⚠️ 以下所有内容总结都来自于 大语言模型的能力,如有错误,仅供参考,谨慎使用
🔴 请注意:千万不要用于严肃的学术场景,只能用于论文阅读前的初筛!
💗 如果您觉得我们的项目对您有帮助 ChatPaperFree ,还请您给我们一些鼓励!⭐️ HuggingFace免费体验
2025-01-10 更新
H-MBA: Hierarchical MamBa Adaptation for Multi-Modal Video Understanding in Autonomous Driving
Authors:Siran Chen, Yuxiao Luo, Yue Ma, Yu Qiao, Yali Wang
With the prevalence of Multimodal Large Language Models(MLLMs), autonomous driving has encountered new opportunities and challenges. In particular, multi-modal video understanding is critical to interactively analyze what will happen in the procedure of autonomous driving. However, videos in such a dynamical scene that often contains complex spatial-temporal movements, which restricts the generalization capacity of the existing MLLMs in this field. To bridge the gap, we propose a novel Hierarchical Mamba Adaptation (H-MBA) framework to fit the complicated motion changes in autonomous driving videos. Specifically, our H-MBA consists of two distinct modules, including Context Mamba (C-Mamba) and Query Mamba (Q-Mamba). First, C-Mamba contains various types of structure state space models, which can effectively capture multi-granularity video context for different temporal resolutions. Second, Q-Mamba flexibly transforms the current frame as the learnable query, and attentively selects multi-granularity video context into query. Consequently, it can adaptively integrate all the video contexts of multi-scale temporal resolutions to enhance video understanding. Via a plug-and-play paradigm in MLLMs, our H-MBA shows the remarkable performance on multi-modal video tasks in autonomous driving, e.g., for risk object detection, it outperforms the previous SOTA method with 5.5% mIoU improvement.
随着多模态大型语言模型(MLLMs)的普及,自动驾驶遇到了新的机遇和挑战。特别是,多模态视频理解对于交互式分析自动驾驶过程中的情况至关重要。然而,这类动态场景中的视频常常包含复杂的时空运动,这限制了现有MLLMs在此领域的泛化能力。为了弥补这一差距,我们提出了一种新颖的分层次玛姆巴适应(H-MBA)框架,以适应自动驾驶视频中复杂的运动变化。具体来说,我们的H-MBA由两个独特的模块组成,包括上下文玛姆巴(C-玛姆巴)和查询玛姆巴(Q-玛姆巴)。首先,C-玛姆巴包含各种类型的结构状态空间模型,可以有效地捕获不同时间分辨率的多粒度视频上下文。其次,Q-玛姆巴灵活地将当前帧转换为可学习的查询,并专注于将多粒度视频上下文转换为查询。因此,它可以自适应地整合所有多尺度时间分辨率的视频上下文,以增强视频理解。通过MLLMs的即插即用范式,我们的H-MBA在自动驾驶的多模态视频任务上表现出卓越的性能,例如在风险对象检测方面,它比之前的最佳方法提高了5.5%的mIoU。
论文及项目相关链接
PDF 7 pages, 4 figures
Summary
多模态大型语言模型(MLLMs)的普及为自动驾驶带来了新的机遇和挑战。关键的多模态视频理解在分析自动驾驶过程中的交互方面发挥着至关重要的作用。针对自动驾驶视频中复杂动态场景的空间时间运动,我们提出了分层玛姆巴适应(H-MBA)框架,包括上下文玛姆巴(C-Mamba)和查询玛姆巴(Q-Mamba)两个模块。C-Mamba通过结构状态空间模型捕捉多粒度视频上下文,而Q-Mamba将当前帧转换为可学习查询,并关注选择多粒度视频上下文进行查询。该框架能自适应融合多尺度时间分辨率的视频上下文,提升视频理解效果,在自动驾驶的多模态视频任务中表现优异。
Key Takeaways
- 多模态大型语言模型(MLLMs)在自动驾驶领域带来新的机遇和挑战。
- 多模态视频理解对分析自动驾驶过程中的交互至关重要。
- 现有MLLMs在应对自动驾驶视频中复杂动态场景时存在局限性。
- 提出了分层玛姆巴适应(H-MBA)框架以应对复杂运动变化的自动驾驶视频。
- H-MBA框架包括捕捉多粒度视频上下文的C-Mamba模块和将当前帧转换为查询的Q-Mamba模块。
- H-MBA框架能自适应融合多尺度时间分辨率的视频上下文,提升视频理解。
点此查看论文截图
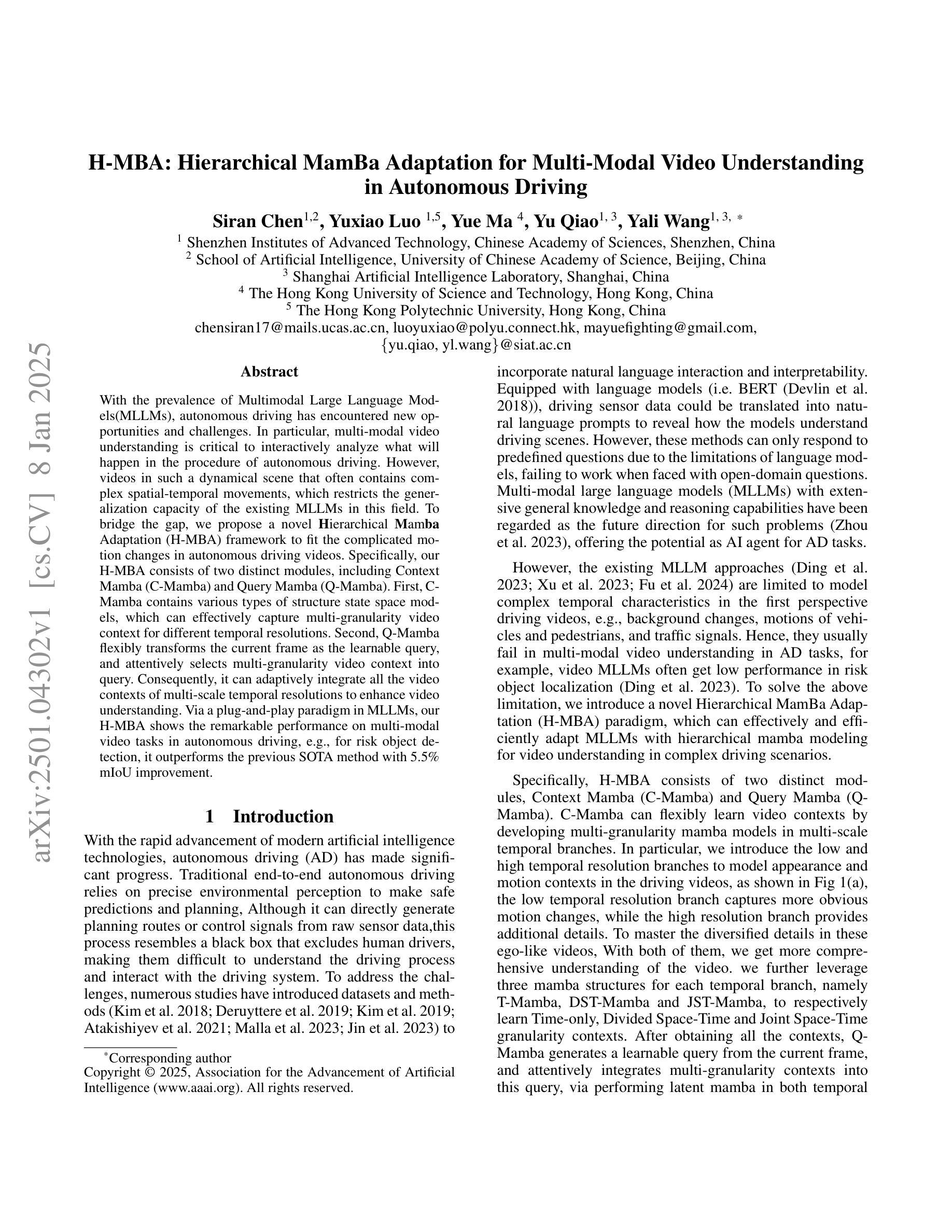
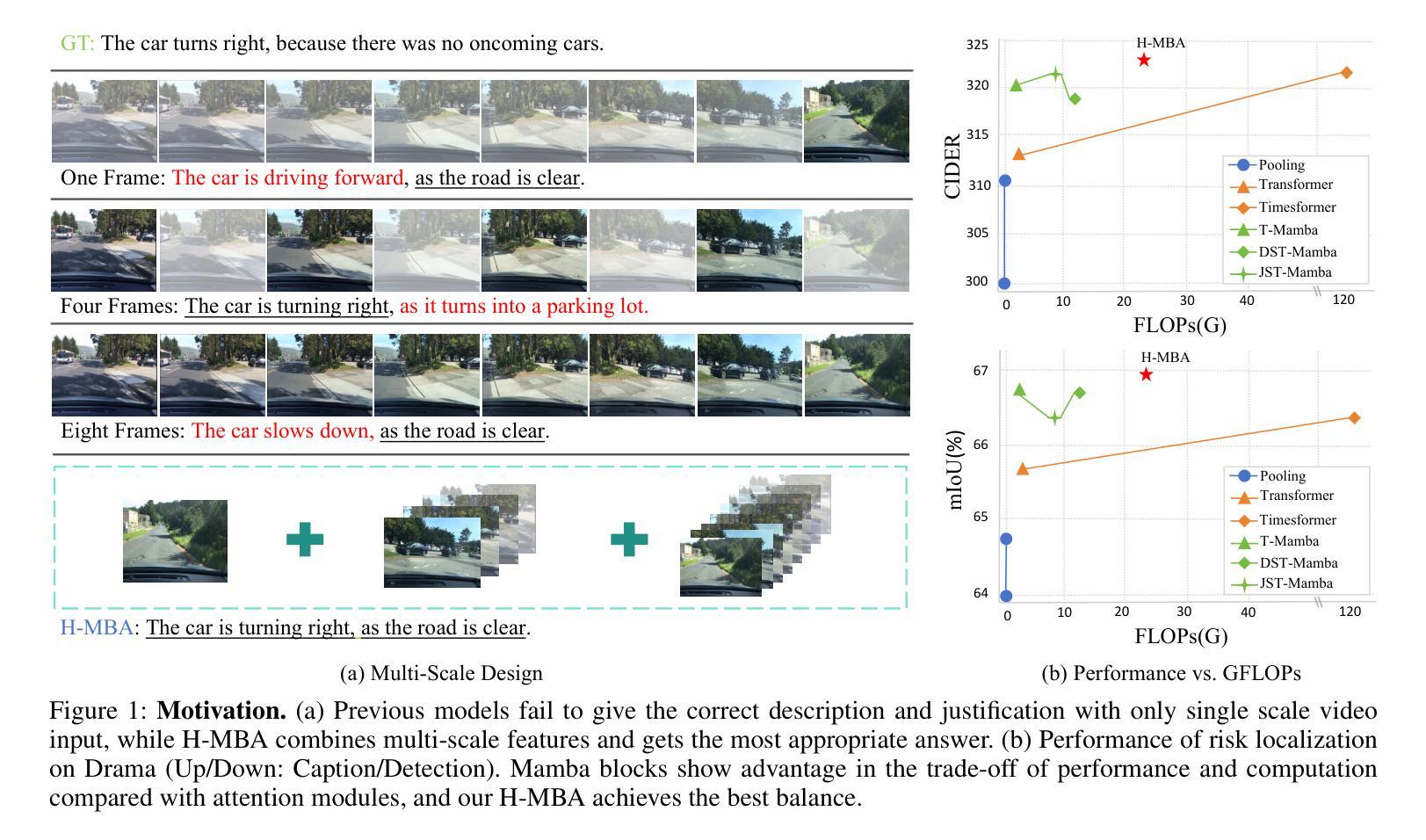
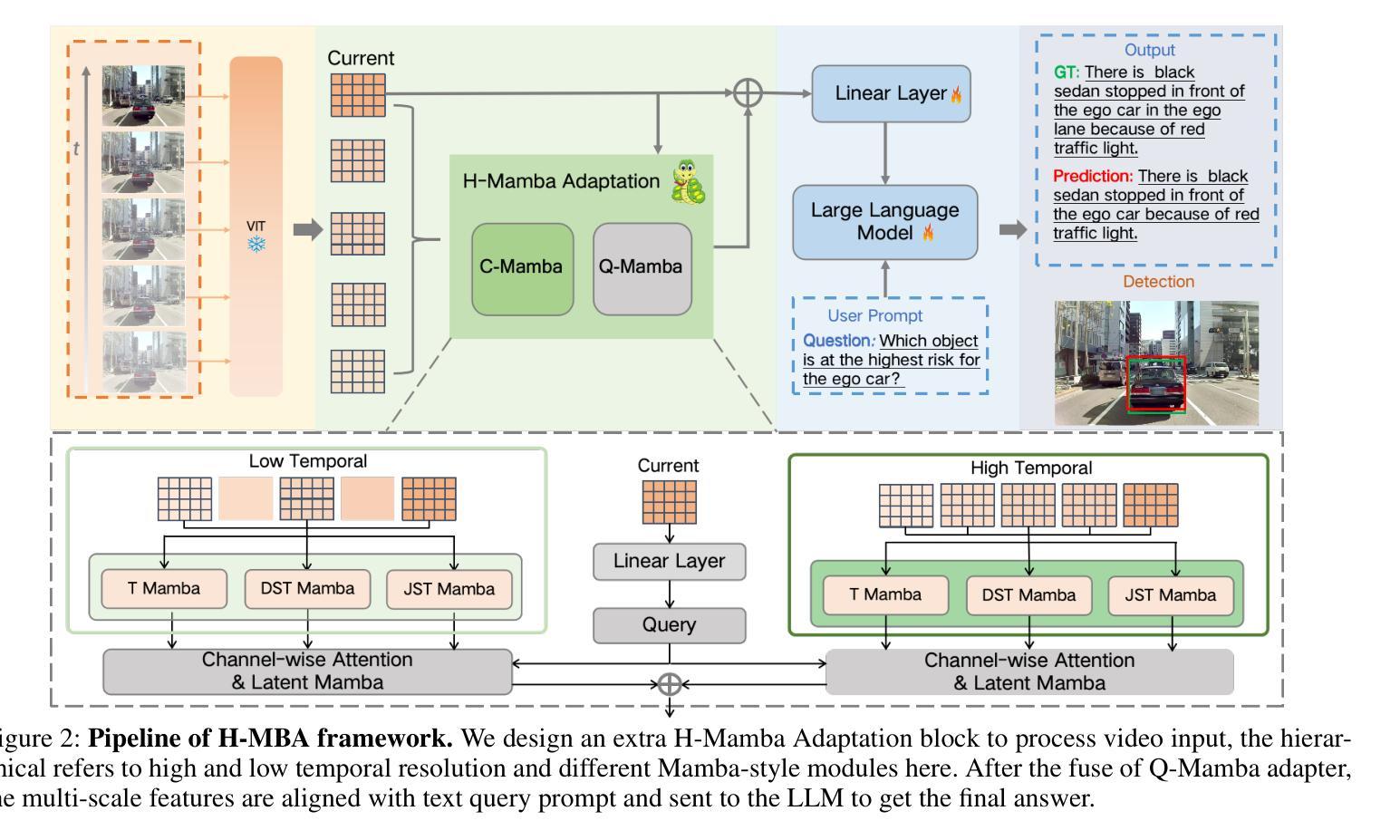
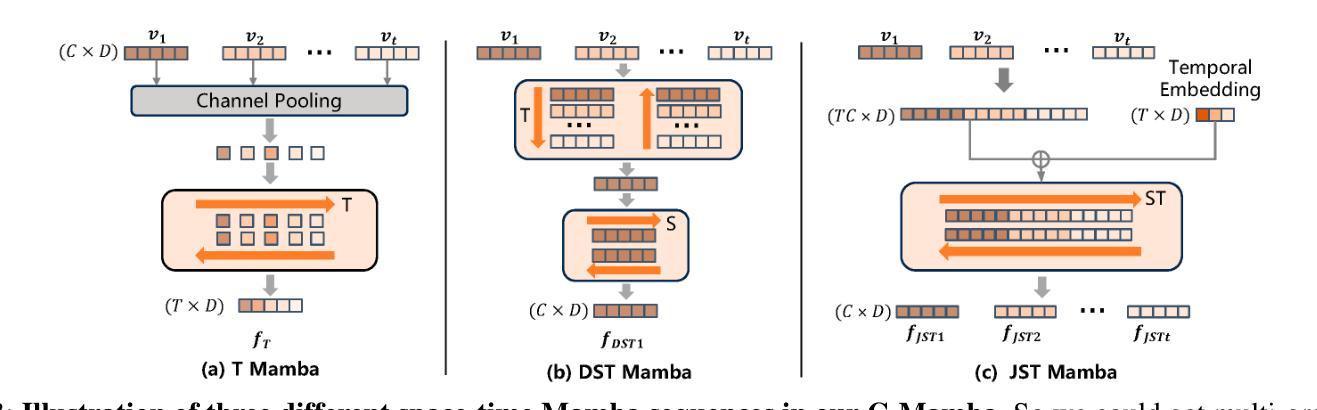
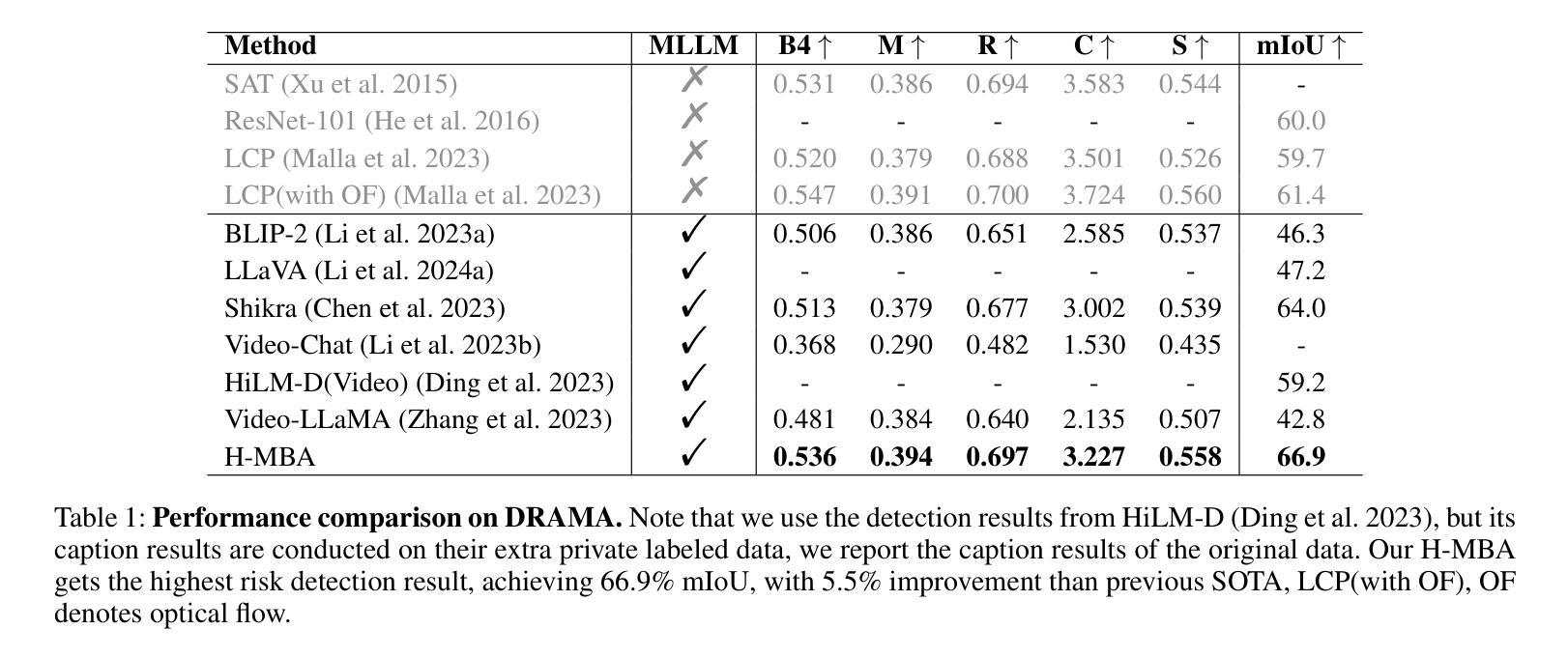
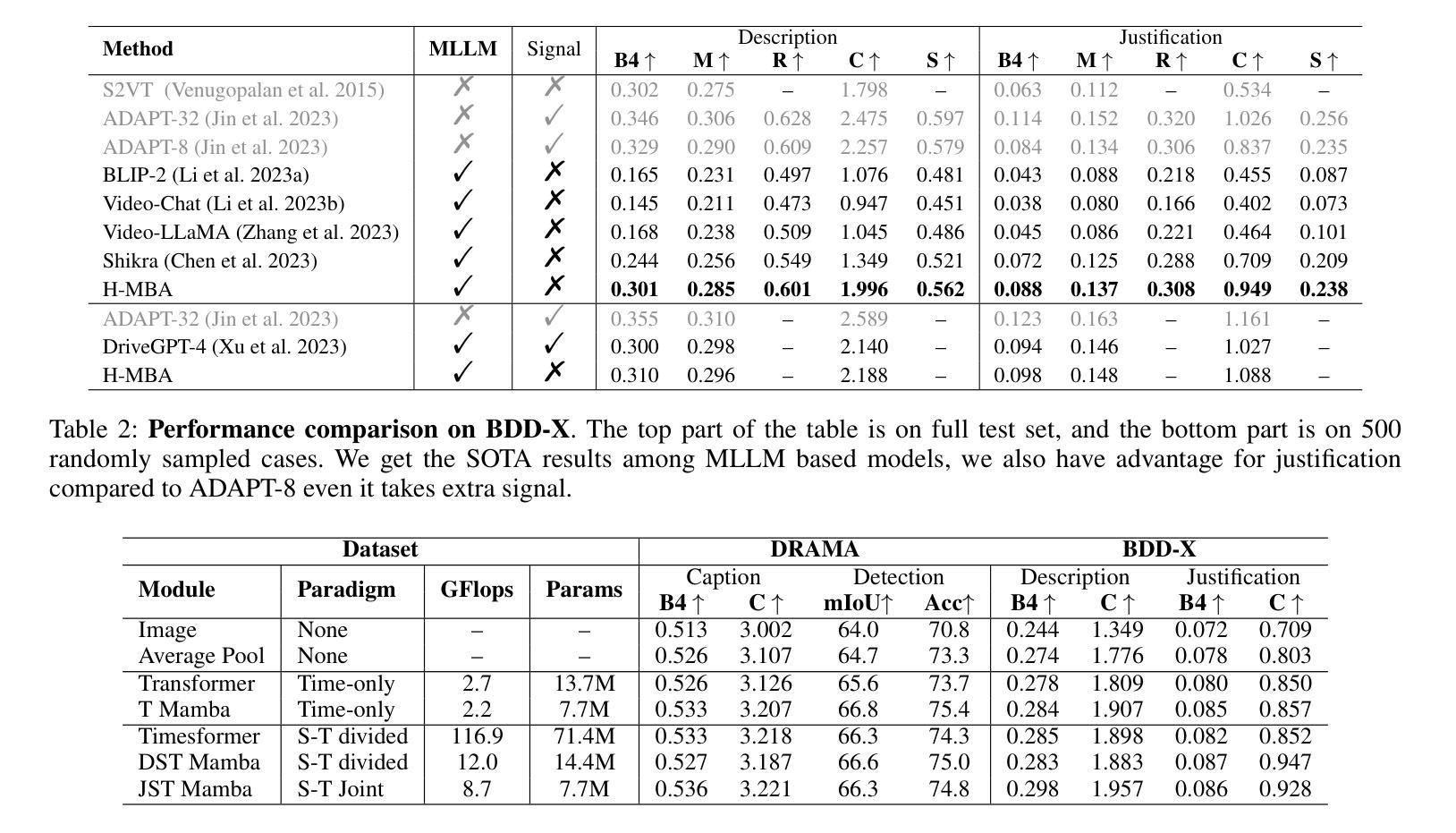