⚠️ 以下所有内容总结都来自于 大语言模型的能力,如有错误,仅供参考,谨慎使用
🔴 请注意:千万不要用于严肃的学术场景,只能用于论文阅读前的初筛!
💗 如果您觉得我们的项目对您有帮助 ChatPaperFree ,还请您给我们一些鼓励!⭐️ HuggingFace免费体验
2025-01-09 更新
CL3DOR: Contrastive Learning for 3D Large Multimodal Models via Odds Ratio on High-Resolution Point Clouds
Authors:Keonwoo Kim, Yeongjae Cho, Taebaek Hwang, Minsoo Jo, Sangdo Han
Recent research has demonstrated that Large Language Models (LLMs) are not limited to text-only tasks but can also function as multimodal models across various modalities, including audio, images, and videos. In particular, research on 3D Large Multimodal Models (3D LMMs) is making notable strides, driven by the potential of processing higher-dimensional data like point clouds. However, upon closer examination, we find that the visual and textual content within each sample of existing training datasets lacks both high informational granularity and clarity, which serve as a bottleneck for precise cross-modal understanding. To address these issues, we propose CL3DOR, Contrastive Learning for 3D large multimodal models via Odds ratio on high-Resolution point clouds, designed to ensure greater specificity and clarity in both visual and textual content. Specifically, we increase the density of point clouds per object and construct informative hard negative responses in the training dataset to penalize unwanted responses. To leverage hard negative responses, we incorporate the odds ratio as an auxiliary term for contrastive learning into the conventional language modeling loss. CL3DOR achieves state-of-the-art performance in 3D scene understanding and reasoning benchmarks. Additionally, we demonstrate the effectiveness of CL3DOR’s key components through extensive experiments.
最新的研究表明,大型语言模型(LLM)不仅限于文本任务,还可以作为跨多种模式的多媒体模型,包括音频、图像和视频。特别是,关于3D大型多媒体模型(3D LMM)的研究正在取得显著的进步,这得益于处理点云等高维数据的潜力。然而,经过仔细观察,我们发现现有训练数据集每个样本中的视觉和文本内容缺乏高度信息粒度和清晰度,这成为精确跨模态理解的瓶颈。为了解决这些问题,我们提出了CL3DOR,即通过高分辨率点云的赔率对比学习3D大型多媒体模型,旨在确保视觉和文本内容具有更高的特异性和清晰度。具体来说,我们增加了每个对象的点云密度,并在训练数据集中构建有信息量的硬负响应来惩罚不需要的响应。为了利用硬负响应,我们将赔率比作为对比学习的辅助术语纳入常规语言建模损失中。CL3DOR在3D场景理解和推理基准测试中达到了最先进的性能。此外,我们还通过大量实验证明了CL3DOR关键组件的有效性。
论文及项目相关链接
Summary
本文研究了大型语言模型在多模态领域的应用,特别是在处理高维数据如点云方面的潜力。然而,现有训练数据集样本中的视觉和文本内容缺乏高信息粒度和清晰度,成为精确跨模态理解的瓶颈。为此,提出了CL3DOR方法,通过高分辨率点云的赔率对比学习,确保视觉和文本内容更具特异性和清晰度。该方法通过增加点云对象的密度和构建训练数据集中的信息性硬负响应来惩罚不需要的响应。CL3DOR在3D场景理解和推理基准测试中实现了最佳性能,并通过大量实验验证了其关键组件的有效性。
Key Takeaways
- 大型语言模型(LLM)不仅能处理文本任务,还能作为多模态模型处理音频、图像和视频等多种模态数据。
- 3D大型多模态模型(3D LMM)在处理高维数据如点云方面有很大潜力。
- 现有训练数据集样本的视觉和文本内容缺乏高信息粒度和清晰度,这是精确跨模态理解的主要障碍。
- CL3DOR方法通过高分辨率点云的对比学习来提高特异性和清晰度,解决上述问题。
- CL3DOR方法通过增加点云对象的密度和构建信息性硬负响应来优化模型。
- CL3DOR在3D场景理解和推理方面达到了最佳性能。
点此查看论文截图
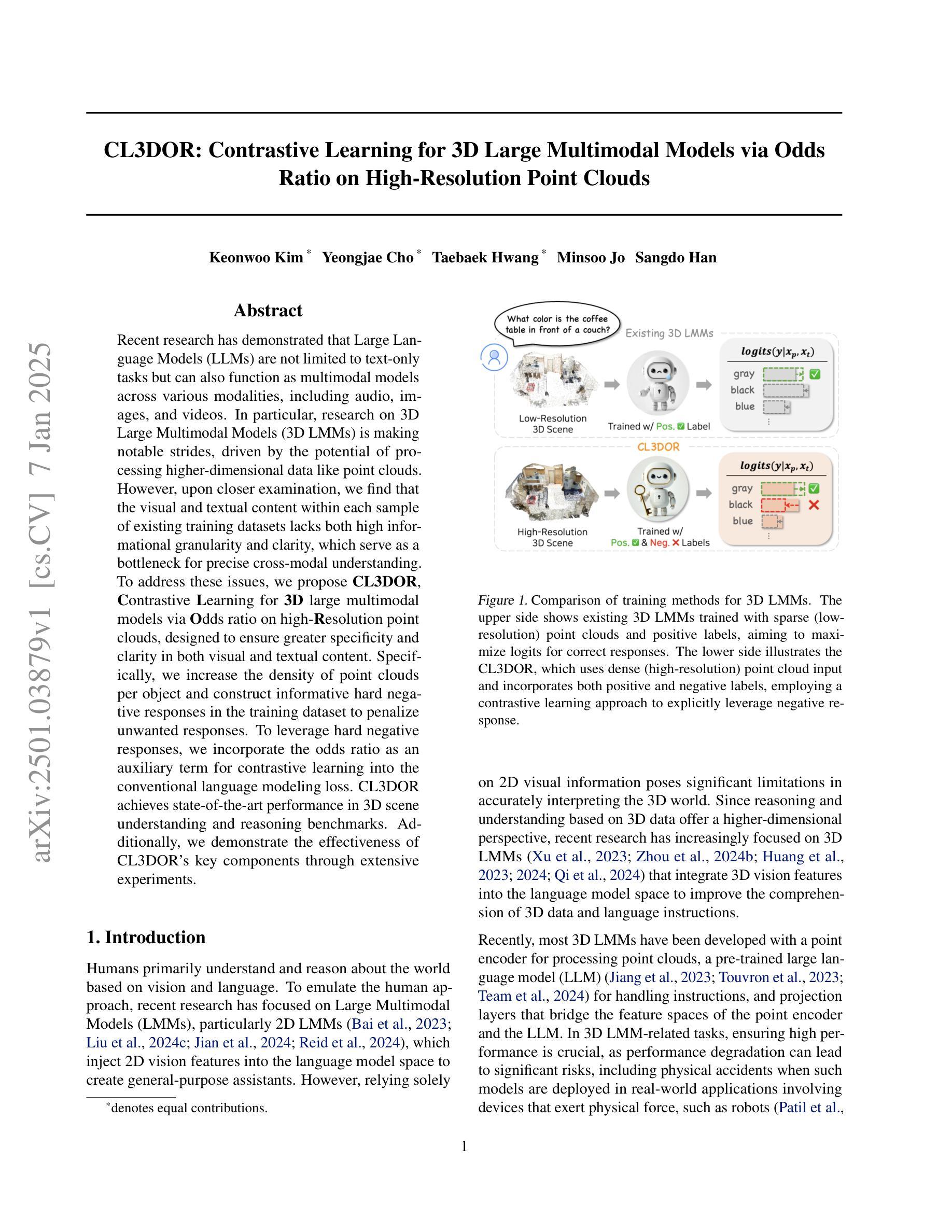
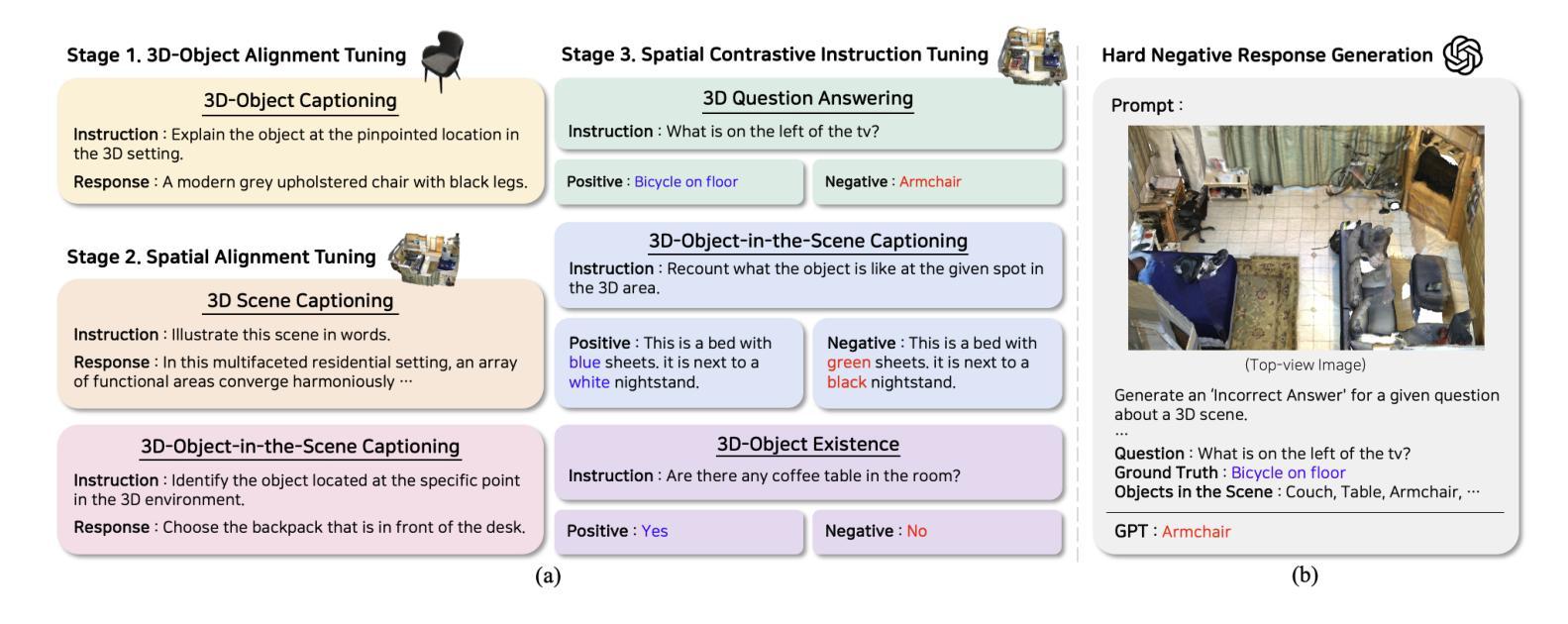
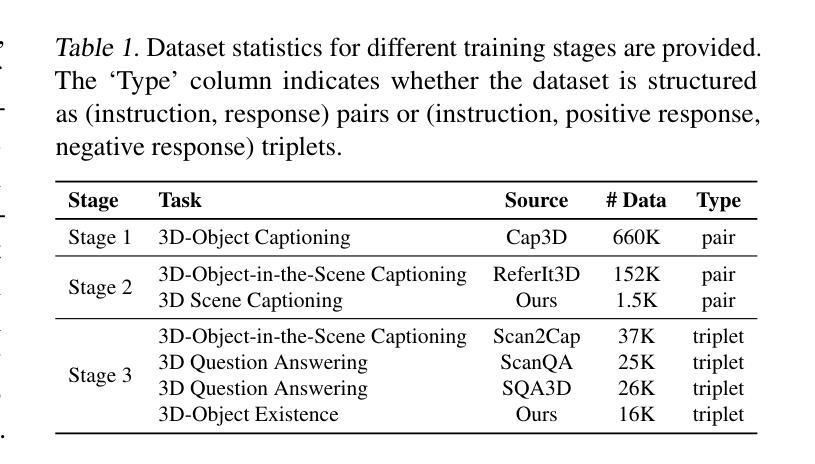
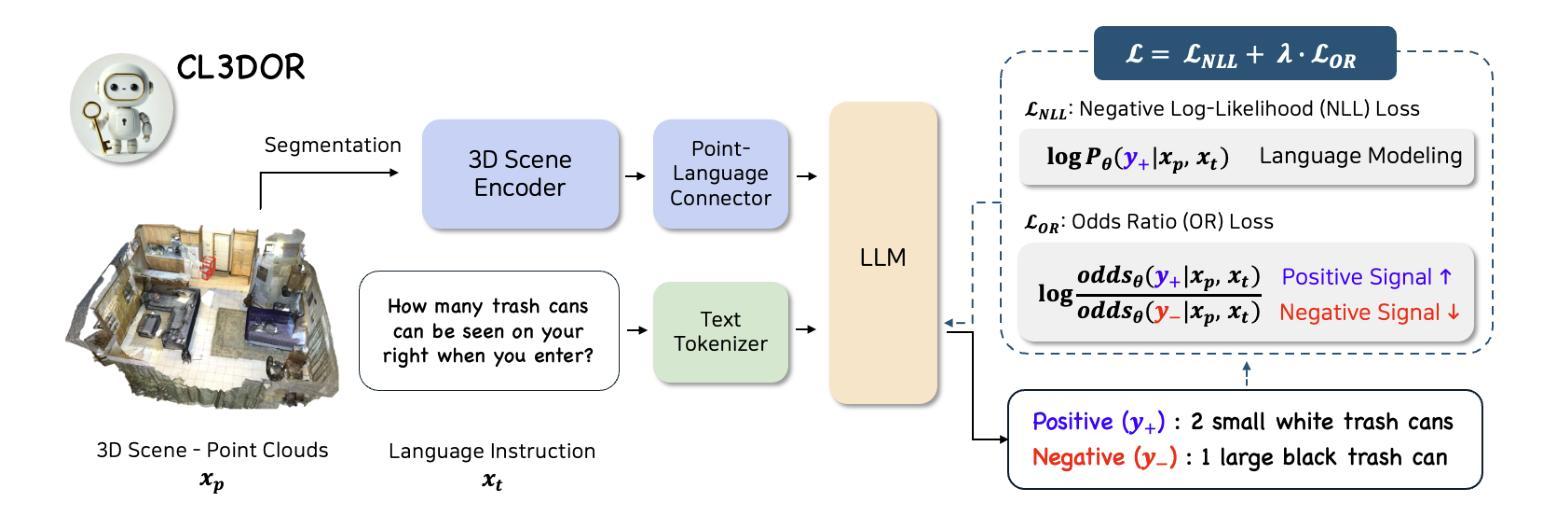
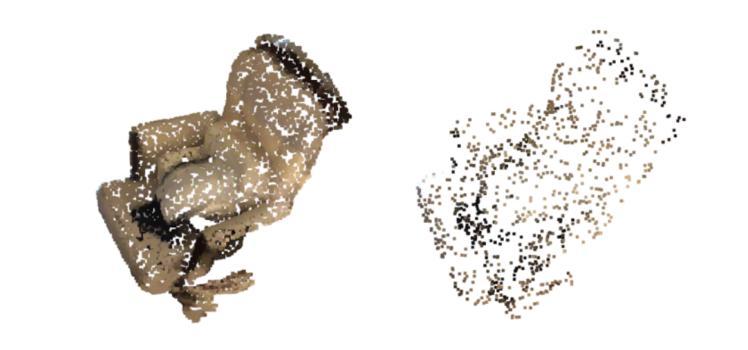
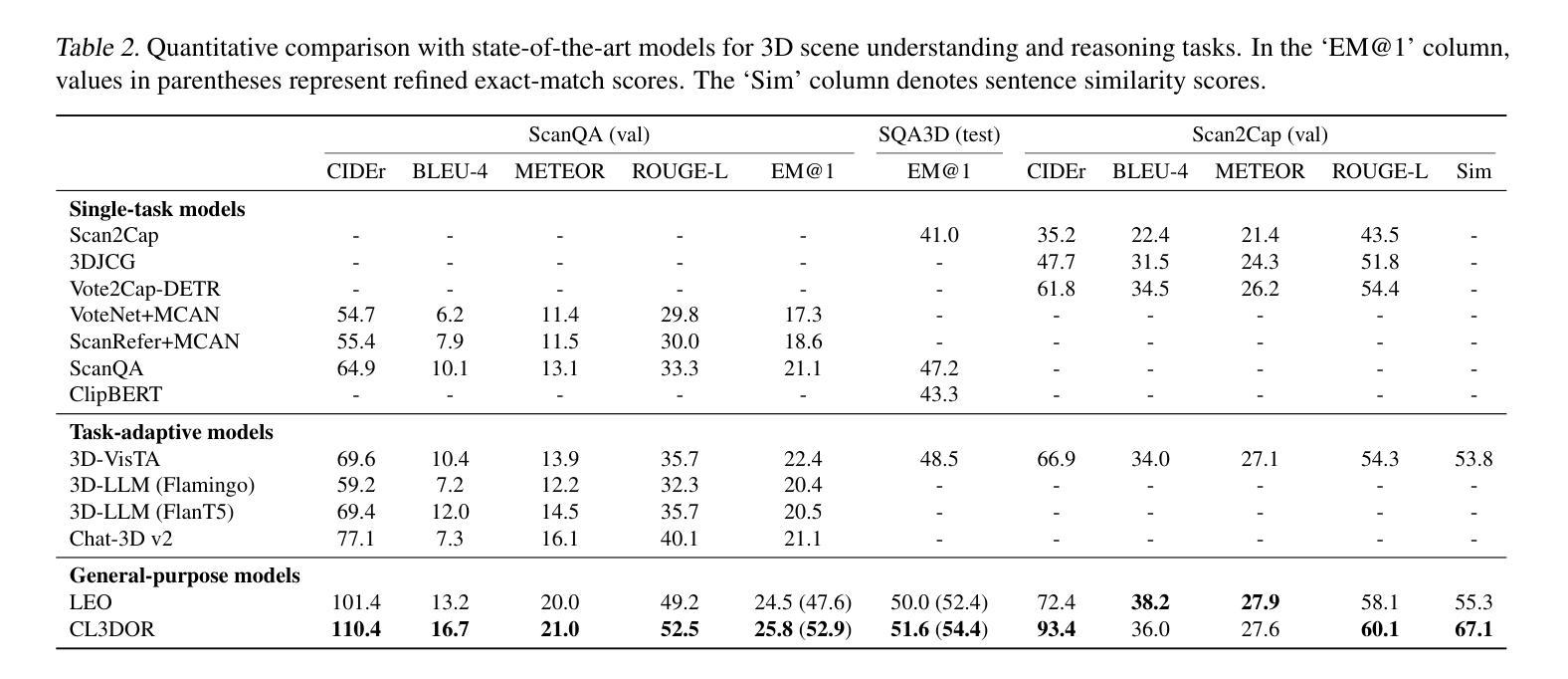
Hyperbolic Contrastive Learning for Hierarchical 3D Point Cloud Embedding
Authors:Yingjie Liu, Pengyu Zhang, Ziyao He, Mingsong Chen, Xuan Tang, Xian Wei
Hyperbolic spaces allow for more efficient modeling of complex, hierarchical structures, which is particularly beneficial in tasks involving multi-modal data. Although hyperbolic geometries have been proven effective for language-image pre-training, their capabilities to unify language, image, and 3D Point Cloud modalities are under-explored. We extend the 3D Point Cloud modality in hyperbolic multi-modal contrastive pre-training. Additionally, we explore the entailment, modality gap, and alignment regularizers for learning hierarchical 3D embeddings and facilitating the transfer of knowledge from both Text and Image modalities. These regularizers enable the learning of intra-modal hierarchy within each modality and inter-modal hierarchy across text, 2D images, and 3D Point Clouds. Experimental results demonstrate that our proposed training strategy yields an outstanding 3D Point Cloud encoder, and the obtained 3D Point Cloud hierarchical embeddings significantly improve performance on various downstream tasks.
超球面空间允许对复杂、层次结构进行更有效的建模,这在涉及多模态数据的任务中特别有益。尽管超几何已被证明在语言图像预训练中是有效的,但其在统一语言、图像和3D点云模态方面的能力尚未得到充分探索。我们在超球面多模态对比预训练中扩展了3D点云模态。此外,我们探索了蕴涵、模态间隙和对齐正则化器,用于学习层次化的3D嵌入并促进文本和图像两种模态的知识迁移。这些正则化器能够在每个模态内部学习模态内层次结构以及跨文本、二维图像和3D点云的模态间层次结构。实验结果表明,我们提出的训练策略产生了出色的3D点云编码器,所获得的3D点云层次嵌入显著提高了各种下游任务的性能。
论文及项目相关链接
总结
本研究探索了超球面空间在多模态预训练中的潜力,特别是在整合语言、图像和3D点云数据方面。研究通过引入超球面几何预训练,扩展了3D点云模态的应用,并探讨了蕴涵、模态差距和对齐正则化在学习分层3D嵌入和促进跨文本和图像模态的知识迁移中的作用。实验结果表明,该研究提出的训练策略对3D点云编码器表现有显著提升,且获得的分层嵌入在多个下游任务上表现出优异性能。
关键见解
- 超球面空间能更有效地建模复杂的层次结构,尤其有助于多模态数据的任务。
- 研究扩展了超球面多模态对比预训练中的3D点云模态应用。
- 引入蕴涵、模态差距和对齐正则化,用于学习跨文本、图像和3D点云的跨模态和模态内层次结构。
- 正则化有助于学习每个模态内的层次结构和跨不同模态的层次结构。
- 实验证明,所提出的训练策略在优化3D点云编码器方面具有显著效果。
- 获得的分层3D点云嵌入在多个下游任务上表现出优异性能。
点此查看论文截图
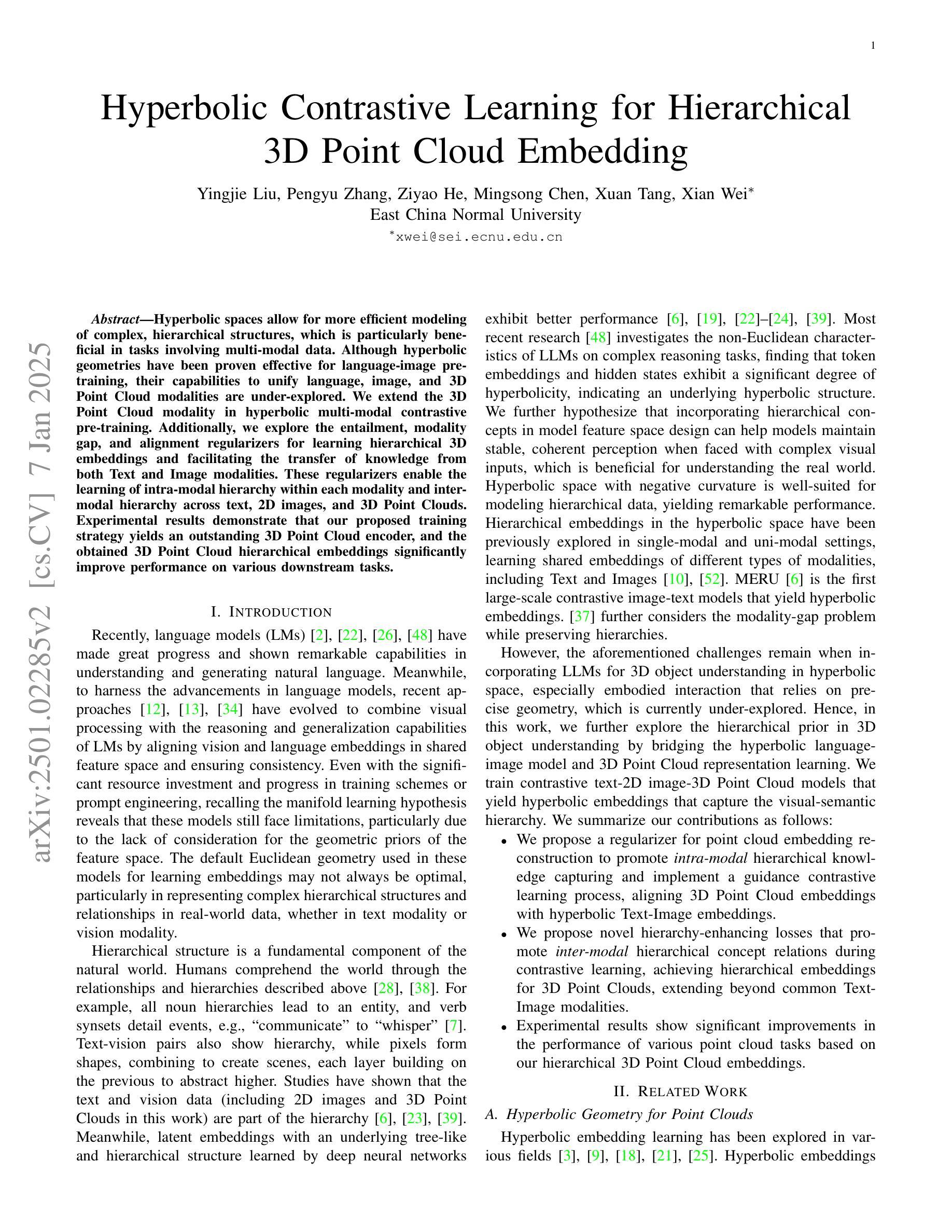
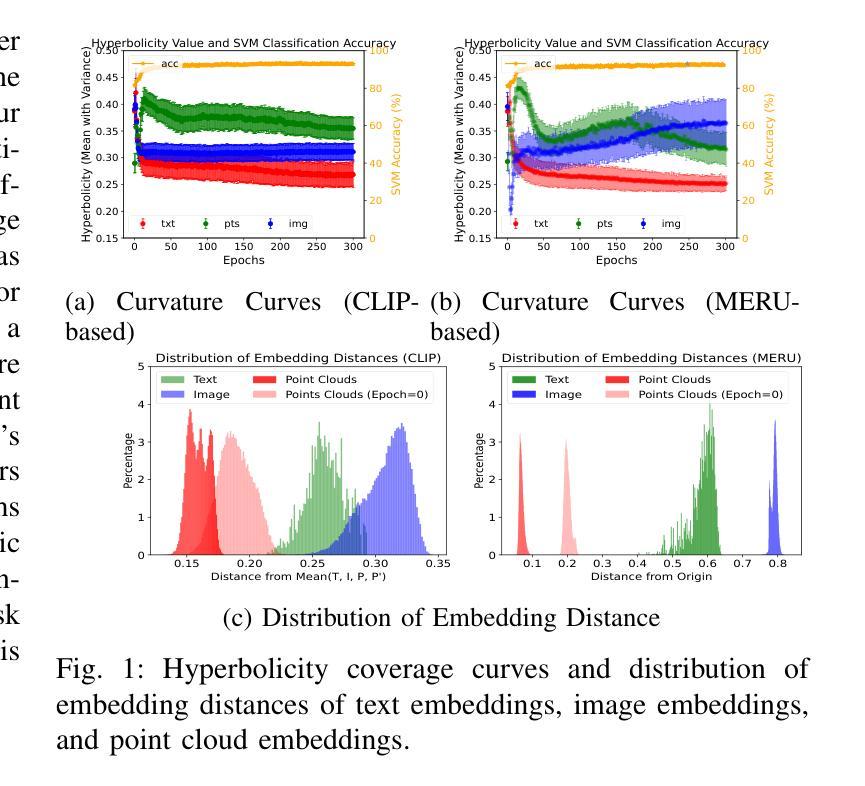
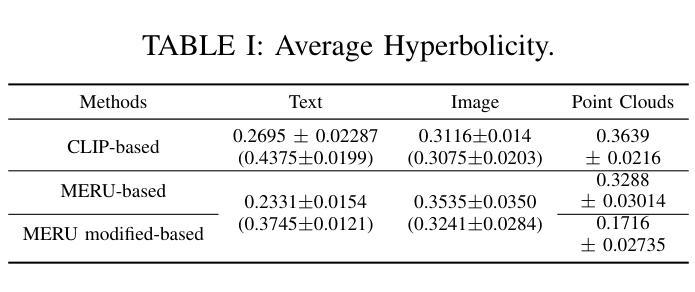
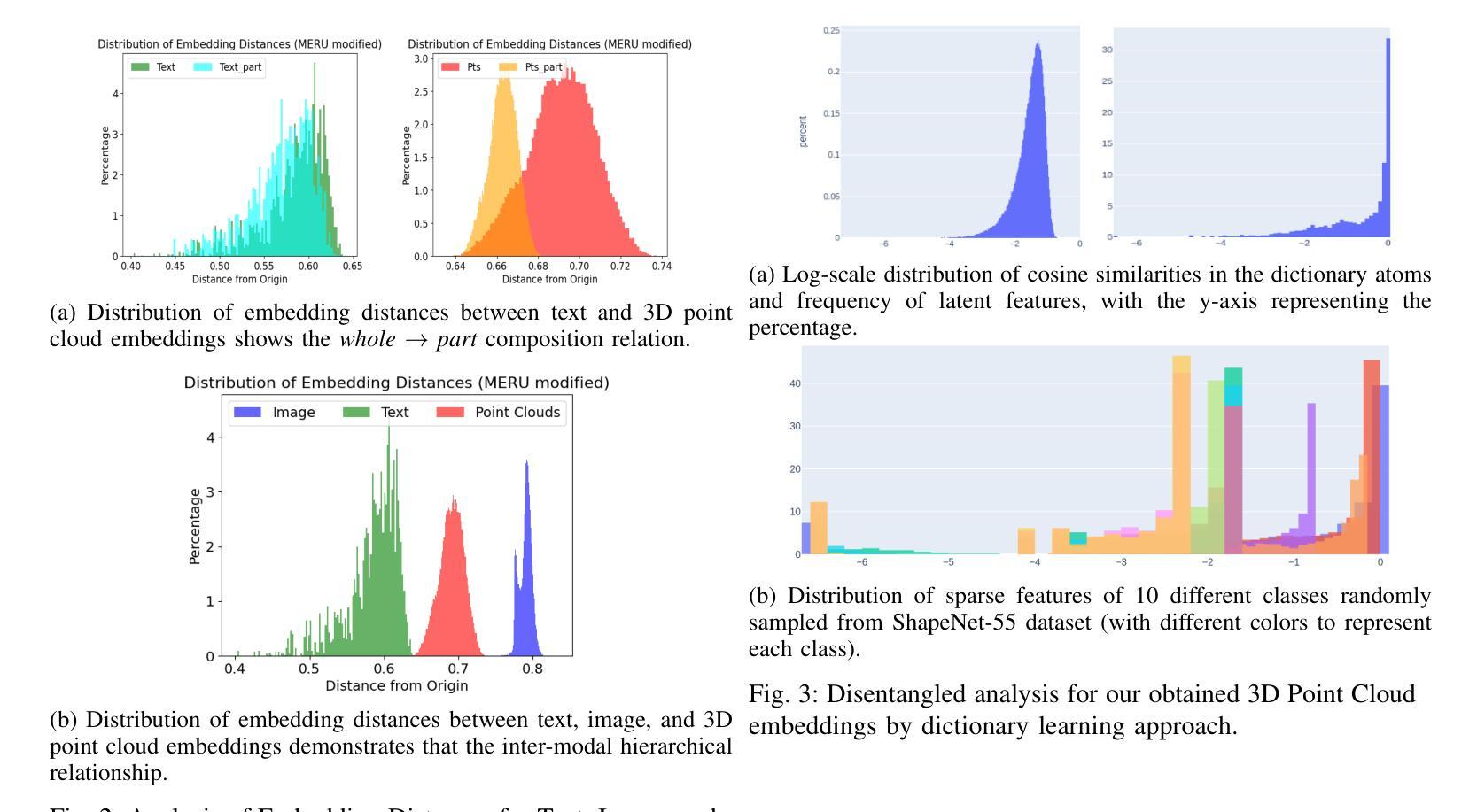