⚠️ 以下所有内容总结都来自于 大语言模型的能力,如有错误,仅供参考,谨慎使用
🔴 请注意:千万不要用于严肃的学术场景,只能用于论文阅读前的初筛!
💗 如果您觉得我们的项目对您有帮助 ChatPaperFree ,还请您给我们一些鼓励!⭐️ HuggingFace免费体验
2025-01-08 更新
AE-NeRF: Augmenting Event-Based Neural Radiance Fields for Non-ideal Conditions and Larger Scene
Authors:Chaoran Feng, Wangbo Yu, Xinhua Cheng, Zhenyu Tang, Junwu Zhang, Li Yuan, Yonghong Tian
Compared to frame-based methods, computational neuromorphic imaging using event cameras offers significant advantages, such as minimal motion blur, enhanced temporal resolution, and high dynamic range. The multi-view consistency of Neural Radiance Fields combined with the unique benefits of event cameras, has spurred recent research into reconstructing NeRF from data captured by moving event cameras. While showing impressive performance, existing methods rely on ideal conditions with the availability of uniform and high-quality event sequences and accurate camera poses, and mainly focus on the object level reconstruction, thus limiting their practical applications. In this work, we propose AE-NeRF to address the challenges of learning event-based NeRF from non-ideal conditions, including non-uniform event sequences, noisy poses, and various scales of scenes. Our method exploits the density of event streams and jointly learn a pose correction module with an event-based NeRF (e-NeRF) framework for robust 3D reconstruction from inaccurate camera poses. To generalize to larger scenes, we propose hierarchical event distillation with a proposal e-NeRF network and a vanilla e-NeRF network to resample and refine the reconstruction process. We further propose an event reconstruction loss and a temporal loss to improve the view consistency of the reconstructed scene. We established a comprehensive benchmark that includes large-scale scenes to simulate practical non-ideal conditions, incorporating both synthetic and challenging real-world event datasets. The experimental results show that our method achieves a new state-of-the-art in event-based 3D reconstruction.
与基于帧的方法相比,使用事件相机进行的计算神经形态成像具有显著优势,例如运动模糊最小化、时间分辨率增强和高动态范围。神经网络辐射场的多视角一致性结合事件相机的独特优势,激发了最近关于从移动事件相机捕获的数据重建NeRF的研究。虽然现有方法表现出令人印象深刻的效果,但它们依赖于理想条件,需要均匀且高质量的事件序列和精确的相机姿态,并且主要关注对象级别的重建,从而限制了其实际应用。在这项工作中,我们提出AE-NeRF来解决基于事件的NeRF从非理想条件(包括非均匀事件序列、噪声姿态和各种场景规模)学习的挑战。我们的方法利用事件流的密度,并联合学习一个姿态校正模块和一个基于事件NeRF(e-NeRF)框架,以实现从不准确相机姿态的稳健三维重建。为了推广到更大的场景,我们提出了分层事件蒸馏方法,使用一个提案e-NeRF网络和一个标准e-NeRF网络来重采样和细化重建过程。我们还提出了事件重建损失和时间损失来提高重建场景的观察一致性。我们建立了一个包括大规模场景的全面基准测试,以模拟实际非理想条件,并结合合成和具有挑战性的现实世界事件数据集。实验结果表明,我们的方法达到了基于事件的3D重建的最新水平。
论文及项目相关链接
PDF Accepted by AAAI 2025. https://github.com/SuperFCR/AE-NeRF
Summary
本文提出一种基于事件相机的NeRF重建方法AE-NeRF,解决了在非理想条件下学习事件NeRF的挑战,包括非均匀事件序列、噪声姿态和各种场景尺度。通过利用事件流的密度,结合姿态校正模块和事件NeRF框架,实现了从不准确相机姿态的鲁棒3D重建。为提高场景重建的视一致性,还提出了事件重建损失和临时损失。建立的综合基准测试包括模拟实际非理想条件的大型场景,结合合成和具有挑战性的真实事件数据集。实验结果表明,该方法在基于事件的三维重建中达到新的先进水平。
Key Takeaways
- 事件相机与NeRF结合具有多视图一致性优势。
- 现有方法依赖理想条件,如均匀高质量事件序列和准确相机姿态。
- AE-NeRF解决非理想条件下的事件NeRF学习挑战,包括非均匀事件序列、噪声姿态和场景尺度。
- 通过利用事件流密度和结合姿态校正模块,实现鲁棒3D重建。
- 引入事件重建损失和临时损失以提高重建场景的视一致性。
- 建立的综合基准测试模拟非理想条件下的大型场景。
点此查看论文截图
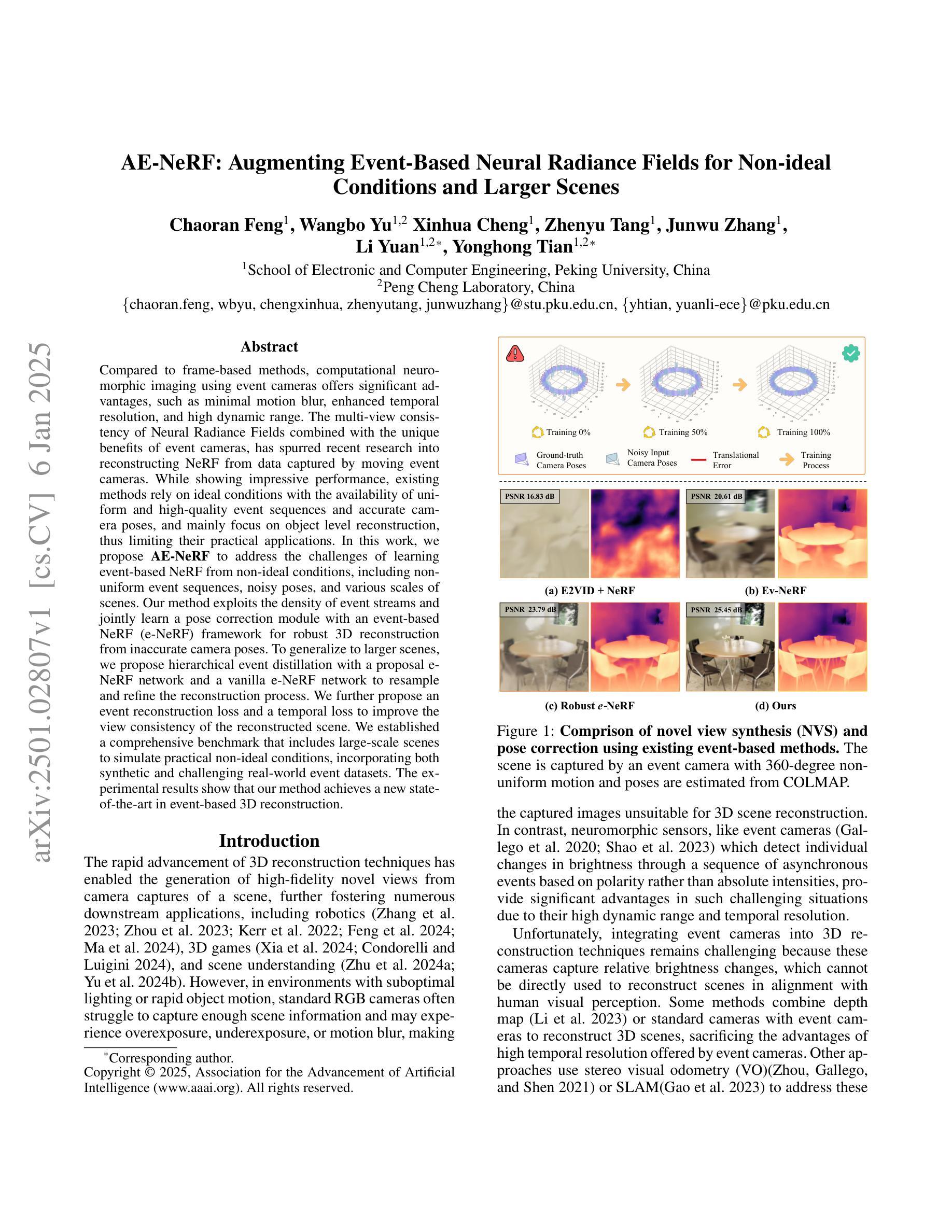
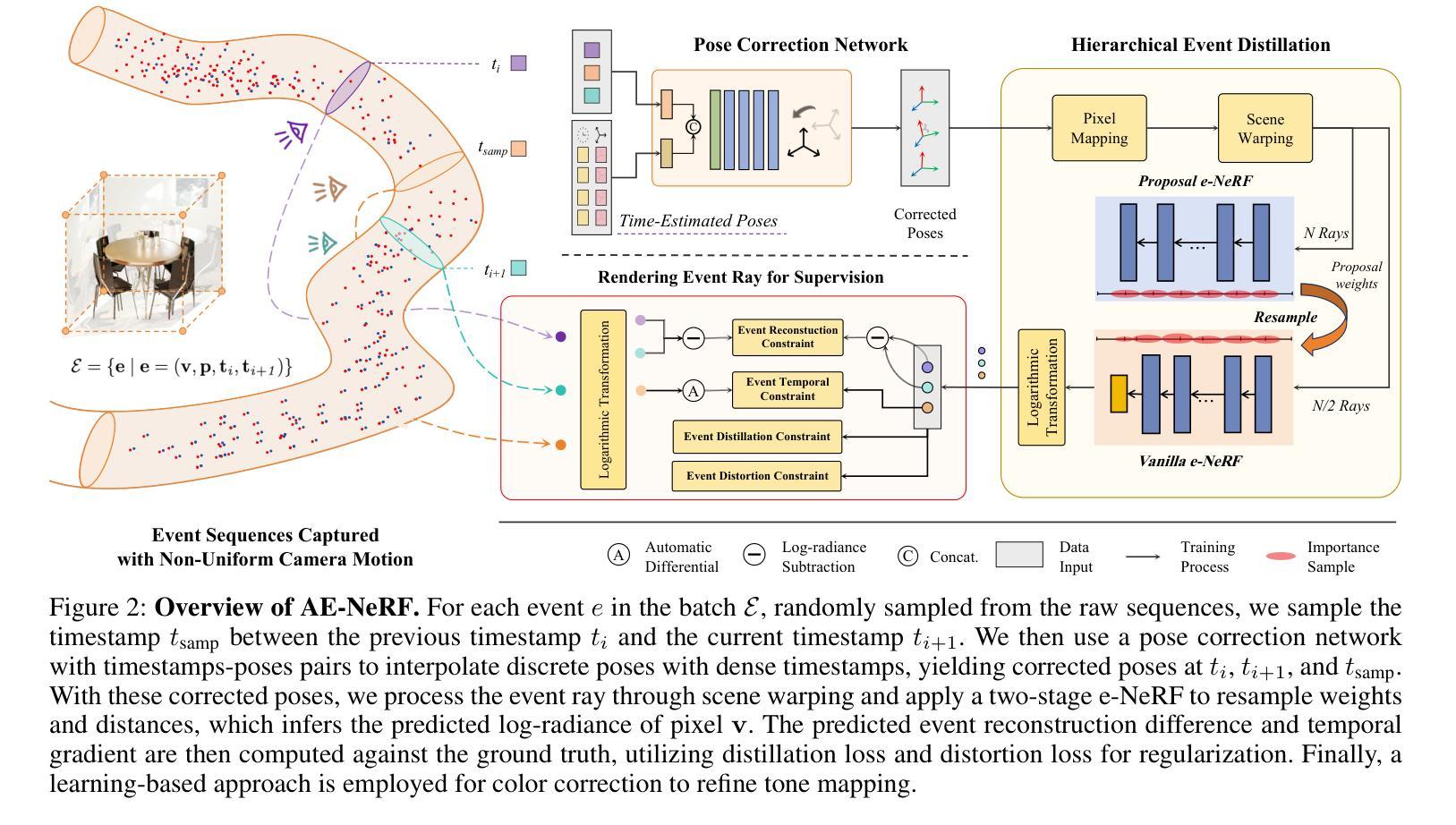
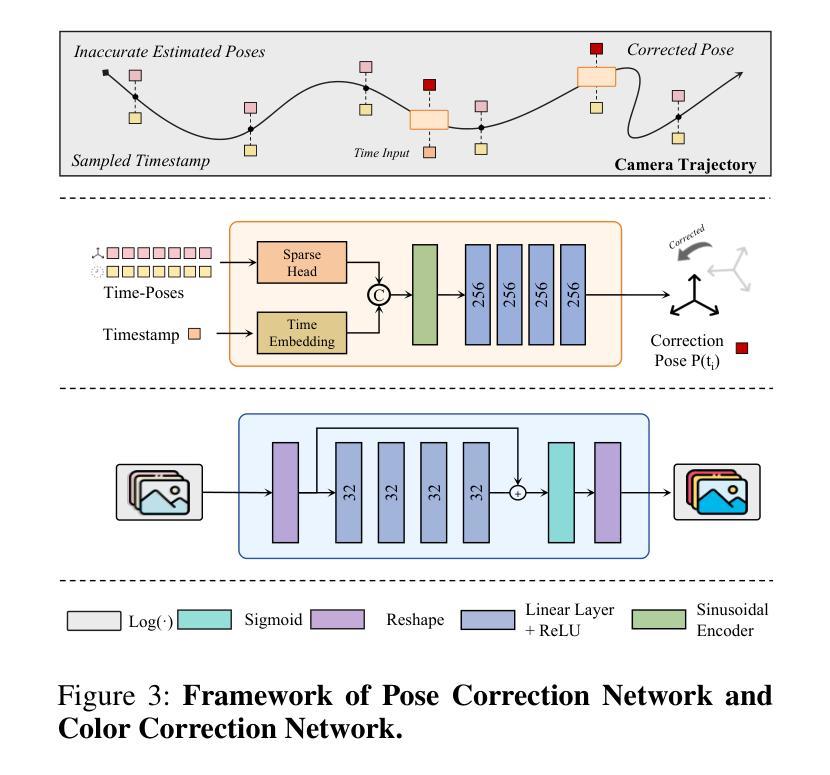
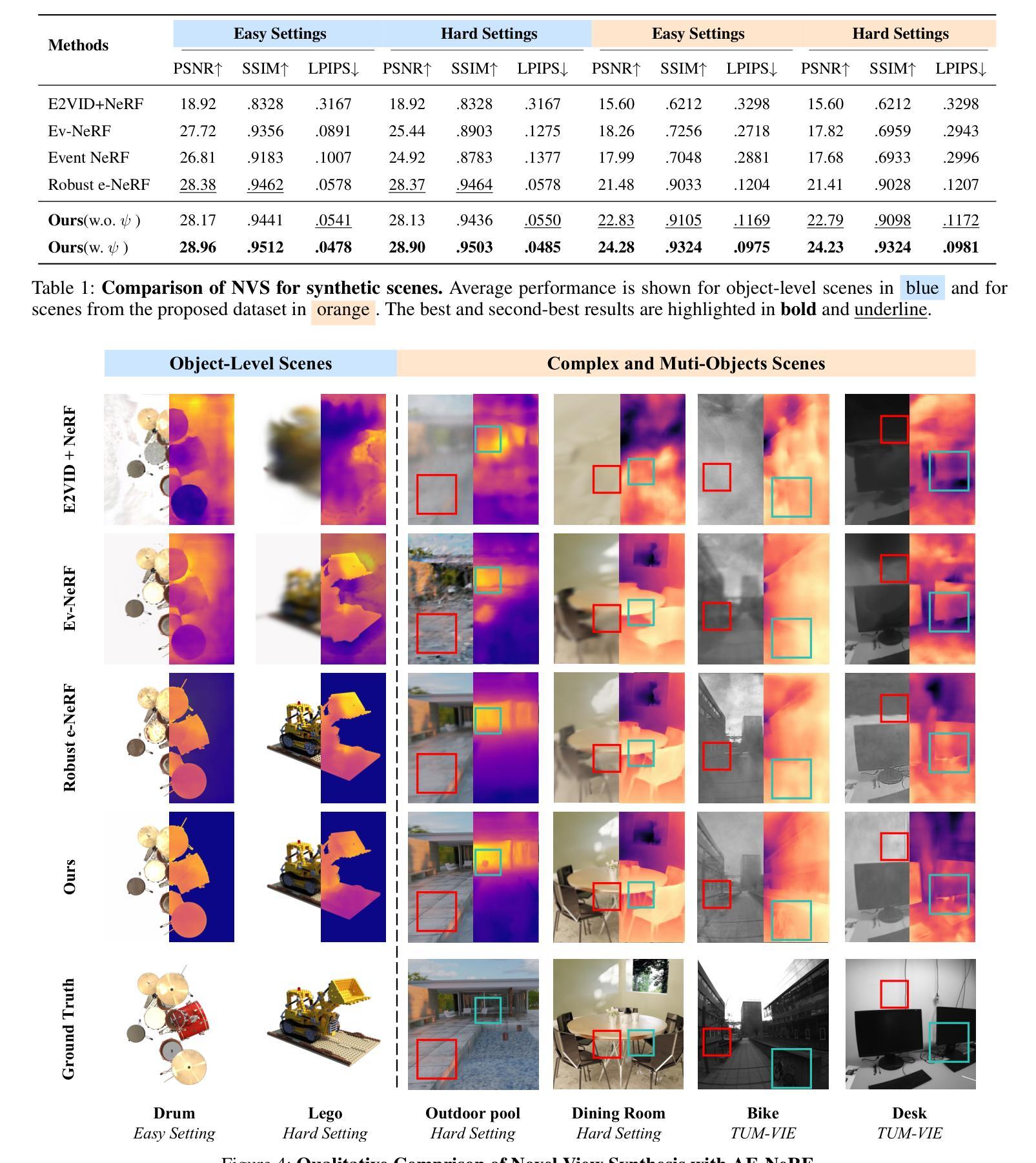
BeSplat: Gaussian Splatting from a Single Blurry Image and Event Stream
Authors:Gopi Raju Matta, Reddypalli Trisha, Kaushik Mitra
Novel view synthesis has been greatly enhanced by the development of radiance field methods. The introduction of 3D Gaussian Splatting (3DGS) has effectively addressed key challenges, such as long training times and slow rendering speeds, typically associated with Neural Radiance Fields (NeRF), while maintaining high-quality reconstructions. In this work (BeSplat), we demonstrate the recovery of sharp radiance field (Gaussian splats) from a single motion-blurred image and its corresponding event stream. Our method jointly learns the scene representation via Gaussian Splatting and recovers the camera motion through Bezier SE(3) formulation effectively, minimizing discrepancies between synthesized and real-world measurements of both blurry image and corresponding event stream. We evaluate our approach on both synthetic and real datasets, showcasing its ability to render view-consistent, sharp images from the learned radiance field and the estimated camera trajectory. To the best of our knowledge, ours is the first work to address this highly challenging ill-posed problem in a Gaussian Splatting framework with the effective incorporation of temporal information captured using the event stream.
随着辐射场方法的发展,新型视图合成技术得到了极大的增强。3D高斯喷涂技术(3DGS)的引入有效地解决了与神经辐射场(NeRF)通常相关的主要挑战,如训练时间长和渲染速度慢,同时保持了高质量的重建。在这项工作(BeSplat)中,我们展示了从单个运动模糊图像及其相应的事件流中恢复锐利的辐射场(高斯喷涂)的能力。我们的方法通过高斯喷涂联合学习场景表示,并通过贝塞尔SE(3)公式有效恢复相机运动,从而最小化合成图像和现实世界测量值之间以及模糊图像和相应事件流之间的差异。我们在合成数据集和真实数据集上评估了我们的方法,展示了其从学习的辐射场和估计的相机轨迹呈现一致、清晰视图的能力。据我们所知,我们的工作是第一个在高斯喷涂框架下解决这一极具挑战性的不适定问题的工作,有效结合了使用事件流捕获的时间信息。
论文及项目相关链接
PDF Accepted for publication at EVGEN2025, WACV-25 Workshop
Summary
本文介绍了通过引入3D高斯拼贴技术(3DGS)改进了神经辐射场(NeRF)的新视角合成效果。此方法在解决NeRF长期存在的训练时间长和渲染速度慢的问题时仍能保持高质量的重建。研究名为“BeSplat”,它能从单一的运动模糊图像和对应的事件流中恢复出锐利的辐射场(高斯拼贴)。该方法通过高斯拼贴场景表示和Bezier SE(3)公式估计相机运动,有效缩小了合成与真实世界测量的模糊图像和事件流之间的差异。实验证明,该方法能在合成和真实数据集上,从学习到的辐射场和估计的相机轨迹中渲染出连贯、清晰的图像。据我们所知,这是首个在高斯拼贴框架下解决这一极具挑战的不适定问题的研究,并有效地结合了通过事件流捕获的时间信息。
Key Takeaways
- 引入3D高斯拼贴技术改进了神经辐射场的新视角合成效果。
- 通过结合高斯拼贴场景表示,BeSplat技术解决了相机运动模糊问题。
- BeSplat方法利用了事件流捕获的时间信息来提高重建的精度和效率。
- 通过联合学习场景表示和相机运动估计,该方法能有效缩小合成与真实测量的差异。
- 该方法在合成和真实数据集上表现出色,能够渲染出连贯且清晰的图像。
- 据悉,这是首个解决利用高斯拼贴框架下处理运动模糊图像恢复问题的工作。
点此查看论文截图
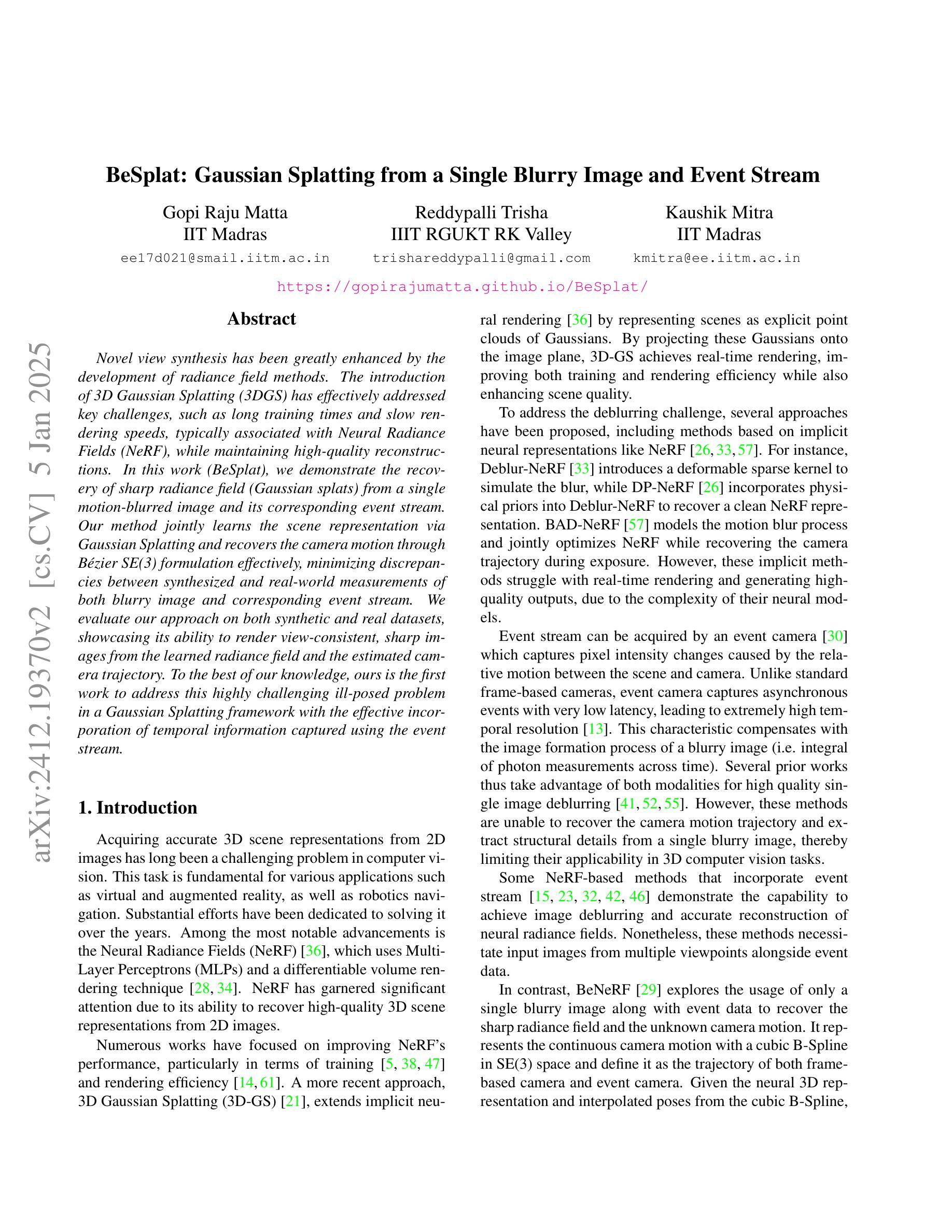
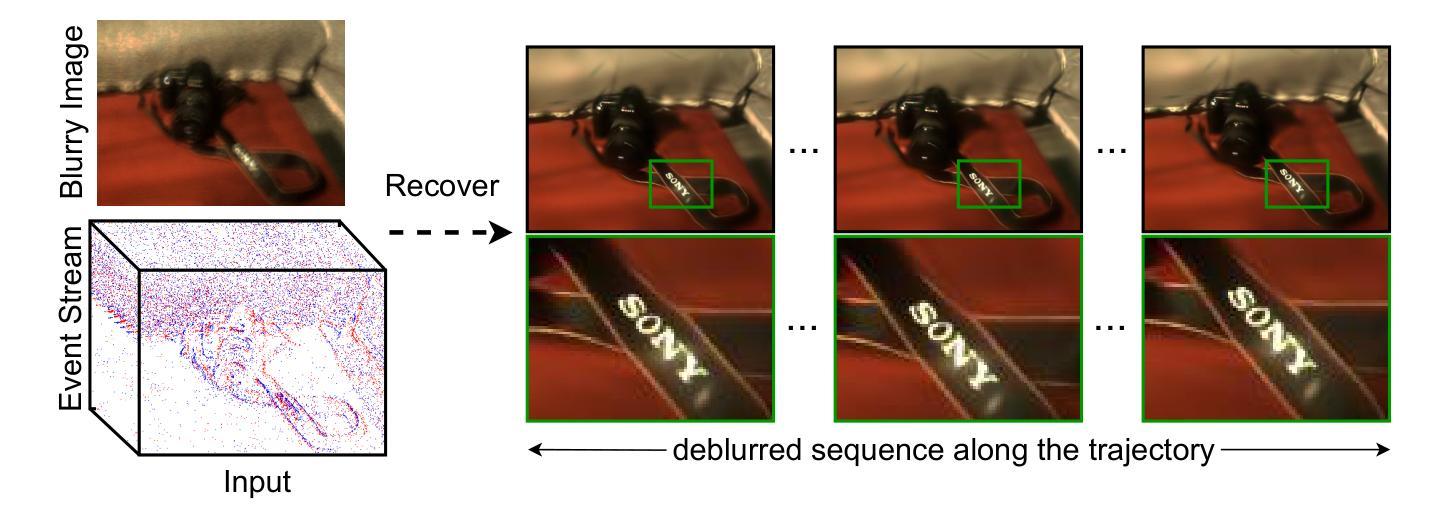
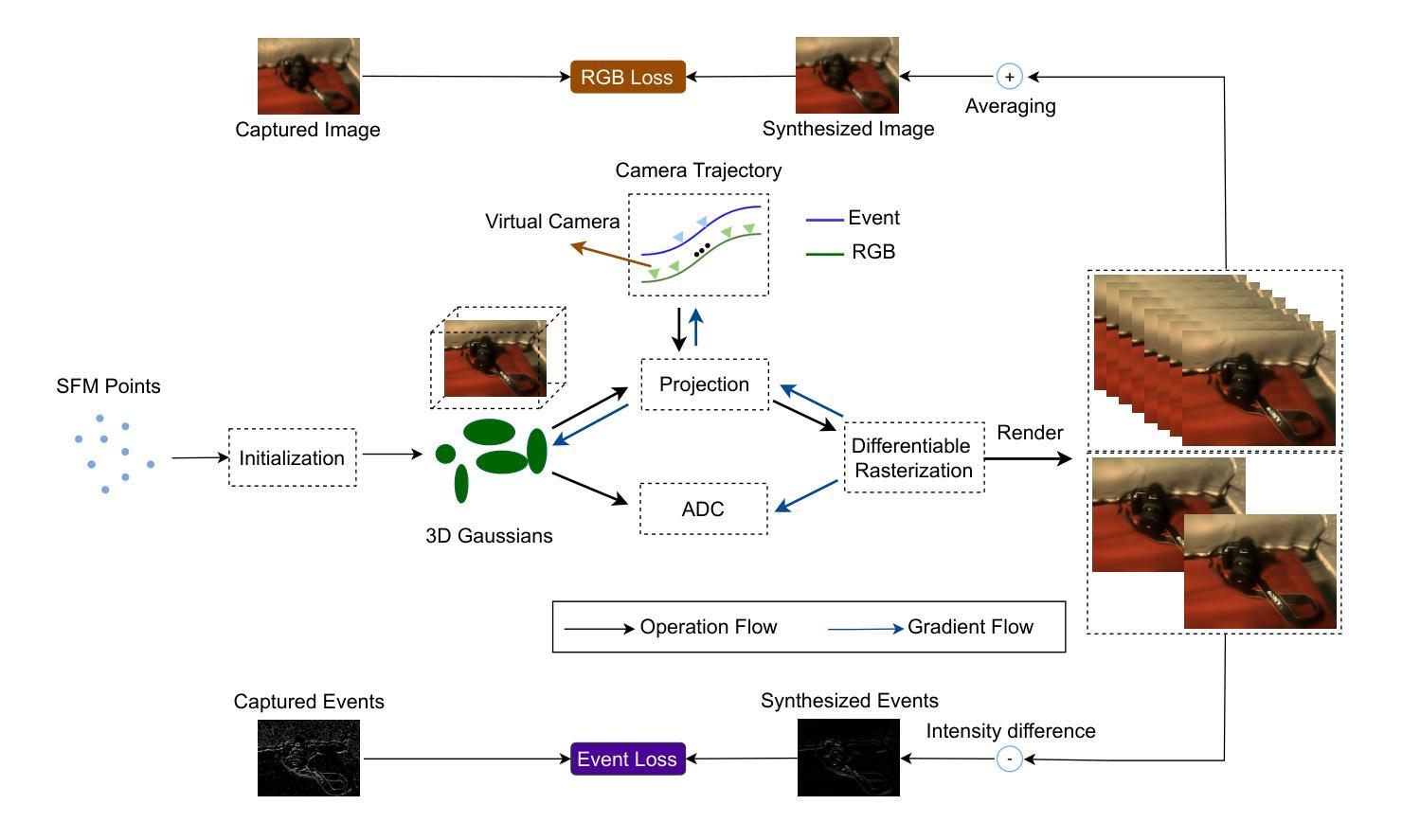
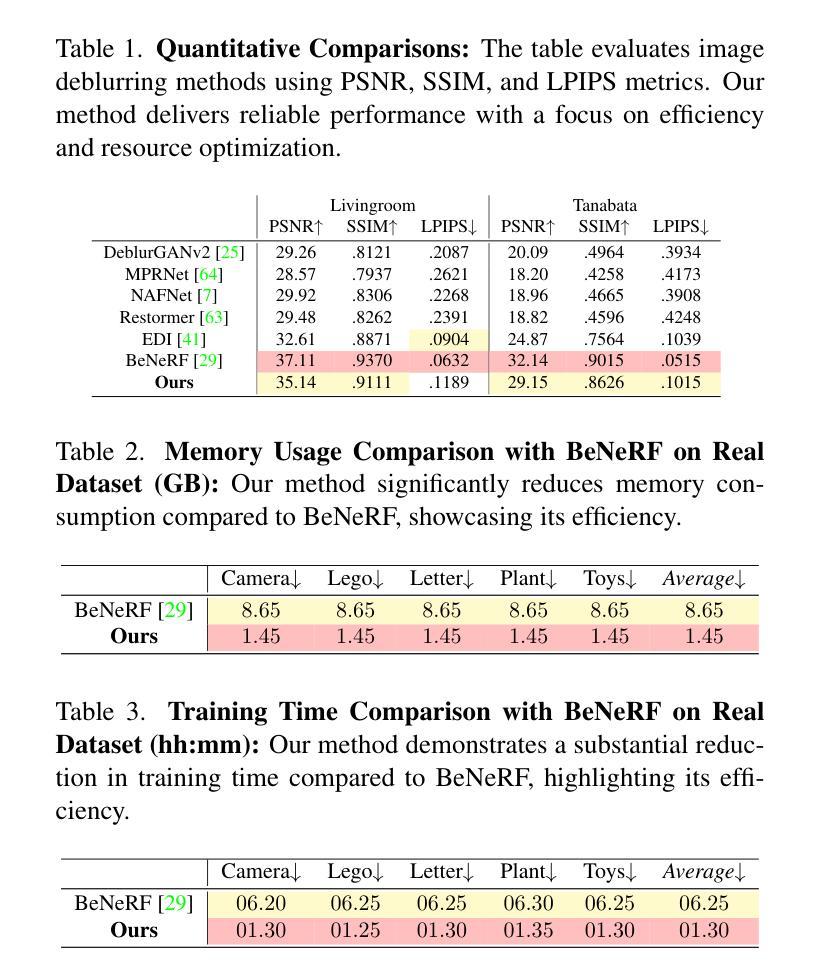
Unusually high-density 2D electron gases in N-polar AlGaN/GaN heterostructures with GaN/AlN superlattice back barriers grown on sapphire substrates
Authors:Maciej Matys, Atsushi Yamada, Toshihiro Ohki
We reported on the observation of extremely high-density ($>10^{14}$cm$^{-2}$) 2D electron gas in N-polar AlGaN/GaN heterostructures grown on sapphire substrates. Due to introducing the GaN/AlN superlattice (SL) back barrier between the GaN buffer layer and AlGaN barrier layer, we observed a giant enhancement of the 2D electron gas density at the GaN/AlGaN interface from $3\times10^{13}$cm$^{-2}$ (without SL) to $1.4\times10^{14}$cm$^{-2}$ (with SL back barrier) that is only one order of magnitude below the intrinsic crystal limit of $\approx10^{15}$cm$^{-2}$. We found that the changes of 2D electron gas density with SL correlated well with the changes of the wafer warp parameter which suggests that the strains are responsible for the 2D electron gas density enhancement (reduction of the piezoelectric polarization in the GaN channel). Nevertheless, this finding is probably insufficient to fully explain the observed high 2D electron gas density. Simultaneously, the room temperature electron mobility was 169 cm$^2$/Vs, which with the electron density of $1.4\times10^{14}$cm$^{-2}$ gives a low sheet resistance of 264 $\Omega$/sq (one of the lowest reported so far for the N-polar 2D electron gas channel). Finally, the possibility of application of such high-density 2D electron gas with low sheet resistance to transistors, emitters and detectors was discussed.
我们报告了在蓝宝石基板上生长的N极AlGaN/GaN异质结构中观察到极高密度(> 10^14 cm^-2)的二维电子气体的观察结果。由于在GaN缓冲层和AlGaN势垒层之间引入了GaN/AlN超晶格(SL)背势垒,我们观察到GaN/AlGaN界面处的二维电子气体密度从无SL时的3×10^13 cm^-2 巨增至带有SL背势垒时的1.4×10^14 cm^-2,仅比固有晶体极限值约10^15 cm^-2低一个数量级。我们发现具有SL的二维电子气体密度的变化与晶圆翘曲参数的变化密切相关,这表明应变是导致二维电子气体密度增强(减少GaN通道中的压电极化)的原因。然而,这一发现可能不足以完全解释观察到的极高二维电子气体密度。同时,室温电子迁移率达到了169 cm^2/Vs,与电子密度1.4×10^14 cm^-2相结合,获得了低达264 Ω/sq的方块电阻(目前针对N极二维电子气体通道所报道的最低值之一)。最后,讨论了这种高密度二维电子气体在低电阻下在晶体管、发射器和探测器中的应用可能性。
论文及项目相关链接
PDF In press at the Physica status solidi (rrl) Rapid Research Letters. This is an update version after peer review
Summary
该文章研究了生长在蓝宝石衬底上的N极AlGaN/GaN异质结构中二维电子气体的密度。通过引入GaN/AlN超晶格(SL)背屏障,观察到二维电子气体密度在GaN/AlGaN界面处从无SL的$3\times10^{13}$cm$^{-2}$增强到带有SL背屏障的$1.4\times10^{14}$cm$^{-2}$,仅比固有晶体极限低一个数量级。文章讨论了这种高密度的二维电子气体在晶体管、发射器和检测器中的应用潜力。
Key Takeaways
- 在N极AlGaN/GaN异质结构上观察到超高密度的二维电子气体。
- 通过引入GaN/AlN超晶格背屏障,实现了二维电子气体密度的巨大提升。
- 高密度二维电子气体与晶片的翘曲参数变化有关,暗示了应变对二维电子气体密度增强的作用。然而,该发现可能不足以完全解释观察到的二维电子气体密度。
- 电子迁移率达到室温下为169 cm$^2$/Vs,与电子密度结合后得到低的面电阻为264 Ω/sq。
- 高密度的二维电子气体和低的面电阻使得这种材料在晶体管、发射器和检测器中有潜在应用。
- 观察到的二维电子气体密度是目前为止报告的N极中最低的之一。
点此查看论文截图
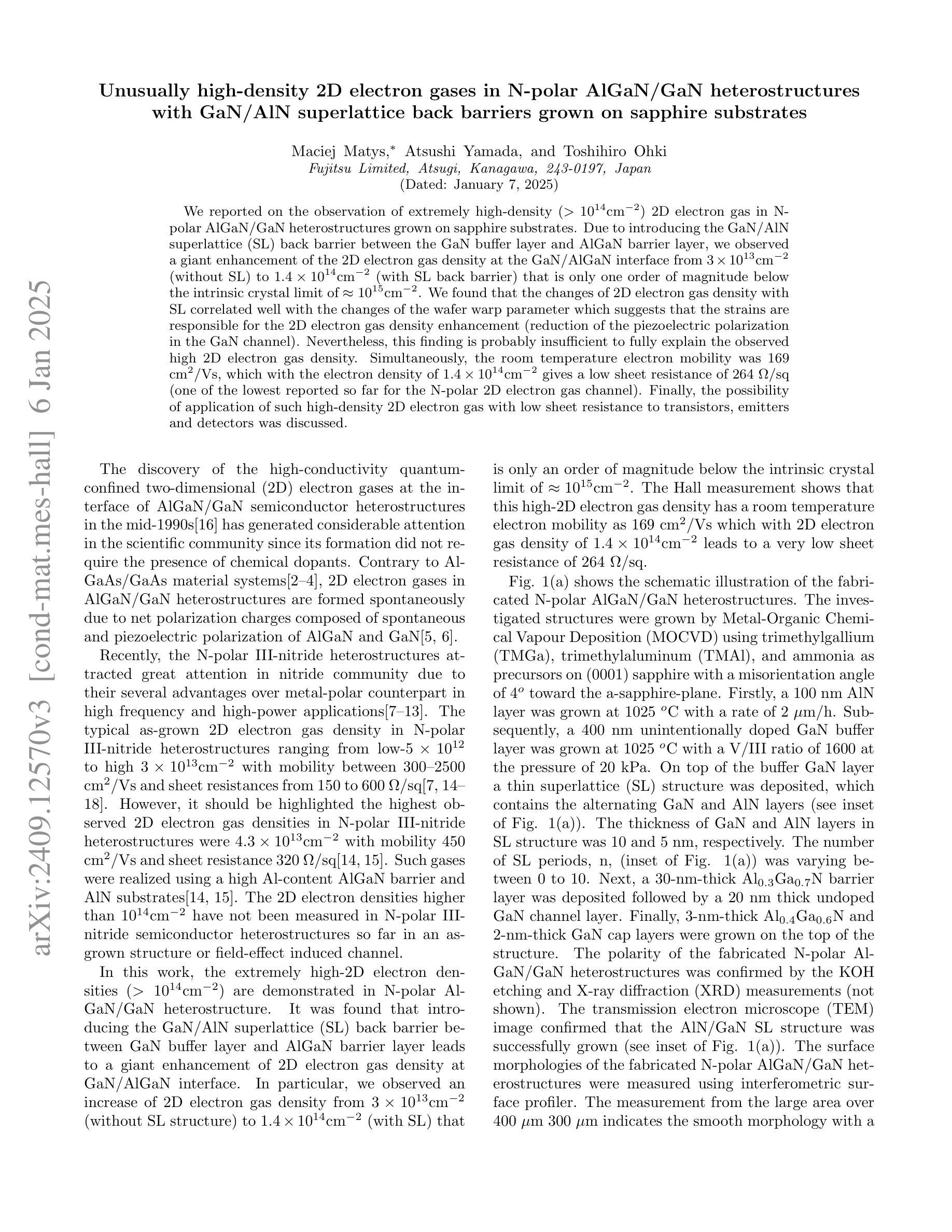
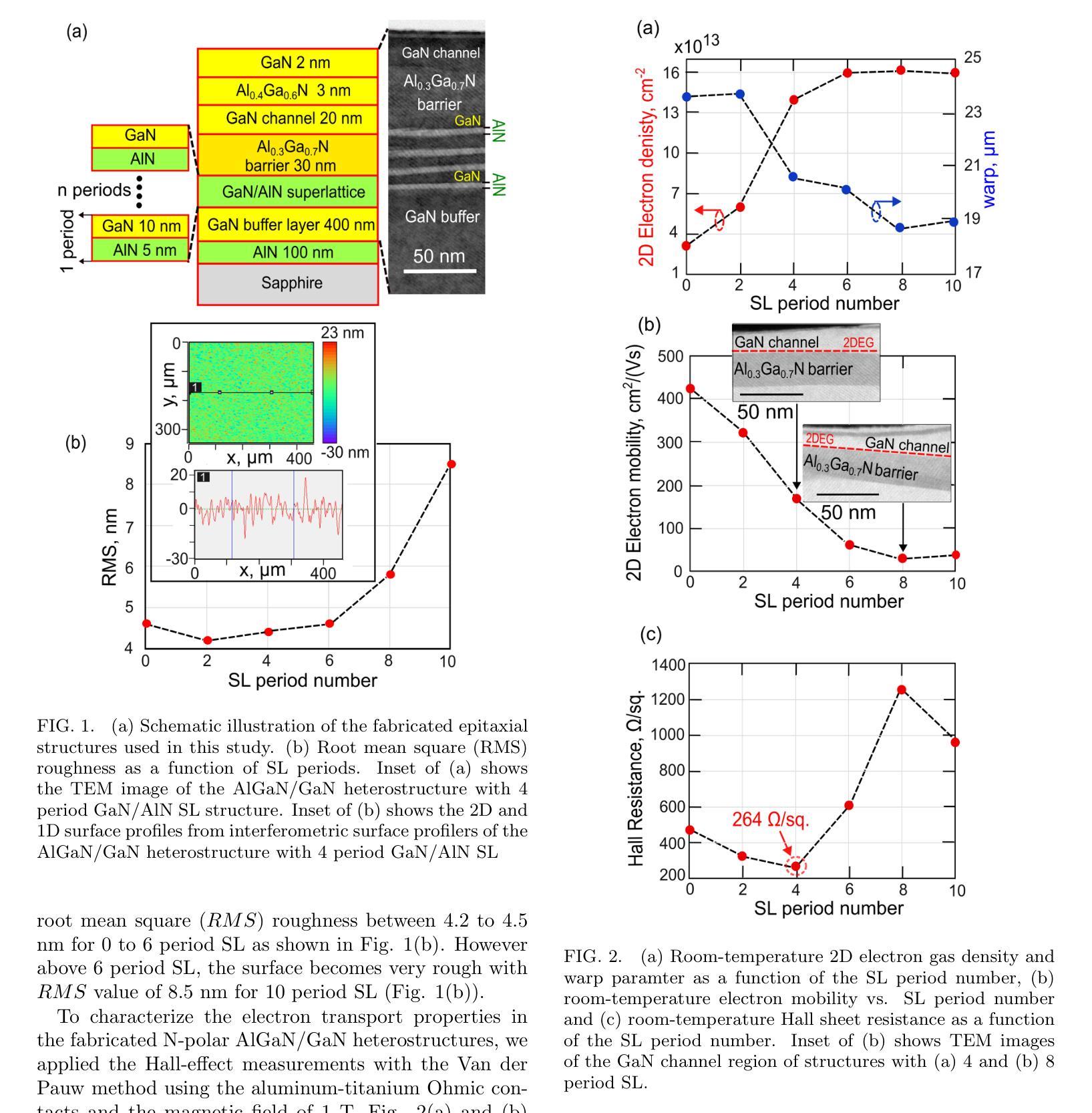
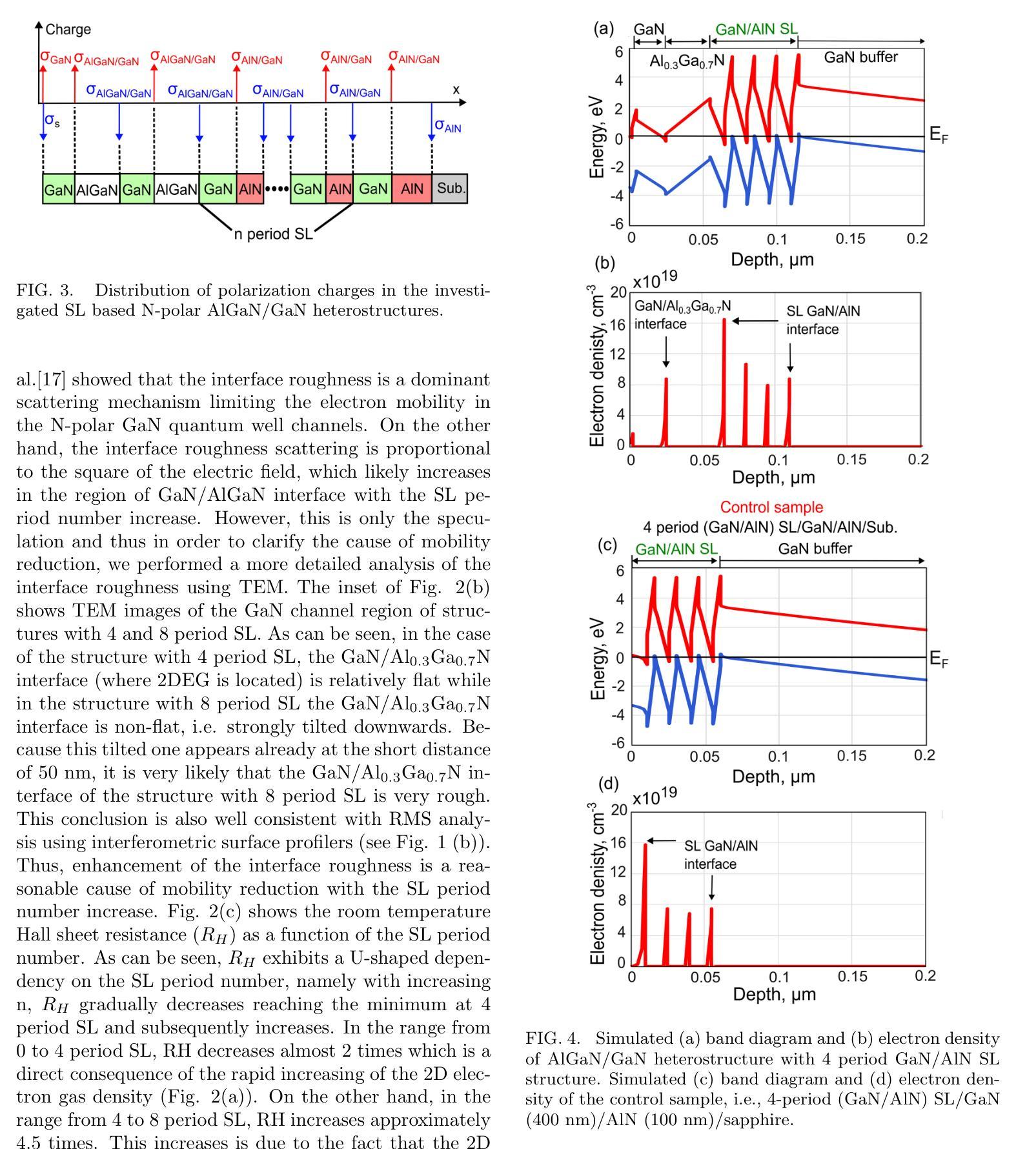
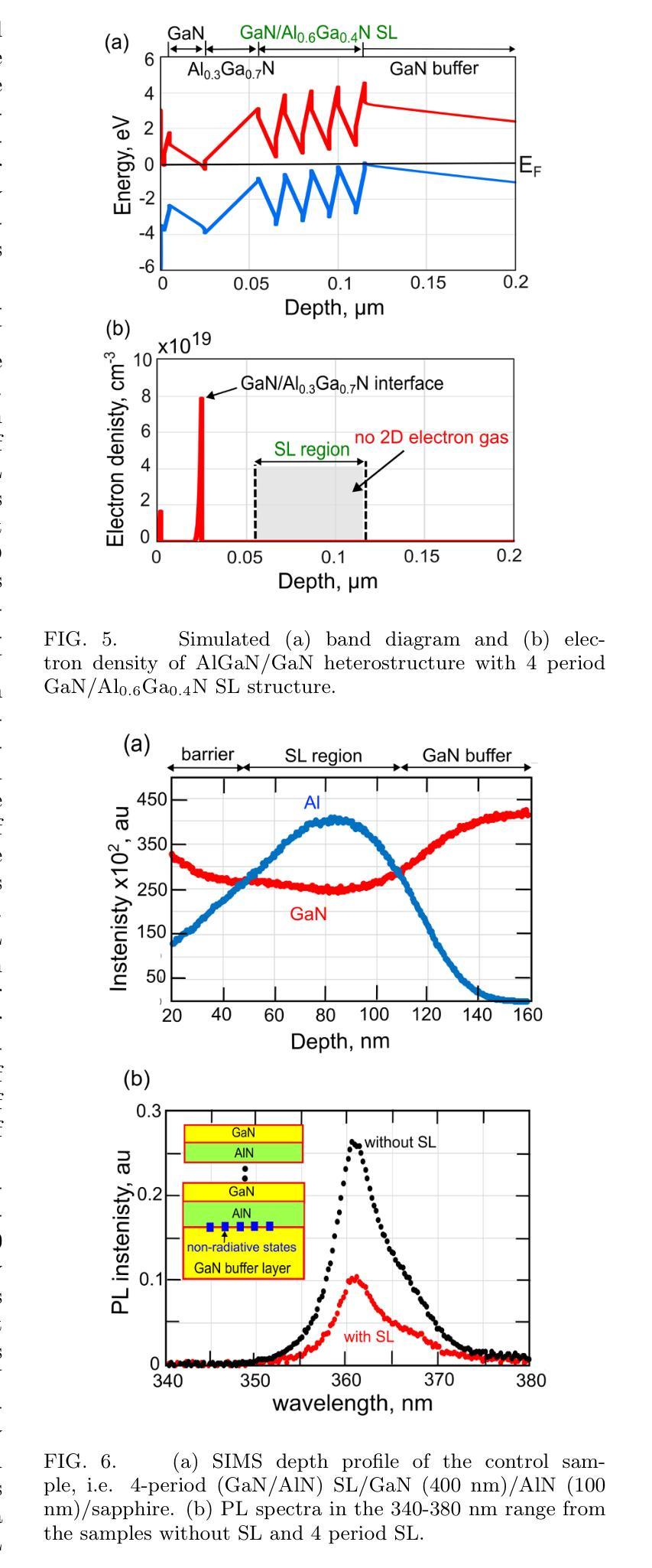
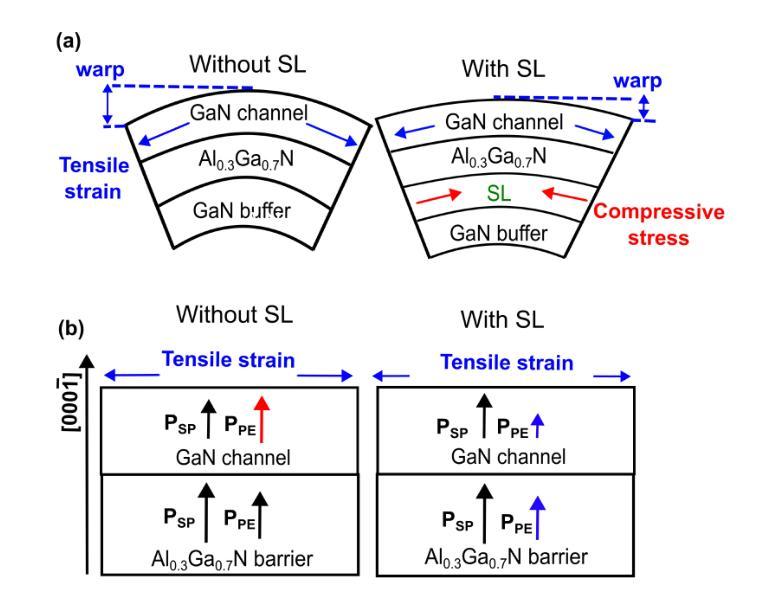

NeRFool: Uncovering the Vulnerability of Generalizable Neural Radiance Fields against Adversarial Perturbations
Authors:Yonggan Fu, Ye Yuan, Souvik Kundu, Shang Wu, Shunyao Zhang, Yingyan Celine Lin
Generalizable Neural Radiance Fields (GNeRF) are one of the most promising real-world solutions for novel view synthesis, thanks to their cross-scene generalization capability and thus the possibility of instant rendering on new scenes. While adversarial robustness is essential for real-world applications, little study has been devoted to understanding its implication on GNeRF. We hypothesize that because GNeRF is implemented by conditioning on the source views from new scenes, which are often acquired from the Internet or third-party providers, there are potential new security concerns regarding its real-world applications. Meanwhile, existing understanding and solutions for neural networks’ adversarial robustness may not be applicable to GNeRF, due to its 3D nature and uniquely diverse operations. To this end, we present NeRFool, which to the best of our knowledge is the first work that sets out to understand the adversarial robustness of GNeRF. Specifically, NeRFool unveils the vulnerability patterns and important insights regarding GNeRF’s adversarial robustness. Built upon the above insights gained from NeRFool, we further develop NeRFool+, which integrates two techniques capable of effectively attacking GNeRF across a wide range of target views, and provide guidelines for defending against our proposed attacks. We believe that our NeRFool/NeRFool+ lays the initial foundation for future innovations in developing robust real-world GNeRF solutions. Our codes are available at: https://github.com/GATECH-EIC/NeRFool.
通用神经辐射场(GNeRF)是实现新颖视角合成的最具有前景的真实世界解决方案之一,归功于其跨场景的通用化能力以及在新场景上进行即时渲染的可能性。虽然对抗鲁棒性对于真实世界应用至关重要,但对于其在GNeRF上的影响却鲜有研究。我们假设,由于GNeRF的实现是通过以新场景中的源视图为条件(这些视图通常来自互联网或第三方提供商),因此其真实世界应用存在潜在的新安全担忧。同时,由于GNeRF的3D特性和独特多样的操作,现有神经网络对抗鲁棒性的理解和解决方案可能不适用于GNeRF。为此,我们提出了NeRFool,据我们所知,这是第一项旨在理解GNeRF对抗鲁棒性的工作。具体来说,NeRFool揭示了关于GNeRF对抗鲁棒性的漏洞模式和一些重要的见解。基于从NeRFool中获得的上述见解,我们进一步开发了NeRFool+,它集成了两种技术,能够在广泛的目标视图中有效地攻击GNeRF,并提供防御我们提出的攻击的指南。我们相信,我们的NeRFool/NeRFool+为未来开发稳健的真实世界GNeRF解决方案的创新奠定了初步基础。我们的代码可在:https://github.com/GATECH-EIC/NeRFool找到。
论文及项目相关链接
PDF Accepted by ICML 2023
Summary
基于GNeRF(通用神经辐射场)在跨场景泛化能力和即时渲染新场景上的优势,使其成为真实世界中新视角合成的重要解决方案。但GNeRF对真实世界应用的潜在安全风险不容忽视,尤其是其在应对网络或第三方提供的新场景源视图时的对抗性稳健性问题。目前对GNeRF的对抗性稳健性的研究尚少,而现有的神经网络对抗性稳健性的理解和解决方案可能不适用于GNeRF。本研究首次提出NeRFool来探索GNeRF的对抗性稳健性问题,揭示其脆弱性模式并给出重要见解。基于NeRFool的见解,进一步开发了NeRFool+,集成了两种技术,能在多种目标视角有效攻击GNeRF,并提供防御建议。本研究为未来开发稳健的真实世界GNeRF解决方案奠定了初步基础。代码可在公开仓库GitHub下载查阅。
Key Takeaways
- GNeRF具有跨场景泛化能力,是真实世界中新视角合成的重要解决方案。
- GNeRF在实际应用中可能存在对抗性稳健性问题,特别是在处理来自互联网或第三方提供的新场景源视图时。
- 目前对GNeRF的对抗性稳健性的研究尚不足,且现有的神经网络对抗性稳健性的理解和解决方案可能不适用于GNeRF。
- NeRFool首次对GNeRF的对抗性稳健性进行探索,揭示了其脆弱性模式并给出了重要见解。
- 基于NeRFool的见解,开发了NeRFool+,能有效攻击多种目标视角下的GNeRF,并提供防御建议。
- 本研究为未来开发稳健的真实世界GNeRF解决方案提供了初步基础。
点此查看论文截图
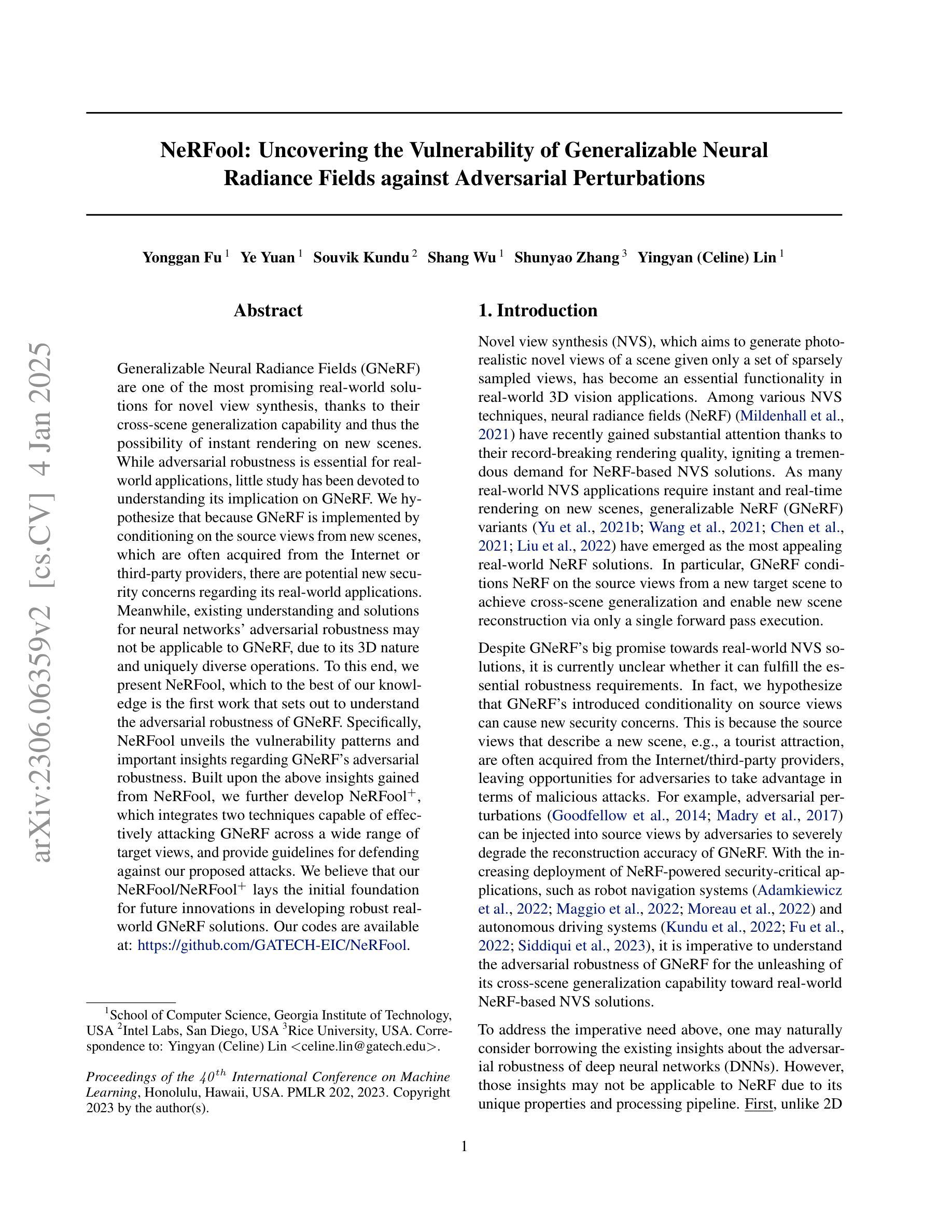
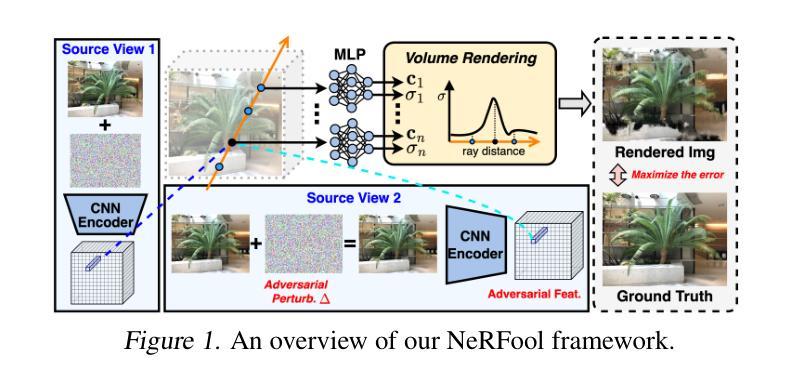
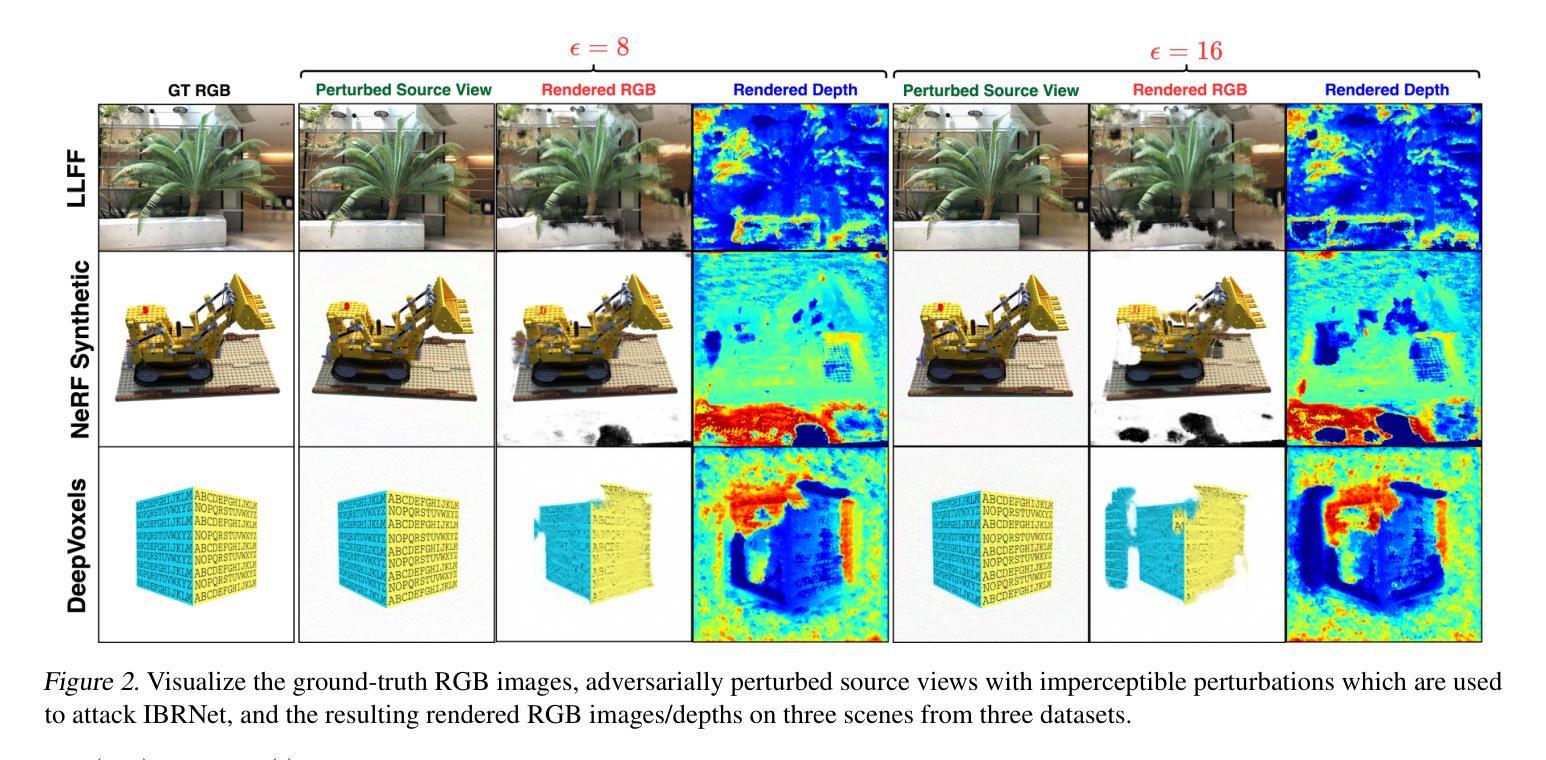
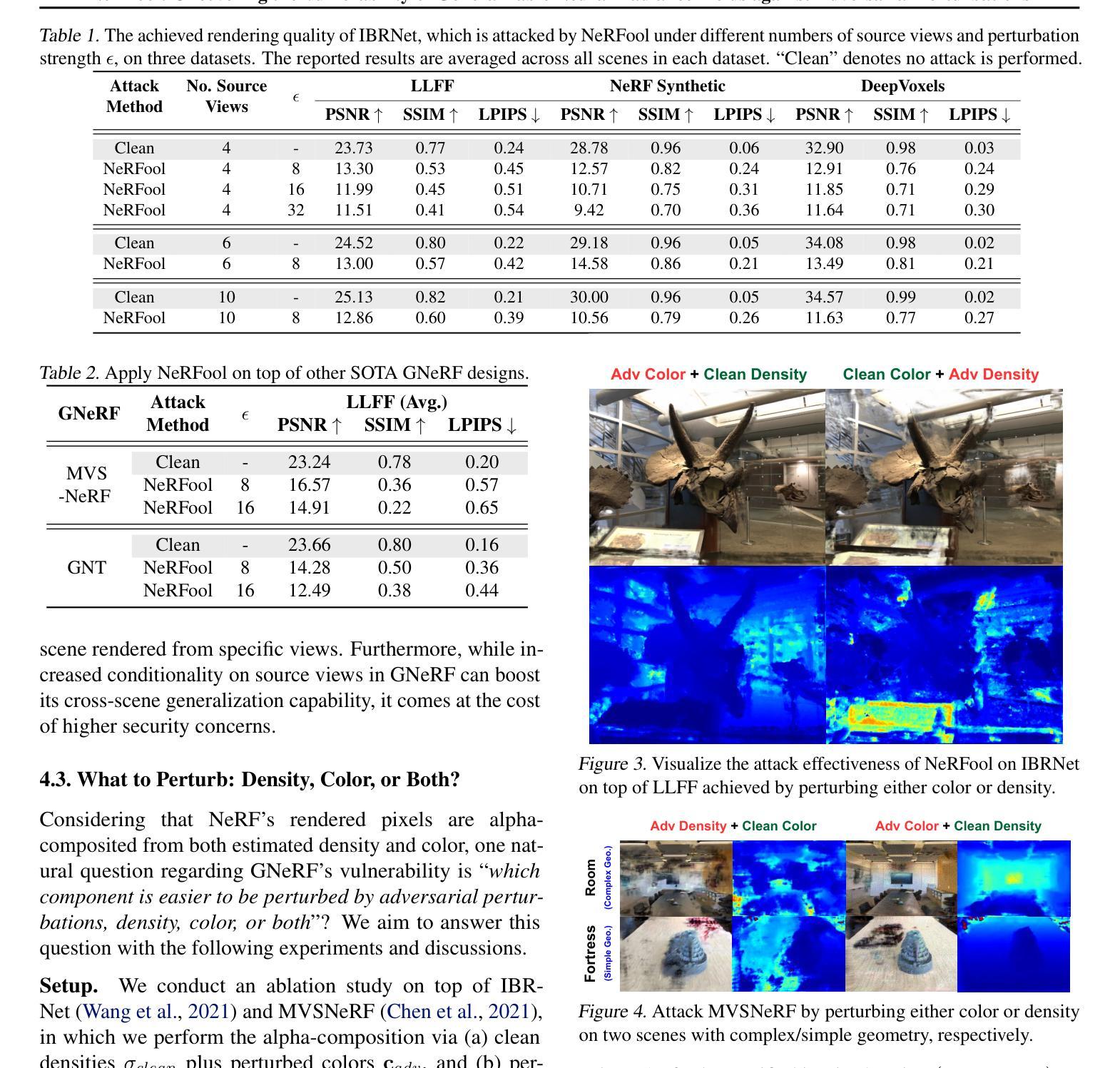
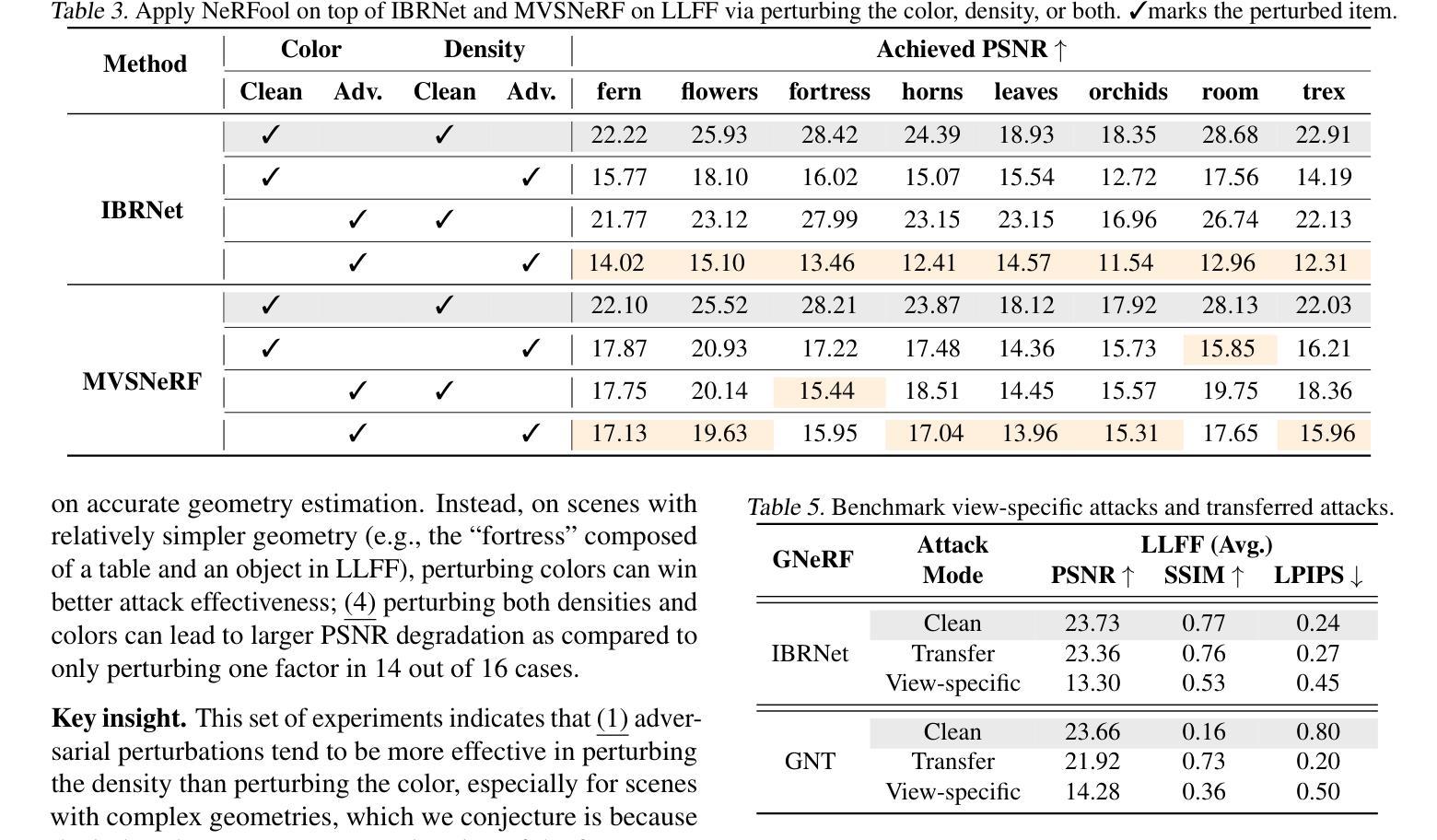
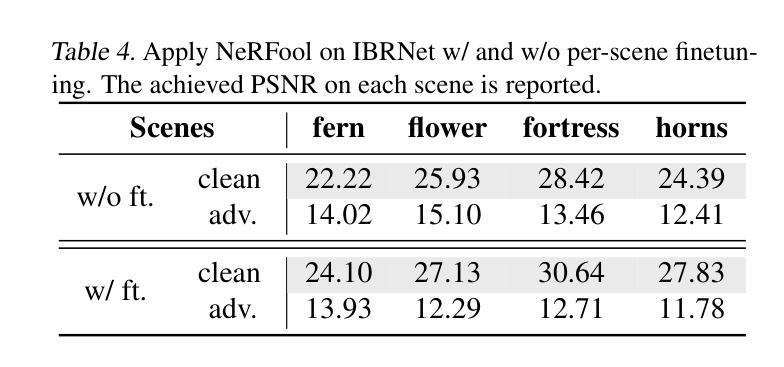