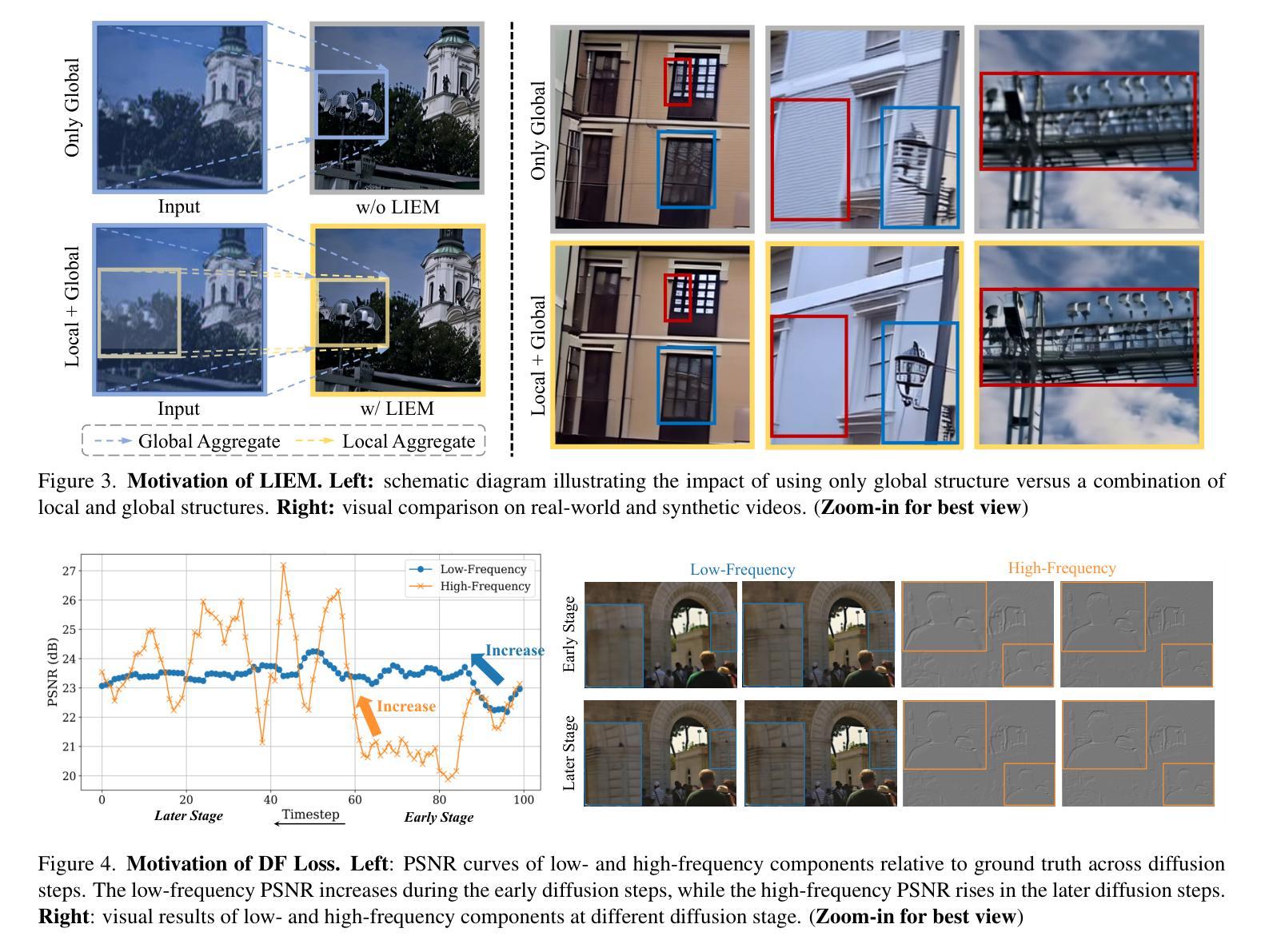
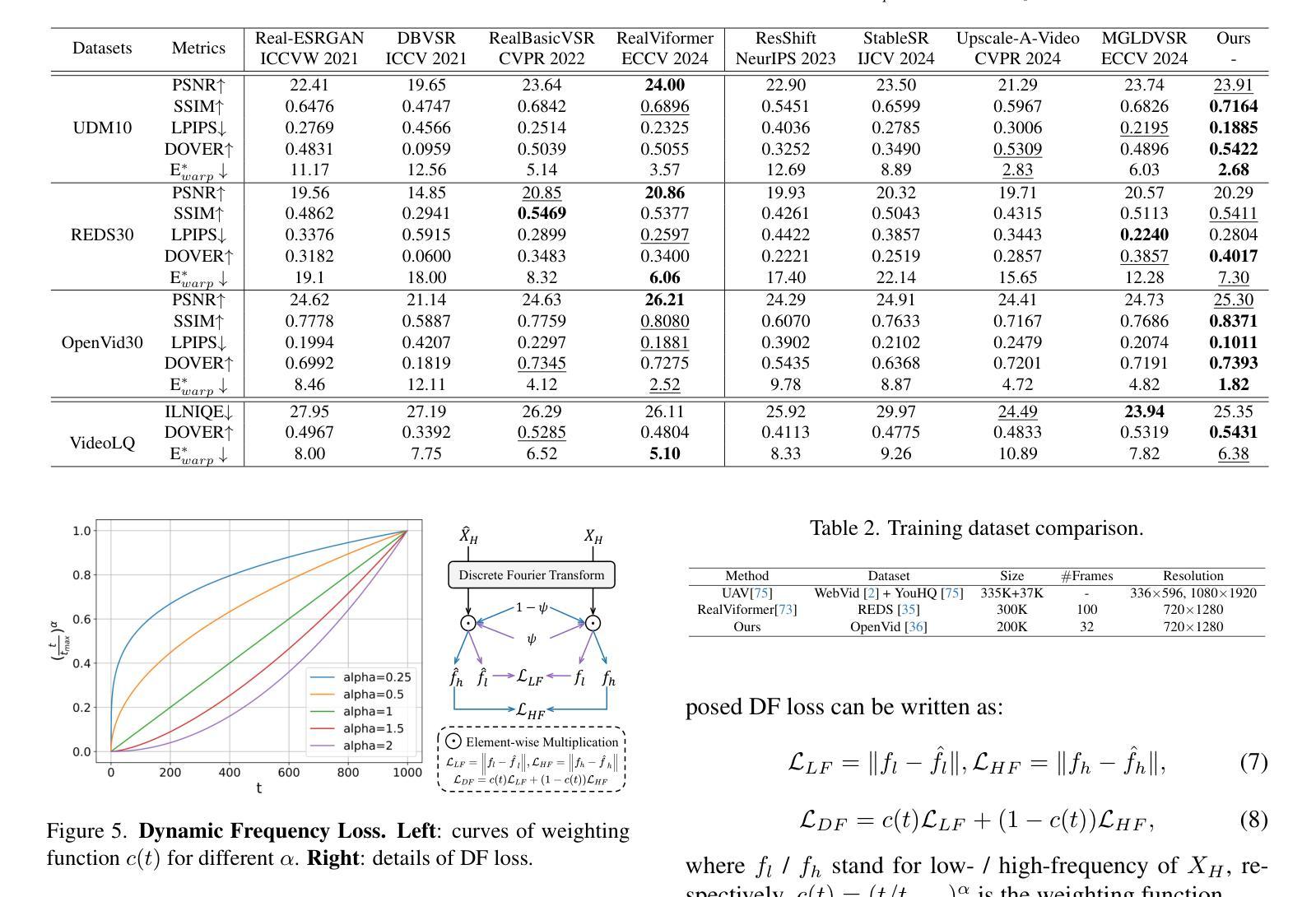
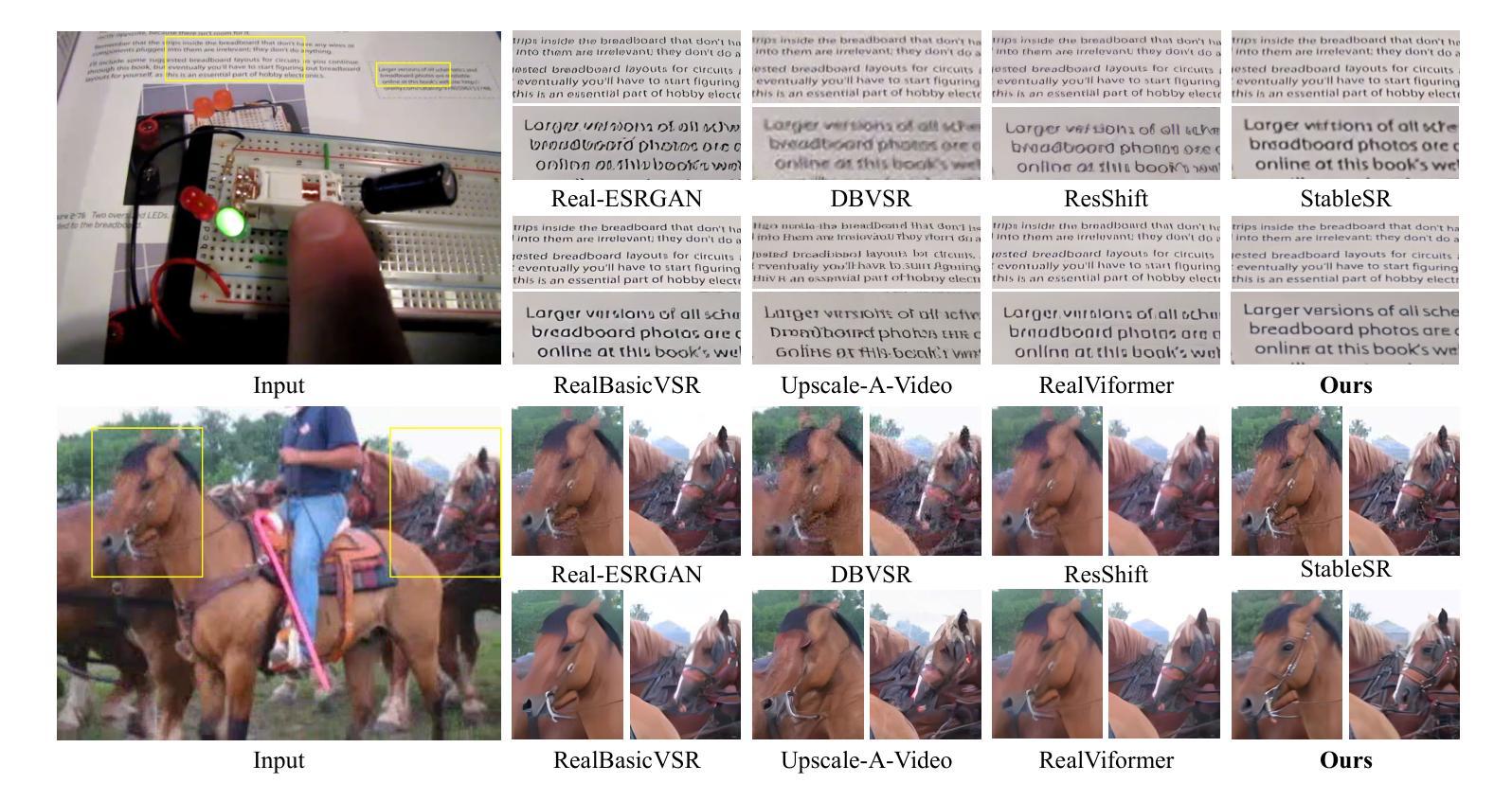
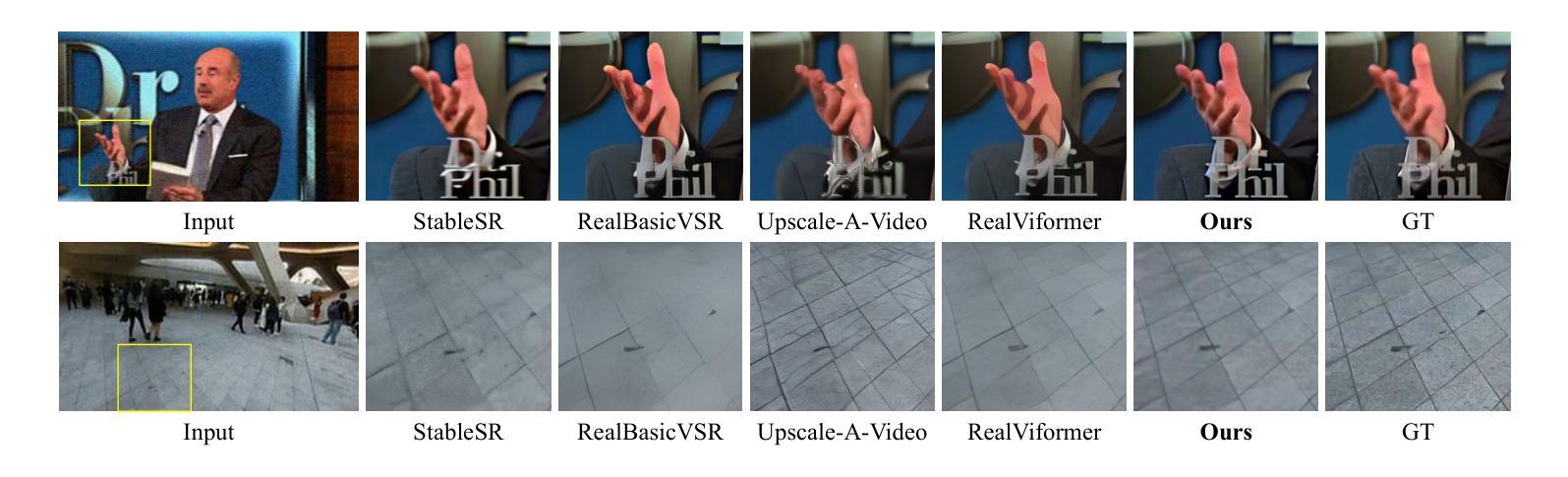
InpDiffusion: Image Inpainting Localization via Conditional Diffusion Models
Authors:Kai Wang, Shaozhang Niu, Qixian Hao, Jiwei Zhang
As artificial intelligence advances rapidly, particularly with the advent of GANs and diffusion models, the accuracy of Image Inpainting Localization (IIL) has become increasingly challenging. Current IIL methods face two main challenges: a tendency towards overconfidence, leading to incorrect predictions; and difficulty in detecting subtle tampering boundaries in inpainted images. In response, we propose a new paradigm that treats IIL as a conditional mask generation task utilizing diffusion models. Our method, InpDiffusion, utilizes the denoising process enhanced by the integration of image semantic conditions to progressively refine predictions. During denoising, we employ edge conditions and introduce a novel edge supervision strategy to enhance the model’s perception of edge details in inpainted objects. Balancing the diffusion model’s stochastic sampling with edge supervision of tampered image regions mitigates the risk of incorrect predictions from overconfidence and prevents the loss of subtle boundaries that can result from overly stochastic processes. Furthermore, we propose an innovative Dual-stream Multi-scale Feature Extractor (DMFE) for extracting multi-scale features, enhancing feature representation by considering both semantic and edge conditions of the inpainted images. Extensive experiments across challenging datasets demonstrate that the InpDiffusion significantly outperforms existing state-of-the-art methods in IIL tasks, while also showcasing excellent generalization capabilities and robustness.
随着人工智能的快速发展,尤其是生成对抗网络(GANs)和扩散模型的兴起,图像修复定位(IIL)的准确性越来越受到挑战。当前的IIL方法面临两个主要挑战:一是过于自信,导致预测错误;二是难以检测修复图像中细微的篡改边界。针对这些问题,我们提出了一种新的范式,将IIL视为利用扩散模型的条件性掩膜生成任务。我们的方法InpDiffusion,利用结合图像语义条件增强的去噪过程,逐步优化预测。在去噪过程中,我们采用边缘条件,并引入一种新的边缘监督策略,增强模型对修复物体边缘细节的认知。平衡扩散模型的随机采样与篡改图像区域的边缘监督,减轻了因过于自信而导致预测错误的风险,并防止了因过于随机的过程而丢失细微的边界。此外,我们还提出了一种创新性的双流多尺度特征提取器(DMFE),用于提取多尺度特征,通过考虑修复图像的语义和边缘条件来增强特征表示。在具有挑战性的数据集上进行的广泛实验表明,InpDiffusion在IIL任务上显著优于现有最先进的方法,同时展示了出色的泛化能力和稳健性。
论文及项目相关链接
Summary
随着人工智能尤其是生成对抗网络(GANs)和扩散模型的快速发展,图像修复定位(IIL)的准确性面临挑战。针对当前IIL方法存在的问题,提出了一种新的基于扩散模型的条件掩膜生成任务范式。通过图像语义条件的融合,逐步优化预测结果,并在去噪过程中采用边缘条件和新型边缘监督策略,提高了模型对修复对象边缘细节的感知能力。该方法平衡了扩散模型的随机采样与篡改图像区域的边缘监督,降低了因过度自信而导致的错误预测风险,避免了因过于随机而丢失的细微边界。此外,还提出了双流多尺度特征提取器(DMFE),用于提取多尺度特征,提高修复图像语义和边缘条件的考虑。实验证明,该方法在IIL任务上显著优于现有先进技术,具有良好的泛化能力和鲁棒性。
Key Takeaways
- 人工智能的进步尤其是GANs和扩散模型的崛起使得图像修复定位(IIL)的准确性面临挑战。
- 当前IIL方法面临两大挑战:过度自信的预测导致错误预测和难以检测修复图像中的细微篡改边界。
- 提出了一种新的基于扩散模型的条件掩膜生成任务范式来处理IIL问题。
- 通过融合图像语义条件逐步优化预测结果,并在去噪过程中采用边缘条件和新型边缘监督策略来提高模型性能。
- 平衡扩散模型的随机采样与篡改图像区域的边缘监督以降低错误预测风险并避免丢失细微边界。
- 引入双流多尺度特征提取器(DMFE)以提高多尺度特征的提取和对修复图像语义和边缘条件的考虑。
点此查看论文截图
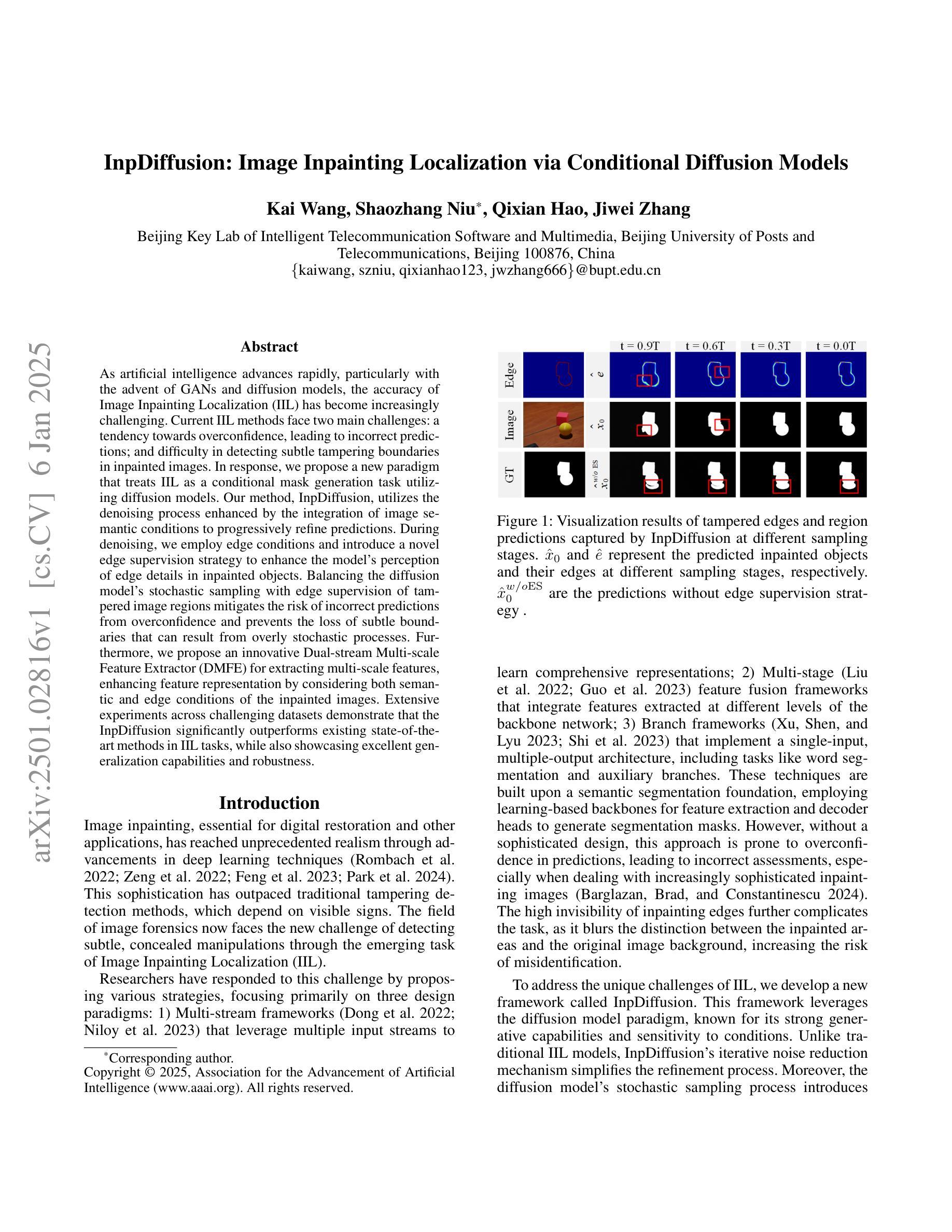
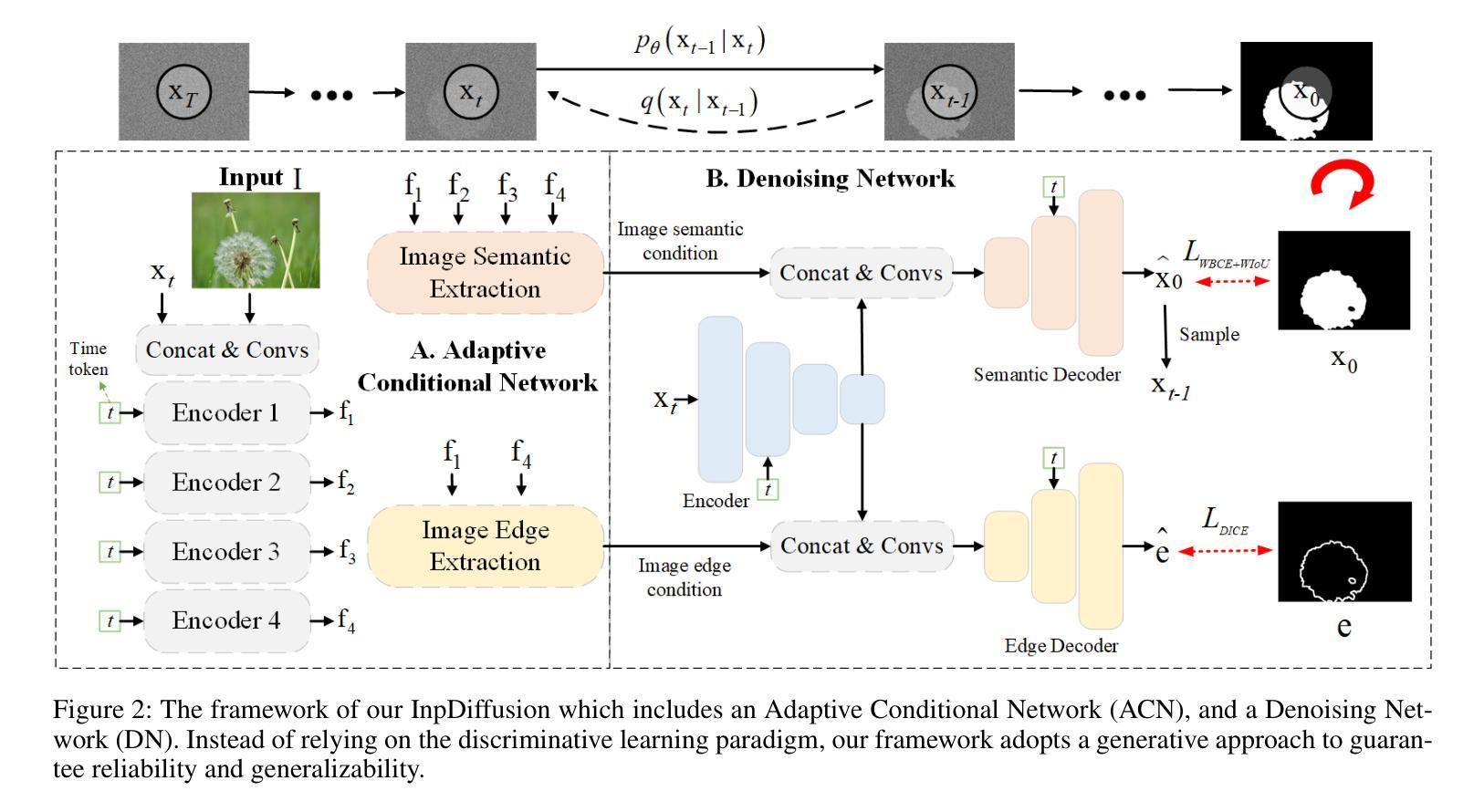
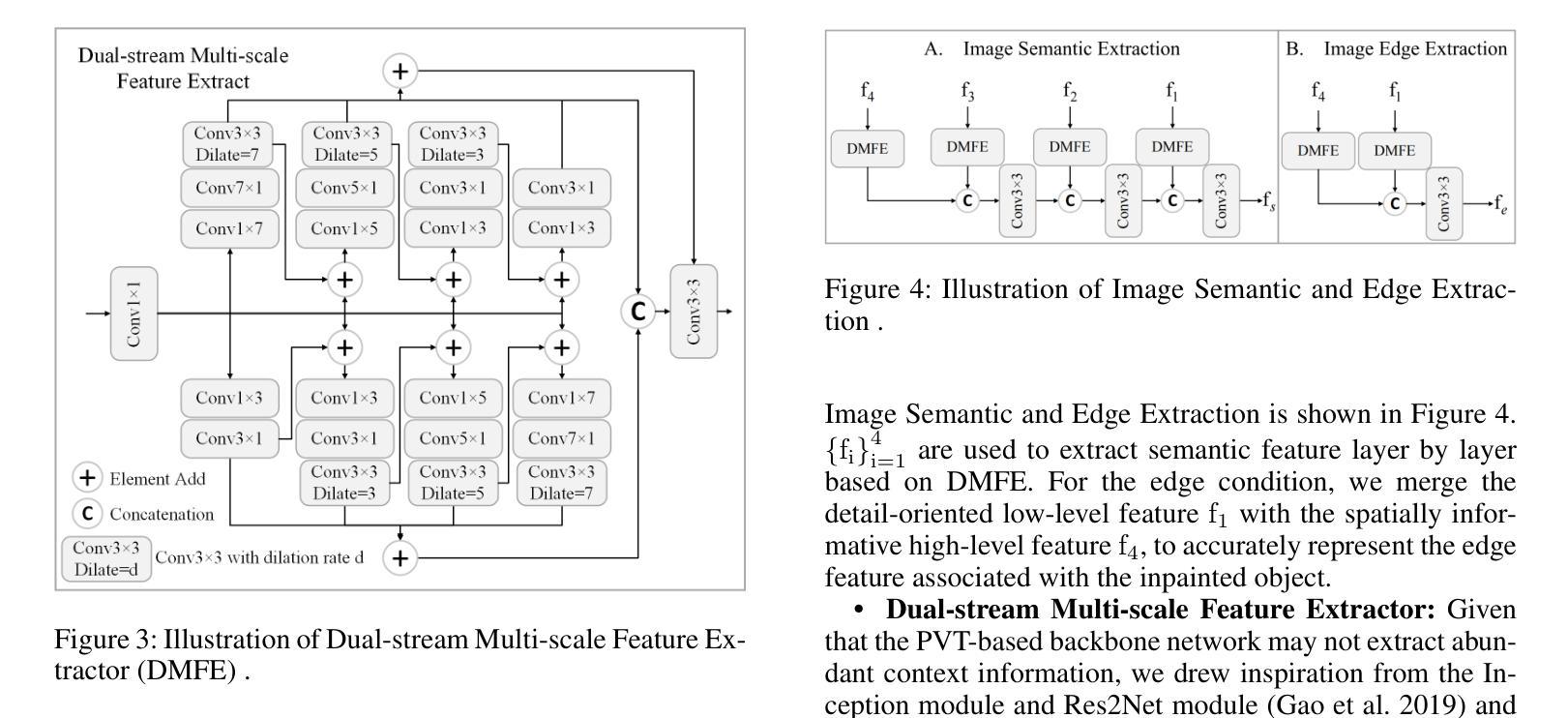
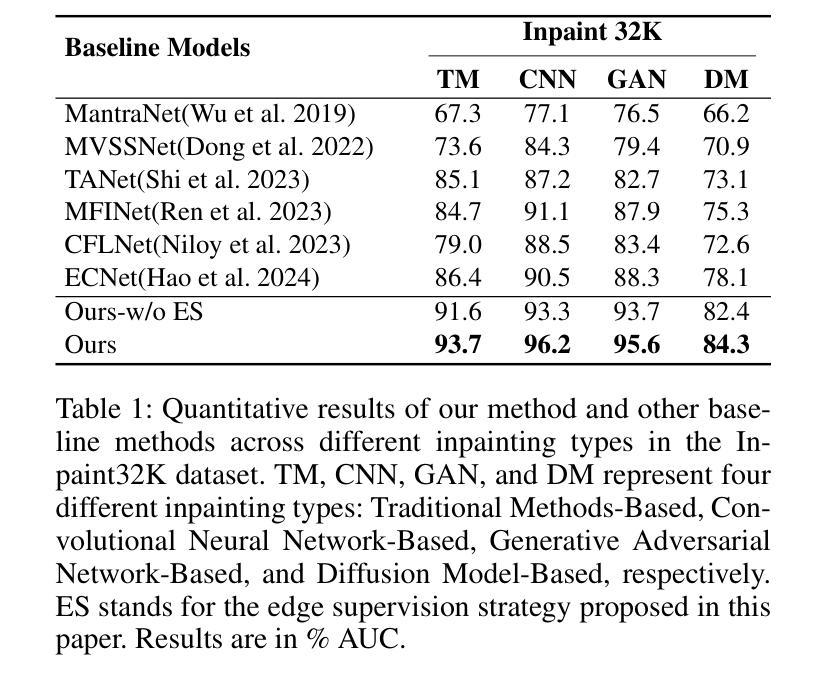
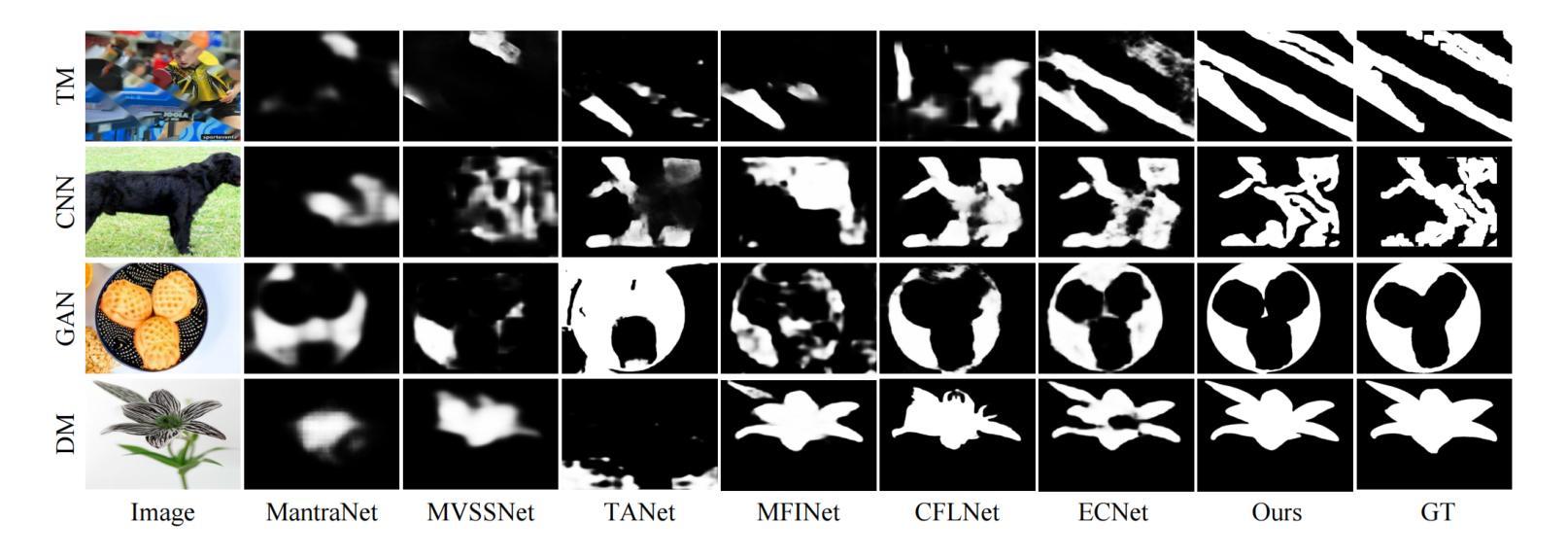
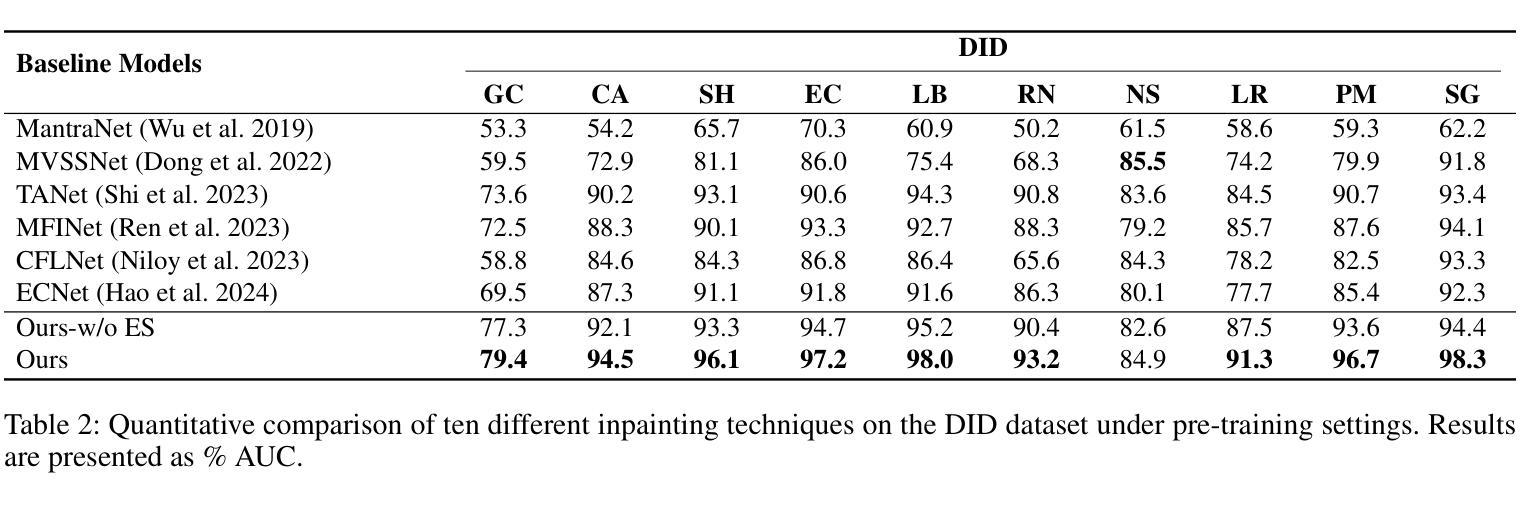
SRAGAN: Saliency Regularized and Attended Generative Adversarial Network for Chinese Ink-wash Painting Generation
Authors:Xiang Gao, Yuqi Zhang
Recent style transfer problems are still largely dominated by Generative Adversarial Network (GAN) from the perspective of cross-domain image-to-image (I2I) translation, where the pivotal issue is to learn and transfer target-domain style patterns onto source-domain content images. This paper handles the problem of translating real pictures into traditional Chinese ink-wash paintings, i.e., Chinese ink-wash painting style transfer. Though a wide range of I2I models tackle this problem, a notable challenge is that the content details of the source image could be easily erased or corrupted due to the transfer of ink-wash style elements. To remedy this issue, we propose to incorporate saliency detection into the unpaired I2I framework to regularize image content, where the detected saliency map is utilized from two aspects: (\romannumeral1) we propose saliency IOU (SIOU) loss to explicitly regularize object content structure by enforcing saliency consistency before and after image stylization; (\romannumeral2) we propose saliency adaptive normalization (SANorm) which implicitly enhances object structure integrity of the generated paintings by dynamically injecting image saliency information into the generator to guide stylization process. Besides, we also propose saliency attended discriminator which harnesses image saliency information to focus generative adversarial attention onto the drawn objects, contributing to generating more vivid and delicate brush strokes and ink-wash textures. Extensive qualitative and quantitative experiments demonstrate superiority of our approach over related advanced image stylization methods in both GAN and diffusion model paradigms.
从跨域图像到图像的翻译角度来看,最近的风格迁移问题在很大程度上仍然受到生成对抗网络(GAN)的主导。其中关键的问题是学习和将目标域的样式模式转移到源域的内容图像上。本文针对将真实图片翻译成传统水墨画的问题,即水墨画风格迁移。尽管有许多I2I模型解决了这个问题,但一个显著的挑战是源图像的内容细节在转移水墨风格元素时可能会被轻易擦除或破坏。为了解决这个问题,我们提出将显著性检测融入非配对的I2I框架以规范图像内容。显著性检测图从两个方面被利用:(1)我们提出了显著性IOU(SIOU)损失,通过强制显著性一致性来明确规范对象内容结构,从而在图像风格化前后实施约束;(2)我们提出了显著性自适应归一化(SANorm),通过动态将图像显著性信息注入生成器来隐式增强生成画作的对象结构完整性,从而指导风格化过程。此外,我们还提出了显著性关注鉴别器,它利用图像显著性信息将生成对抗的注意力集中在绘制对象上,有助于生成更生动、更精细的笔触和水墨纹理。大量的定性和定量实验表明,我们的方法在GAN和扩散模型范式中,相对于相关的先进图像风格化方法具有优越性。
论文及项目相关链接
PDF 34 pages, 15 figures
Summary
在跨域图像到图像(I2I)的翻译中,风格迁移问题仍然主要由生成对抗网络(GAN)主导。本文处理将真实图片翻译成传统水墨画的问题,即水墨画风格迁移。针对因水墨风格元素转移而容易擦除或破坏源图像内容细节的问题,本文提出将显著性检测融入非配对I2I框架来规范图像内容。使用显著性检测地图,我们提出两种方法来增强图像内容结构完整性:一是显著性IOU(SIOU)损失,通过强制风格化前后的显著性一致性来显式地规范对象内容结构;二是显著性自适应归一化(SANorm),它通过动态地将图像显著性信息注入生成器以指导风格化过程,隐式地增强对象结构完整性。此外,我们还提出了显著性关注鉴别器,利用图像显著性信息将生成对抗注意集中在绘画对象上,有助于生成更生动和精致的水墨纹理和笔触。实验证明,我们的方法在GAN和扩散模型范式下的图像风格化方法上具有优势。
Key Takeaways
- 最新风格迁移问题仍由GAN在跨域图像到图像翻译的角度主导,重点在于学习并转移目标域的风格模式至源域内容图像。
- 本文处理将真实图片转换为传统水墨画的问题,即水墨画风格迁移。
- 在水墨画风格迁移中,源图像的内容细节容易因风格元素转移而被擦除或破坏。
- 为解决此问题,引入了显著性检测机制来规范图像内容,使用显著性检测地图提出SIOU损失和SANorm方法,以增强图像内容结构的完整性。
- 提出的显著性关注鉴别器利用图像显著性信息集中生成对抗注意在绘画对象上,有助于生成更生动和精致的水墨纹理和笔触。
点此查看论文截图
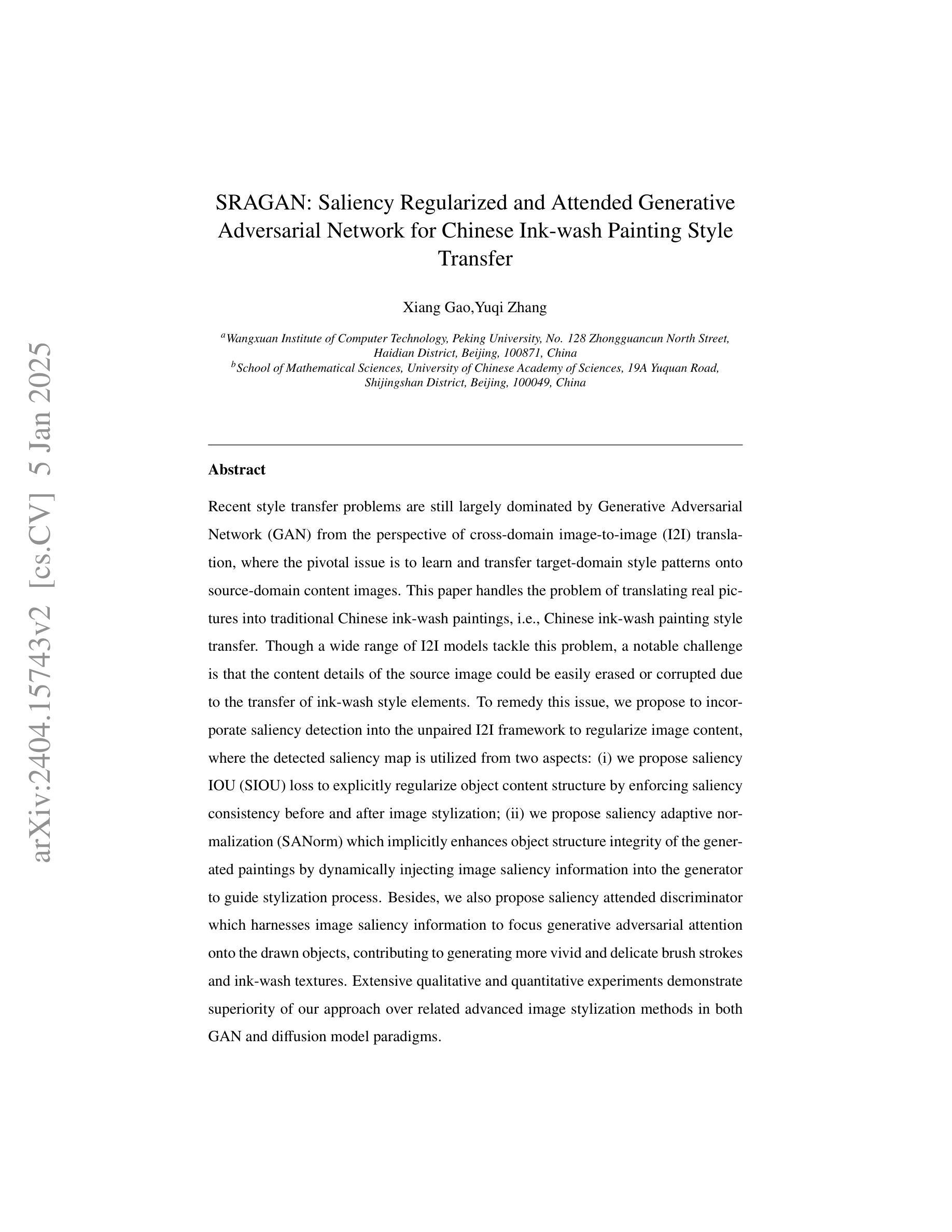
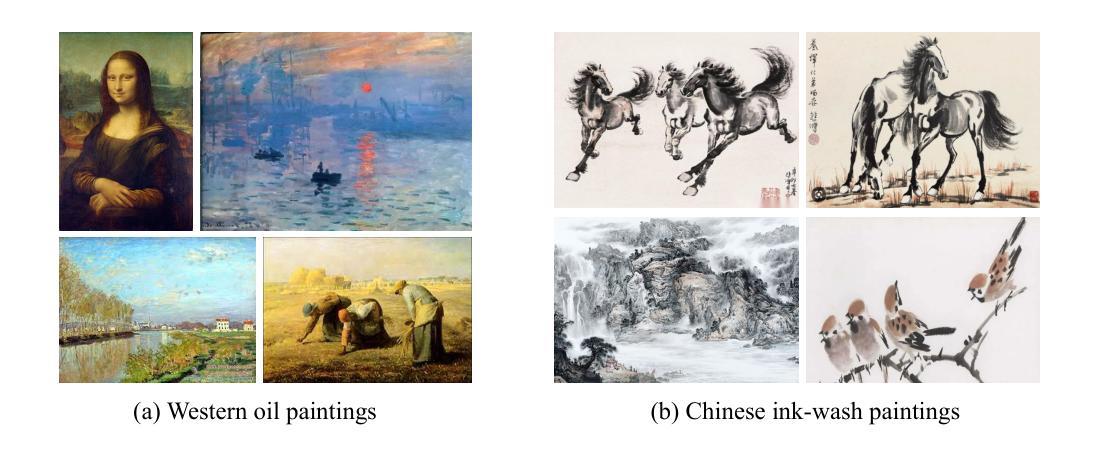
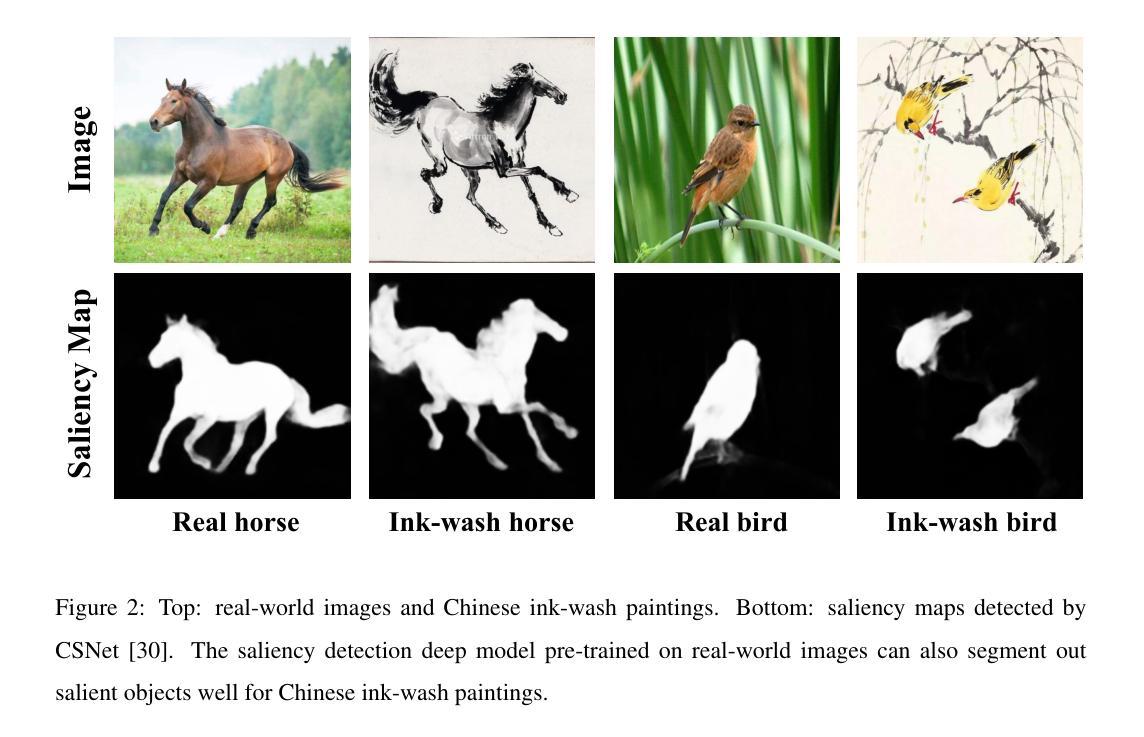