⚠️ 以下所有内容总结都来自于 大语言模型的能力,如有错误,仅供参考,谨慎使用
🔴 请注意:千万不要用于严肃的学术场景,只能用于论文阅读前的初筛!
💗 如果您觉得我们的项目对您有帮助 ChatPaperFree ,还请您给我们一些鼓励!⭐️ HuggingFace免费体验
2025-01-08 更新
RDD4D: 4D Attention-Guided Road Damage Detection And Classification
Authors:Asma Alkalbani, Muhammad Saqib, Ahmed Salim Alrawahi, Abbas Anwar, Chandarnath Adak, Saeed Anwar
Road damage detection and assessment are crucial components of infrastructure maintenance. However, current methods often struggle with detecting multiple types of road damage in a single image, particularly at varying scales. This is due to the lack of road datasets with various damage types having varying scales. To overcome this deficiency, first, we present a novel dataset called Diverse Road Damage Dataset (DRDD) for road damage detection that captures the diverse road damage types in individual images, addressing a crucial gap in existing datasets. Then, we provide our model, RDD4D, that exploits Attention4D blocks, enabling better feature refinement across multiple scales. The Attention4D module processes feature maps through an attention mechanism combining positional encoding and “Talking Head” components to capture local and global contextual information. In our comprehensive experimental analysis comparing various state-of-the-art models on our proposed, our enhanced model demonstrated superior performance in detecting large-sized road cracks with an Average Precision (AP) of 0.458 and maintained competitive performance with an overall AP of 0.445. Moreover, we also provide results on the CrackTinyNet dataset; our model achieved around a 0.21 increase in performance. The code, model weights, dataset, and our results are available on \href{https://github.com/msaqib17/Road_Damage_Detection}{https://github.com/msaqib17/Road\_Damage\_Detection}.
道路损伤检测和评估是基础设施维护的重要组成部分。然而,当前的方法往往难以在单张图像中检测多种道路损伤,尤其是在不同尺度上。这是由于缺乏包含各种损伤类型的道路数据集。为了弥补这一缺陷,我们首先推出了一个新的数据集,名为多样化道路损伤数据集(DRDD),用于道路损伤检测,该数据集捕捉了单张图像中的多种道路损伤类型,填补了现有数据集的空白。接着,我们提供了我们的模型RDD4D,该模型利用Attention4D块,能够在多个尺度上实现更好的特征细化。Attention4D模块通过注意力机制处理特征图,结合位置编码和“Talking Head”组件,以捕获局部和全局上下文信息。在我们提出的模型上,我们对各种最新模型进行了全面的实验分析比较,增强后的模型在检测大型道路裂缝方面表现出卓越的性能,平均精度(AP)为0.458,总体平均精度为0.445,保持了竞争力。此外,我们在CrackTinyNet数据集上的模型性能也提高了约0.21。代码、模型权重、数据集和我们的结果可在https://github.com/msaqib17/Road_Damage_Detection上找到。
论文及项目相关链接
Summary
本文介绍了一种新型的路面损伤检测数据集——多样路面损伤数据集(DRDD),以及一个针对该数据集的模型RDD4D。RDD4D利用Attention4D模块,能在不同尺度上更好地进行特征细化。实验表明,RDD4D在检测大型路面裂缝方面表现出卓越性能,并在整体平均精度上保持竞争力。相关代码、模型权重、数据集及结果已公开分享。
Key Takeaways
- 提出了多样路面损伤数据集(DRDD),解决了现有数据集缺乏多样化路面损伤类型的问题。
- 介绍了RDD4D模型,利用Attention4D模块,能捕获局部和全局的上下文信息,实现多尺度特征细化。
- RDD4D模型在检测大型路面裂缝方面表现出卓越性能,平均精度(AP)达到0.458。
- RDD4D模型在整体平均精度上保持竞争力,总体AP为0.445。
- RDD4D模型在CrackTinyNet数据集上的性能有所提升,实现了约0.21的增长。
- 相关代码、模型权重、数据集及结果已公开分享,便于后续研究使用。
点此查看论文截图
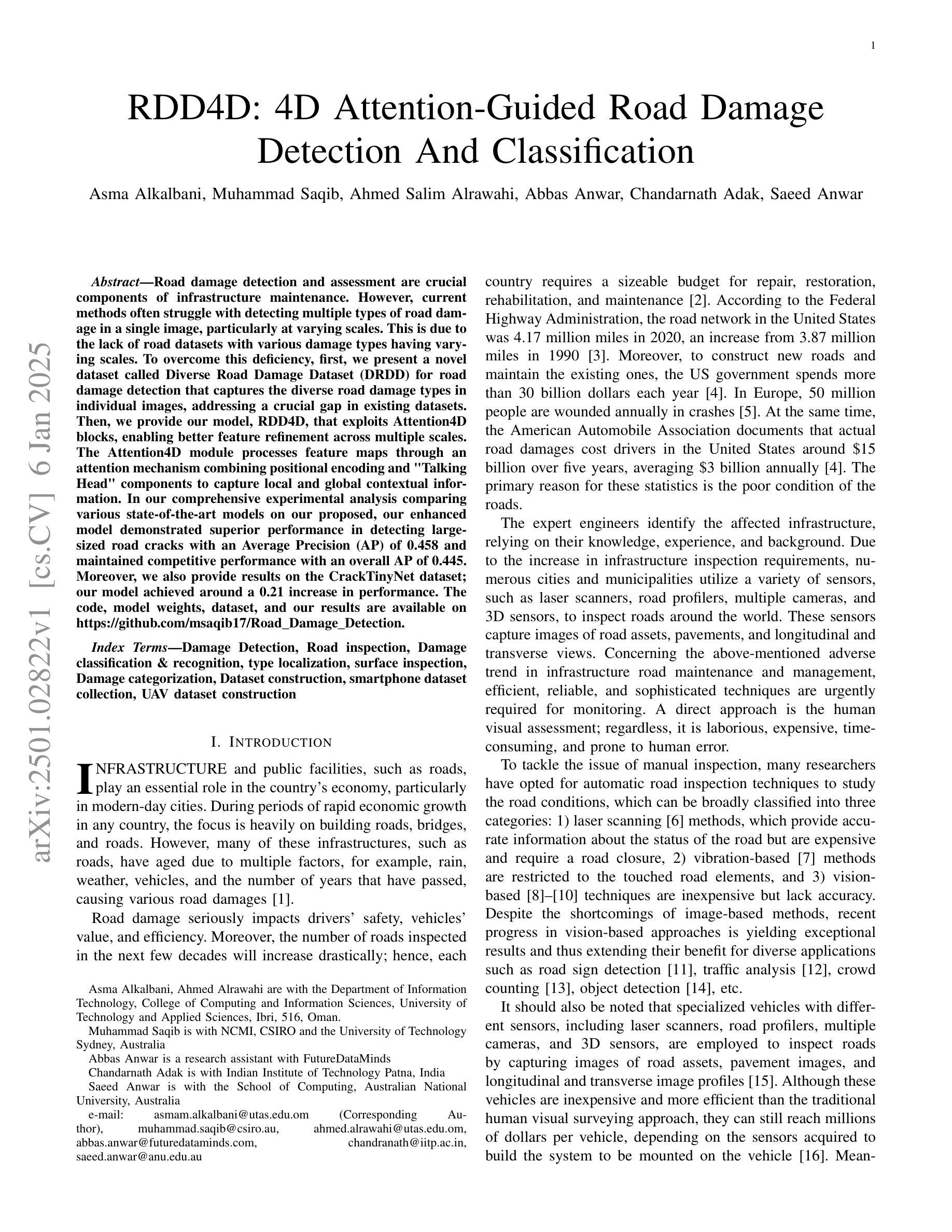
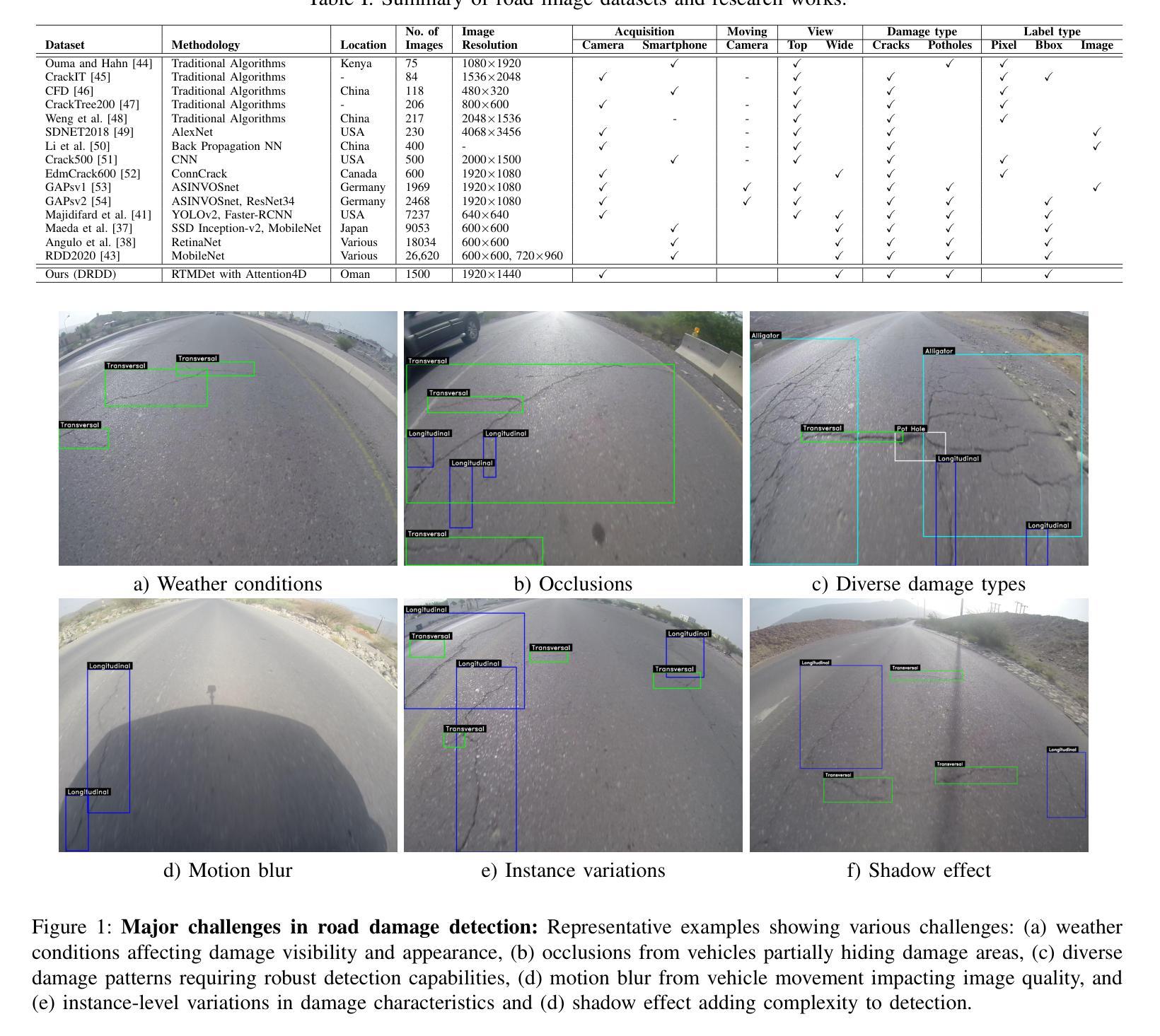
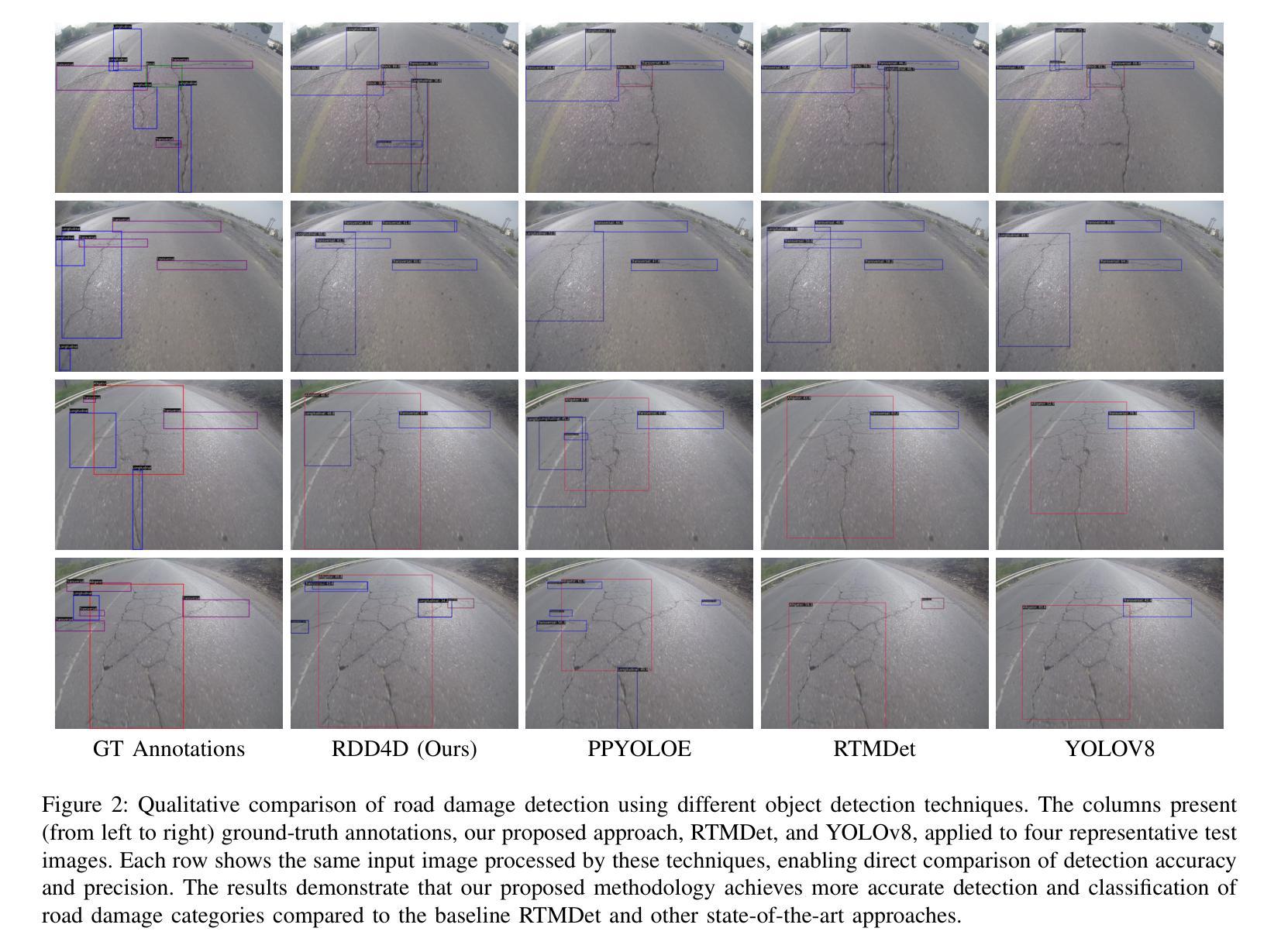
VideoAnydoor: High-fidelity Video Object Insertion with Precise Motion Control
Authors:Yuanpeng Tu, Hao Luo, Xi Chen, Sihui Ji, Xiang Bai, Hengshuang Zhao
Despite significant advancements in video generation, inserting a given object into videos remains a challenging task. The difficulty lies in preserving the appearance details of the reference object and accurately modeling coherent motions at the same time. In this paper, we propose VideoAnydoor, a zero-shot video object insertion framework with high-fidelity detail preservation and precise motion control. Starting from a text-to-video model, we utilize an ID extractor to inject the global identity and leverage a box sequence to control the overall motion. To preserve the detailed appearance and meanwhile support fine-grained motion control, we design a pixel warper. It takes the reference image with arbitrary key-points and the corresponding key-point trajectories as inputs. It warps the pixel details according to the trajectories and fuses the warped features with the diffusion U-Net, thus improving detail preservation and supporting users in manipulating the motion trajectories. In addition, we propose a training strategy involving both videos and static images with a weighted loss to enhance insertion quality. VideoAnydoor demonstrates significant superiority over existing methods and naturally supports various downstream applications (e.g., talking head generation, video virtual try-on, multi-region editing) without task-specific fine-tuning.
尽管视频生成领域已经取得了重大进展,但在视频中插入给定对象仍然是一项具有挑战性的任务。难点在于同时保留参考对象的外貌细节并准确模拟连贯的动作。在本文中,我们提出了VideoAnydoor,这是一个零样本视频对象插入框架,具有高保真细节保留和精确运动控制的特点。我们从文本到视频模型出发,利用ID提取器注入全局身份,并利用框序列控制整体运动。为了保留详细的外观同时支持精细的运动控制,我们设计了一个像素扭曲器。它接受带有任意关键点的参考图像和相应的关键点轨迹作为输入。它根据轨迹扭曲像素细节,并将扭曲的特征与扩散U-Net融合,从而提高了细节保留性,并支持用户操作运动轨迹。此外,我们提出了一种涉及视频和静态图像的训练策略,并使用加权损失来提高插入质量。VideoAnydoor相较于现有方法表现出显著的优势,并且无需特定任务的微调即可自然地支持各种下游应用(例如说话人头生成、视频虚拟试穿、多区域编辑)。
论文及项目相关链接
PDF Project page: https://videoanydoor.github.io/
Summary
本文提出一种名为VideoAnydoor的零样本视频物体插入框架,具备高保真细节保留和精确运动控制特点。该框架从文本到视频模型出发,利用ID提取器注入全局身份,并通过序列框控制整体运动。为保留细节并实现精细运动控制,设计像素扭曲器,其根据轨迹对参考图像进行像素扭曲,并与扩散U-Net融合,从而提高细节保留支持用户操作运动轨迹。此外,本文提出一种结合视频和静态图像的训练策略,并使用加权损失增强插入质量。VideoAnydoor相较于现有方法具有显著优势,可自然应用于各种下游应用,如说话人头部生成、视频虚拟试穿、多区域编辑等,无需特定任务微调。
Key Takeaways
- VideoAnydoor是一个零样本视频物体插入框架,具备高保真细节保留和精确运动控制。
- 该框架从文本到视频模型出发,利用ID提取器注入全局身份。
- 像素扭曲器设计用于保留细节并支持精细运动控制,根据轨迹对参考图像进行像素扭曲。
- 像素扭曲器的特点是可以与扩散U-Net融合,提高细节保留效果。
- 框架中提出一种结合视频和静态图像的训练策略,并使用加权损失以增强插入质量。
- VideoAnydoor在多种下游应用上表现优越,如说话人头部生成、视频虚拟试穿、多区域编辑等。
点此查看论文截图
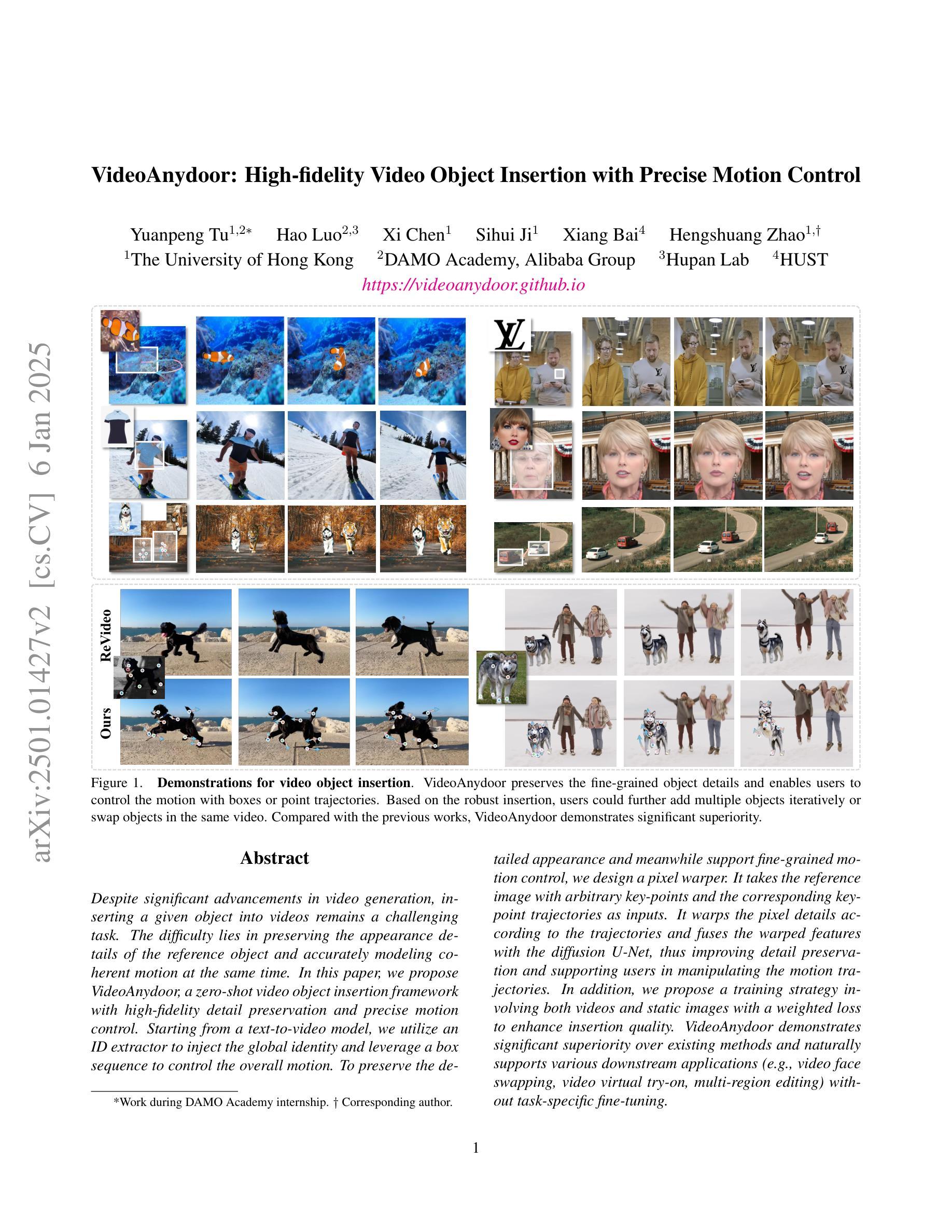
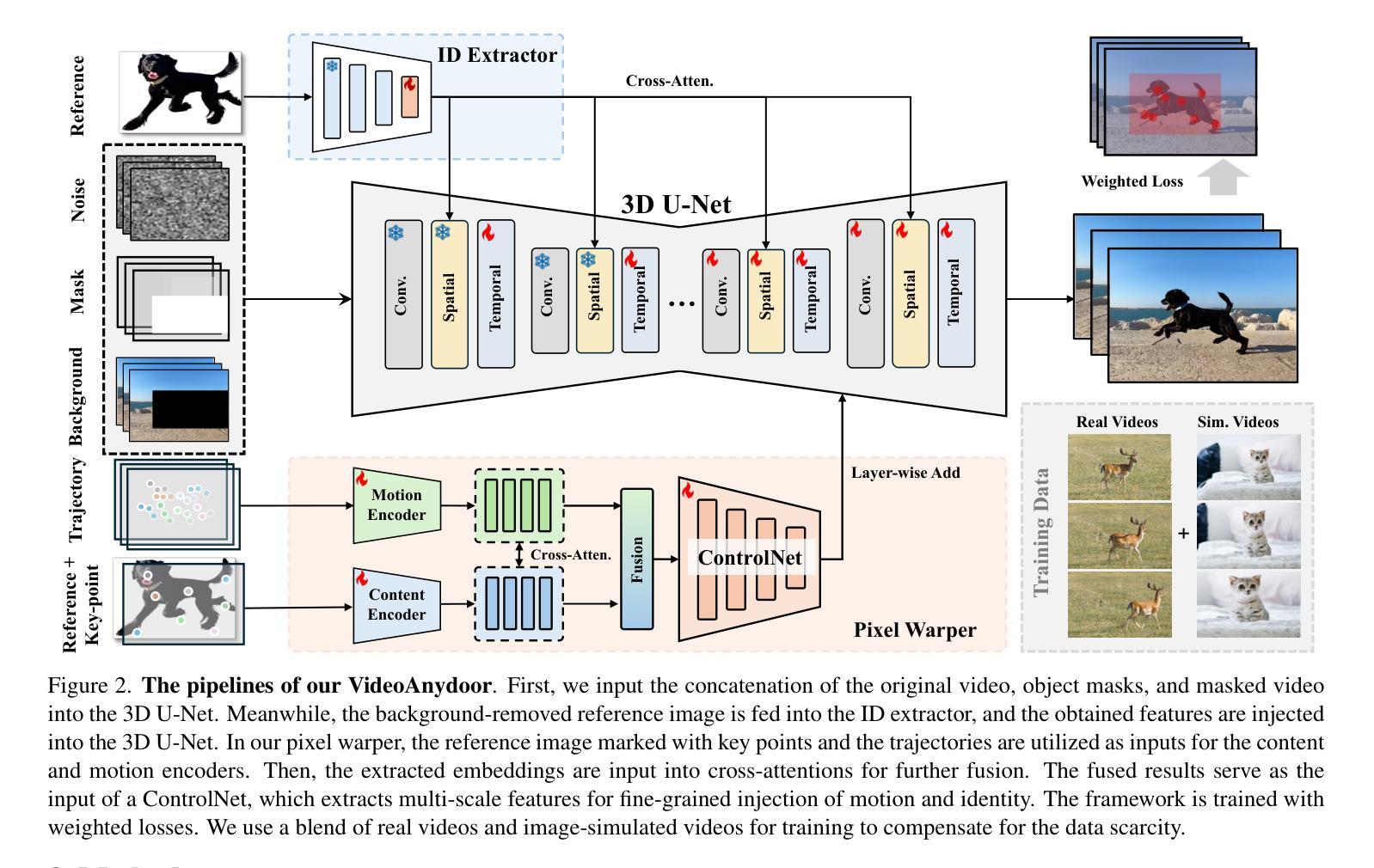
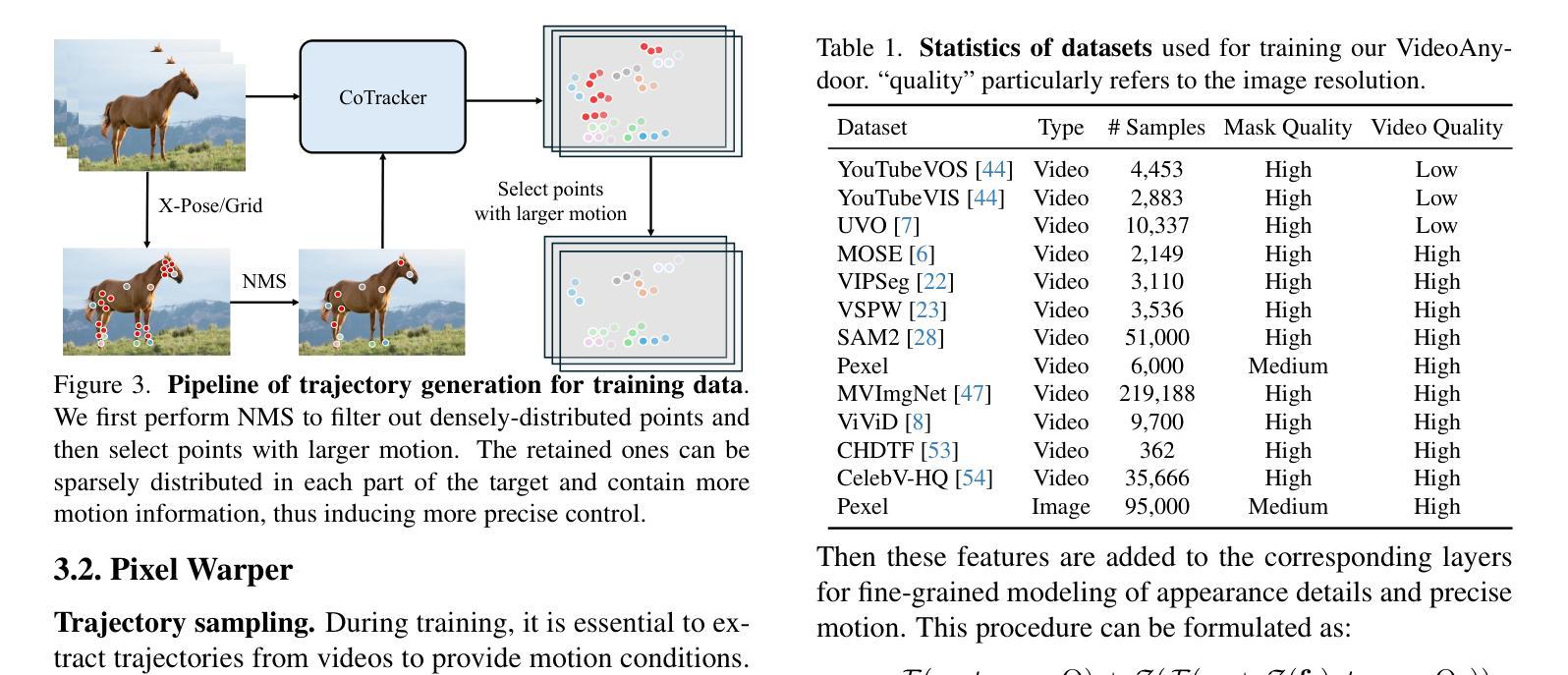
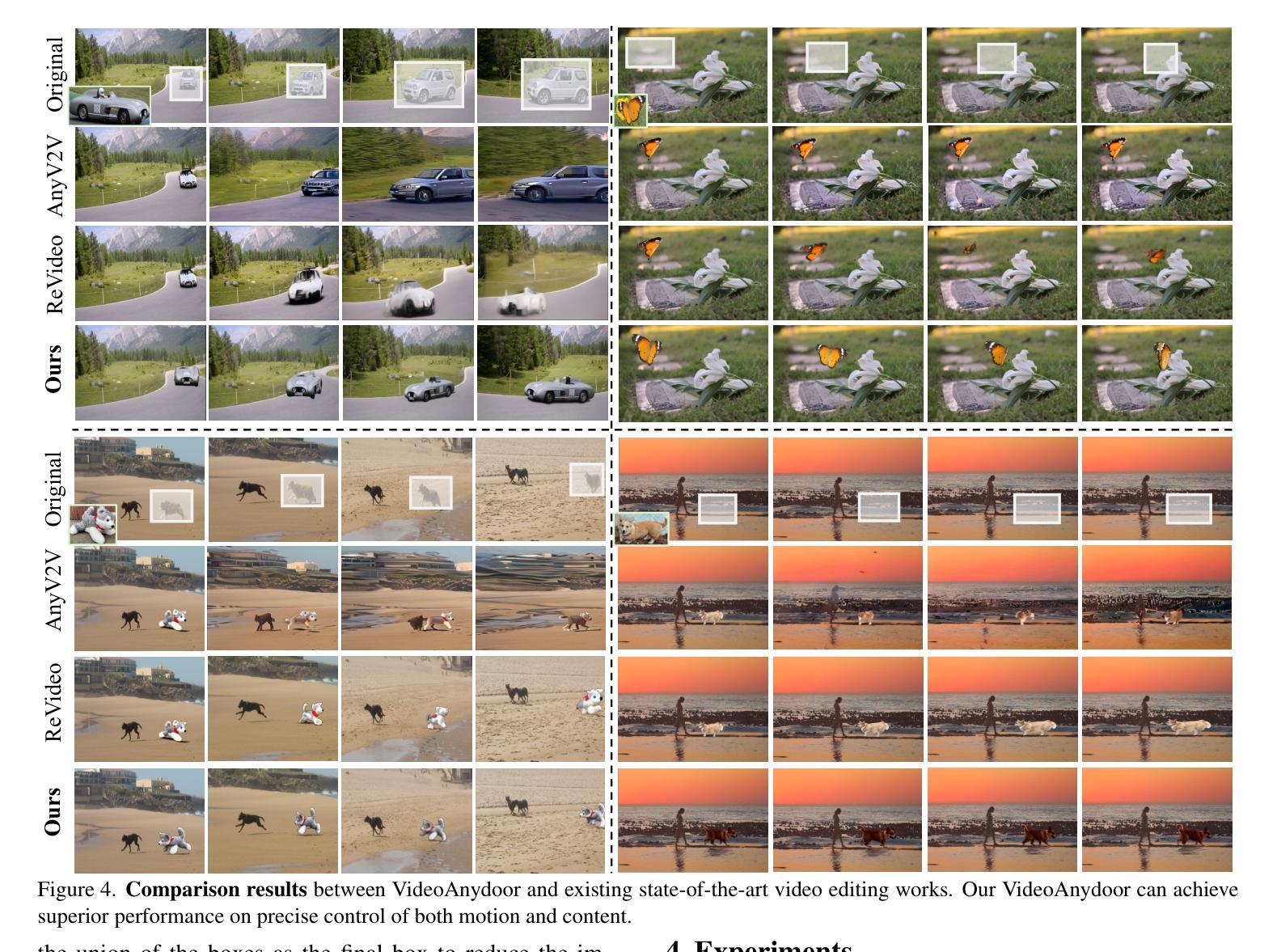
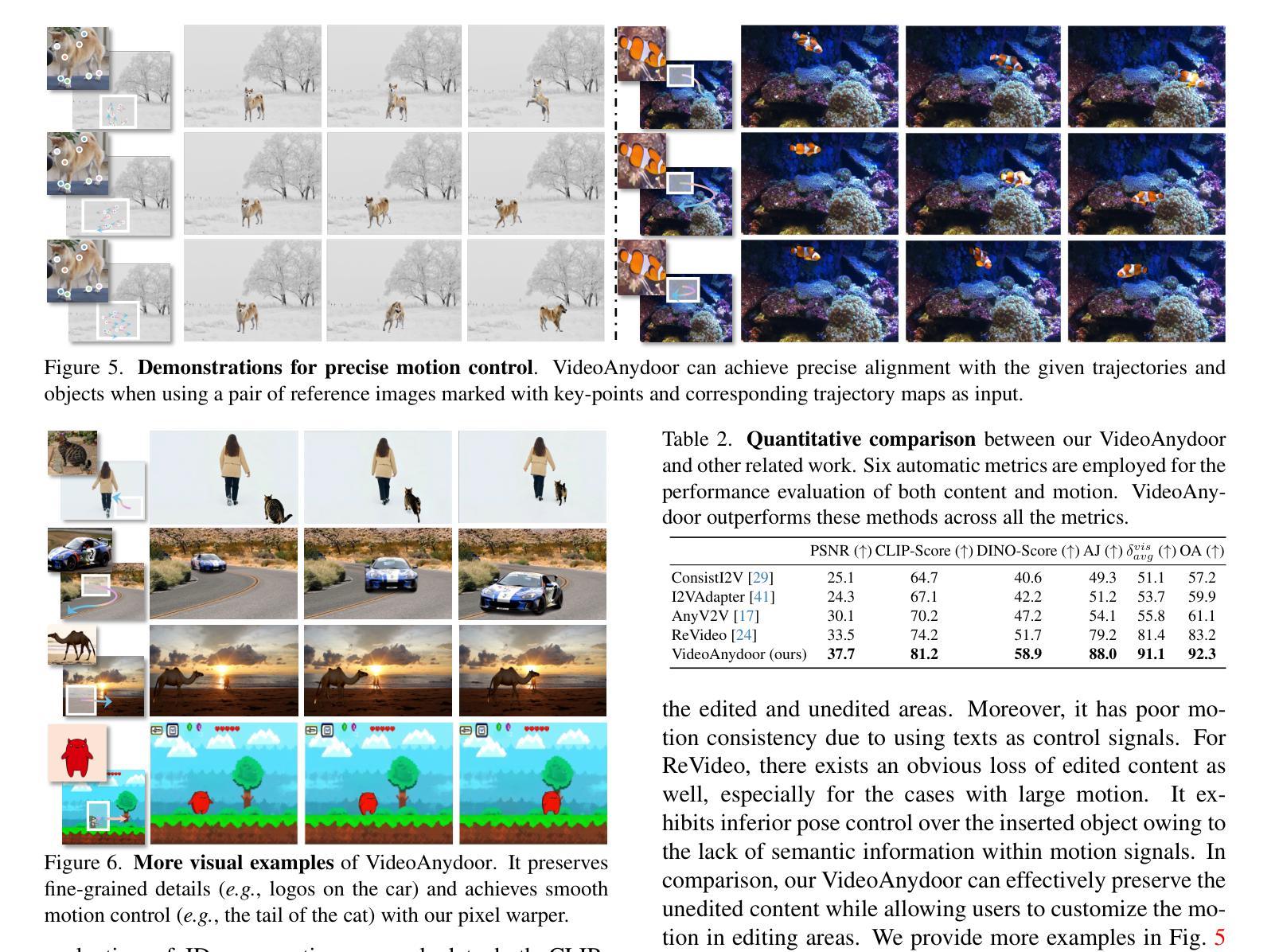