⚠️ 以下所有内容总结都来自于 大语言模型的能力,如有错误,仅供参考,谨慎使用
🔴 请注意:千万不要用于严肃的学术场景,只能用于论文阅读前的初筛!
💗 如果您觉得我们的项目对您有帮助 ChatPaperFree ,还请您给我们一些鼓励!⭐️ HuggingFace免费体验
2025-01-10 更新
iFADIT: Invertible Face Anonymization via Disentangled Identity Transform
Authors:Lin Yuan, Kai Liang, Xiong Li, Tao Wu, Nannan Wang, Xinbo Gao
Face anonymization aims to conceal the visual identity of a face to safeguard the individual’s privacy. Traditional methods like blurring and pixelation can largely remove identifying features, but these techniques significantly degrade image quality and are vulnerable to deep reconstruction attacks. Generative models have emerged as a promising solution for anonymizing faces while preserving a natural appearance.However, many still face limitations in visual quality and often overlook the potential to recover the original face from the anonymized version, which can be valuable in specific contexts such as image forensics. This paper proposes a novel framework named iFADIT, an acronym for Invertible Face Anonymization via Disentangled Identity Transform.The framework features a disentanglement architecture coupled with a secure flow-based model: the former decouples identity information from non-identifying attributes, while the latter transforms the decoupled identity into an anonymized version in an invertible manner controlled by a secret key. The anonymized face can then be reconstructed based on a pre-trained StyleGAN that ensures high image quality and realistic facial details. Recovery of the original face (aka de-anonymization) is possible upon the availability of the matching secret, by inverting the anonymization process based on the same set of model parameters. Furthermore, a dedicated secret-key mechanism along with a dual-phase training strategy is devised to ensure the desired properties of face anonymization. Qualitative and quantitative experiments demonstrate the superiority of the proposed approach in anonymity, reversibility, security, diversity, and interpretability over competing methods.
面部匿名化的目的是隐藏面部的视觉身份以保障个人隐私。传统的方法如模糊处理和像素化可以大量移除识别特征,但这些技术会显著破坏图像质量,并且容易受到深度重建攻击。生成模型的出现作为一种对面部进行匿名化处理的同时保持自然外观的很有前景的解决方案。然而,许多生成模型在视觉质量方面仍面临局限,并且往往忽视了从匿名化版本中恢复原始面孔的潜力,这在图像取证等特定情境中可能很有价值。本文提出了一种新型框架,名为iFADIT,即基于解纠缠身份变换的可逆面部匿名化的缩写。该框架结合了分离架构和安全的流模型:前者将身份信息与非识别属性分离,后者以秘密密钥控制的方式,将分离的身份可逆地转换为匿名化版本。然后,基于预训练的StyleGAN重建匿名化的面孔,确保图像质量高且面部细节逼真。在拥有匹配的密钥的情况下,可以通过逆转匿名化过程并基于相同的模型参数集恢复原始面孔(即反匿名化)。此外,设计了一个专用的秘密密钥机制以及双阶段训练策略,以确保面部匿名化所需的属性。定性和定量实验证明了该方法在匿名性、可逆性、安全性、多样性和可解释性方面优于其他方法。
论文及项目相关链接
摘要
基于生成模型的新型面部匿名技术利用可倒置的秘密键,有效进行身份解耦并保留面部匿名版本的高图像质量和逼真细节。与传统方法相比,此方法更能有效保护隐私且难以进行深度重建攻击。此框架由可拆解身份转换的身份识别分离架构和安全流模型组成,旨在确保在拥有相应秘密密钥的情况下恢复原始面部信息。此外,其还拥有专用的秘密密钥机制和双阶段训练策略来保障面部匿名所需的各项特性。实验结果证明了其在匿名性、可逆性、安全性、多样性和可解释性方面的优越性。
关键见解
- 生成模型为解决面部匿名问题提供了新的方向,能有效去除身份特征同时保留自然外观。
- 传统模糊和像素化方法会降低图像质量并易受深度重建攻击,生成模型更为高效且有效对抗攻击。
- 新提出的框架iFADIT利用身份解耦技术,实现了面部匿名化并保证较高的图像质量。
- iFADIT采用了秘密密钥机制以实现面部匿名化和反匿名化过程可逆控制,使面部信息的恢复成为可能。此过程依赖于相同的模型参数集。
- 通过专用的秘密密钥机制和双阶段训练策略,iFADIT确保了面部匿名所需的各项特性,包括匿名性、可逆性、安全性等。
点此查看论文截图
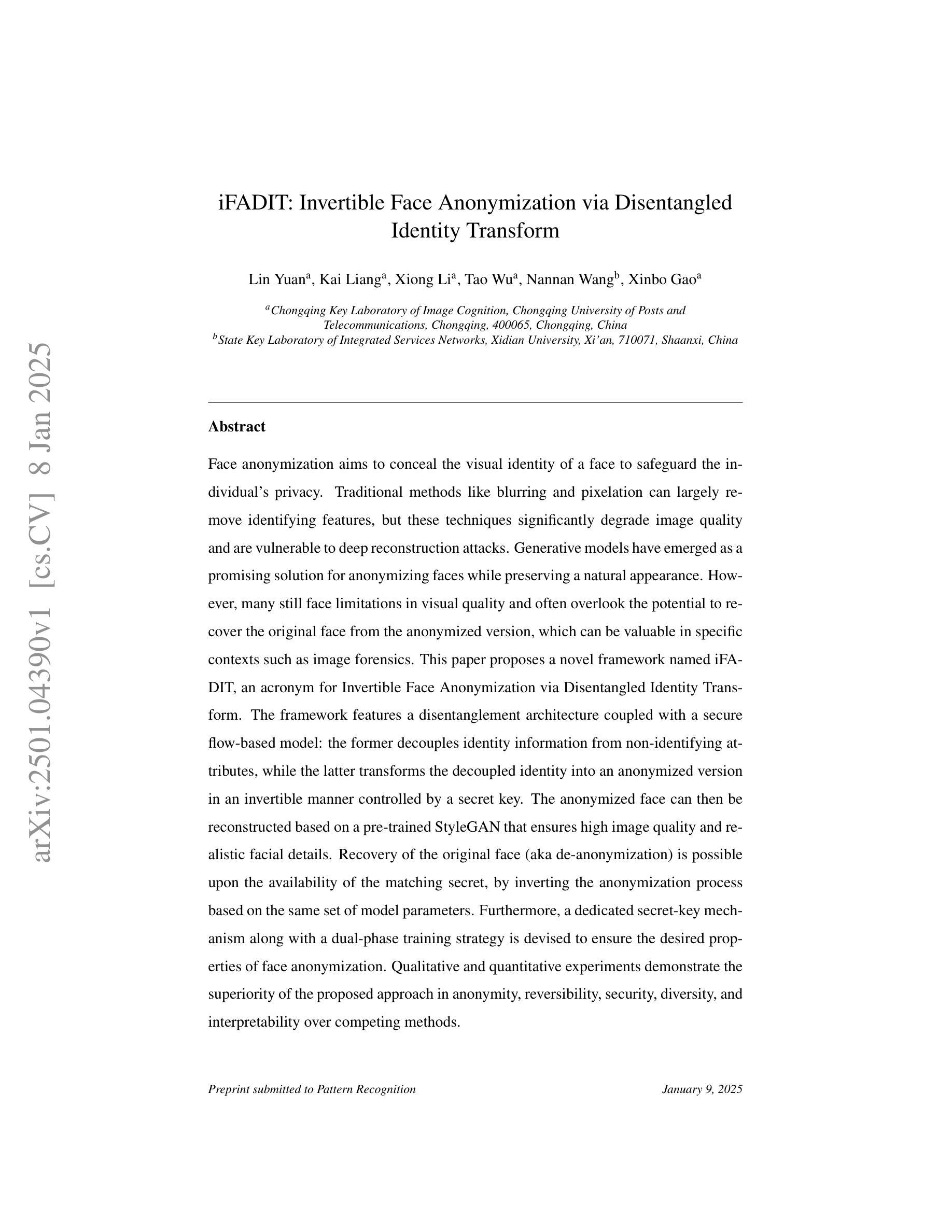
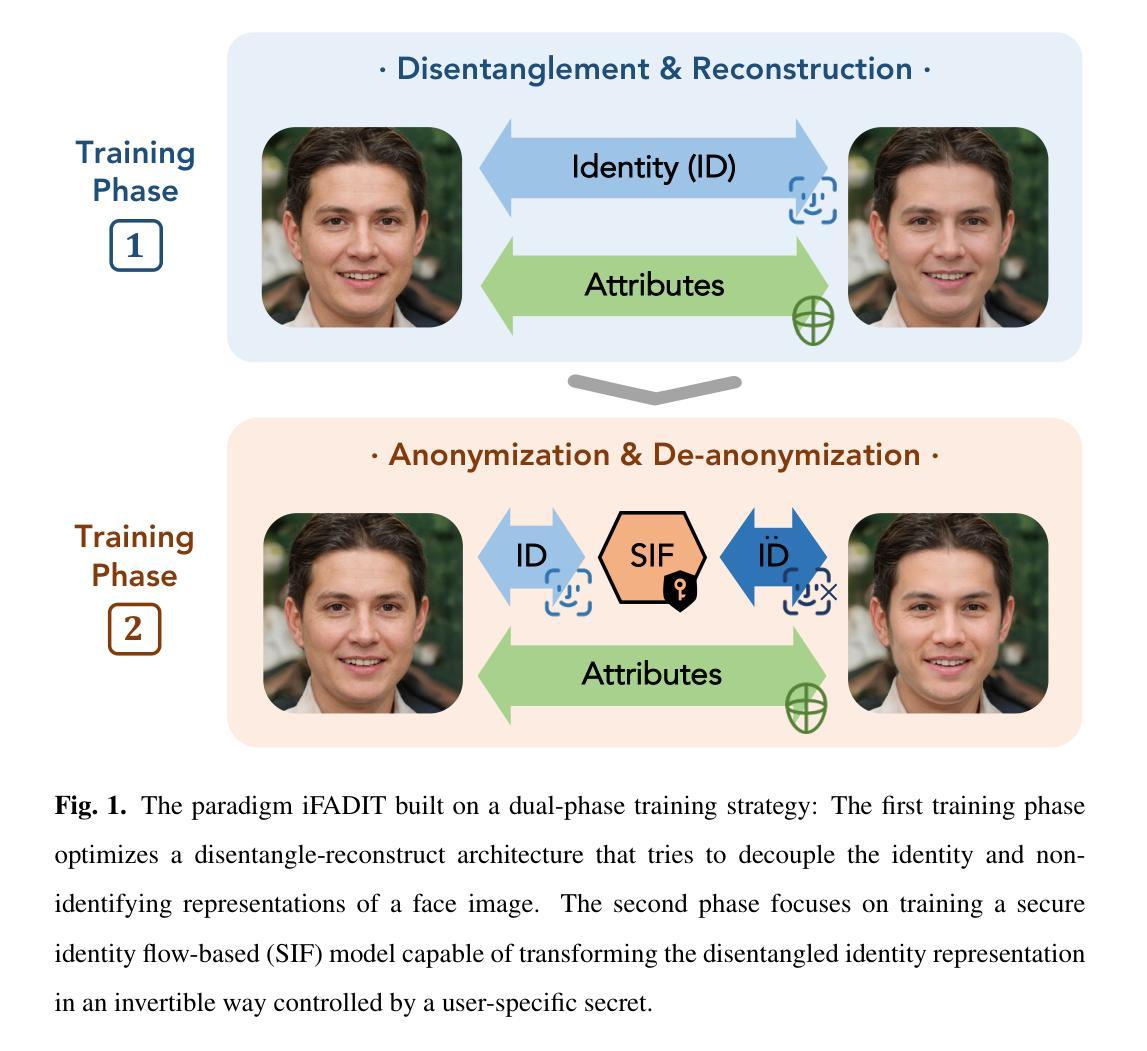