⚠️ 以下所有内容总结都来自于 大语言模型的能力,如有错误,仅供参考,谨慎使用
🔴 请注意:千万不要用于严肃的学术场景,只能用于论文阅读前的初筛!
💗 如果您觉得我们的项目对您有帮助 ChatPaperFree ,还请您给我们一些鼓励!⭐️ HuggingFace免费体验
2025-01-09 更新
A Soft Sensor Method with Uncertainty-Awareness and Self-Explanation Based on Large Language Models Enhanced by Domain Knowledge Retrieval
Authors:Shuo Tong, Runyuan Guo, Wenqing Wang, Xueqiong Tian, Lingyun Wei, Lin Zhang, Huayong Wu, Ding Liu, Youmin Zhang
Data-driven soft sensors are crucial in predicting key performance indicators in industrial systems. However, current methods predominantly rely on the supervised learning paradigms of parameter updating, which inherently faces challenges such as high development costs, poor robustness, training instability, and lack of interpretability. Recently, large language models (LLMs) have demonstrated significant potential across various domains, notably through In-Context Learning (ICL), which enables high-performance task execution with minimal input-label demonstrations and no prior training. This paper aims to replace supervised learning with the emerging ICL paradigm for soft sensor modeling to address existing challenges and explore new avenues for advancement. To achieve this, we propose a novel framework called the Few-shot Uncertainty-aware and self-Explaining Soft Sensor (LLM-FUESS), which includes the Zero-shot Auxiliary Variable Selector (LLM-ZAVS) and the Uncertainty-aware Few-shot Soft Sensor (LLM-UFSS). The LLM-ZAVS retrieves from the Industrial Knowledge Vector Storage to enhance LLMs’ domain-specific knowledge, enabling zero-shot auxiliary variable selection. In the LLM-UFSS, we utilize text-based context demonstrations of structured data to prompt LLMs to execute ICL for predicting and propose a context sample retrieval augmentation strategy to improve performance. Additionally, we explored LLMs’ AIGC and probabilistic characteristics to propose self-explanation and uncertainty quantification methods for constructing a trustworthy soft sensor. Extensive experiments demonstrate that our method achieved state-of-the-art predictive performance, strong robustness, and flexibility, effectively mitigates training instability found in traditional methods. To the best of our knowledge, this is the first work to establish soft sensor utilizing LLMs.
数据驱动型软传感器对于预测工业系统的关键性能指标至关重要。然而,当前的方法主要依赖于参数更新的监督学习模式,这固有地面临着高开发成本、鲁棒性差、训练不稳定和缺乏可解释性等挑战。最近,大型语言模型(LLM)在各个领域表现出了巨大的潜力,尤其是通过上下文学习(ICL),它能够在极少输入标签演示的情况下且无预先训练的情况下实现高性能的任务执行。
论文及项目相关链接
Summary
数据驱动型软传感器对预测工业系统关键性能指标至关重要。然而,现有方法主要依赖监督学习模式的参数更新,面临高开发成本、鲁棒性差、训练不稳定和缺乏可解释性等问题。近期,大型语言模型(LLMs)展现出显著潜力,通过上下文学习(ICL)在多个领域实现高性能任务执行。本文旨在将新兴的ICL范式应用于软传感器建模,解决现有挑战并探索新的发展途径。为此,我们提出了一种名为Few-shot Uncertainty-aware and self-Explaining Soft Sensor(LLM-FUESS)的新框架,包括Zero-shot Auxiliary Variable Selector(LLM-ZAVS)和Uncertainty-aware Few-shot Soft Sensor(LLM-UFSS)。LLM-ZAVS从工业知识向量存储中检索,增强LLMs的领域特定知识,实现零射击辅助变量选择。在LLM-UFSS中,我们利用结构化数据的文本上下文演示来提示LLMs执行ICL进行预测,并提出一种上下文样本检索增强策略来提高性能。此外,我们探索了LLMs的人工智能生成内容和概率特性,提出自我解释和不确定性量化方法,构建可信的软传感器。大量实验表明,我们的方法达到了先进的预测性能、强大的鲁棒性和灵活性,有效缓解了传统方法中的训练不稳定问题。
Key Takeaways
- 数据驱动型软传感器是预测工业系统关键性能指标的关键。
- 当前方法主要依赖监督学习,存在高成本、缺乏鲁棒性和可解释性问题。
- 大型语言模型(LLMs)具有显著潜力,通过上下文学习(ICL)实现高性能任务执行。
- 本文提出一种新型软传感器框架LLM-FUESS,结合LLM-ZAVS和LLM-UFSS解决现有问题。
- LLM-ZAVS利用工业知识向量存储增强LLMs的领域知识,实现零射击辅助变量选择。
- LLM-UFSS利用文本上下文演示和上下文样本检索增强策略提高预测性能。
点此查看论文截图
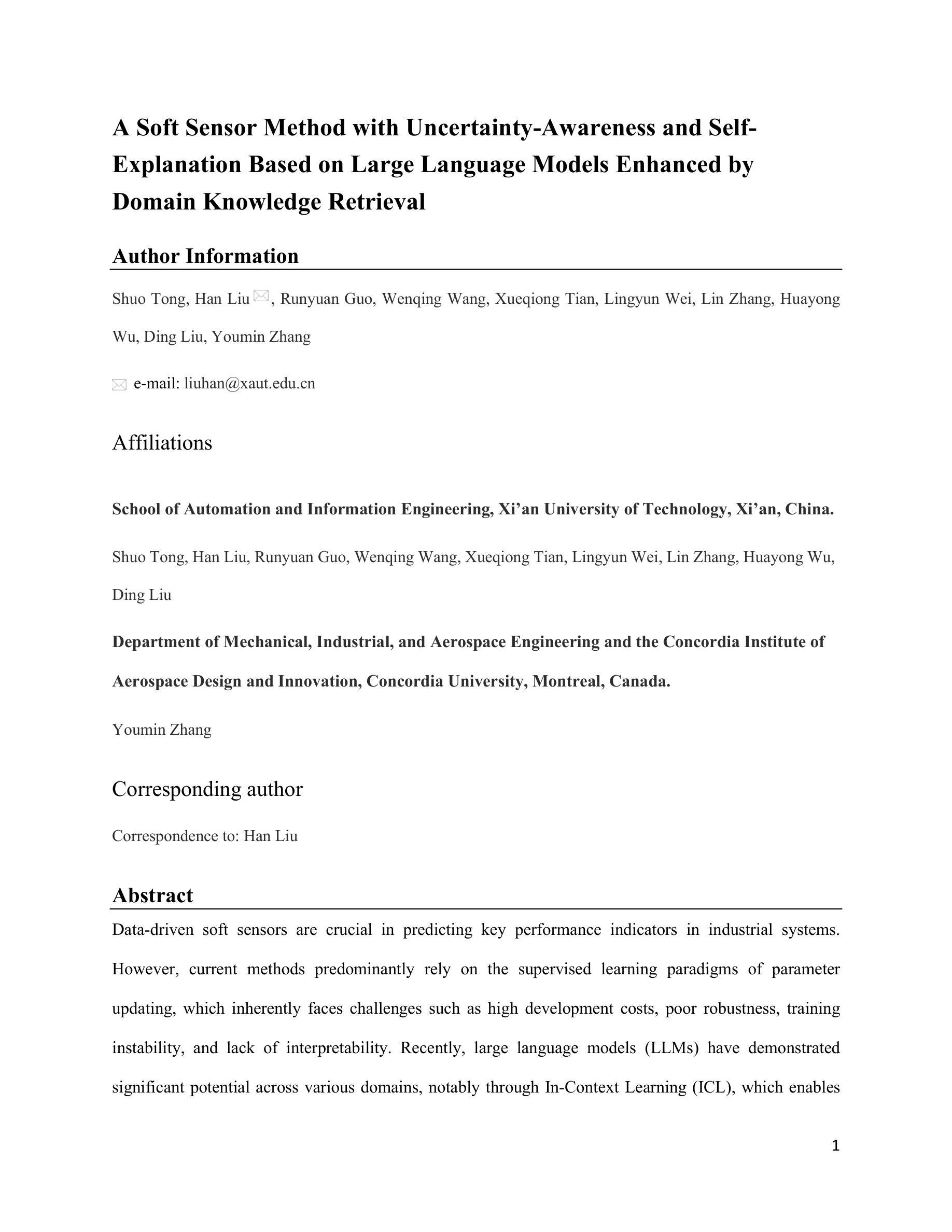
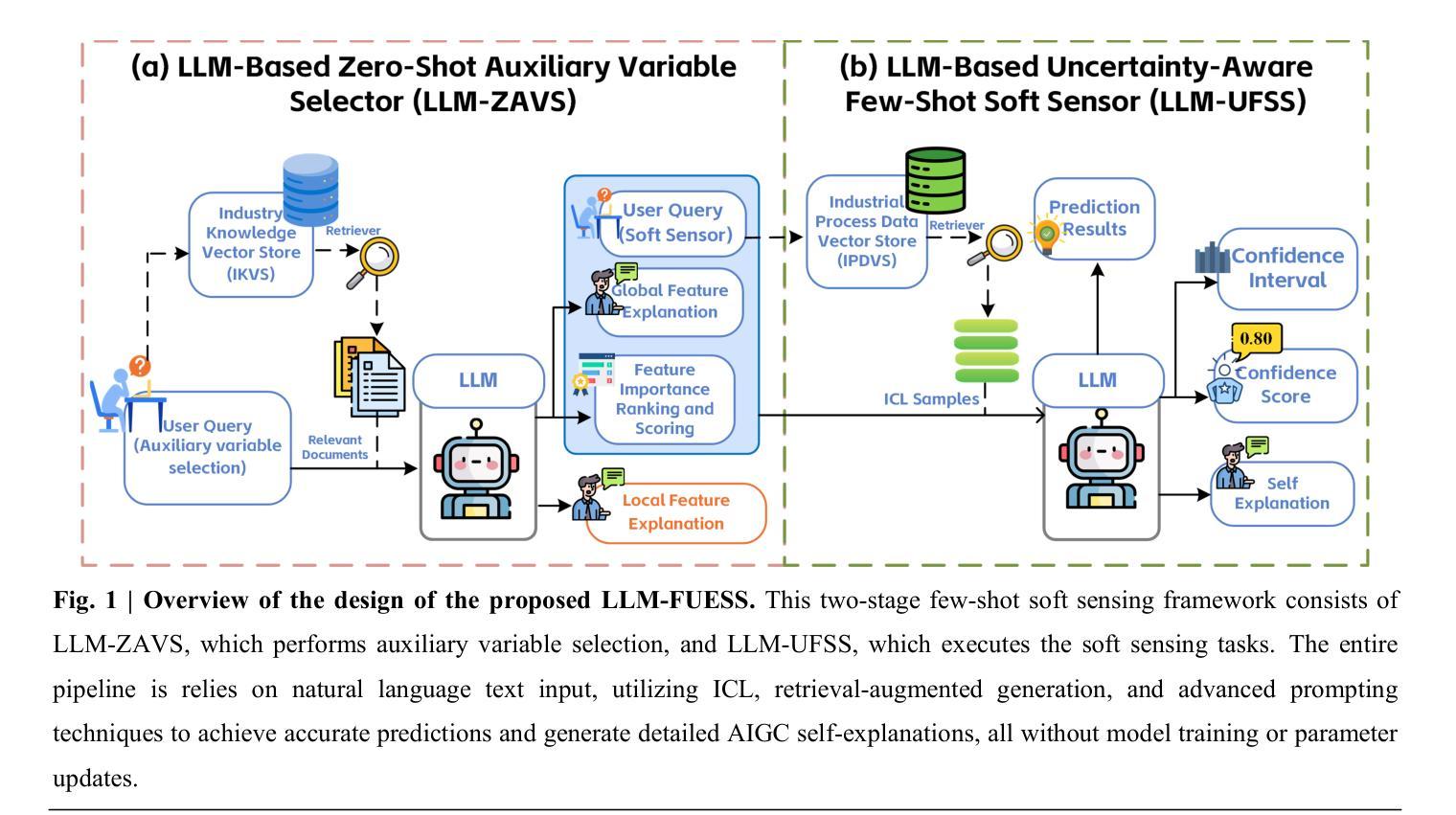
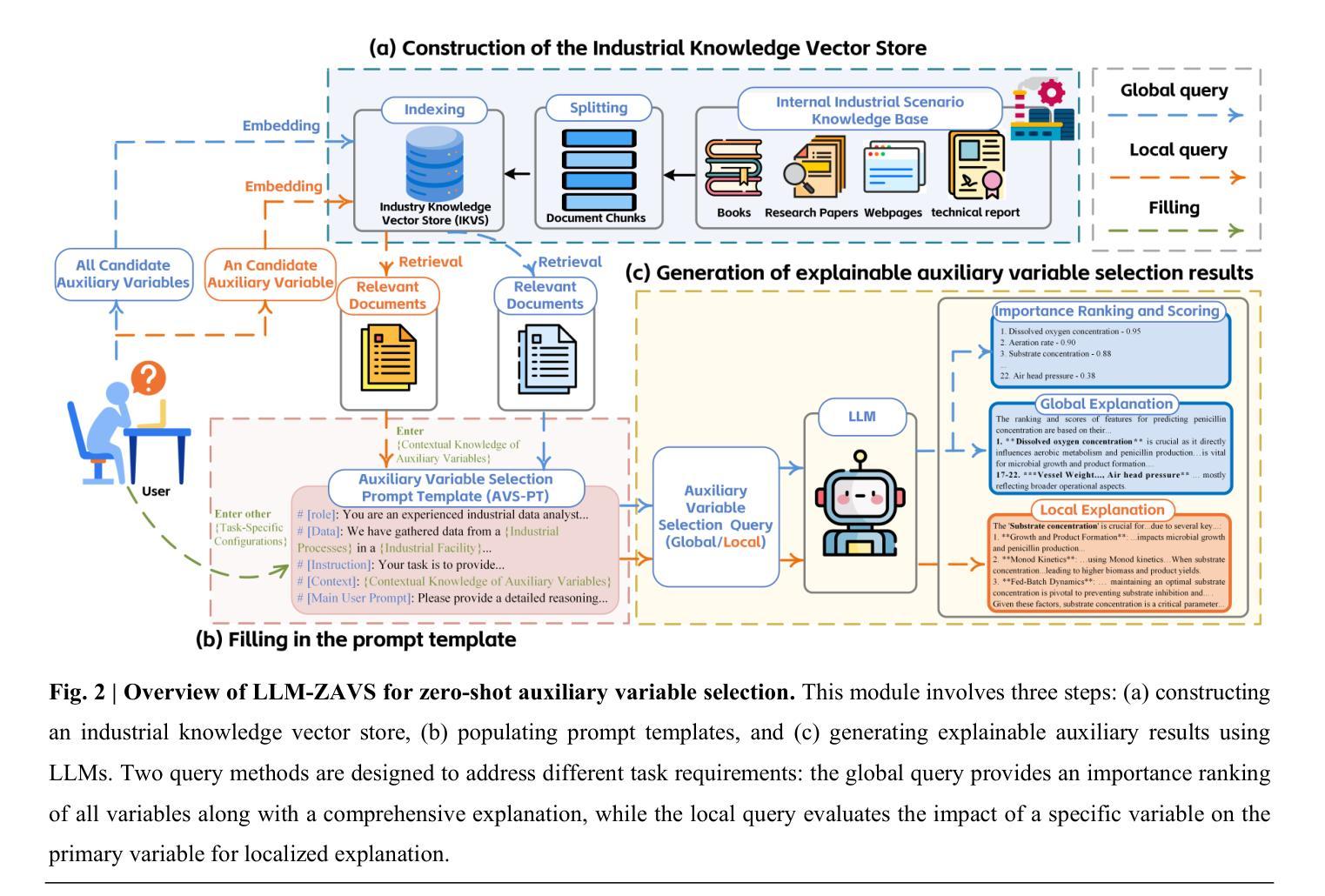
AllSpark: A Multimodal Spatio-Temporal General Intelligence Model with Ten Modalities via Language as a Reference Framework
Authors:Run Shao, Cheng Yang, Qiujun Li, Qing Zhu, Yongjun Zhang, YanSheng Li, Yu Liu, Yong Tang, Dapeng Liu, Shizhong Yang, Haifeng Li
Leveraging multimodal data is an inherent requirement for comprehending geographic objects. However, due to the high heterogeneity in structure and semantics among various spatio-temporal modalities, the joint interpretation of multimodal spatio-temporal data has long been an extremely challenging problem. The primary challenge resides in striking a trade-off between the cohesion and autonomy of diverse modalities. This trade-off becomes progressively nonlinear as the number of modalities expands. Inspired by the human cognitive system and linguistic philosophy, where perceptual signals from the five senses converge into language, we introduce the Language as Reference Framework (LaRF), a fundamental principle for constructing a multimodal unified model. Building upon this, we propose AllSpark, a multimodal spatio-temporal general artificial intelligence model. Our model integrates ten different modalities into a unified framework. To achieve modal cohesion, AllSpark introduces a modal bridge and multimodal large language model (LLM) to map diverse modal features into the language feature space. To maintain modality autonomy, AllSpark uses modality-specific encoders to extract the tokens of various spatio-temporal modalities. Finally, observing a gap between the model’s interpretability and downstream tasks, we designed modality-specific prompts and task heads, enhancing the model’s generalization capability across specific tasks. Experiments indicate that the incorporation of language enables AllSpark to excel in few-shot classification tasks for RGB and point cloud modalities without additional training, surpassing baseline performance by up to 41.82%. The source code is available at https://github.com/GeoX-Lab/AllSpark.
利用多模态数据是理解地理对象的基本要求。然而,由于各种时空模态在结构和语义上的高度异质性,多模态时空数据的联合解释一直是一个极具挑战性的问题。主要挑战在于如何在不同模态的连贯性和自主性之间取得平衡。随着模态数量的增加,这种平衡逐渐变得非线性。受人类认知系统和语言哲学的启发,即五种感官的感知信号汇入语言,我们引入了语言作为参考框架(LaRF),这是构建多模态统一模型的基本原则。在此基础上,我们提出了多模态时空通用人工智能模型AllSpark。我们的模型将十种不同的模态集成到一个统一框架中。为了实现模态连贯性,AllSpark引入了一个模态桥和多模态大型语言模型(LLM),将各种模态特征映射到语言特征空间中。为了保持模态自主性,AllSpark使用特定模态编码器提取各种时空模态的标记。最后,我们观察到模型的可解释性与下游任务之间存在差距,因此我们设计了特定于模态的提示和任务头,以提高模型在特定任务上的泛化能力。实验表明,语言的结合使AllSpark在RGB和点云模态的少量样本分类任务中表现出色,无需额外训练即可超越基线性能高达41.82%。源代码可在https://github.com/GeoX-Lab/AllSpark获取。
论文及项目相关链接
PDF 19 pages, 19 tables, 3 figures
Summary
基于多模态数据理解地理对象的一个内在要求是进行多模态时空数据的联合解读。由于不同时空模态在结构和语义上的高度异质性,这一任务一直是极具挑战的问题。主要挑战在于如何在不同模态的连贯性和自主性之间取得平衡。随着模态数量的增加,这种平衡变得愈加复杂非线性。受人类认知系统和语言哲学的启发,我们提出了语言参考框架(LaRF)这一构建多模态统一模型的基本原则。在此基础上,我们提出了多模态时空通用人工智能模型AllSpark,它整合了十种不同模态到一个统一框架内。为实现模态连贯性,AllSpark引入了模态桥梁和多模态大型语言模型(LLM),将各种模态特征映射到语言特征空间。同时,为了保持模态自主性,AllSpark使用特定模态编码器提取各种时空模态的标记。最后,我们设计了特定模态的提示和任务头,以解决模型可解释性与下游任务之间存在的差距,增强了模型在特定任务上的泛化能力。实验表明,融入语言使AllSpark在RGB和点云模态的少量样本分类任务中表现卓越,无需额外训练即可超越基线性能高达41.82%。
Key Takeaways
- 多模态数据的联合解读是理解地理对象的内在要求。
- 不同时空模态在结构和语义上的异质性导致联合解读极具挑战。
- 在多模态模型的构建中,需要在模态连贯性和自主性之间取得平衡。
- 受人类认知系统和语言哲学的启发,提出了语言参考框架(LaRF)作为构建多模态统一模型的基本原则。
- AllSpark是一个多模态时空通用人工智能模型,整合了十种不同模态。
- AllSpark通过引入模态桥梁和多模态大型语言模型实现模态连贯性。
点此查看论文截图
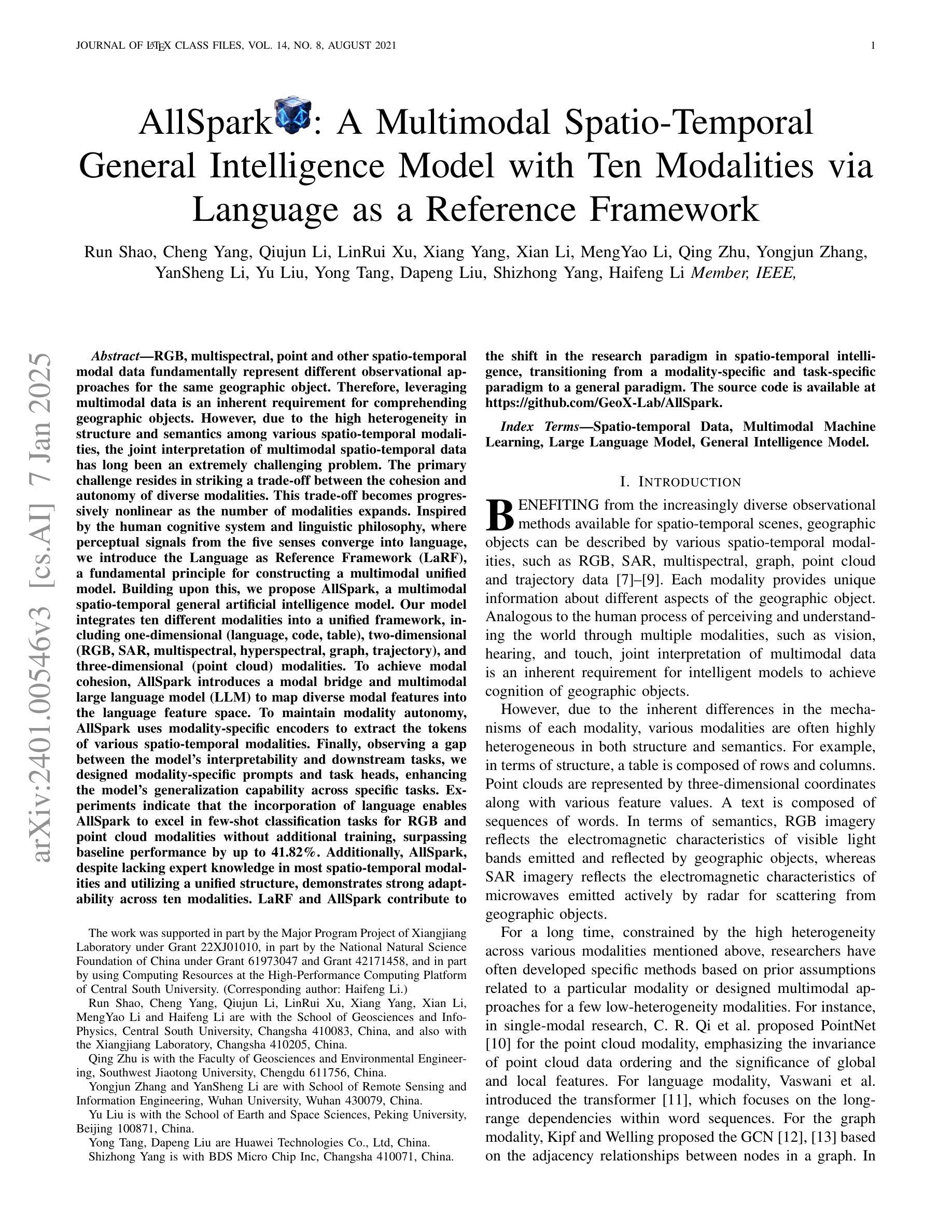
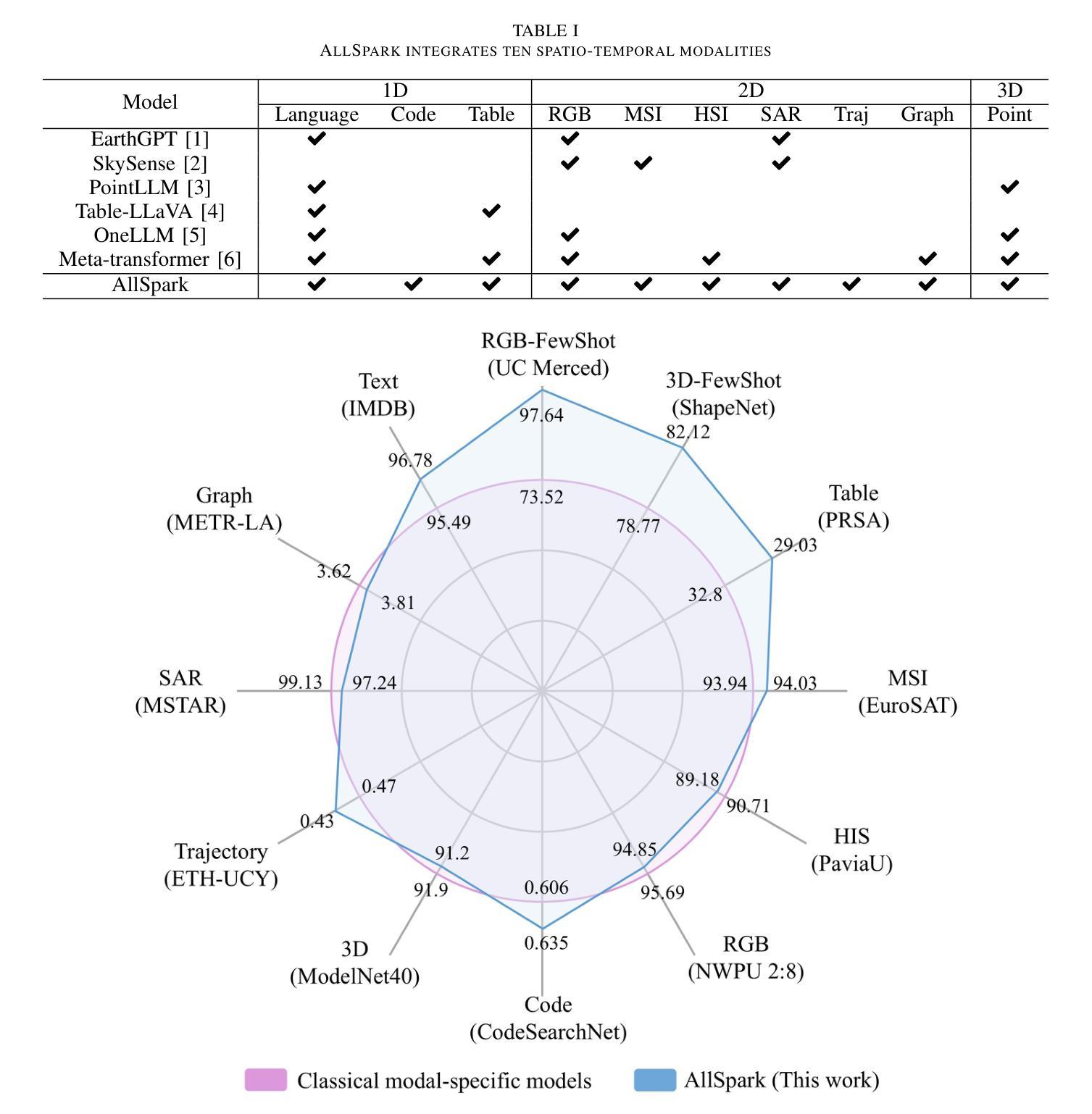
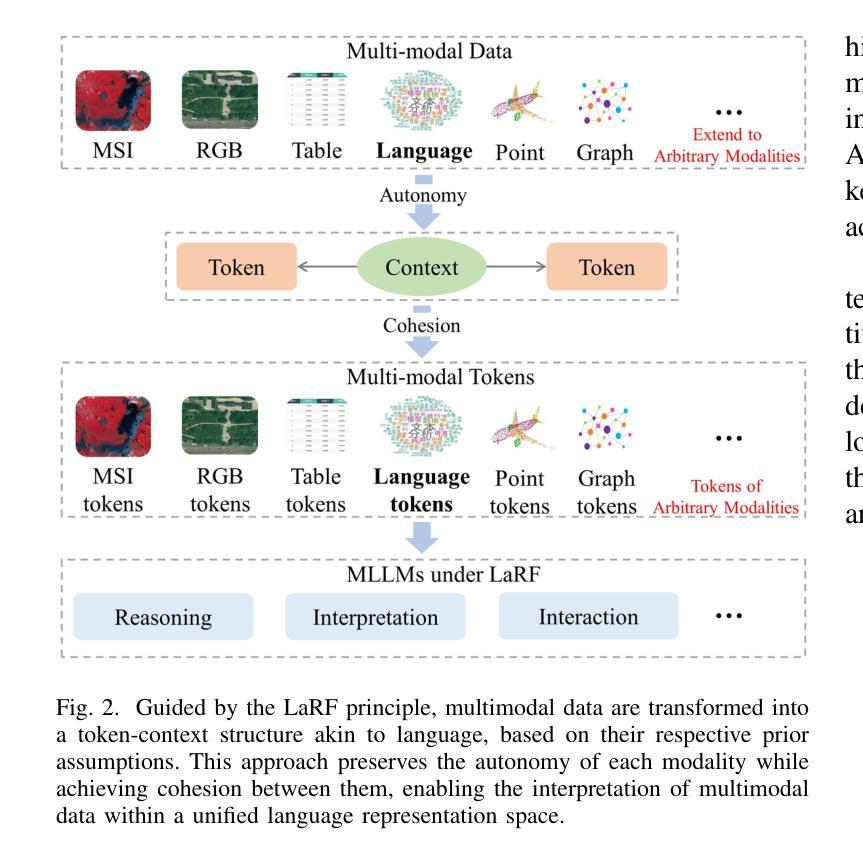
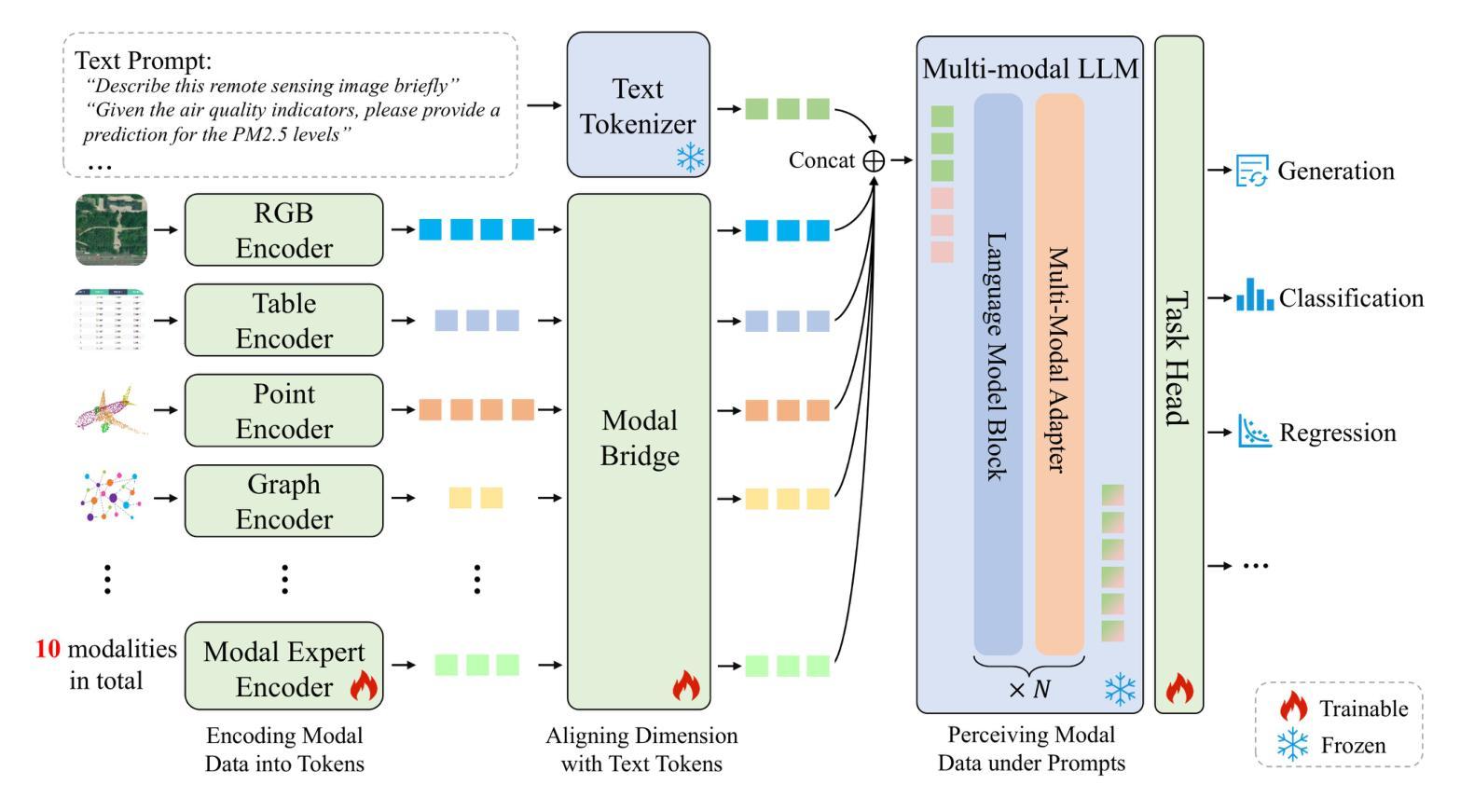
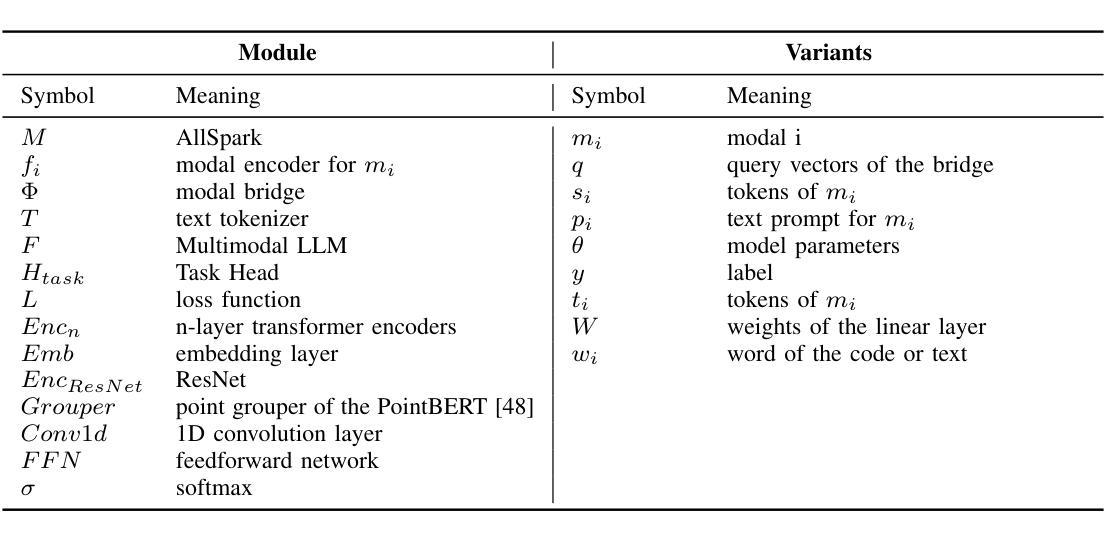
Bongard-OpenWorld: Few-Shot Reasoning for Free-form Visual Concepts in the Real World
Authors:Rujie Wu, Xiaojian Ma, Zhenliang Zhang, Wei Wang, Qing Li, Song-Chun Zhu, Yizhou Wang
We introduce Bongard-OpenWorld, a new benchmark for evaluating real-world few-shot reasoning for machine vision. It originates from the classical Bongard Problems (BPs): Given two sets of images (positive and negative), the model needs to identify the set that query images belong to by inducing the visual concepts, which is exclusively depicted by images from the positive set. Our benchmark inherits the few-shot concept induction of the original BPs while adding the two novel layers of challenge: 1) open-world free-form concepts, as the visual concepts in Bongard-OpenWorld are unique compositions of terms from an open vocabulary, ranging from object categories to abstract visual attributes and commonsense factual knowledge; 2) real-world images, as opposed to the synthetic diagrams used by many counterparts. In our exploration, Bongard-OpenWorld already imposes a significant challenge to current few-shot reasoning algorithms. We further investigate to which extent the recently introduced Large Language Models (LLMs) and Vision-Language Models (VLMs) can solve our task, by directly probing VLMs, and combining VLMs and LLMs in an interactive reasoning scheme. We even conceived a neuro-symbolic reasoning approach that reconciles LLMs & VLMs with logical reasoning to emulate the human problem-solving process for Bongard Problems. However, none of these approaches manage to close the human-machine gap, as the best learner achieves 64% accuracy while human participants easily reach 91%. We hope Bongard-OpenWorld can help us better understand the limitations of current visual intelligence and facilitate future research on visual agents with stronger few-shot visual reasoning capabilities.
我们介绍了Bongard-OpenWorld,这是一个新的基准测试,用于评估机器视觉在现实世界的少量样本推理能力。它源于经典的Bongard问题(BPs):给定两组图像(正面和负面),模型需要通过诱导视觉概念来判断查询图像属于哪一组,这些视觉概念仅由正面组的图像唯一描述。我们的基准测试继承了原始Bongard问题的少量样本概念诱导,同时增加了两个新的挑战层面:1)开放世界的自由形式概念,Bongard-OpenWorld中的视觉概念是由开放词汇表中的术语独特组合而成的,从对象类别到抽象视觉属性和常识知识;2)现实世界图像,与许多同类测试使用的合成图表相反。在我们的探索中,Bongard-OpenWorld已经对当前少量样本推理算法构成了重大挑战。我们进一步调查了最近引入的大型语言模型(LLMs)和视觉语言模型(VLMs)能在多大程度上解决我们的任务,通过直接探测VLMs,并将VLMs和LLMs结合在一种交互推理方案中。我们还构思了一种神经符号推理方法,该方法协调了LLMs和VLMs与逻辑推理,以模拟人类解决Bongard问题的过程。然而,没有任何一种方法能够弥补人机差距,因为最好的学习者只能达到64%的准确率,而人类参与者可以轻松达到91%。我们希望Bongard-OpenWorld能帮助我们更好地了解当前视觉智能的局限性,并促进未来对具有更强少量样本视觉推理能力的视觉智能体的研究。
论文及项目相关链接
PDF Accepted to ICLR 2024
Summary
本文介绍了一个新基准测试Bongard-OpenWorld,用于评估机器视觉在现实世界的少样本推理能力。该基准测试源自经典的Bongard问题,需要模型通过识别图像集来推断查询图像所属的类别。它继承了原始Bongard问题的少样本概念归纳,并增加了两个新的挑战:一是开放世界自由形式的概念,二是使用真实世界图像而非合成图像。文章探讨了大型语言模型(LLMs)和视觉语言模型(VLMs)在该任务上的表现,并提出了一种神经符号推理方法。然而,当前的方法仍无法缩小与人类的差距,最佳学习模型的准确率仅为64%,而人类参与者的准确率高达91%。希望通过Bongard-OpenWorld了解当前视觉智能的局限性并推动未来研究。
Key Takeaways
- Bongard-OpenWorld是一个新的基准测试,用于评估机器在真实世界少样本推理的能力。
- 该测试继承了Bongard问题的少样本概念归纳,并增加了开放世界自由形式概念和真实世界图像两个新的挑战。
- 文章探讨了大型语言模型和视觉语言模型在该任务上的表现。
- 提出了一种神经符号推理方法来模拟人类解决问题的过程。
- 当前的方法仍无法缩小与人类的差距,最佳模型的准确率仅为64%。
- 通过Bongard-OpenWorld可以了解当前视觉智能的局限性。
点此查看论文截图
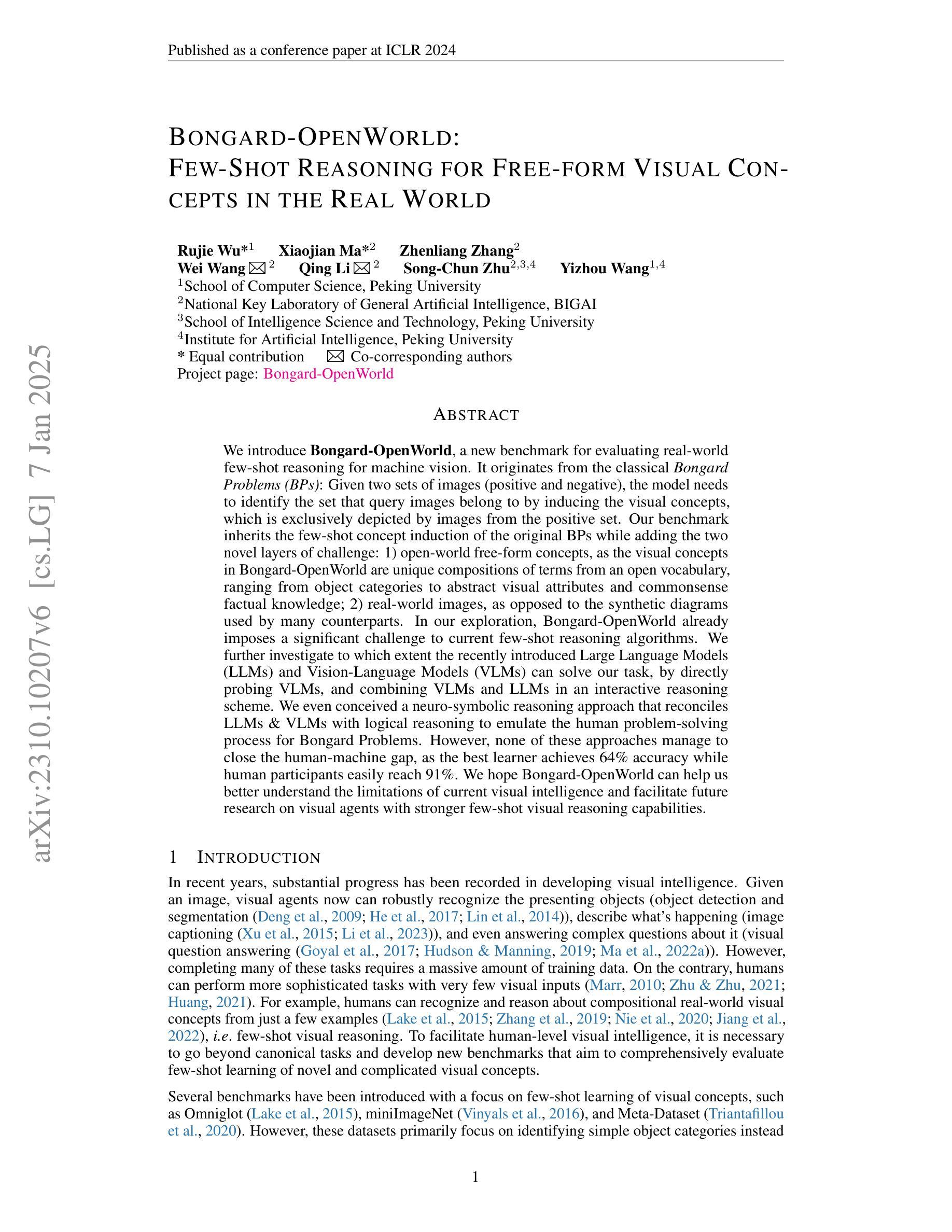
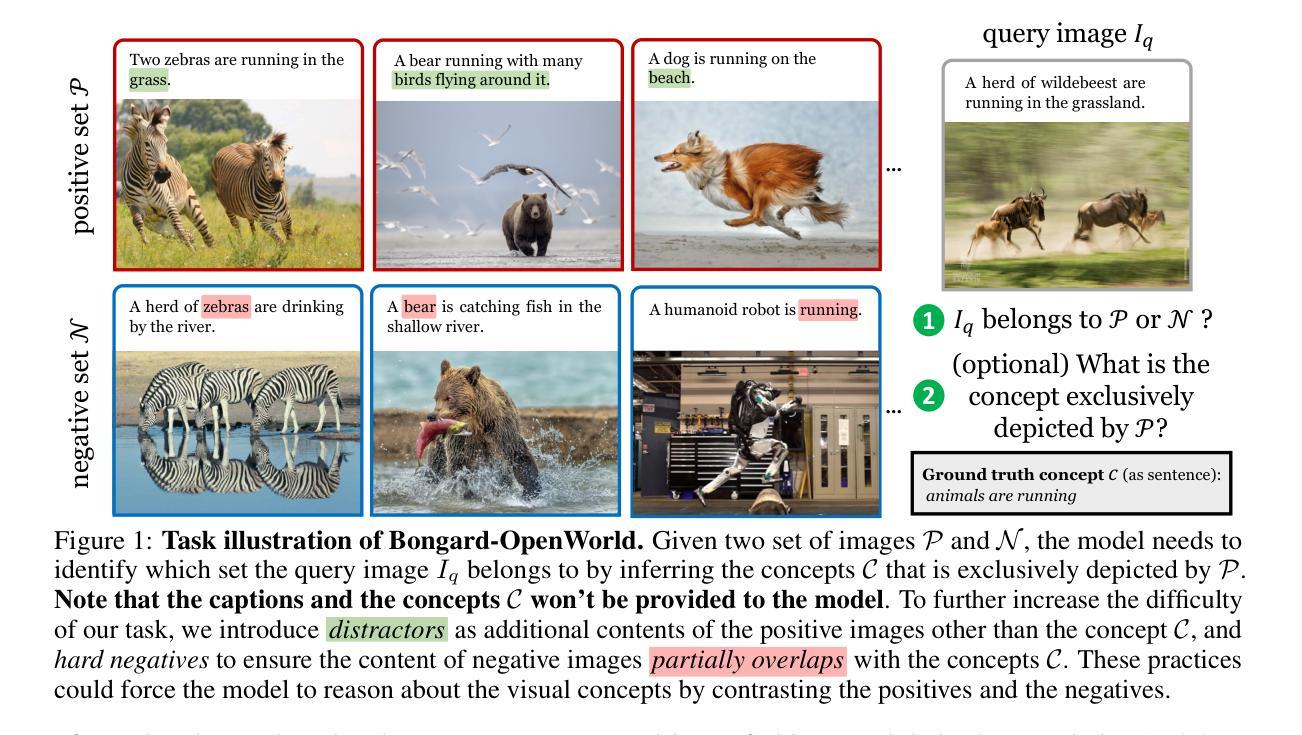
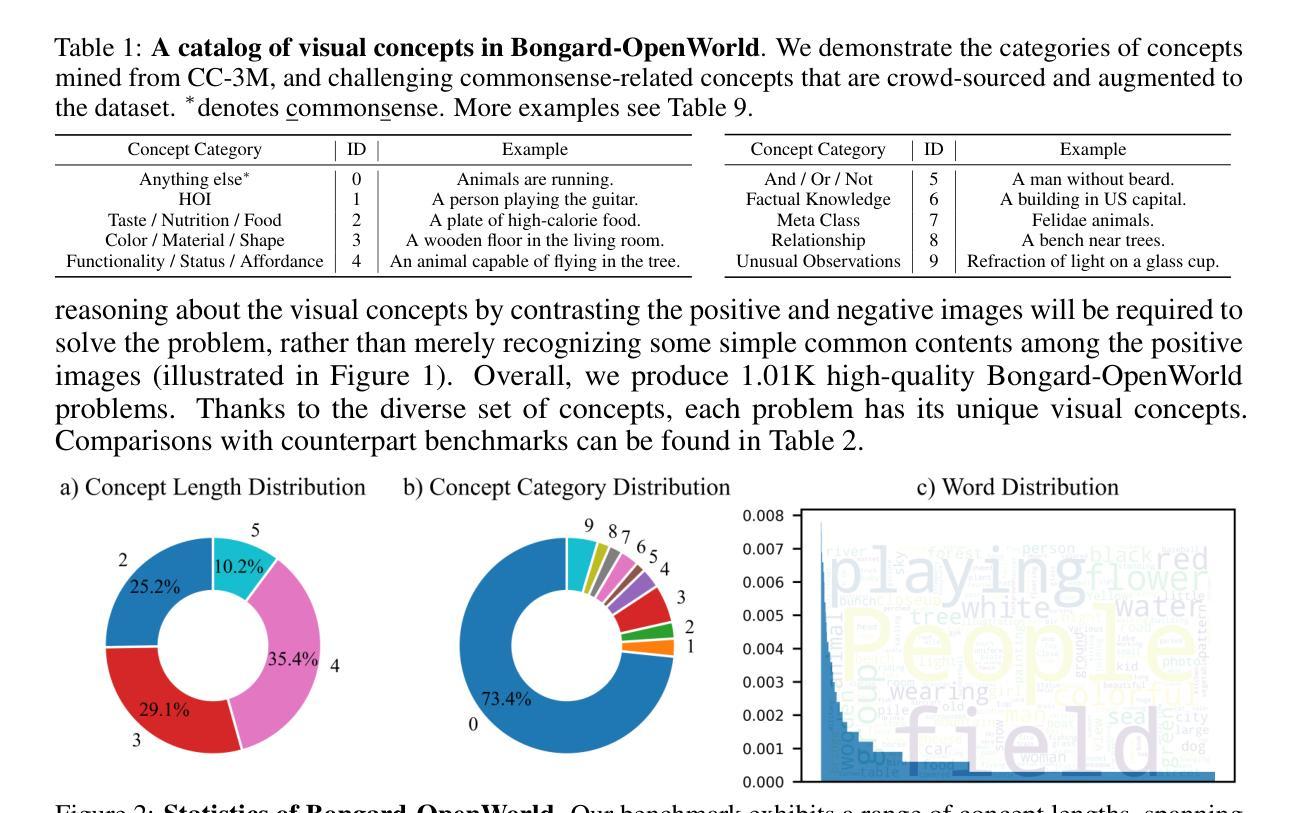
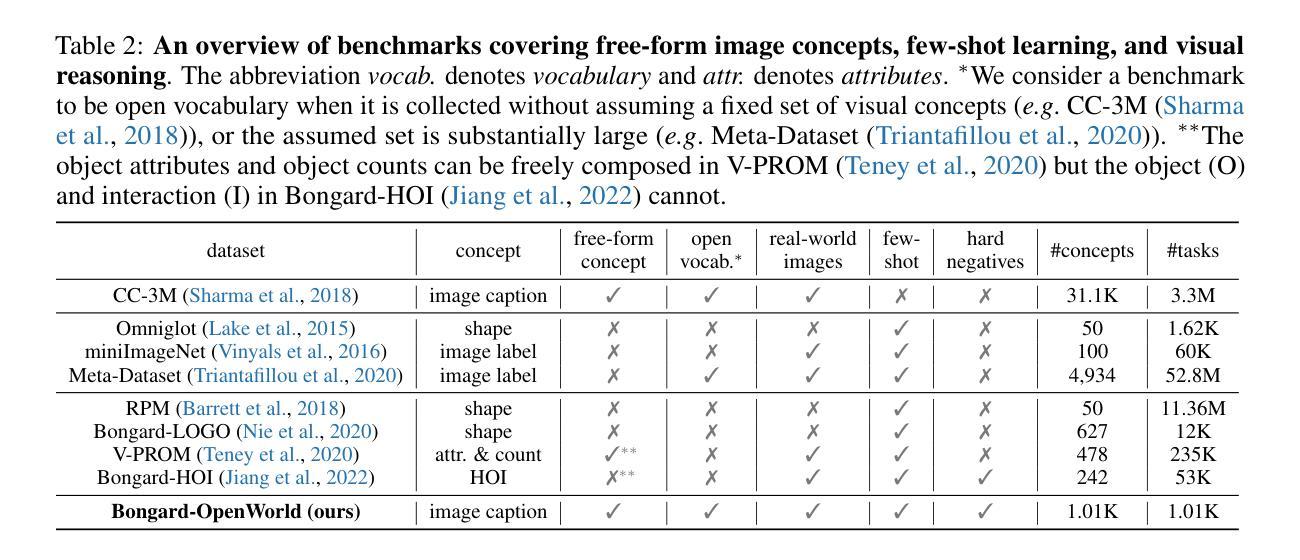
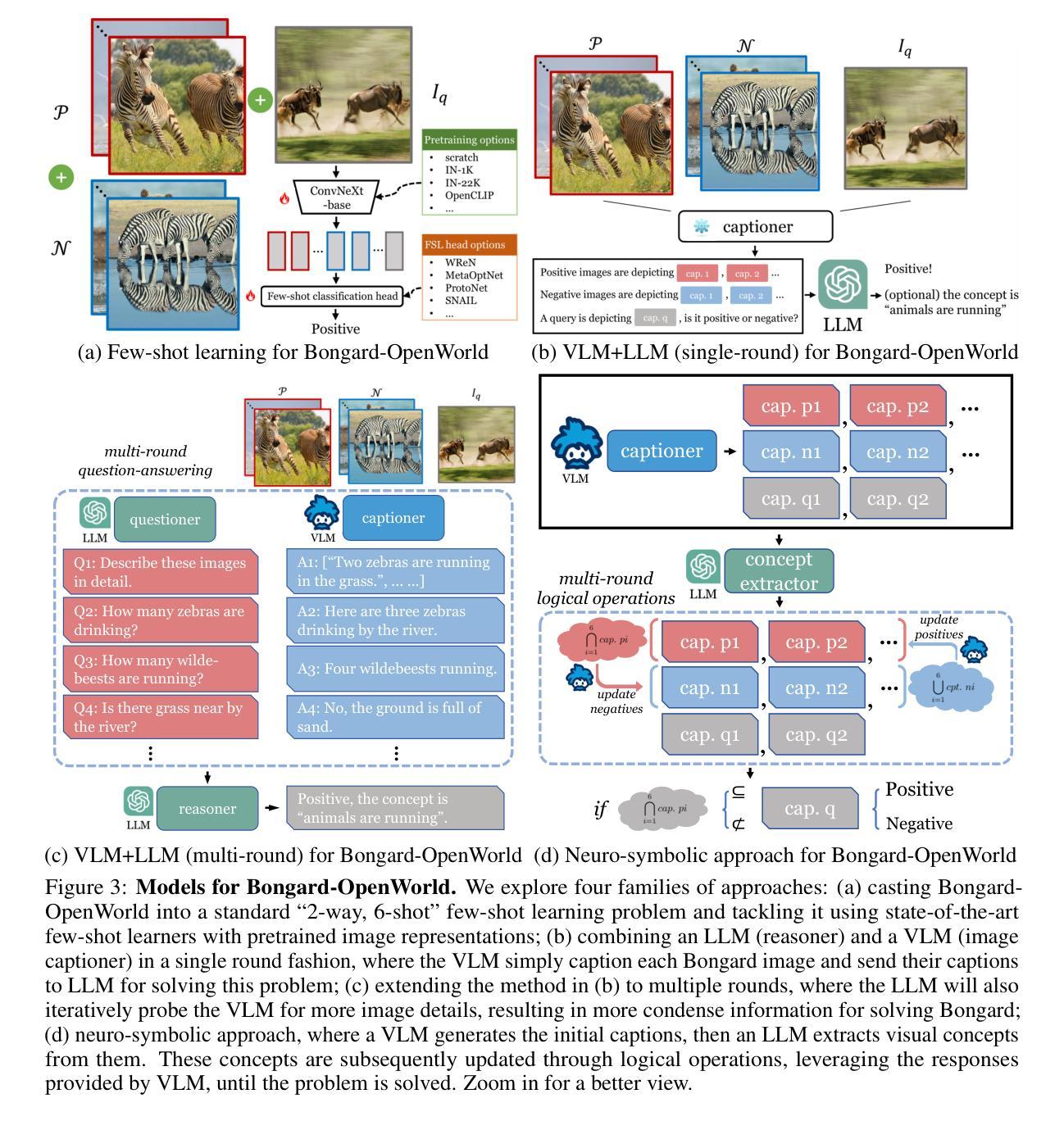