⚠️ 以下所有内容总结都来自于 大语言模型的能力,如有错误,仅供参考,谨慎使用
🔴 请注意:千万不要用于严肃的学术场景,只能用于论文阅读前的初筛!
💗 如果您觉得我们的项目对您有帮助 ChatPaperFree ,还请您给我们一些鼓励!⭐️ HuggingFace免费体验
2025-01-09 更新
ZDySS – Zero-Shot Dynamic Scene Stylization using Gaussian Splatting
Authors:Abhishek Saroha, Florian Hofherr, Mariia Gladkova, Cecilia Curreli, Or Litany, Daniel Cremers
Stylizing a dynamic scene based on an exemplar image is critical for various real-world applications, including gaming, filmmaking, and augmented and virtual reality. However, achieving consistent stylization across both spatial and temporal dimensions remains a significant challenge. Most existing methods are designed for static scenes and often require an optimization process for each style image, limiting their adaptability. We introduce ZDySS, a zero-shot stylization framework for dynamic scenes, allowing our model to generalize to previously unseen style images at inference. Our approach employs Gaussian splatting for scene representation, linking each Gaussian to a learned feature vector that renders a feature map for any given view and timestamp. By applying style transfer on the learned feature vectors instead of the rendered feature map, we enhance spatio-temporal consistency across frames. Our method demonstrates superior performance and coherence over state-of-the-art baselines in tests on real-world dynamic scenes, making it a robust solution for practical applications.
基于范例图像对动态场景进行风格化在各种现实世界应用(包括游戏、电影制作和增强现实及虚拟现实)中至关重要。然而,在空间和时间的维度上实现一致的风格化仍然是一个巨大的挑战。大多数现有方法都是针对静态场景的,并且经常需要对每种风格图像进行优化处理,这限制了它们的适应性。我们引入了ZDySS,这是一种用于动态场景的无源风格化框架,使我们的模型能够在推断时推广到之前未见过的风格图像。我们的方法采用高斯拼贴技术进行场景表示,将每个高斯与一个学习的特征向量相关联,为特征映射的任何给定视图和时间戳生成特征映射。我们在学习的特征向量上应用风格转移,而不是在渲染的特征映射上,从而提高了跨帧的时空一致性。我们的方法在现实世界动态场景的测试中对最先进的基线进行了展示,表现出卓越的性能和连贯性,使其成为实际应用中可靠的解决方案。
论文及项目相关链接
Summary
动态场景基于示例图像进行风格化对于游戏、电影制作、增强和虚拟现实等实际应用至关重要。然而,在空间和时间的维度上实现一致的风格化仍是一大挑战。现有的方法多为静态场景设计,针对每种风格图像都需要优化过程,这限制了其适应性。我们推出ZDySS,一个用于动态场景的无射击风格化框架,使模型能够在推理时推广到以前未见过的风格图像。我们的方法采用高斯斑点技术进行场景表示,将每个高斯与学习的特征向量联系起来,为给定视图和时间戳呈现特征图。我们在学习的特征向量上应用风格转移,而不是在呈现的特征图上,这增强了帧之间的时空一致性。我们的方法在真实动态场景测试中的表现和连贯性都超越了最先进的基线,成为实际应用中稳健的解决方案。
Key Takeaways
- 动态场景的风格化对于游戏、电影制作、增强和虚拟现实等应用非常重要。
- 实现动态场景在空间和时间的风格化一致性是一大挑战。
- 现有方法多针对静态场景设计,缺乏对新风格图像的适应性。
- 推出ZDySS框架,一种用于动态场景的无射击风格化方法。
- 使用高斯斑点技术进行场景表示,将每个高斯与学习的特征向量联系起来。
- 在学习的特征向量上应用风格转移,增强了帧之间的时空一致性。
点此查看论文截图
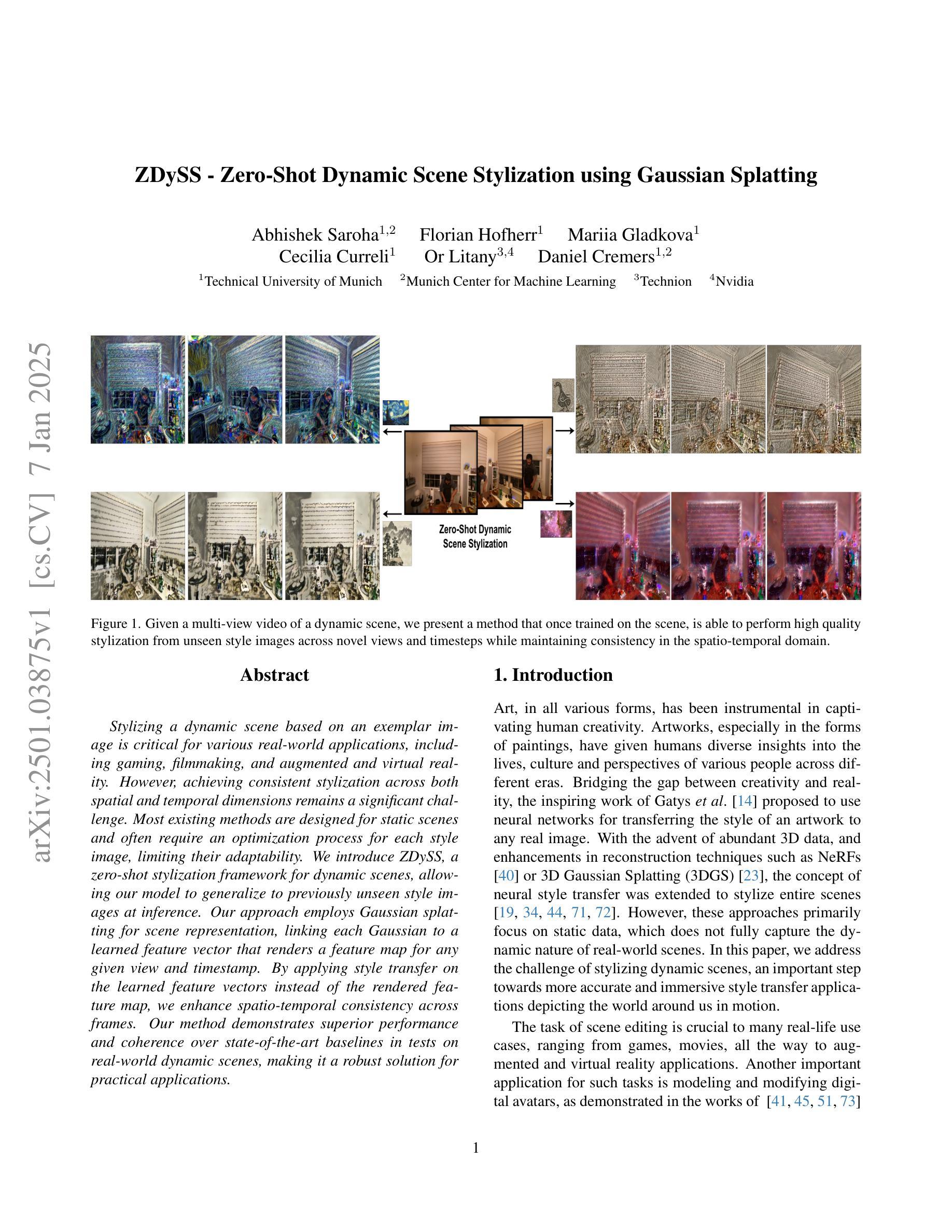
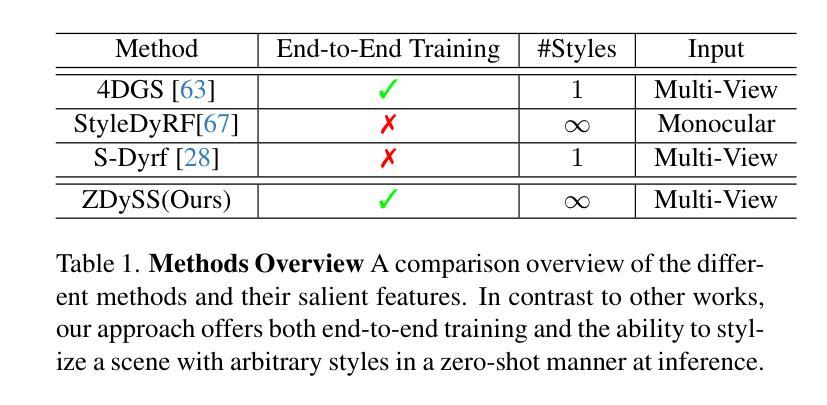
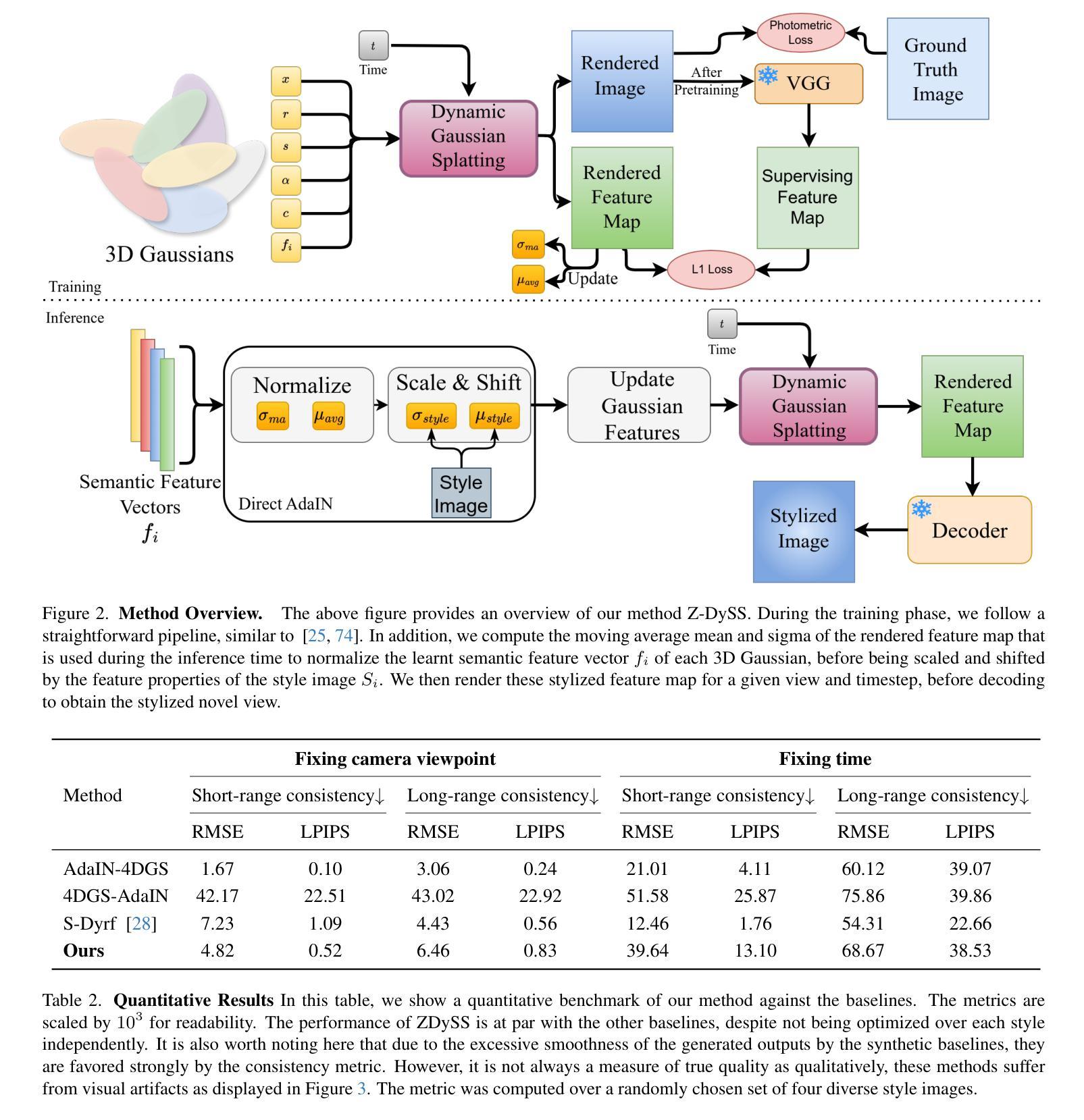
DehazeGS: Seeing Through Fog with 3D Gaussian Splatting
Authors:Jinze Yu, Yiqun Wang, Zhengda Lu, Jianwei Guo, Yong Li, Hongxing Qin, Xiaopeng Zhang
Current novel view synthesis tasks primarily rely on high-quality and clear images. However, in foggy scenes, scattering and attenuation can significantly degrade the reconstruction and rendering quality. Although NeRF-based dehazing reconstruction algorithms have been developed, their use of deep fully connected neural networks and per-ray sampling strategies leads to high computational costs. Moreover, NeRF’s implicit representation struggles to recover fine details from hazy scenes. In contrast, recent advancements in 3D Gaussian Splatting achieve high-quality 3D scene reconstruction by explicitly modeling point clouds into 3D Gaussians. In this paper, we propose leveraging the explicit Gaussian representation to explain the foggy image formation process through a physically accurate forward rendering process. We introduce DehazeGS, a method capable of decomposing and rendering a fog-free background from participating media using only muti-view foggy images as input. We model the transmission within each Gaussian distribution to simulate the formation of fog. During this process, we jointly learn the atmospheric light and scattering coefficient while optimizing the Gaussian representation of the hazy scene. In the inference stage, we eliminate the effects of scattering and attenuation on the Gaussians and directly project them onto a 2D plane to obtain a clear view. Experiments on both synthetic and real-world foggy datasets demonstrate that DehazeGS achieves state-of-the-art performance in terms of both rendering quality and computational efficiency.
当前的新型视图合成任务主要依赖于高质量、清晰的图像。然而,在雾天场景中,散射和衰减会显著降重建和渲染的质量。尽管已经开发了基于NeRF的去雾重建算法,但它们使用深度全连接神经网络和逐射线采样策略,导致计算成本高昂。此外,NeRF的隐式表示很难从雾天场景中恢复细节。相比之下,3D高斯涂斑技术的最新进展通过显式建模点云为3D高斯实现了高质量的三维场景重建。在本文中,我们提出利用显式的高斯表示,通过一个物理准确的前向渲染过程来解释雾天图像的形成过程。我们引入了DehazeGS方法,该方法能够从参与介质中分解并渲染无雾背景,仅使用多视角雾天图像作为输入。我们模拟了每个高斯分布内的传输以形成雾。在此过程中,我们联合学习大气光和散射系数,同时优化雾天场景的高斯表示。在推理阶段,我们消除了散射和衰减对高斯的影响,并将其直接投影到二维平面上以获得清晰视图。在合成和真实世界的雾天数据集上的实验表明,DehazeGS在渲染质量和计算效率方面都达到了最先进的性能。
论文及项目相关链接
PDF 9 pages,4 figures
Summary
本文提出一种基于3D高斯散斑(Gaussian Splatting)的去雾新方法,称为DehazeGS。该方法利用显式高斯表示对雾天图像形成过程进行物理准确的前向渲染,能从多视角雾天图像中分解并渲染出无雾背景。通过优化高斯表示,联合学习大气光和散射系数,并在推理阶段消除散射和衰减对高斯的影响,直接将清晰视图投影到2D平面上。实验表明,DehazeGS在渲染质量和计算效率方面均达到最佳水平。
Key Takeaways
- 当前视图合成任务主要依赖于高质量清晰图像,但在雾天场景中,散射和衰减会严重影响重建和渲染质量。
- NeRF的隐式表示在恢复雾天场景的细节方面存在困难,且其深度全连接神经网络和每射线采样策略导致高计算成本。
- 3D高斯散斑技术能实现高质量3D场景重建,通过显式建模点云为3D高斯。
- 本文提出利用显式高斯表示通过物理准确的前向渲染过程解释雾天图像形成过程。
- DehazeGS方法能从多视角雾天图像中分解并渲染出无雾背景。
- DehazeGS通过优化高斯表示、联合学习大气光和散射系数来提升性能。
点此查看论文截图
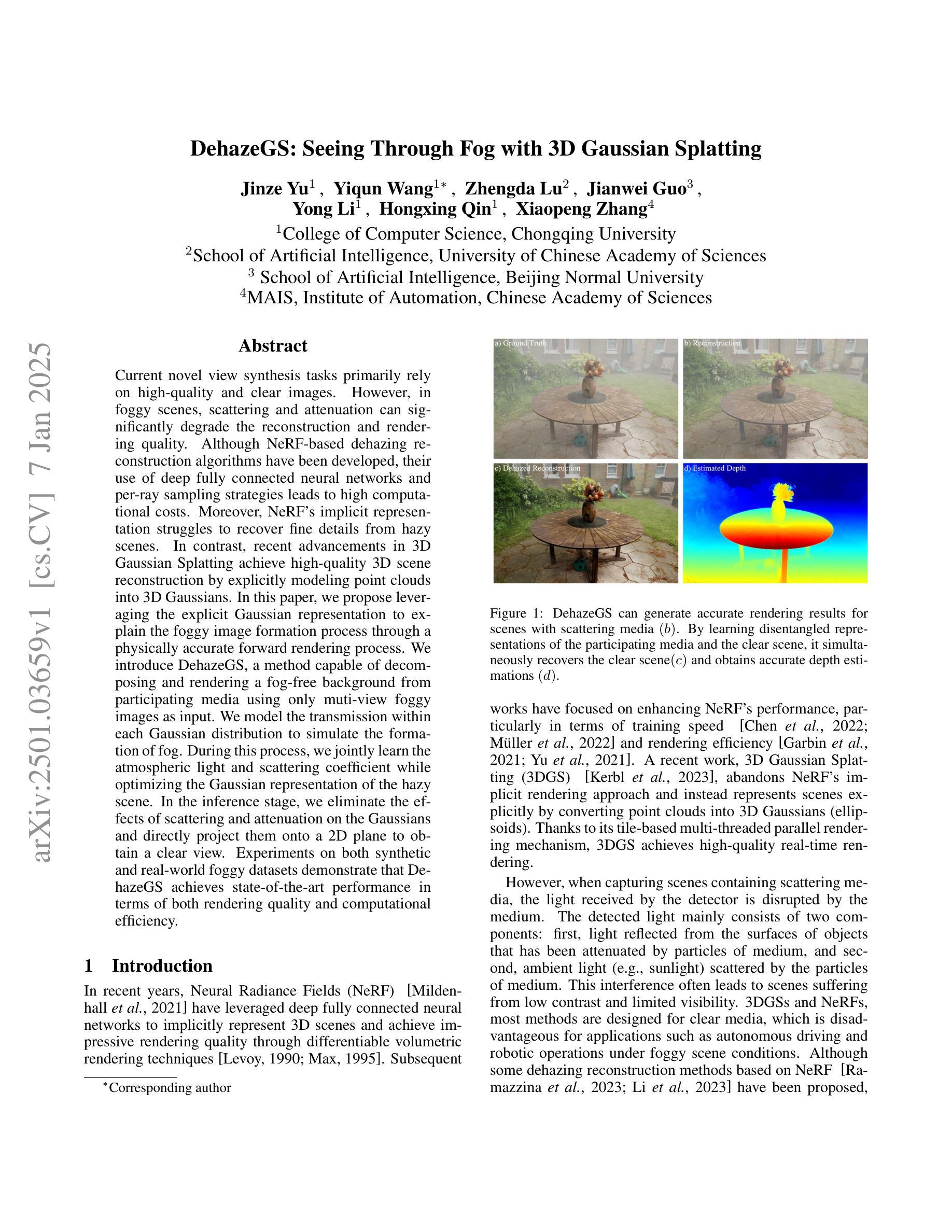
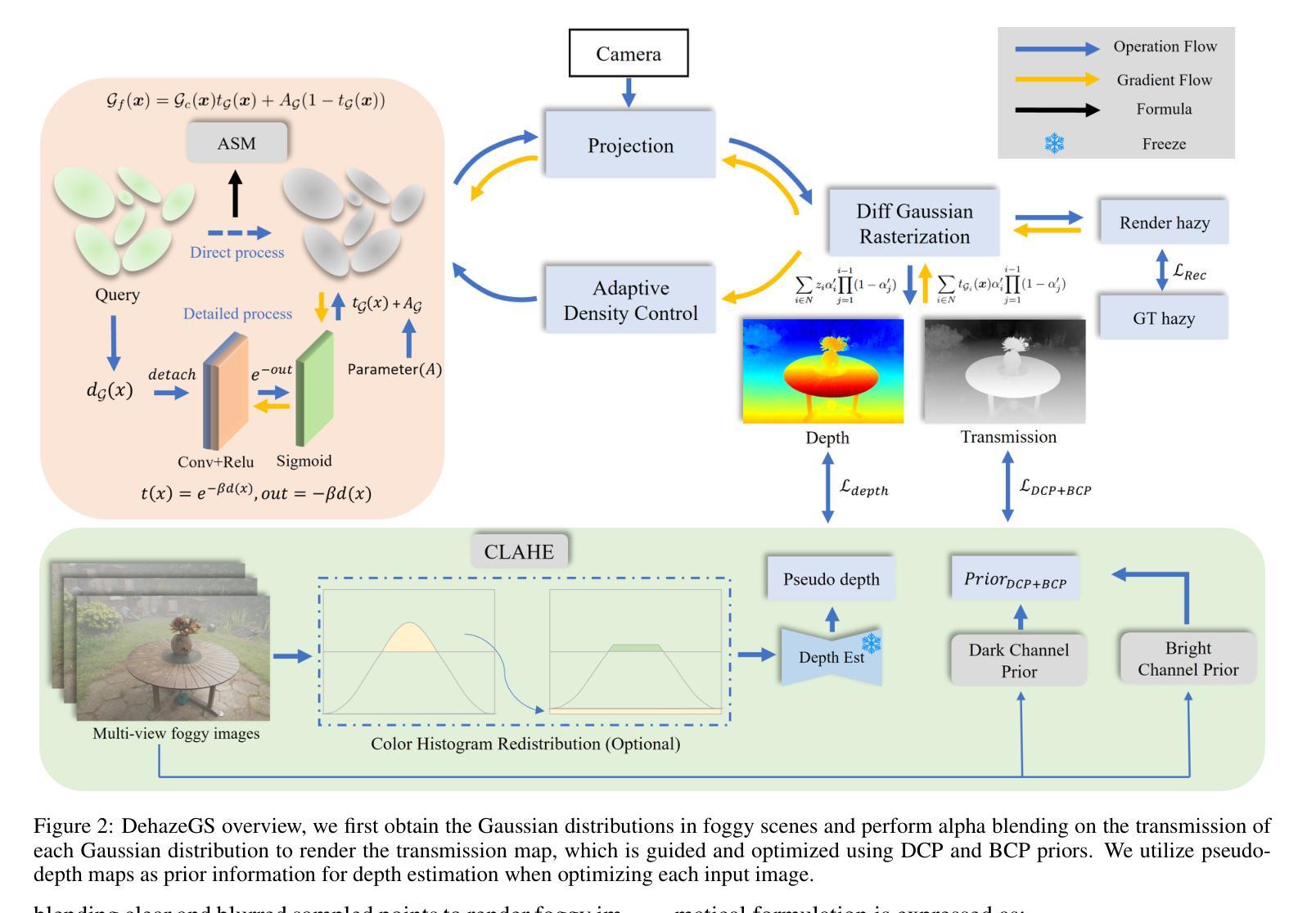
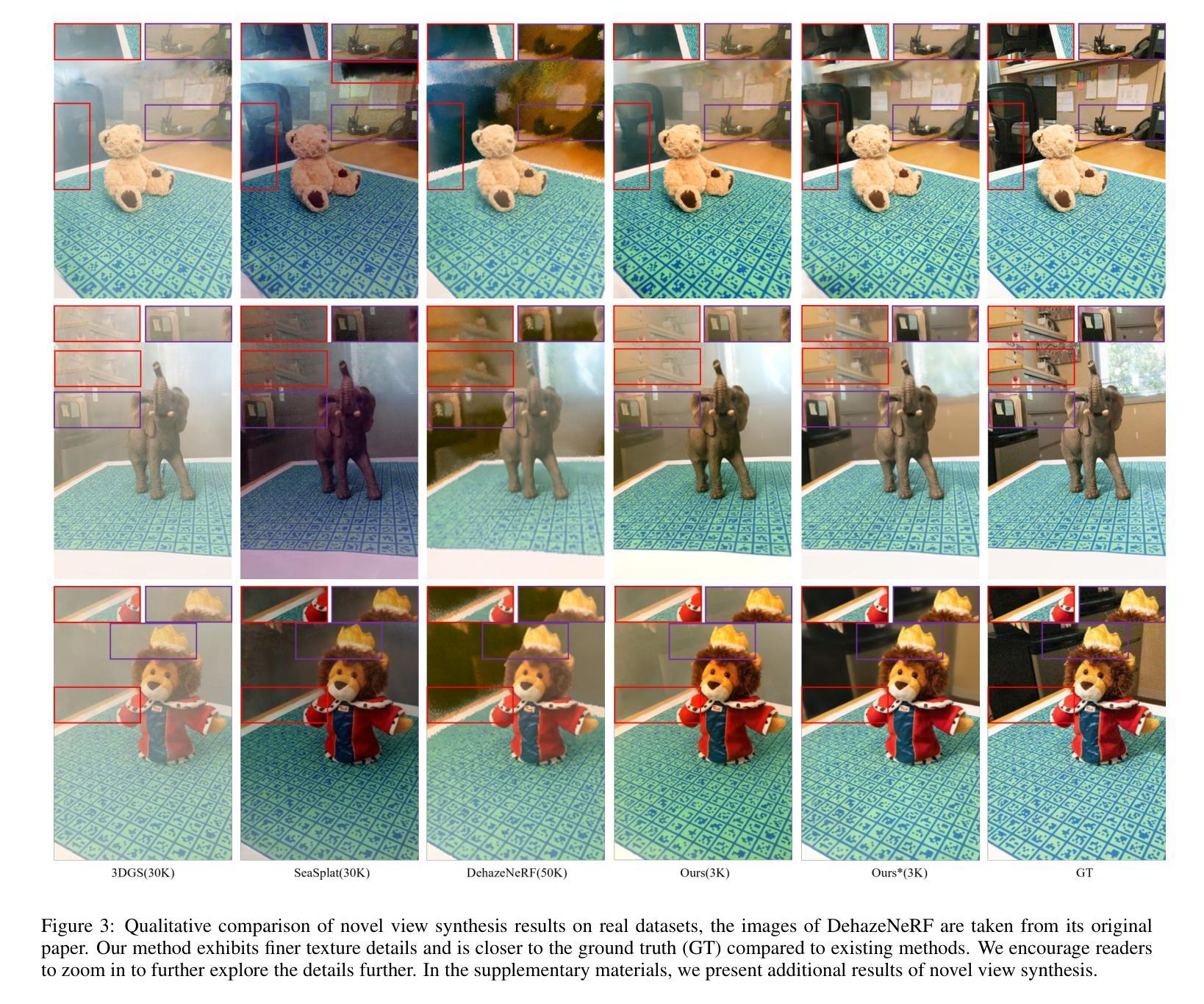
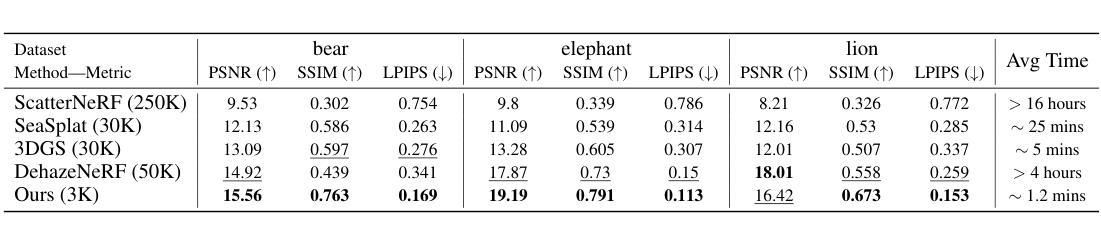
ConcealGS: Concealing Invisible Copyright Information in 3D Gaussian Splatting
Authors:Yifeng Yang, Hengyu Liu, Chenxin Li, Yining Sun, Wuyang Li, Yifan Liu, Yiyang Lin, Yixuan Yuan, Nanyang Ye
With the rapid development of 3D reconstruction technology, the widespread distribution of 3D data has become a future trend. While traditional visual data (such as images and videos) and NeRF-based formats already have mature techniques for copyright protection, steganographic techniques for the emerging 3D Gaussian Splatting (3D-GS) format have yet to be fully explored. To address this, we propose ConcealGS, an innovative method for embedding implicit information into 3D-GS. By introducing the knowledge distillation and gradient optimization strategy based on 3D-GS, ConcealGS overcomes the limitations of NeRF-based models and enhances the robustness of implicit information and the quality of 3D reconstruction. We evaluate ConcealGS in various potential application scenarios, and experimental results have demonstrated that ConcealGS not only successfully recovers implicit information but also has almost no impact on rendering quality, providing a new approach for embedding invisible and recoverable information into 3D models in the future.
随着3D重建技术的快速发展,3D数据的广泛分布已成为未来趋势。虽然传统视觉数据(如图像和视频)和基于NeRF的格式已经拥有成熟的版权保护技术,但针对新兴的三维高斯拼贴(3D-GS)格式的隐写技术尚未被完全探索。为解决这一问题,我们提出ConcealGS,这是一种将隐形信息嵌入到三维高斯拼贴(3D-GS)中的创新方法。通过引入基于三维高斯拼贴的知识蒸馏和梯度优化策略,ConcealGS克服了基于NeRF模型的局限性,提高了隐形信息的稳健性和三维重建的质量。我们在各种潜在的应用场景中评估了ConcealGS,实验结果表明,ConcealGS不仅成功恢复了隐形信息,而且对渲染质量几乎没有影响,为未来在三维模型中嵌入不可见并可恢复的信息提供了新的途径。
论文及项目相关链接
Summary
随着3D重建技术的快速发展,3D数据的广泛分布已成为未来趋势。针对新兴的3D高斯拼贴(3D-GS)格式,我们提出ConcealGS方法,通过引入知识蒸馏和基于3D-GS的梯度优化策略,实现隐性信息的嵌入。评估表明,ConcealGS不仅成功恢复隐性信息,而且对渲染质量几乎无影响,为未来3D模型中的隐形可恢复信息嵌入提供了新的途径。
Key Takeaways
- 3D重建技术的快速发展促进了3D数据的广泛分布,成为未来趋势。
- 传统视觉数据和NeRF格式的版权保护技术已成熟,但3D-GS格式的隐写技术尚未充分探索。
- ConcealGS是一种基于3D-GS的知识蒸馏和梯度优化策略,能够实现隐性信息嵌入。
- ConcealGS克服了NeRF模型的局限性,提高了隐性信息的稳健性和3D重建的质量。
- 实验结果表明,ConcealGS能成功恢复隐性信息,且对渲染质量影响极小。
点此查看论文截图
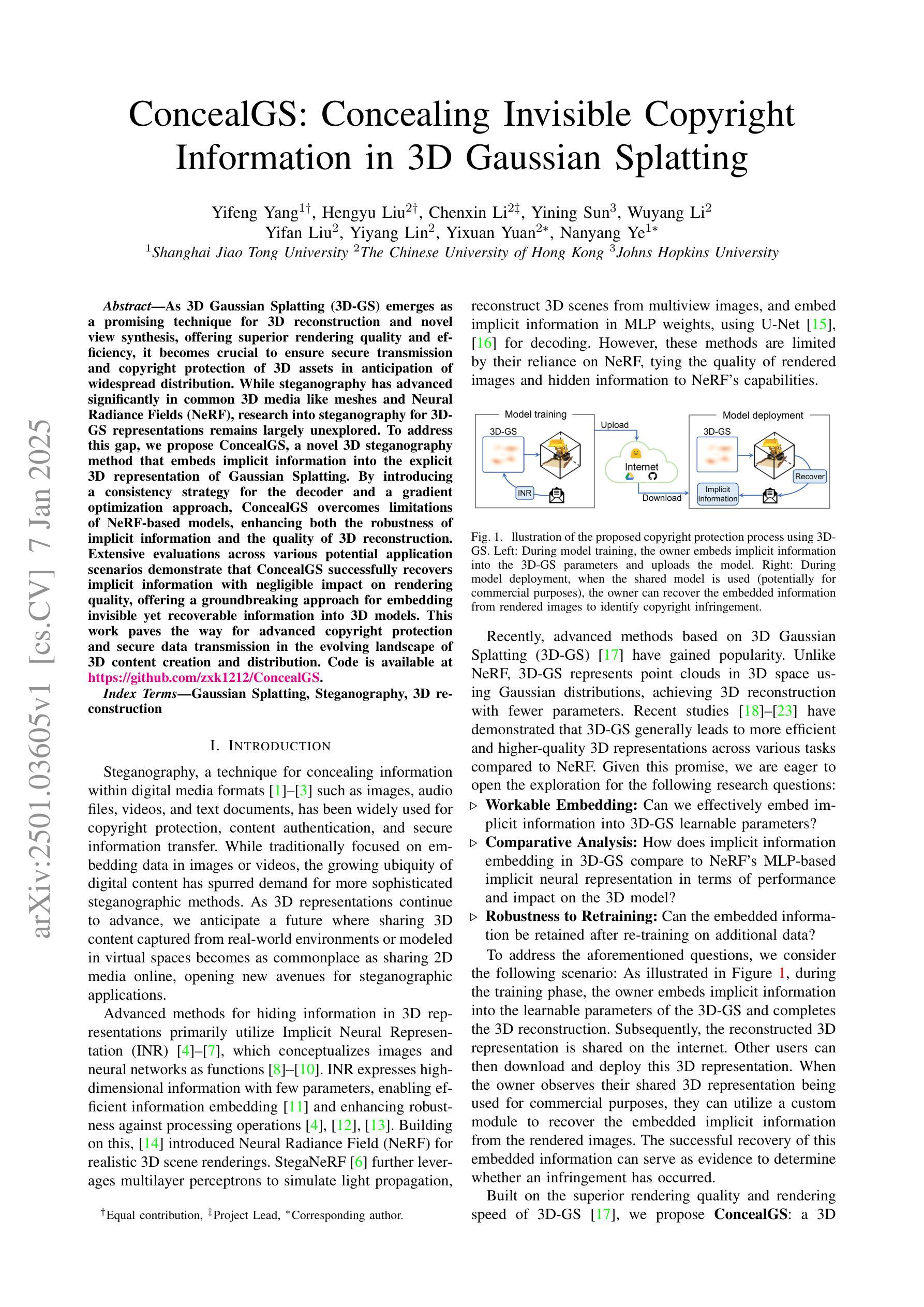
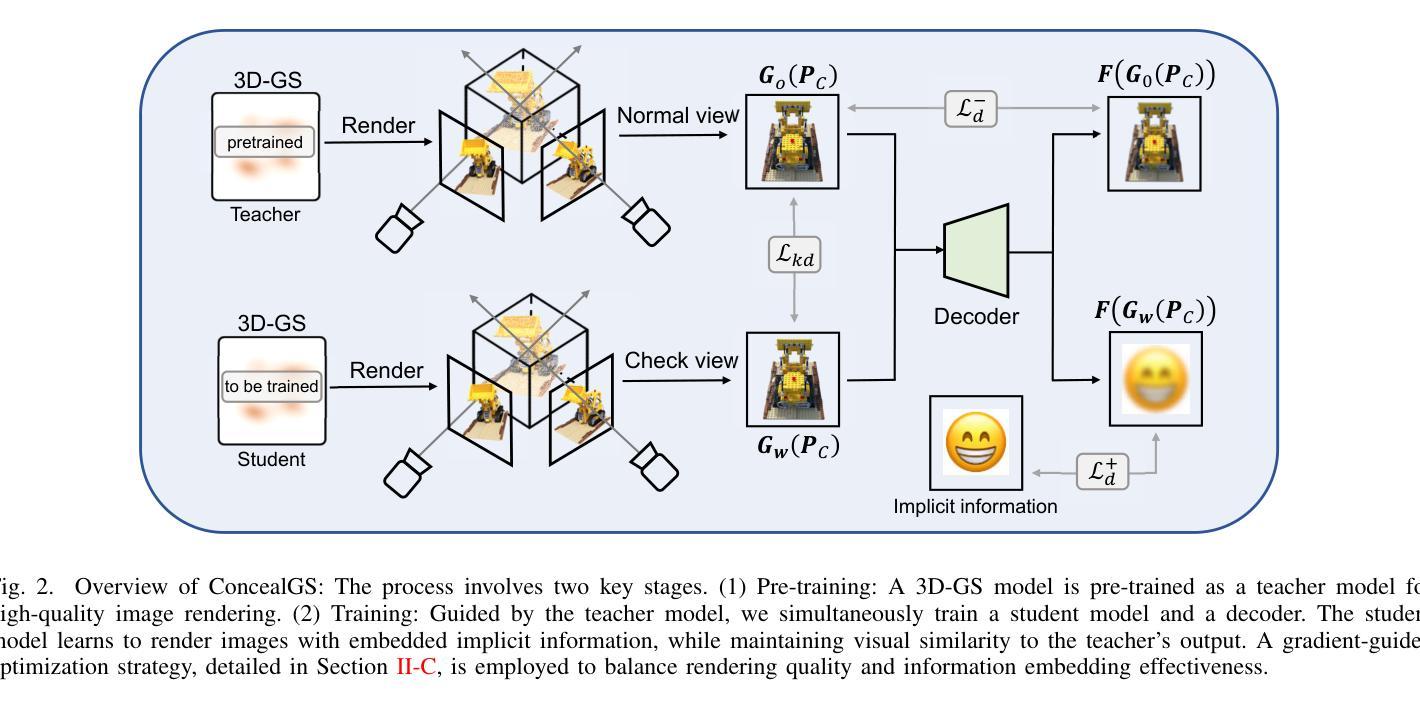
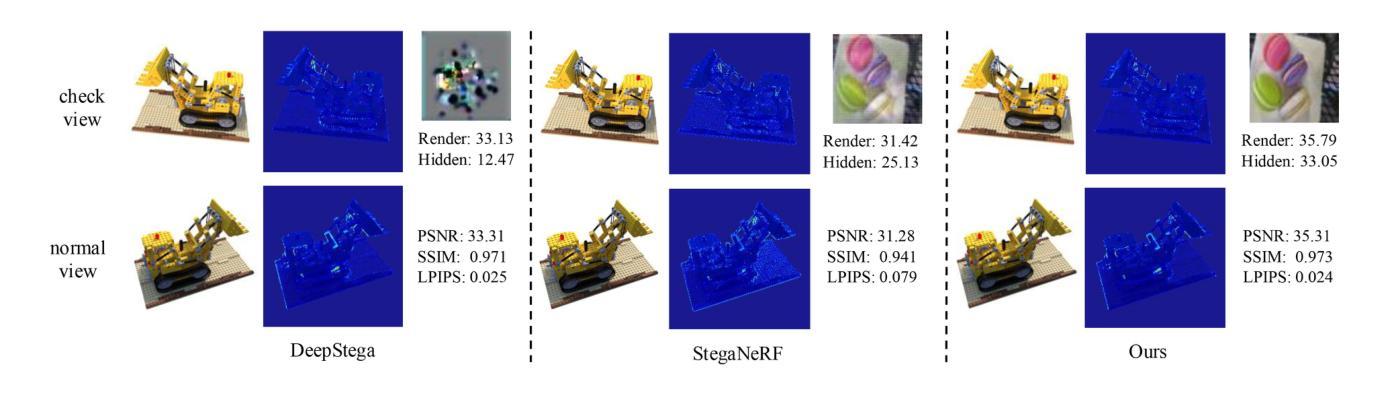
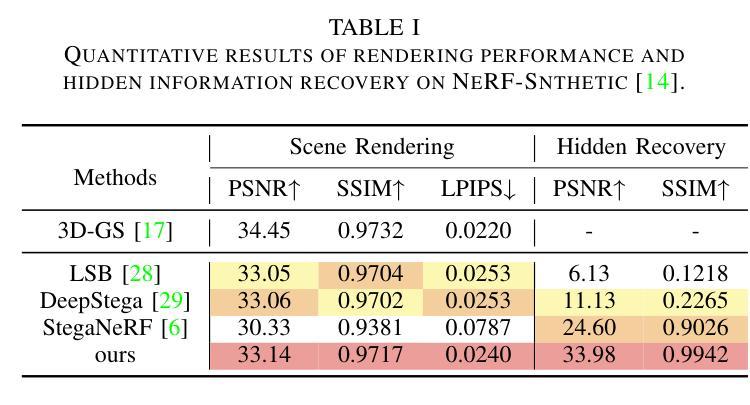
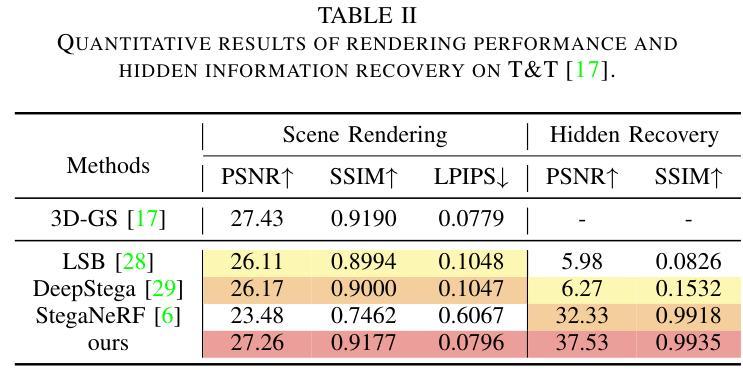
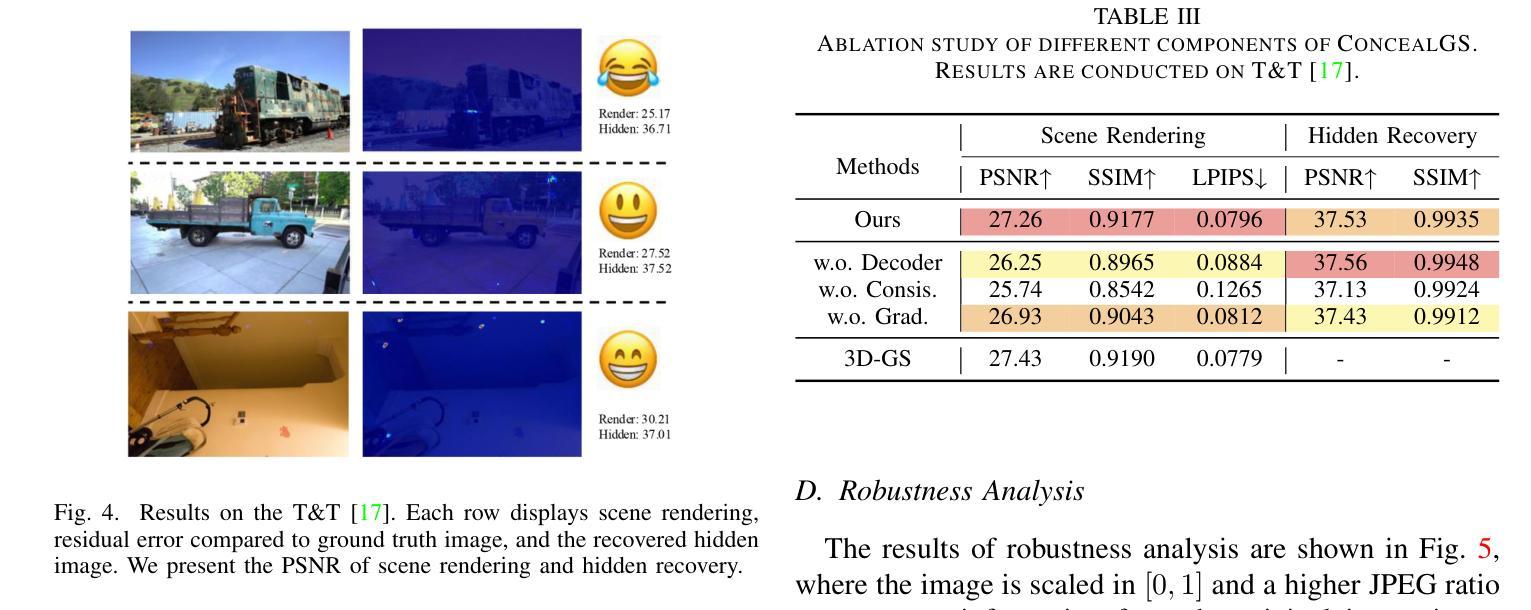
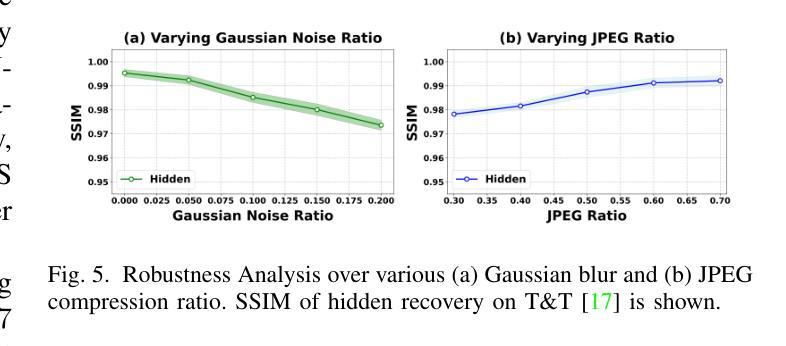
Compression of 3D Gaussian Splatting with Optimized Feature Planes and Standard Video Codecs
Authors:Soonbin Lee, Fangwen Shu, Yago Sanchez, Thomas Schierl, Cornelius Hellge
3D Gaussian Splatting is a recognized method for 3D scene representation, known for its high rendering quality and speed. However, its substantial data requirements present challenges for practical applications. In this paper, we introduce an efficient compression technique that significantly reduces storage overhead by using compact representation. We propose a unified architecture that combines point cloud data and feature planes through a progressive tri-plane structure. Our method utilizes 2D feature planes, enabling continuous spatial representation. To further optimize these representations, we incorporate entropy modeling in the frequency domain, specifically designed for standard video codecs. We also propose channel-wise bit allocation to achieve a better trade-off between bitrate consumption and feature plane representation. Consequently, our model effectively leverages spatial correlations within the feature planes to enhance rate-distortion performance using standard, non-differentiable video codecs. Experimental results demonstrate that our method outperforms existing methods in data compactness while maintaining high rendering quality. Our project page is available at https://fraunhoferhhi.github.io/CodecGS
3D高斯展开是一种公认的3D场景表示方法,以其高质量和高速渲染而闻名。然而,其巨大的数据需求对实际应用提出了挑战。在本文中,我们介绍了一种高效的压缩技术,该技术通过使用紧凑表示来显著减少存储开销。我们提出了一种统一架构,通过渐进的三平面结构将点云数据和特征平面结合起来。我们的方法利用二维特征平面,实现连续的空间表示。为了进一步优化这些表示,我们在频域中引入熵模型,专门用于标准视频编码。我们还提出通道位分配,以实现比特率消耗与特征平面表示之间的更好权衡。因此,我们的模型有效利用特征平面内的空间相关性,利用标准不可微视频编码提高速率失真性能。实验结果表明,我们的方法在数据紧凑性方面优于现有方法,同时保持高质量渲染。我们的项目页面可通过https://fraunhoferhhi.github.io/CodecGS访问。
论文及项目相关链接
Summary
本文介绍了一种基于3D高斯 Splatting的高效压缩技术,该技术使用紧凑表示来显著降低存储开销。文章提出了一种结合点云数据和特征平面的统一架构,通过渐进的三平面结构实现。该方法利用二维特征平面实现连续空间表示,并在频率域引入熵建模,针对标准视频编码进行优化。此外,还提出了通道位分配策略,以在比特率消耗与特征平面表示之间取得更好的平衡。实验结果表明,该方法在保持高渲染质量的同时,数据紧凑性优于现有方法。
Key Takeaways
- 3D Gaussian Splatting是高效的三维场景表示方法,具有高渲染质量和速度。
- 引入紧凑表示法以降低存储开销。
- 提出结合点云数据和特征平面的统一架构。
- 利用二维特征平面实现连续空间表示。
- 在频率域引入熵建模,针对标准视频编码进行优化。
- 提出通道位分配策略,以优化比特率消耗和特征平面表示之间的平衡。
点此查看论文截图
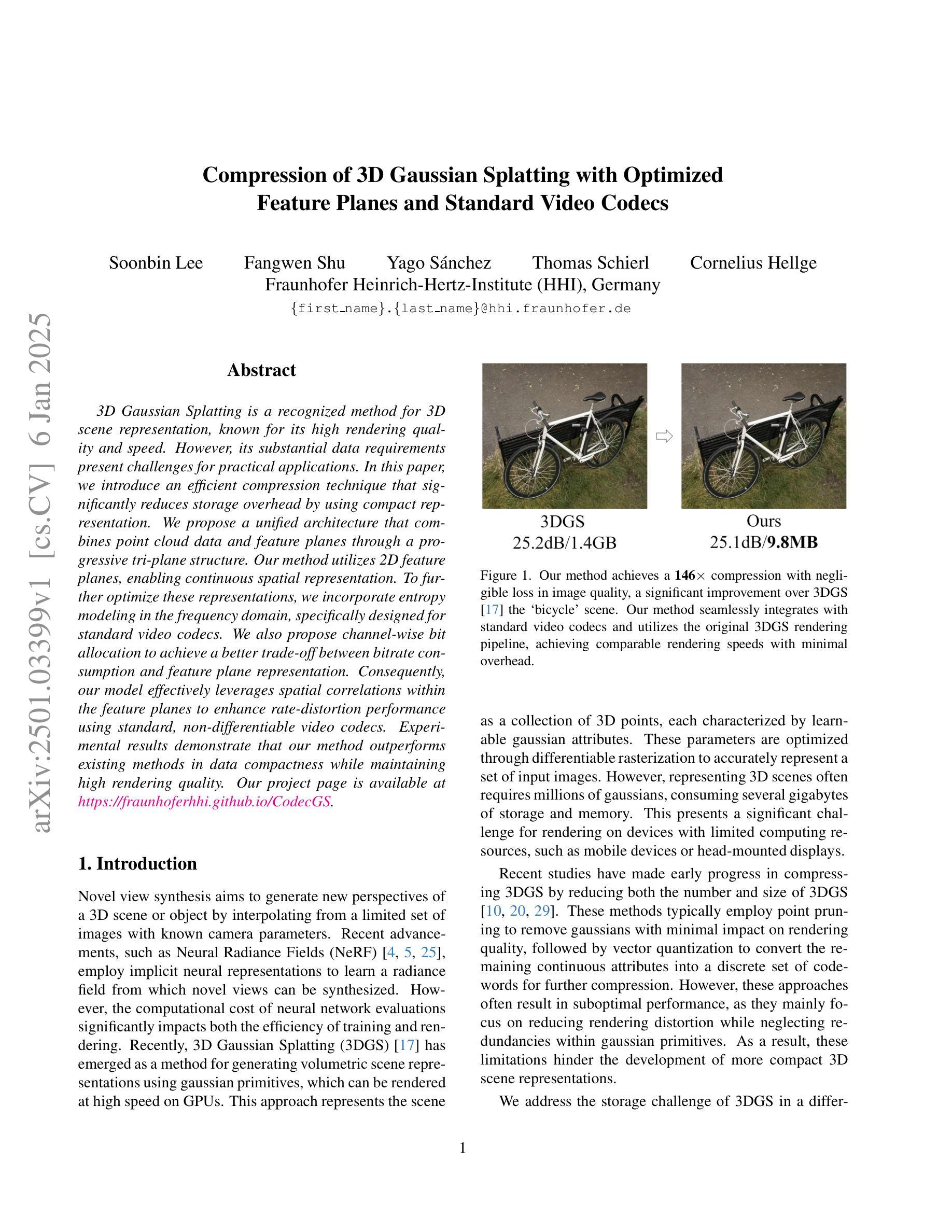
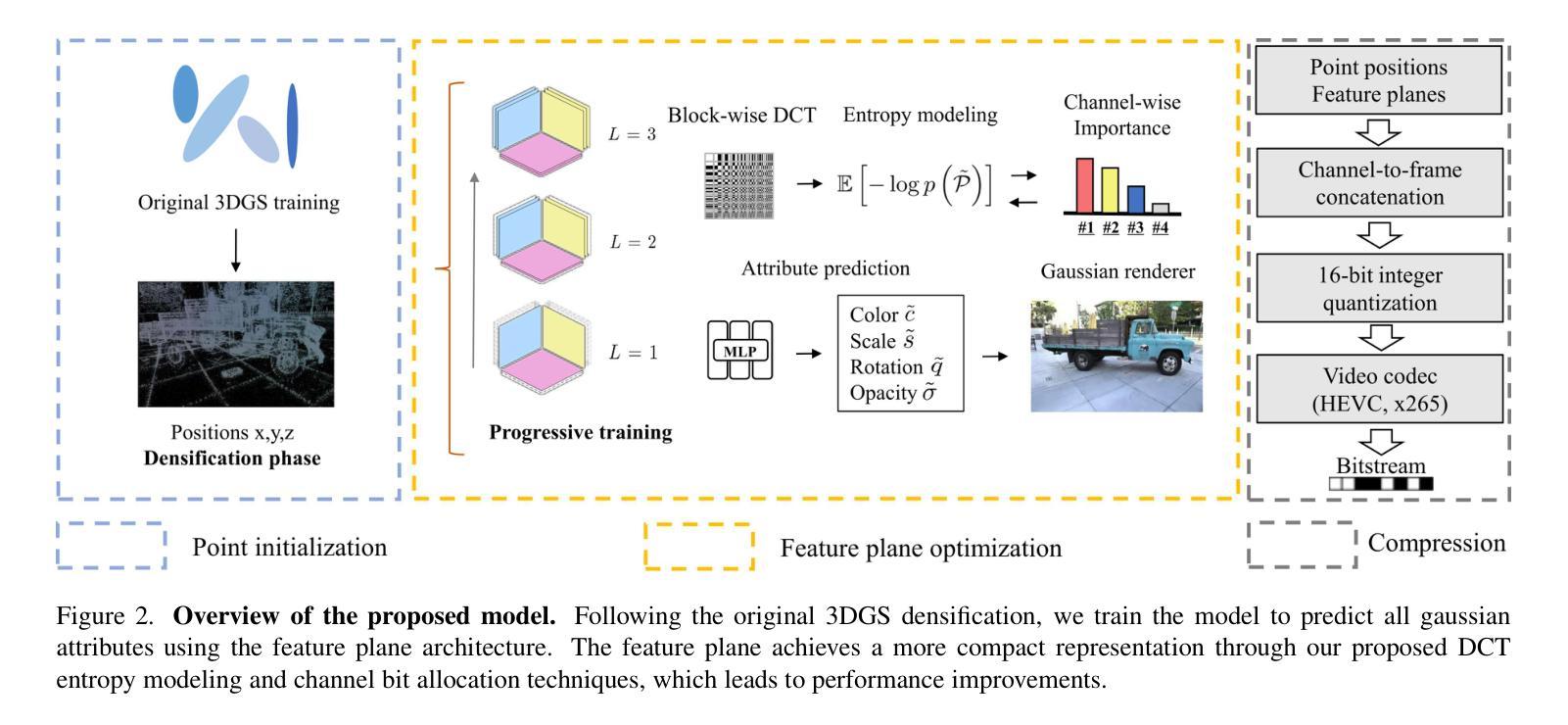
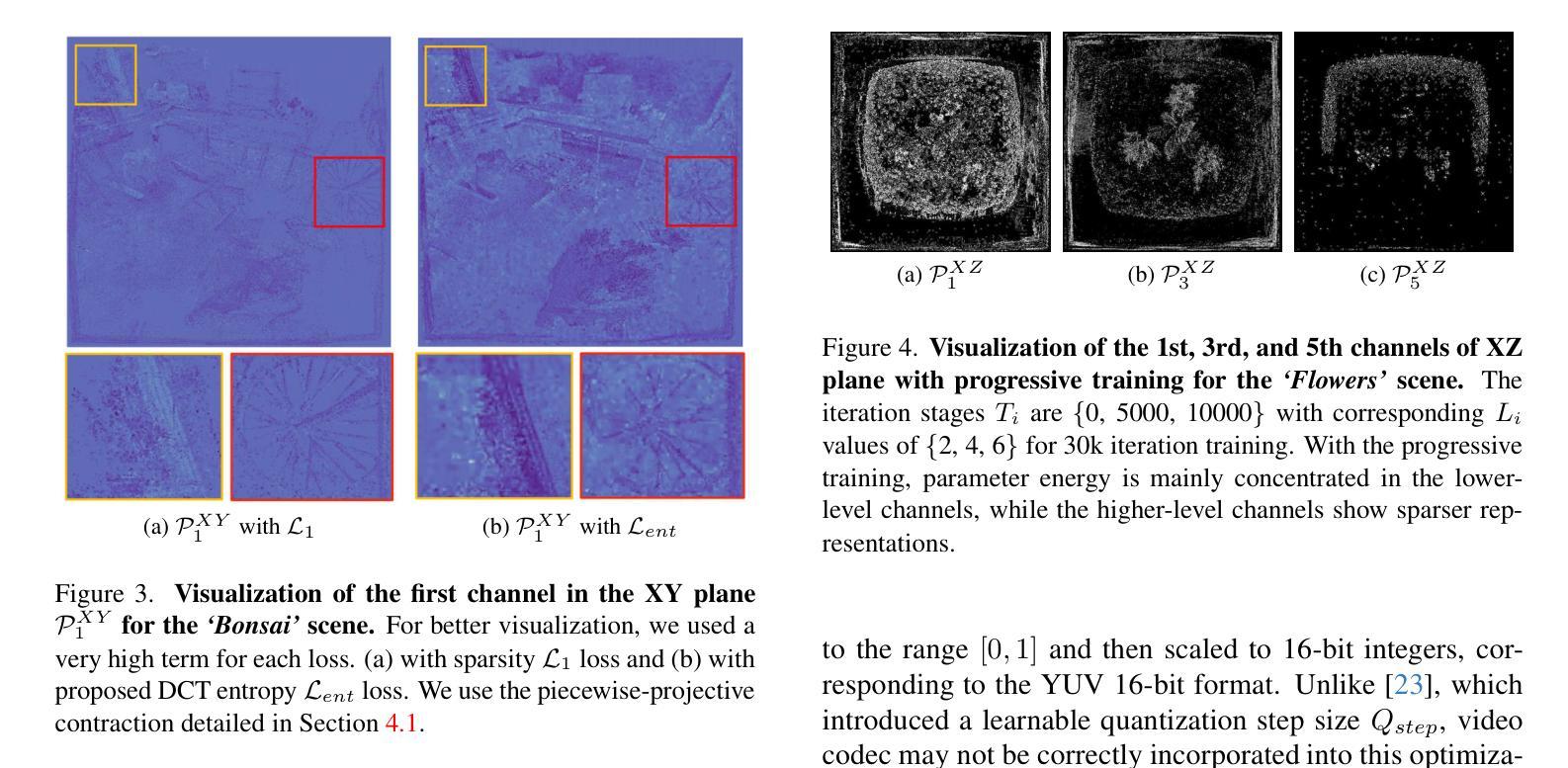
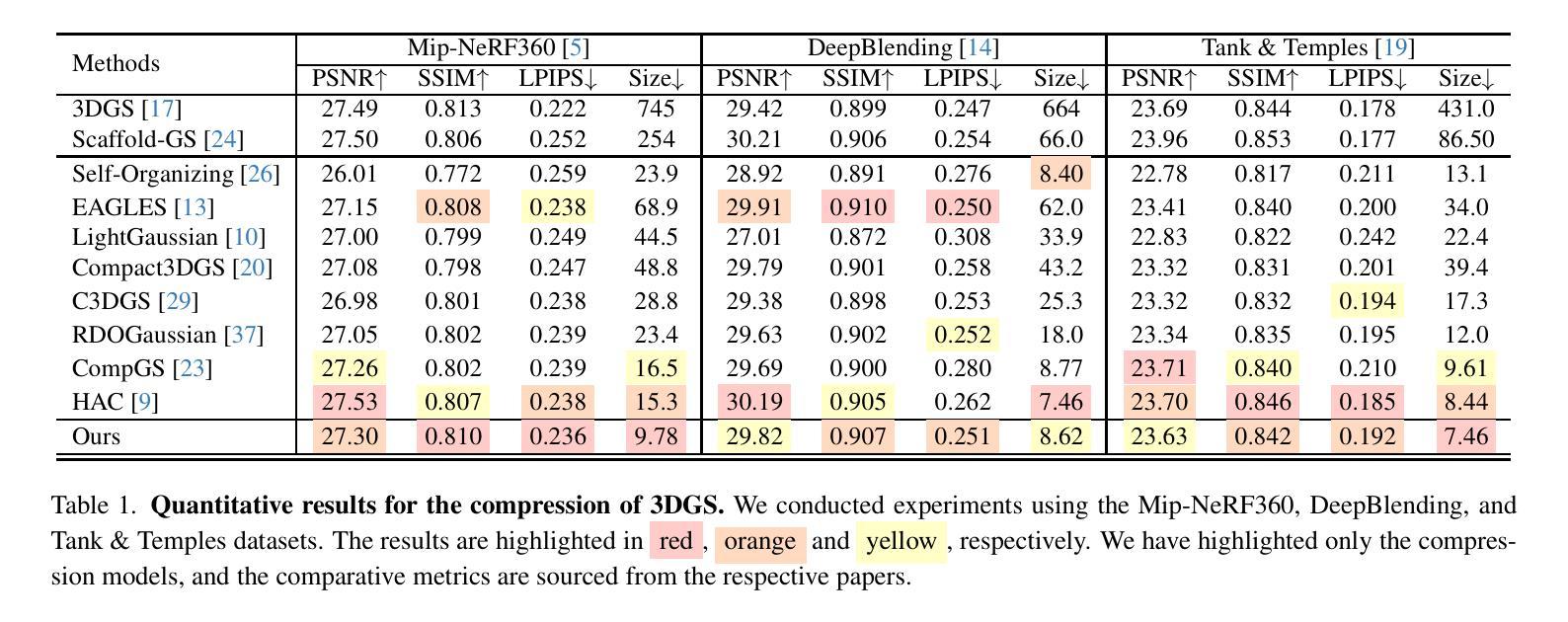
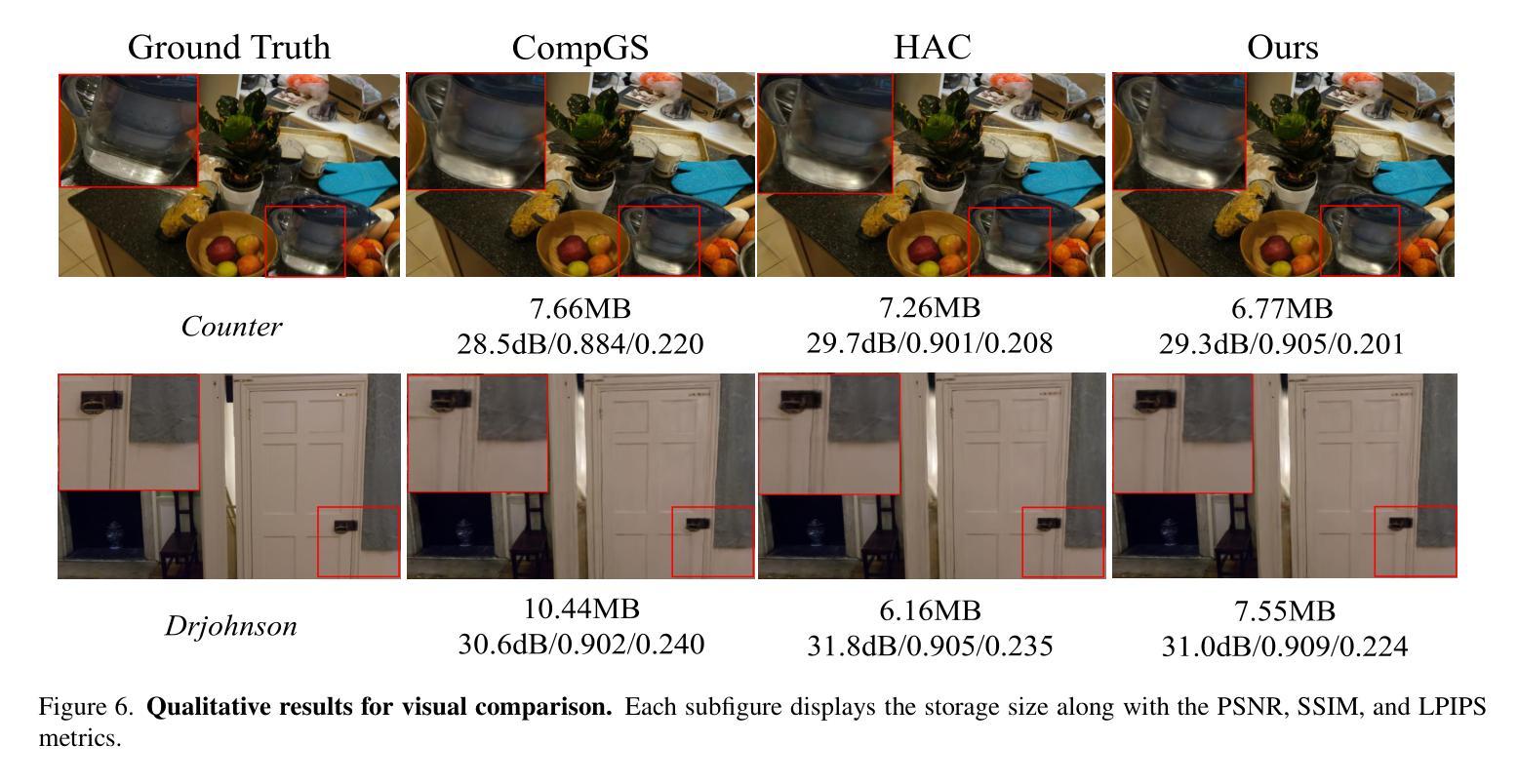
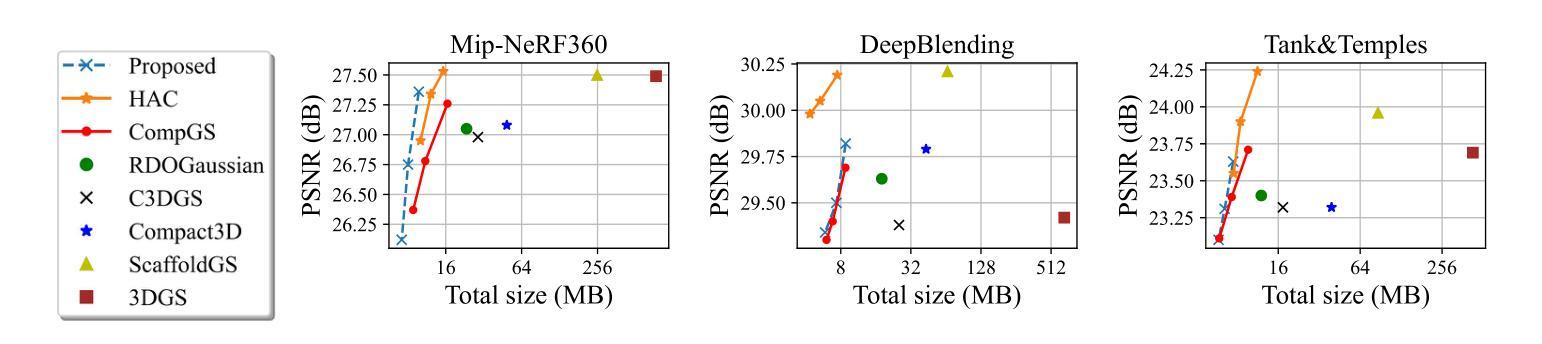