⚠️ 以下所有内容总结都来自于 大语言模型的能力,如有错误,仅供参考,谨慎使用
🔴 请注意:千万不要用于严肃的学术场景,只能用于论文阅读前的初筛!
💗 如果您觉得我们的项目对您有帮助 ChatPaperFree ,还请您给我们一些鼓励!⭐️ HuggingFace免费体验
2025-01-10 更新
FatesGS: Fast and Accurate Sparse-View Surface Reconstruction using Gaussian Splatting with Depth-Feature Consistency
Authors:Han Huang, Yulun Wu, Chao Deng, Ge Gao, Ming Gu, Yu-Shen Liu
Recently, Gaussian Splatting has sparked a new trend in the field of computer vision. Apart from novel view synthesis, it has also been extended to the area of multi-view reconstruction. The latest methods facilitate complete, detailed surface reconstruction while ensuring fast training speed. However, these methods still require dense input views, and their output quality significantly degrades with sparse views. We observed that the Gaussian primitives tend to overfit the few training views, leading to noisy floaters and incomplete reconstruction surfaces. In this paper, we present an innovative sparse-view reconstruction framework that leverages intra-view depth and multi-view feature consistency to achieve remarkably accurate surface reconstruction. Specifically, we utilize monocular depth ranking information to supervise the consistency of depth distribution within patches and employ a smoothness loss to enhance the continuity of the distribution. To achieve finer surface reconstruction, we optimize the absolute position of depth through multi-view projection features. Extensive experiments on DTU and BlendedMVS demonstrate that our method outperforms state-of-the-art methods with a speedup of 60x to 200x, achieving swift and fine-grained mesh reconstruction without the need for costly pre-training.
近期,高斯摊铺(Gaussian Splatting)在计算机视觉领域掀起了一股新热潮。除了新颖视图合成(novel view synthesis)之外,它还扩展到了多视图重建(multi-view reconstruction)领域。最新的方法能够实现完整且详细的表面重建,同时确保快速训练速度。然而,这些方法仍需要密集输入视图,并且在视图稀疏时输出质量显著下降。我们观察到,高斯基本体(Gaussian primitives)倾向于过度适应有限的训练视图,导致出现噪声浮动和重建表面不完整的问题。在本文中,我们提出了一种创新性的稀疏视图重建框架,该框架利用视图内深度和多视图特征一致性来实现极其精确的表面重建。具体来说,我们利用单眼深度排序信息来监督补丁内深度分布的连续性,并采用平滑损失(smoothness loss)来提高分布的连续性。为了实现更精细的表面重建,我们通过多视图投影特征优化深度的绝对位置。在DTU和BlendedMVS上的大量实验表明,我们的方法在速度上比最先进的方法提高了60倍至200倍,实现了无需昂贵预训练的快速且精细的网格重建。
论文及项目相关链接
PDF Accepted by AAAI 2025. Project page: https://alvin528.github.io/FatesGS/
Summary
本文介绍了基于高斯拼贴的新稀疏视图重建框架,该框架利用单眼深度排名信息监督深度分布的一致性,并利用平滑损失增强分布的连续性。通过多视图投影特征优化深度绝对位置,实现了精细的表面重建。该框架在DTU和BlendedMVS上的实验表明,该方法在速度上比现有技术提高了60倍至200倍,实现了快速且精细的网格重建,无需昂贵的预训练。
Key Takeaways
- 高斯拼贴已成为计算机视觉领域的新趋势,不仅用于新颖视图合成,还扩展到多视图重建。
- 现有方法虽然能够实现完整详细的表面重建,但需要密集的输入视图,且在稀疏视图下输出质量显著下降。
- 高斯原始数据在稀疏训练视图下容易过拟合,导致噪声浮动和不完全重建表面。
- 本文提出了一个创新的稀疏视图重建框架,利用单眼深度排名信息监督深度分布的一致性,增强了表面重建的准确性。
- 通过利用平滑损失和多视图投影特征优化深度绝对位置,实现了更精细的表面重建。
- 该方法在DTU和BlendedMVS上的实验结果表明,其性能优于现有技术,实现了快速和精细的网格重建。
点此查看论文截图
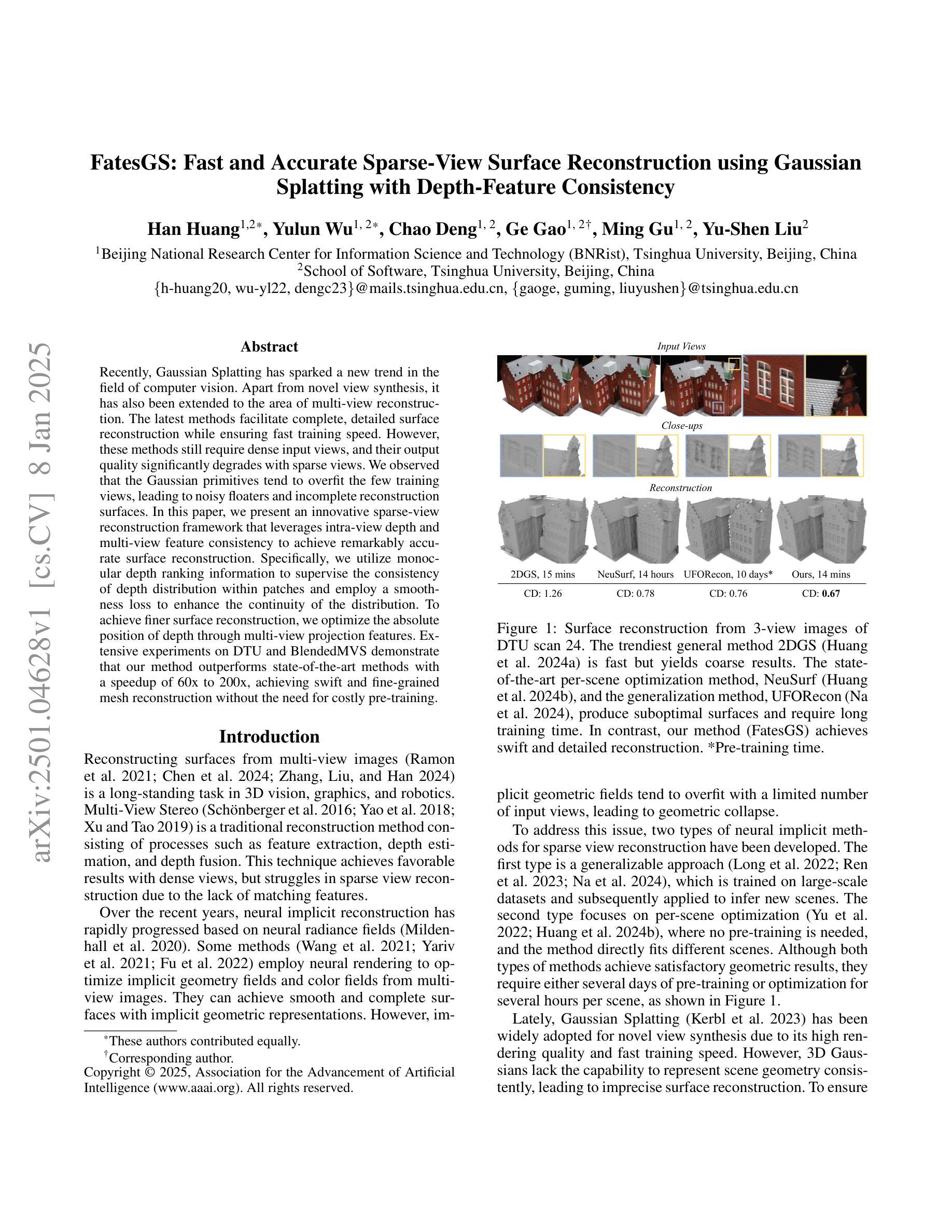
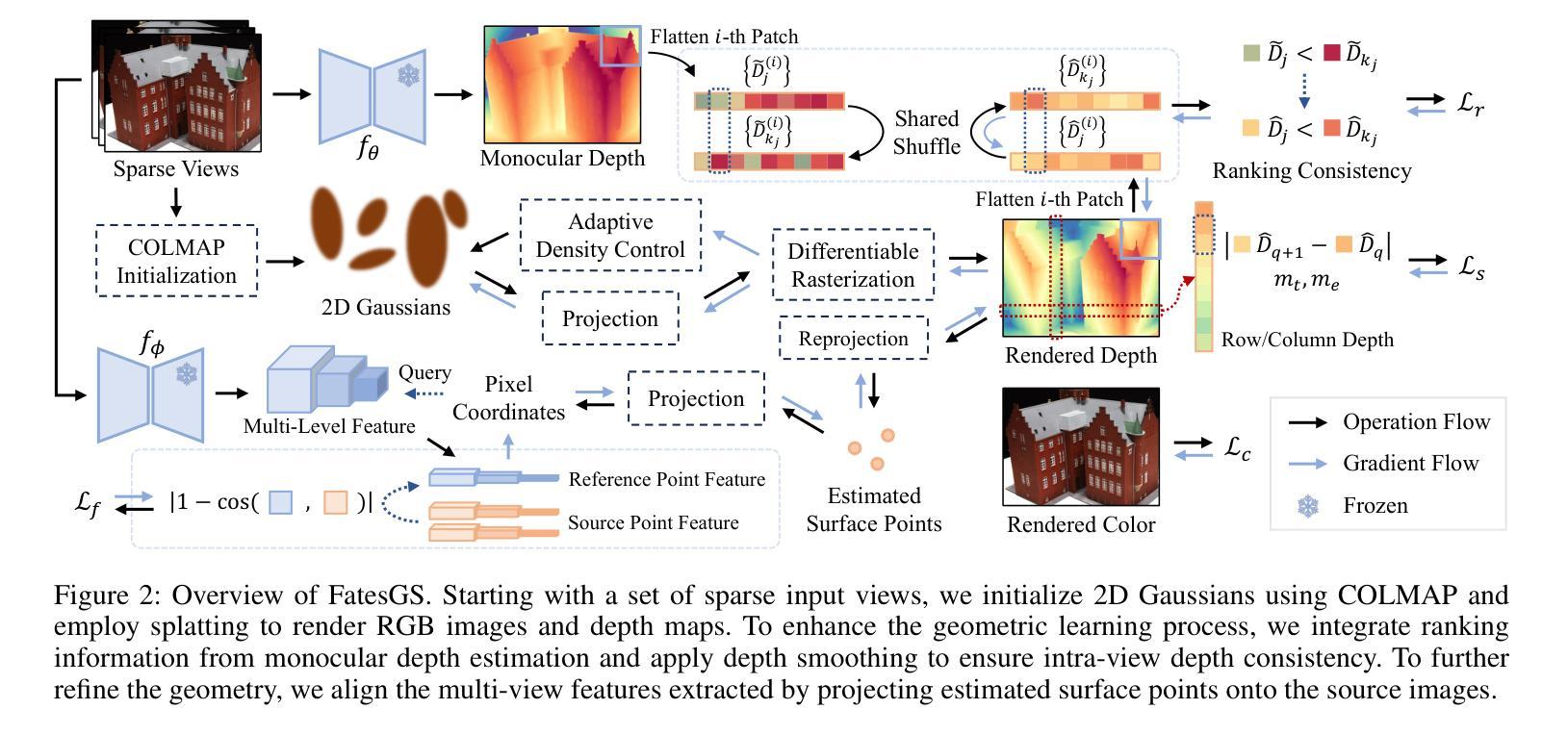
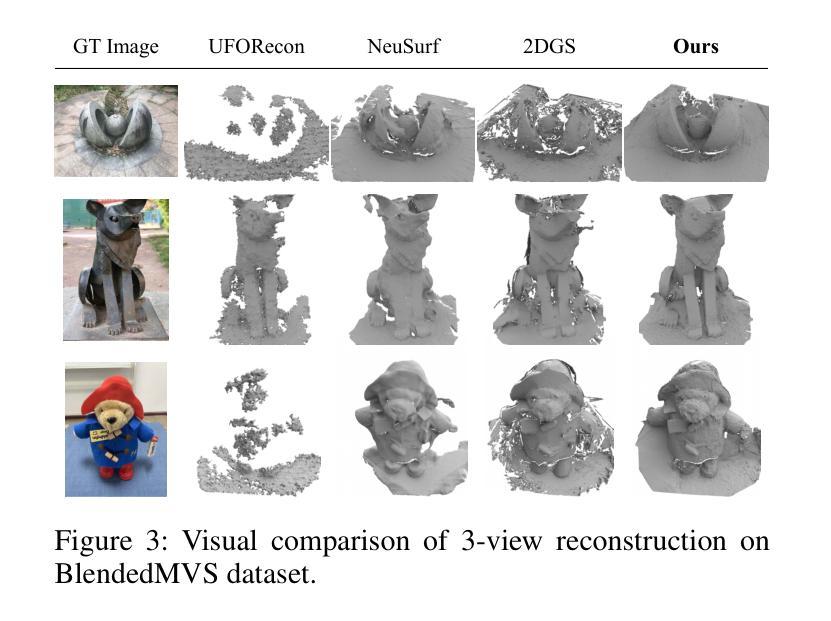
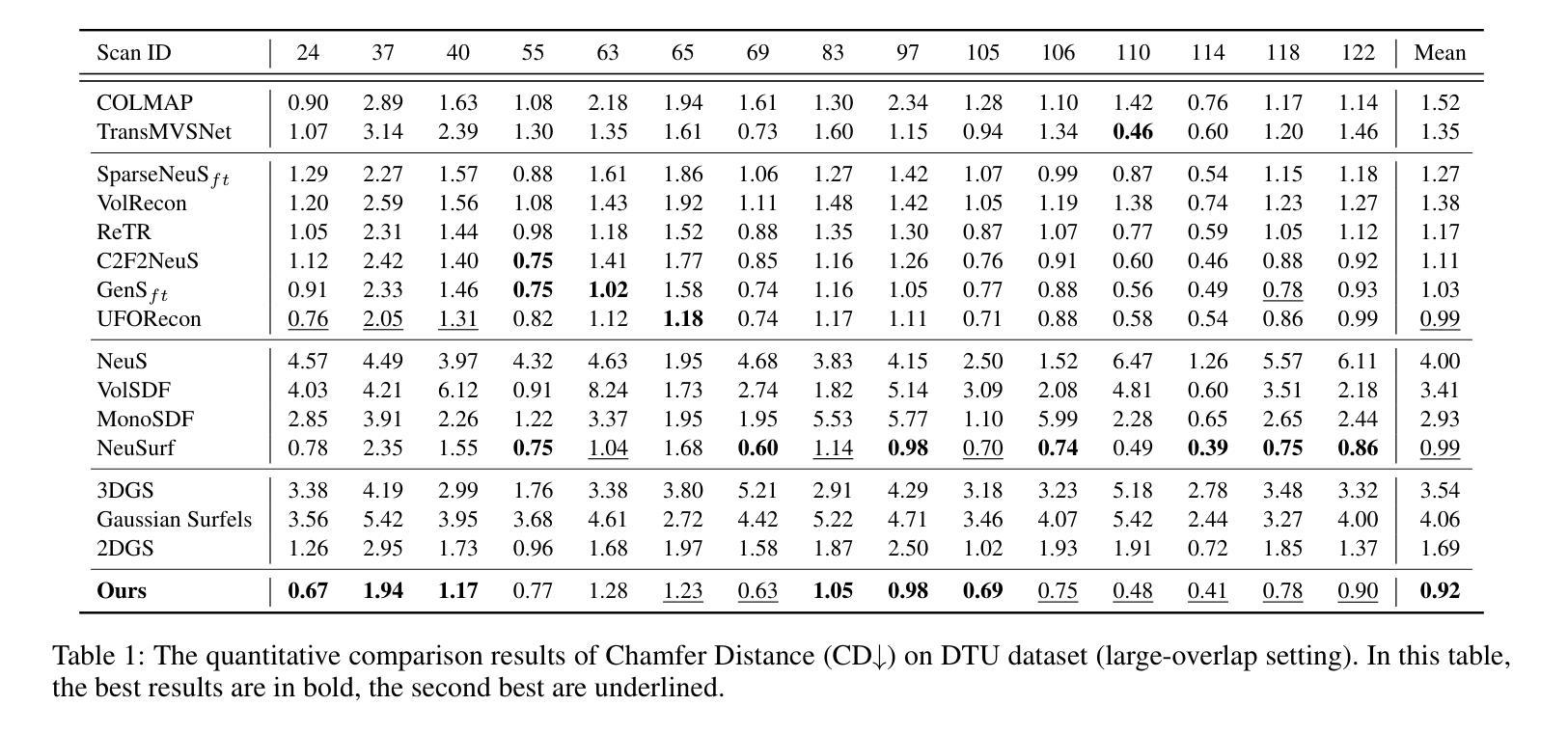
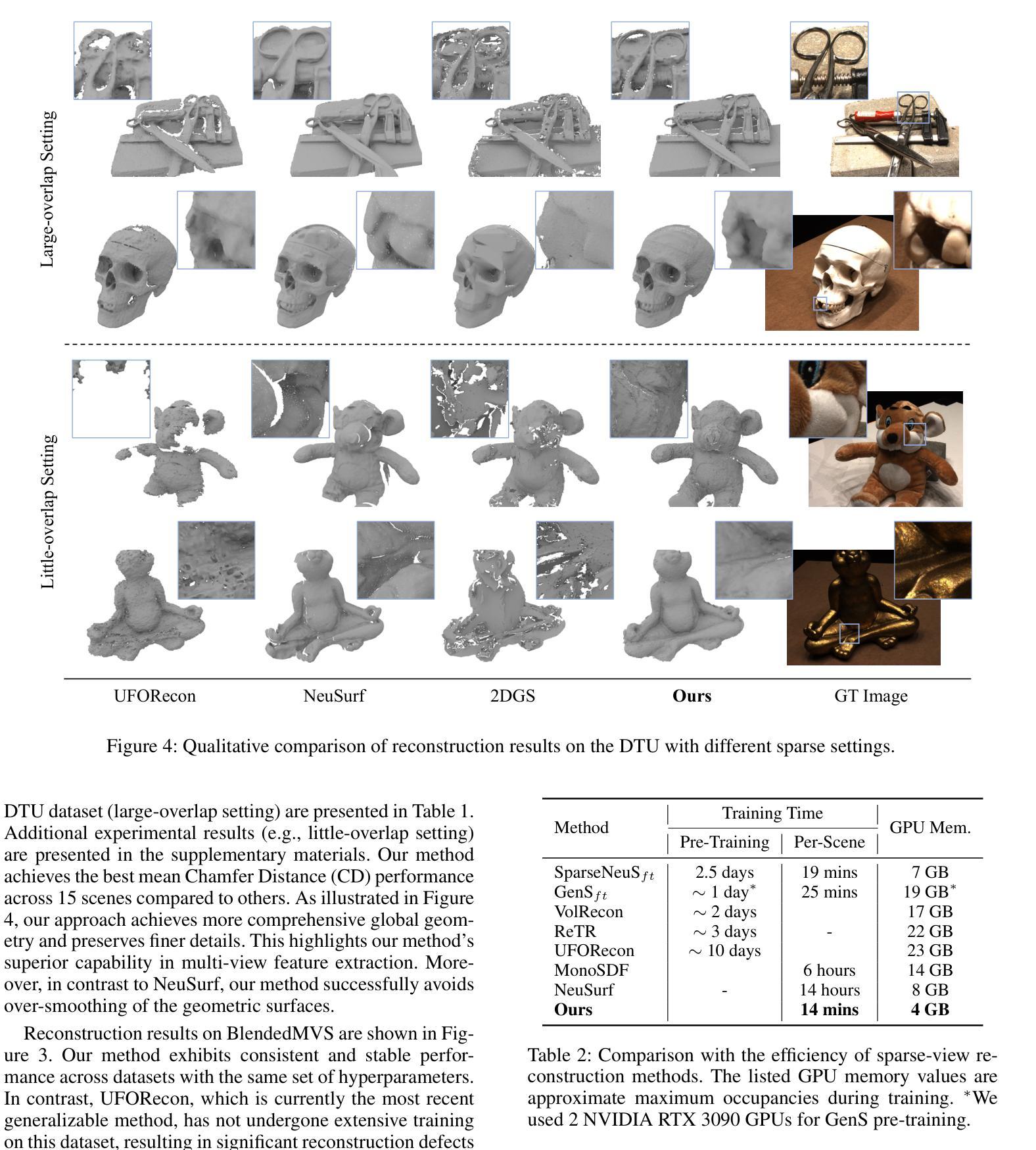