⚠️ 以下所有内容总结都来自于 大语言模型的能力,如有错误,仅供参考,谨慎使用
🔴 请注意:千万不要用于严肃的学术场景,只能用于论文阅读前的初筛!
💗 如果您觉得我们的项目对您有帮助 ChatPaperFree ,还请您给我们一些鼓励!⭐️ HuggingFace免费体验
2025-01-08 更新
Plasma-CycleGAN: Plasma Biomarker-Guided MRI to PET Cross-modality Translation Using Conditional CycleGAN
Authors:Yanxi Chen, Yi Su, Celine Dumitrascu, Kewei Chen, David Weidman, Richard J Caselli, Nicholas Ashton, Eric M Reiman, Yalin Wang
Cross-modality translation between MRI and PET imaging is challenging due to the distinct mechanisms underlying these modalities. Blood-based biomarkers (BBBMs) are revolutionizing Alzheimer’s disease (AD) detection by identifying patients and quantifying brain amyloid levels. However, the potential of BBBMs to enhance PET image synthesis remains unexplored. In this paper, we performed a thorough study on the effect of incorporating BBBM into deep generative models. By evaluating three widely used cross-modality translation models, we found that BBBMs integration consistently enhances the generative quality across all models. By visual inspection of the generated results, we observed that PET images generated by CycleGAN exhibit the best visual fidelity. Based on these findings, we propose Plasma-CycleGAN, a novel generative model based on CycleGAN, to synthesize PET images from MRI using BBBMs as conditions. This is the first approach to integrate BBBMs in conditional cross-modality translation between MRI and PET.
跨模态MRI和PET成像之间的转换是一项挑战,因为它们基于不同的机制。血液生物标志物(BBBMs)正在通过识别患者和量化脑淀粉样蛋白水平来革新阿尔茨海默病(AD)的检测。然而,BBBMs增强PET图像合成的潜力尚未被探索。在本文中,我们对将BBBM纳入深度生成模型的影响进行了彻底的研究。通过评估三种常用的跨模态翻译模型,我们发现BBBM的整合在所有模型中均提高了生成质量。通过对生成结果的视觉检查,我们观察到CycleGAN生成的PET图像具有最佳视觉保真度。基于这些发现,我们提出了基于CycleGAN的新型生成模型Plasma-CycleGAN,利用BBBMs作为条件合成PET图像。这是第一个将BBBMs整合到MRI和PET之间的条件跨模态翻译中的方法。
论文及项目相关链接
PDF Accepted by ISBI 2025
Summary
本文探索了将血液生物标志物(BBBMs)融入深度生成模型以增强PET图像合成的效果。研究发现在三种常用的跨模态转换模型中,融入BBBMs可显著提高生成质量。通过对比,使用CycleGAN生成的PET图像具有最佳视觉保真度。基于此,提出了结合BBBMs条件的基于CycleGAN的新型生成模型Plasma-CycleGAN,用于从MRI合成PET图像。
Key Takeaways
- 跨模态翻译在MRI和PET成像之间具有挑战性,主要由于这两种模式的底层机制不同。
- 血液生物标志物(BBBMs)正在通过识别患者和量化大脑淀粉样蛋白水平来革新阿尔茨海默病(AD)的检测。
- BBBMs在深度生成模型中的融入能够普遍提高跨模态转换模型的生成质量。
- 使用CycleGAN生成的PET图像具有最佳视觉保真度。
- 提出了首个结合BBBMs条件的跨MRI和PET模态转换的生成模型——Plasma-CycleGAN。
- Plasma-CycleGAN模型利用BBBMs作为条件,能够从MRI合成PET图像。
点此查看论文截图
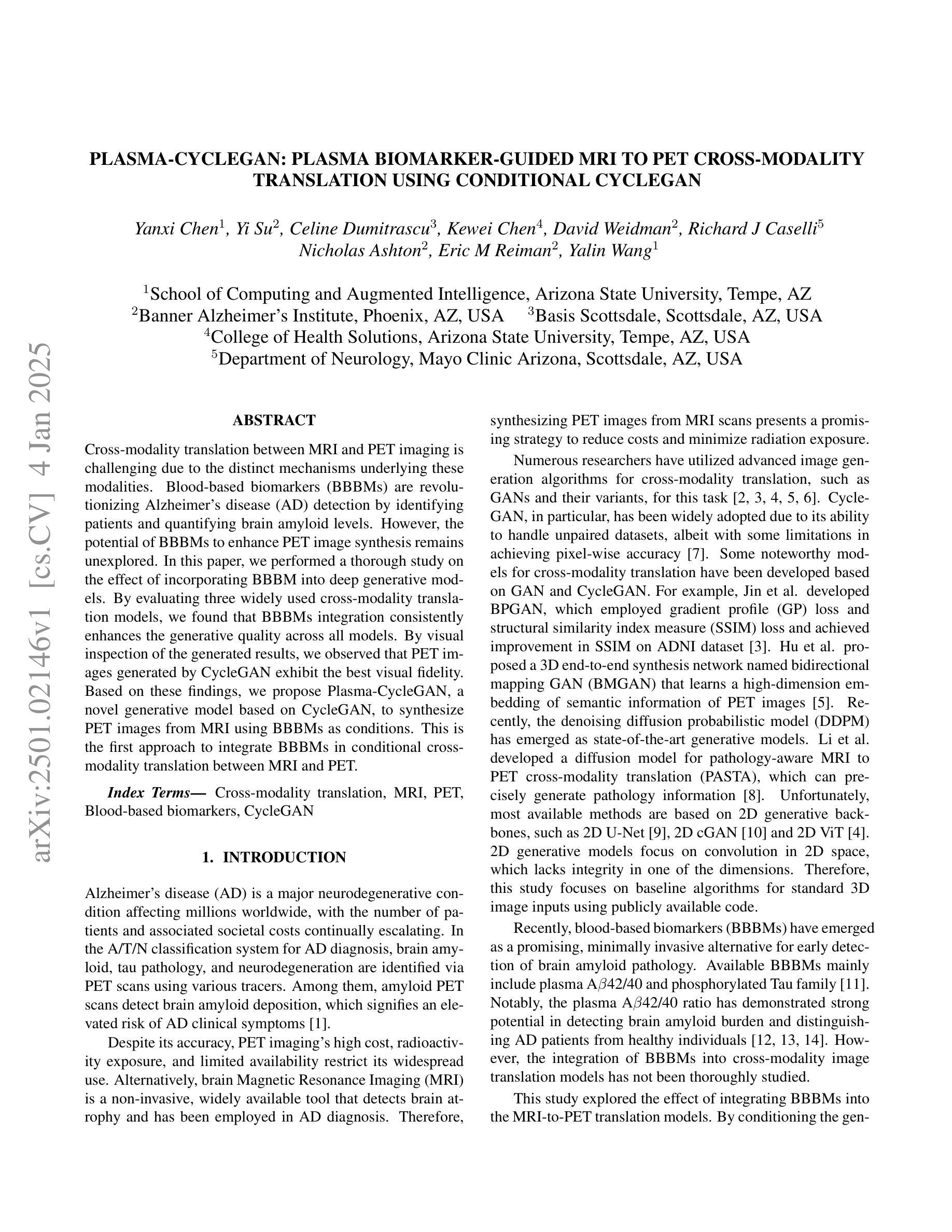
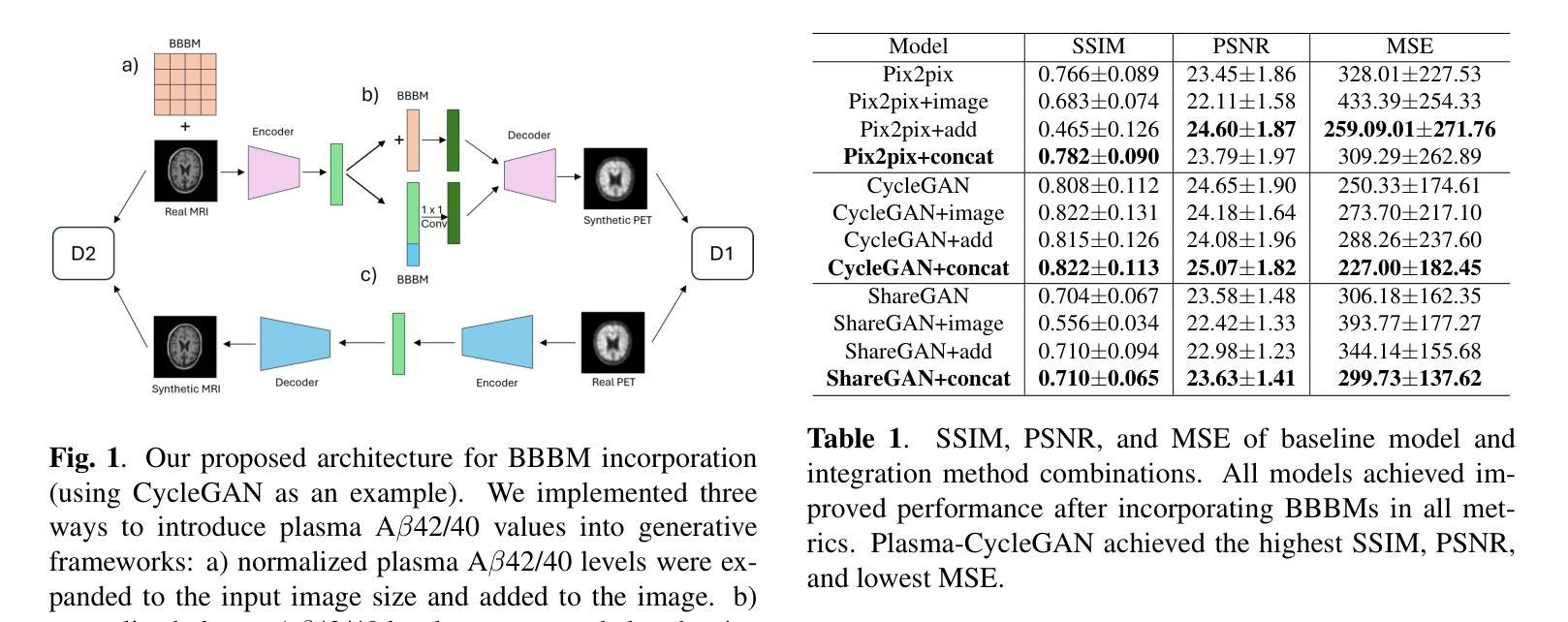
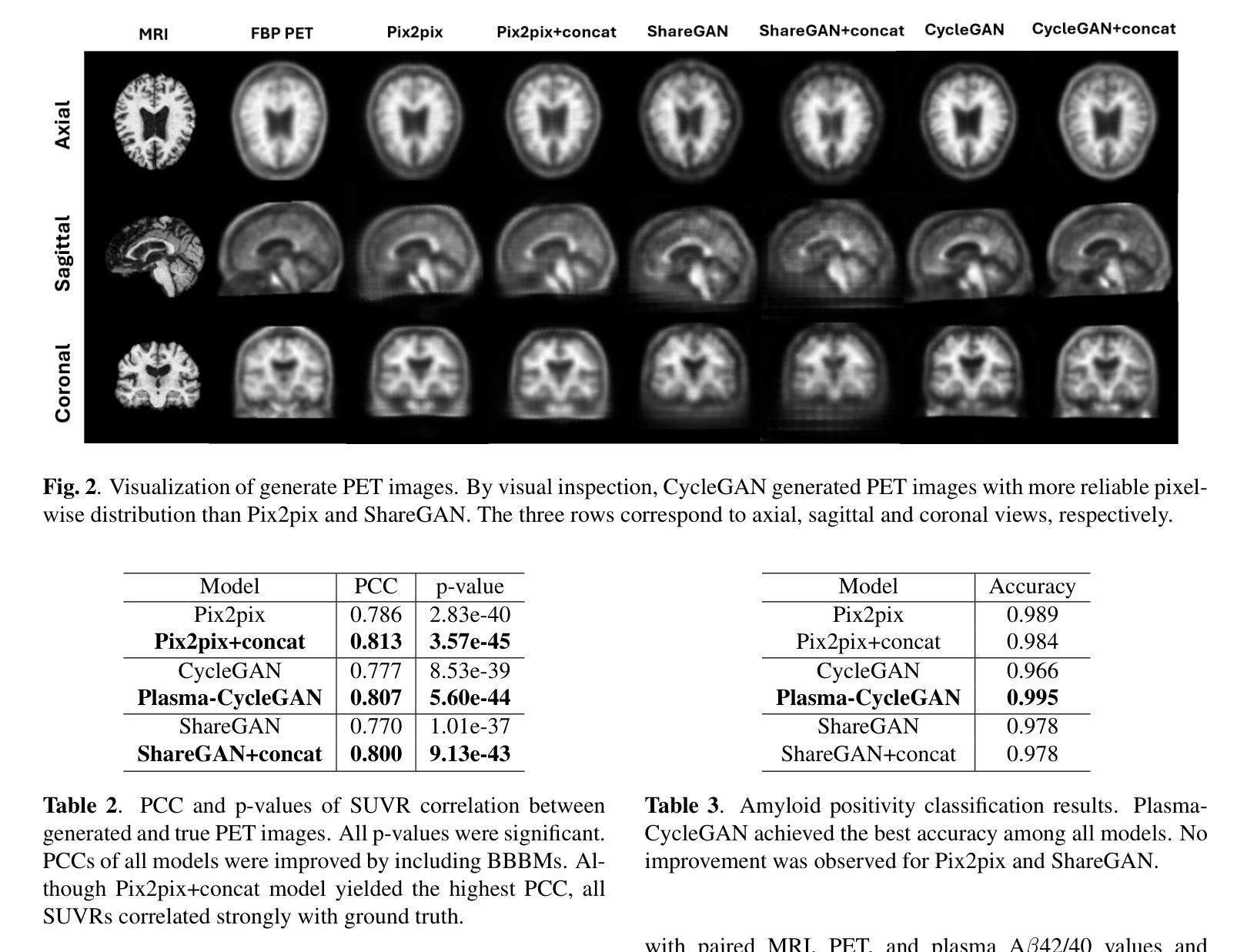
Make Imagination Clearer! Stable Diffusion-based Visual Imagination for Multimodal Machine Translation
Authors:Andong Chen, Yuchen Song, Kehai Chen, Muyun Yang, Tiejun Zhao, Min Zhang
Visual information has been introduced for enhancing machine translation (MT), and its effectiveness heavily relies on the availability of large amounts of bilingual parallel sentence pairs with manual image annotations. In this paper, we introduce a stable diffusion-based imagination network into a multimodal large language model (MLLM) to explicitly generate an image for each source sentence, thereby advancing the multimodel MT. Particularly, we build heuristic human feedback with reinforcement learning to ensure the consistency of the generated image with the source sentence without the supervision of image annotation, which breaks the bottleneck of using visual information in MT. Furthermore, the proposed method enables imaginative visual information to be integrated into large-scale text-only MT in addition to multimodal MT. Experimental results show that our model significantly outperforms existing multimodal MT and text-only MT, especially achieving an average improvement of more than 14 BLEU points on Multi30K multimodal MT benchmarks.
视觉信息已被引入以增强机器翻译(MT)的效果,其有效性在很大程度上依赖于大量带有手动图像注释的双语并行句子对的使用。在本文中,我们将基于稳定扩散的想象网络引入多模态大型语言模型(MLLM)中,为每种源语言句子明确生成对应的图像,从而推动多模态机器翻译的进步。特别地,我们借助强化学习与启发式人工反馈来确保生成的图像与源语言句子的一致性,无需图像注释的监督,从而打破了机器翻译中使用视觉信息的瓶颈。此外,该方法允许将想象的视觉信息整合到大型纯文本机器翻译模型以及多模态机器翻译模型中。实验结果表明,我们的模型在现有多模态机器翻译和纯文本机器翻译上表现显著优势,特别是在Multi30K多模态机器翻译基准测试上平均提高了超过14个BLEU点。
论文及项目相关链接
PDF Work in progress
摘要
视觉信息被引入以增强机器翻译(MT),其有效性很大程度上依赖于大量双语平行句子对和手动图像注释的可用性。在本文中,我们将基于稳定扩散的想象网络引入多模态大型语言模型(MLLM)中,为每个源句子生成对应的图像,从而促进多模态机器翻译的发展。特别是,我们利用启发式人类反馈和强化学习来确保生成的图像与源句子的一致性,无需图像注释的监督,从而打破了机器翻译中使用视觉信息的瓶颈。此外,该方法不仅适用于多模态机器翻译,还能将想象性视觉信息集成到大规模纯文本机器翻译中。实验结果表明,我们的模型在多模态机器翻译方面显著优于现有模型,特别是在Multi30K多模态机器翻译基准测试中,平均提高了超过14个BLEU点。
要点
- 引入视觉信息以增强机器翻译效果。
- 提出基于稳定扩散的想象网络的多模态语言模型方法,为每个源句子生成相应的图像。
- 使用启发式人类反馈和强化学习确保生成的图像与源句子的一致性,无需图像注释监督。
- 该方法打破机器翻译使用视觉信息的瓶颈,并适用于多模态和纯文本机器翻译。
- 实验证明该模型在多模态机器翻译方面表现优异。
- 在Multi30K基准测试中,模型平均提高了超过14个BLEU点。
- 该方法对未来机器翻译的发展具有重要意义。
点此查看论文截图
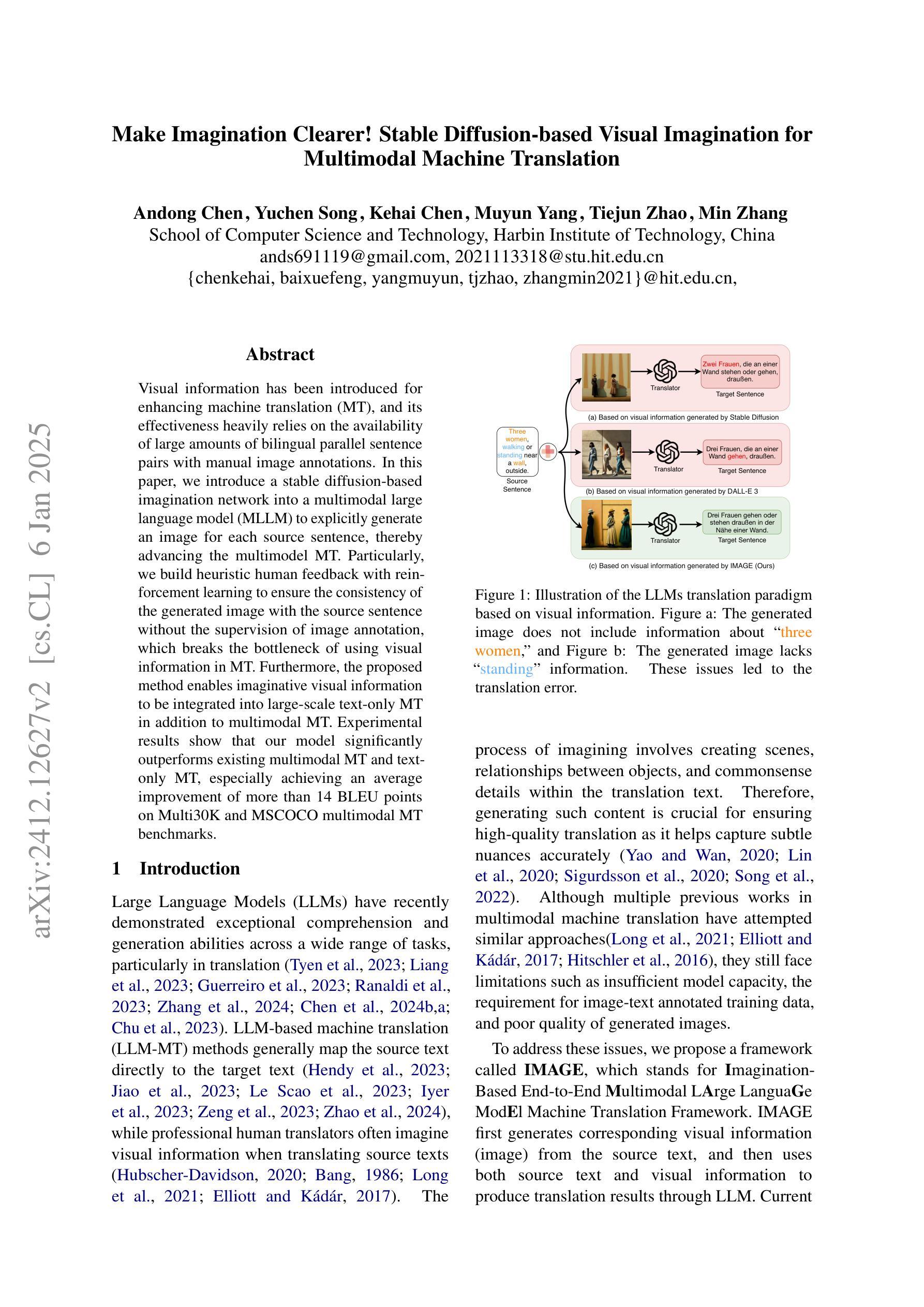
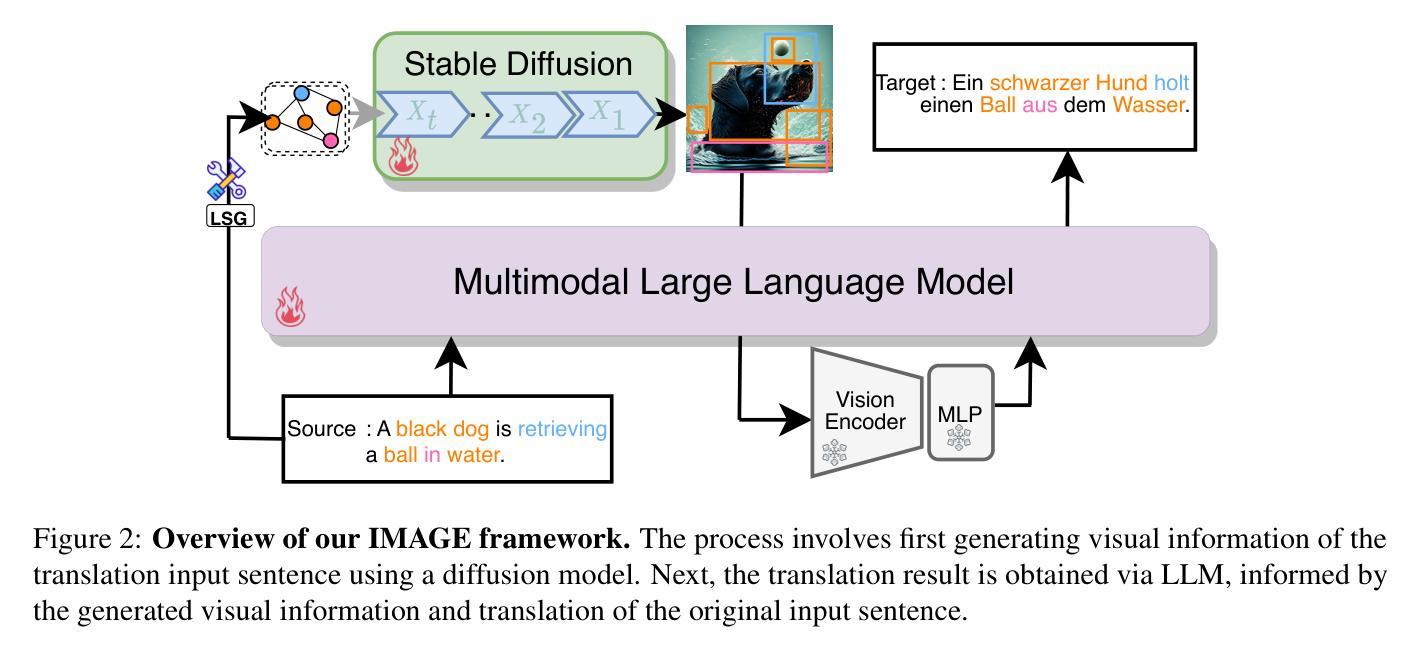
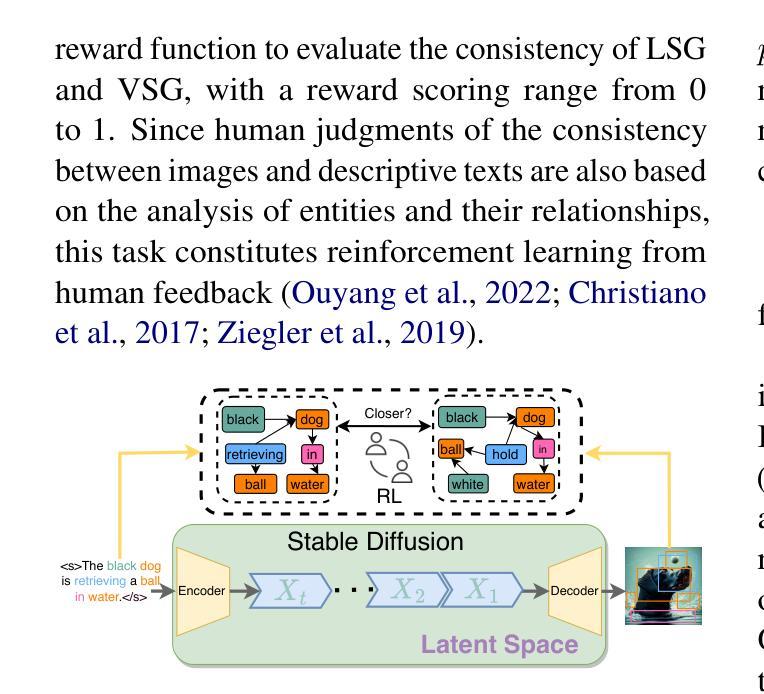
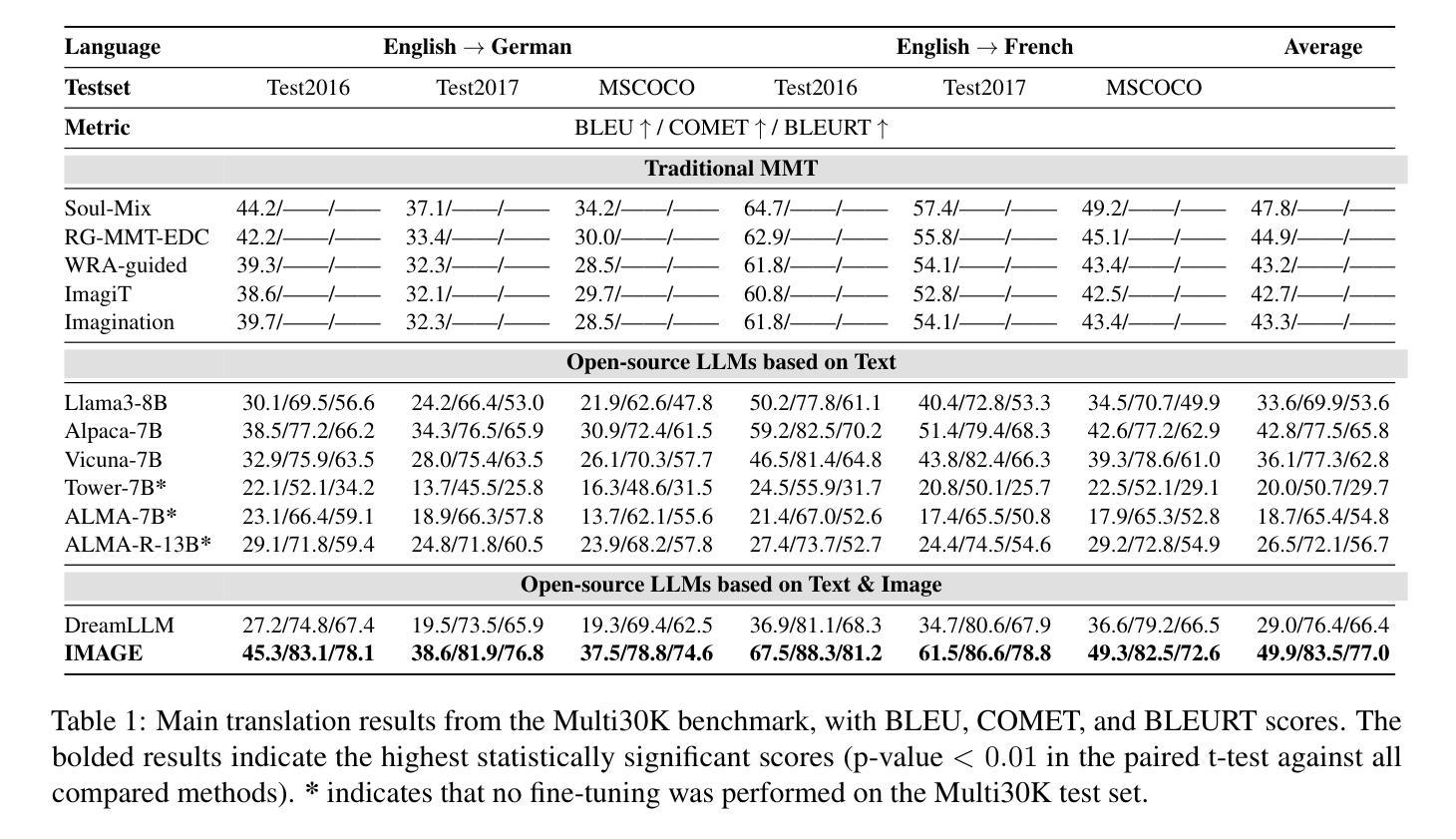
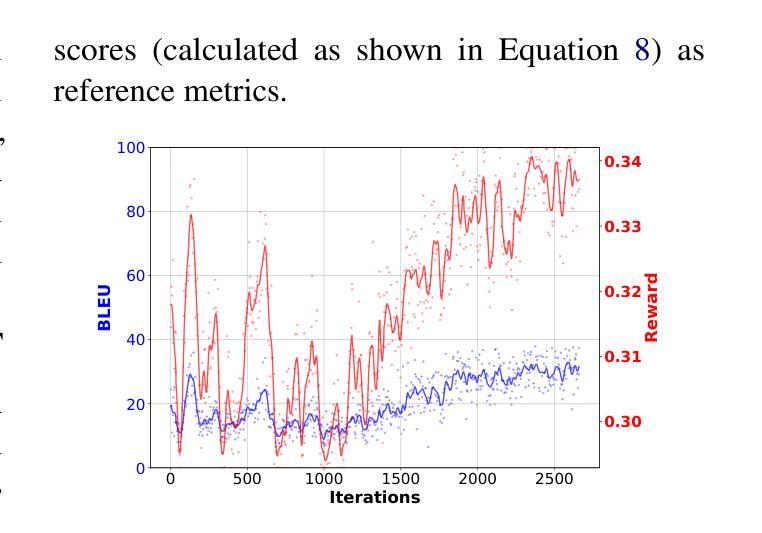
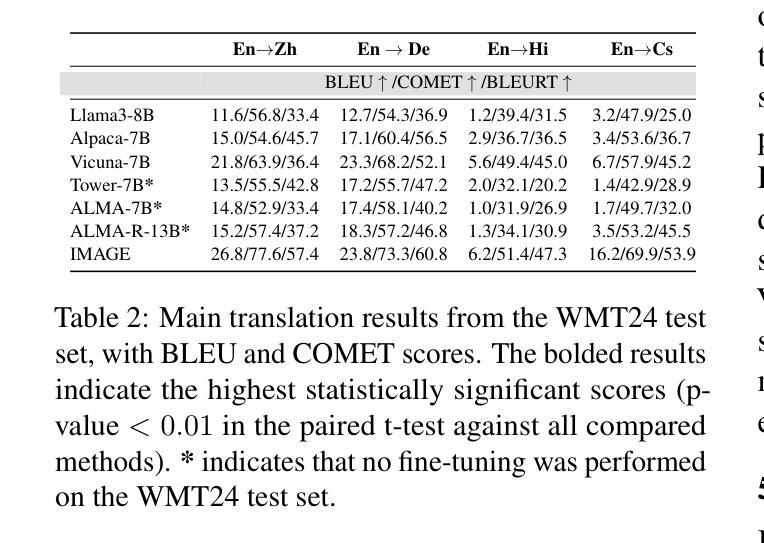
Advancing Neural Network Performance through Emergence-Promoting Initialization Scheme
Authors:Johnny Jingze Li, Vivek Kurien George, Gabriel A. Silva
Emergence in machine learning refers to the spontaneous appearance of complex behaviors or capabilities that arise from the scale and structure of training data and model architectures, despite not being explicitly programmed. We introduce a novel yet straightforward neural network initialization scheme that aims at achieving greater potential for emergence. Measuring emergence as a kind of structural nonlinearity, our method adjusts the layer-wise weight scaling factors to achieve higher emergence values. This enhancement is easy to implement, requiring no additional optimization steps for initialization compared to GradInit. We evaluate our approach across various architectures, including MLP and convolutional architectures for image recognition and transformers for machine translation. We demonstrate substantial improvements in both model accuracy and training speed, with and without batch normalization. The simplicity, theoretical innovation, and demonstrable empirical advantages of our method make it a potent enhancement to neural network initialization practices. These results suggest a promising direction for leveraging emergence to improve neural network training methodologies. Code is available at: https://github.com/johnnyjingzeli/EmergenceInit.
机器学习中的涌现现象指的是由于训练数据和模型架构的规模和结构,即使没有明确的编程设定,也会自发出现复杂的行为或能力。我们提出了一种新型且直观神经网络初始化方案,旨在实现更大的涌现潜力。我们将涌现作为一种结构非线性进行测量,通过调整逐层权重缩放因子来实现更高的涌现值。这种增强措施易于实现,与GradInit相比,无需为初始化增加额外的优化步骤。我们在各种架构上评估了我们的方法,包括用于图像识别的MLP和卷积架构以及用于机器翻译的变压器。我们在有和无批量标准化的情况下,都展示了模型精度和训练速度的实质性改进。我们方法的简单性、理论创新和明显的经验优势使其成为神经网络初始化实践的强大增强措施。这些结果表明,利用涌现现象改进神经网络训练方法是很有前景的方向。相关代码可在https://github.com/johnnyjingzeli/EmergenceInit找到。
论文及项目相关链接
Summary:本文介绍了一种新型神经网络初始化方案,旨在实现更大的涌现潜力。通过测量结构非线性作为涌现的一种度量,该方法通过调整逐层权重缩放因子来实现更高的涌现值。该方法易于实现,与GradInit相比无需额外的优化步骤。在多种架构上进行了评估,包括MLP和卷积架构用于图像识别以及变压器用于机器翻译,证明该方法在提高模型精度和训练速度方面都有显著改进。
Key Takeaways:
- 文章中描述了一种新的神经网络初始化方案,旨在提高涌现潜力。
- 通过调整逐层权重缩放因子,实现了更高的涌现值。
- 该方法简单,与现有初始化方法相比,无需额外的优化步骤。
- 在多种神经网络架构上进行了评估,包括MLP、卷积架构和变压器。
- 该方法在模型精度和训练速度方面都有显著改进。
- 方法的简单性、理论创新和实证优势使其成为神经网络初始化实践的强大增强。
点此查看论文截图
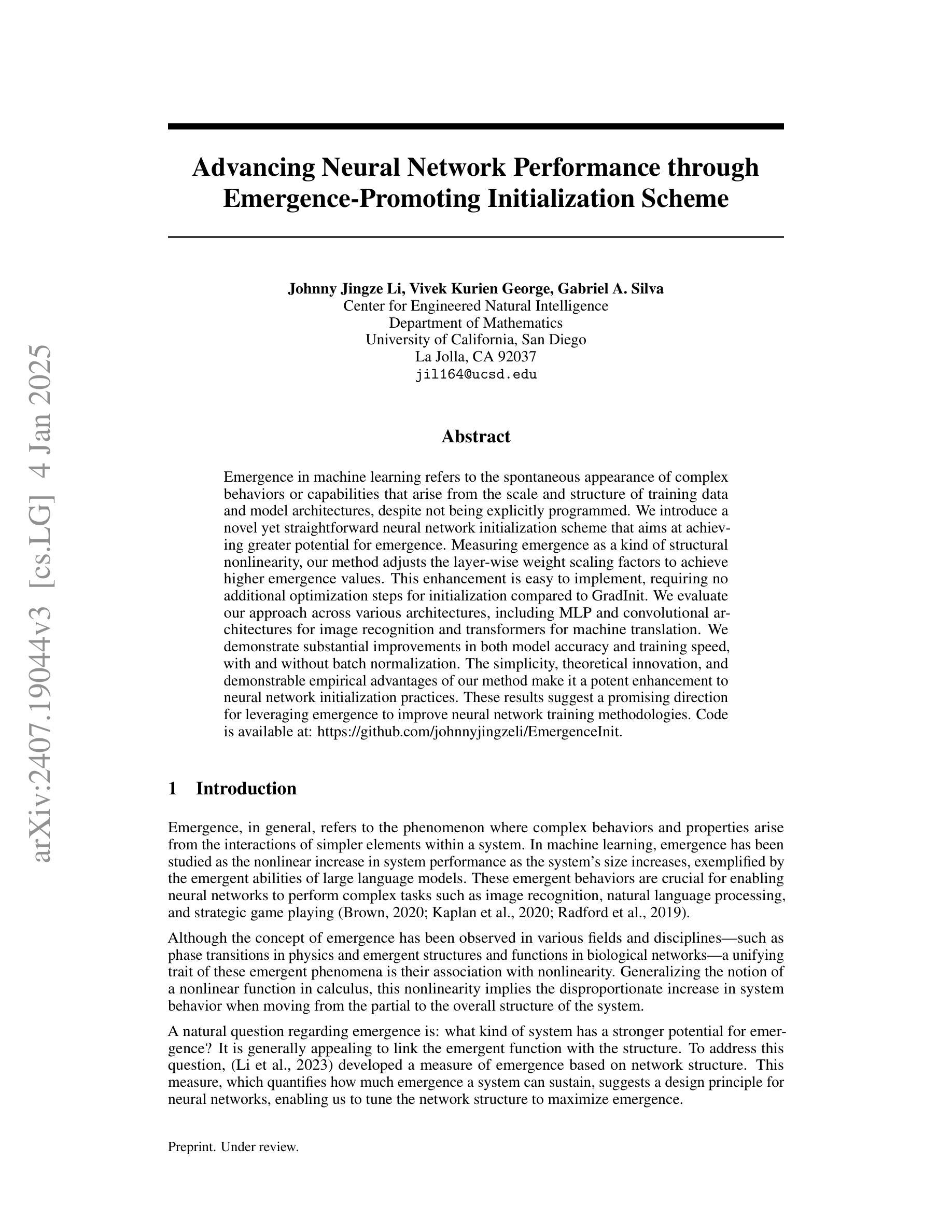
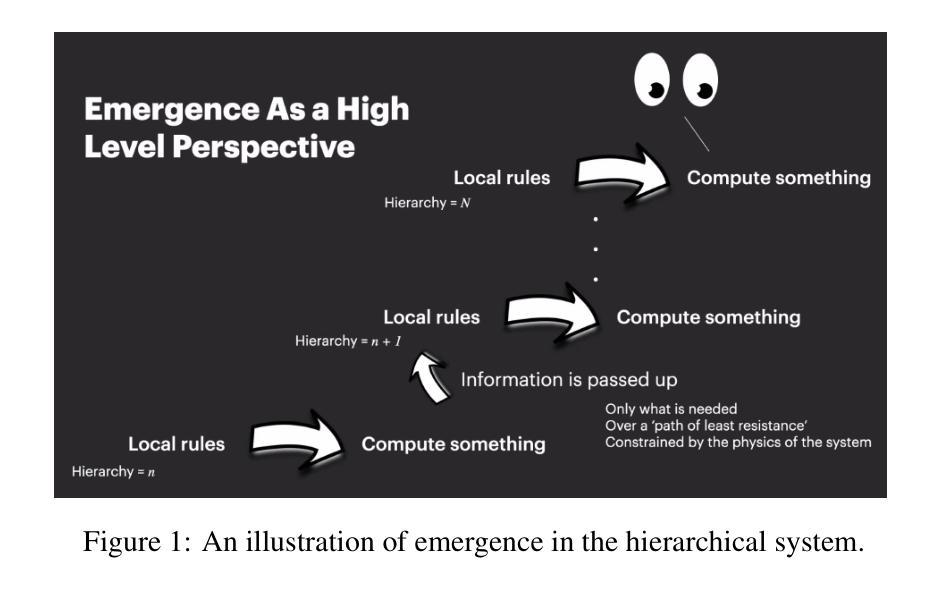
SRAGAN: Saliency Regularized and Attended Generative Adversarial Network for Chinese Ink-wash Painting Generation
Authors:Xiang Gao, Yuqi Zhang
Recent style transfer problems are still largely dominated by Generative Adversarial Network (GAN) from the perspective of cross-domain image-to-image (I2I) translation, where the pivotal issue is to learn and transfer target-domain style patterns onto source-domain content images. This paper handles the problem of translating real pictures into traditional Chinese ink-wash paintings, i.e., Chinese ink-wash painting style transfer. Though a wide range of I2I models tackle this problem, a notable challenge is that the content details of the source image could be easily erased or corrupted due to the transfer of ink-wash style elements. To remedy this issue, we propose to incorporate saliency detection into the unpaired I2I framework to regularize image content, where the detected saliency map is utilized from two aspects: (\romannumeral1) we propose saliency IOU (SIOU) loss to explicitly regularize object content structure by enforcing saliency consistency before and after image stylization; (\romannumeral2) we propose saliency adaptive normalization (SANorm) which implicitly enhances object structure integrity of the generated paintings by dynamically injecting image saliency information into the generator to guide stylization process. Besides, we also propose saliency attended discriminator which harnesses image saliency information to focus generative adversarial attention onto the drawn objects, contributing to generating more vivid and delicate brush strokes and ink-wash textures. Extensive qualitative and quantitative experiments demonstrate superiority of our approach over related advanced image stylization methods in both GAN and diffusion model paradigms.
最近的风格迁移问题在跨域图像到图像(I2I)转换方面仍然在很大程度上受到生成对抗网络(GAN)的主导。这里的关键问题是学习和将目标域的风格模式转移到源域内容图像上。本文针对将真实图片翻译成传统水墨画的问题,即水墨画风格迁移。尽管有许多I2I模型处理这个问题,但一个显著的挑战是源图像的内容细节在转换水墨风格元素时很容易被擦除或破坏。为了解决这个问题,我们提出将显著性检测融入到非配对的I2I框架中来规范图像内容,显著性检测图从两个方面得到应用:(一)我们提出了显著性IOU(SIOU)损失,通过强制显著性一致性来明确规范对象内容结构,即在图像风格化之前和之后;(二)我们提出了显著性自适应归一化(SANorm),它通过动态将图像显著性信息注入生成器来隐含地增强生成画作的对象结构完整性,从而引导风格化过程。此外,我们还提出了显著性关注鉴别器,它利用图像显著性信息将生成对抗注意力集中在绘制对象上,有助于生成更生动、更精细的笔触和水墨纹理。大量的定性和定量实验表明,我们的方法在生成对抗网络和扩散模型范式中的相关先进图像风格化方法上具有优势。
论文及项目相关链接
PDF 34 pages, 15 figures
Summary:最新风格迁移问题仍以生成对抗网络(GAN)为主导,在跨域图像到图像(I2I)翻译领域,关键问题是学习和将目标域的风格模式转移到源域内容图像上。本文处理将真实图片翻译成传统水墨画的问题,即水墨画风转移。尽管有许多I2I模型可以解决此问题,但一个显著挑战是源图像的内容细节可能会因水墨风格元素的转移而轻易被擦除或损坏。为解决此问题,我们提出将显著性检测融入非配对I2I框架以规范图像内容,从两个方面利用检测到的显著性图:(一)我们提出显著性IOU(SIOU)损失,通过强制显著性一致性显式地规范对象内容结构,在图像风格化之前和之后;(二)我们提出显著性自适应归一化(SANorm),通过动态将图像显著性信息注入生成器以指导风格化过程,隐含地增强生成画作的对象结构完整性。此外,我们还提出了显著性关注鉴别器,利用图像显著性信息将生成对抗注意力集中在绘画对象上,有助于生成更生动、更精细的笔触和水墨纹理。广泛的定性和定量实验表明,我们的方法在GAN和扩散模型范式中均优于相关的高级图像风格化方法。
Key Takeaways:
- 最新风格迁移问题仍由GAN主导,尤其在跨域I2I翻译领域。
- 面临的关键挑战在于学习和转移目标域的风格模式到源域内容图像上。
- 面临的一个显著问题是源图像内容细节在风格迁移过程中容易被擦除或损坏。
- 论文通过将显著性检测融入非配对I2I框架来解决这一问题,提出显著IOU损失和显著自适应归一化方法来规范和维护图像内容。
- 还提出了显著性关注鉴别器,以集中生成对抗注意力在绘画对象上,生成更生动、精细的笔触和水墨纹理。
- 广泛的实验证明,该方法在GAN和扩散模型范式中均表现出优越性。
点此查看论文截图
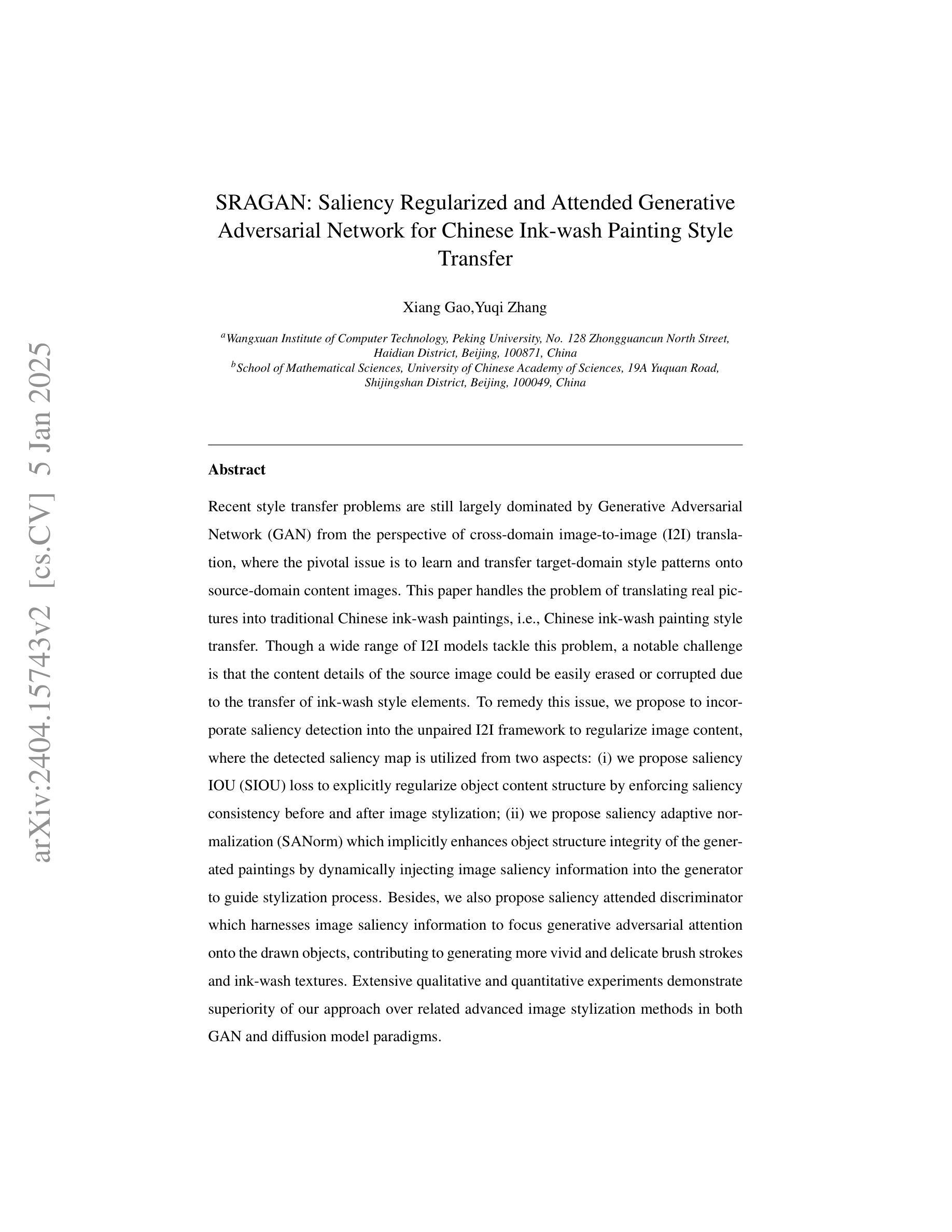
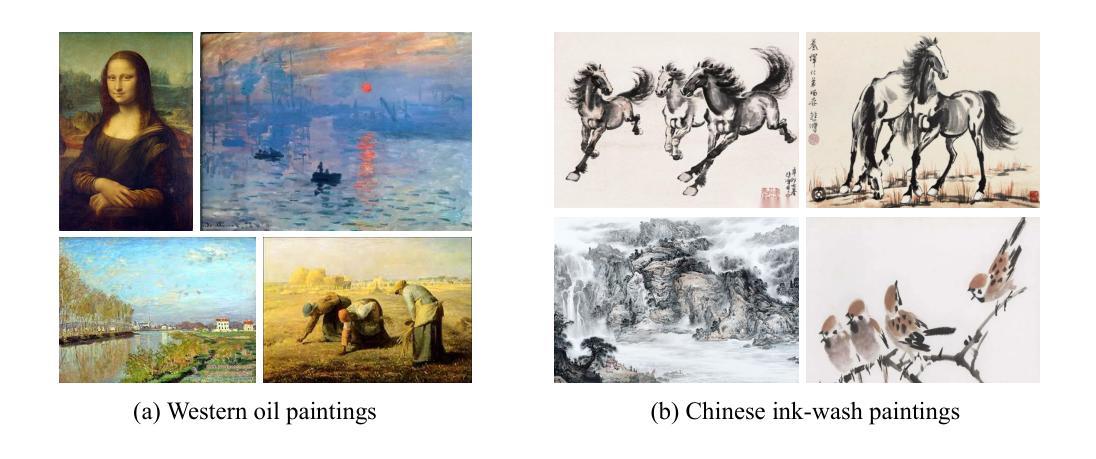
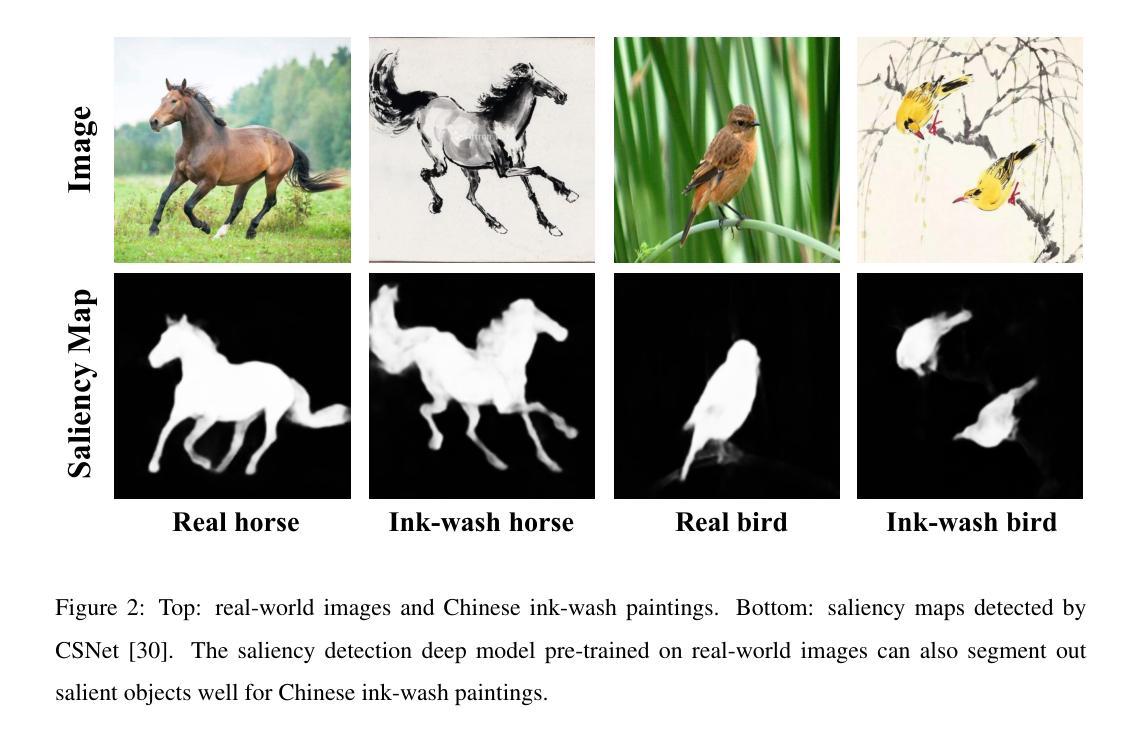