⚠️ 以下所有内容总结都来自于 大语言模型的能力,如有错误,仅供参考,谨慎使用
🔴 请注意:千万不要用于严肃的学术场景,只能用于论文阅读前的初筛!
💗 如果您觉得我们的项目对您有帮助 ChatPaperFree ,还请您给我们一些鼓励!⭐️ HuggingFace免费体验
2025-01-10 更新
FleSpeech: Flexibly Controllable Speech Generation with Various Prompts
Authors:Hanzhao Li, Yuke Li, Xinsheng Wang, Jingbin Hu, Qicong Xie, Shan Yang, Lei Xie
Controllable speech generation methods typically rely on single or fixed prompts, hindering creativity and flexibility. These limitations make it difficult to meet specific user needs in certain scenarios, such as adjusting the style while preserving a selected speaker’s timbre, or choosing a style and generating a voice that matches a character’s visual appearance. To overcome these challenges, we propose \textit{FleSpeech}, a novel multi-stage speech generation framework that allows for more flexible manipulation of speech attributes by integrating various forms of control. FleSpeech employs a multimodal prompt encoder that processes and unifies different text, audio, and visual prompts into a cohesive representation. This approach enhances the adaptability of speech synthesis and supports creative and precise control over the generated speech. Additionally, we develop a data collection pipeline for multimodal datasets to facilitate further research and applications in this field. Comprehensive subjective and objective experiments demonstrate the effectiveness of FleSpeech. Audio samples are available at https://kkksuper.github.io/FleSpeech/
可控的语音生成方法通常依赖于单一或固定的提示,这限制了创造性和灵活性。这些局限使得在某些场景中难以满足特定用户的需求,例如在保持选定演讲者的音色同时调整风格,或者选择一种风格并生成与角色视觉外观相匹配的语音。为了克服这些挑战,我们提出了FleSpeech,这是一个新型的多阶段语音生成框架,通过整合各种控制形式,允许更灵活地操作语音属性。FleSpeech采用多模态提示编码器,处理和统一不同的文本、音频和视觉提示,形成一个连贯的表示。这种方法提高了语音合成的适应性,支持对生成的语音进行创造性和精确的控制。此外,我们为开发此领域进一步的研究和应用开发了一个多模态数据集的数据收集管道。全面的主观和客观实验证明了FleSpeech的有效性。音频样本可在[https://kkksuper.github.io/FleSpeech/]找到。
论文及项目相关链接
PDF 14 pages, 3 figures
Summary
文本中提出了一种名为FleSpeech的新型多阶段语音生成框架,旨在解决现有可控语音生成方法在创意和灵活性方面的局限性。FleSpeech通过整合多种形式的控制,实现了更灵活的语音属性操作,包括处理并统一不同形式的文本、音频和视觉提示,从而提升语音合成的适应性并支持对生成语音的精准控制。此外,该研究还建立了多模态数据集的数据收集流程,为相关领域的研究和应用提供了便利。实验证明FleSpeech的有效性。
Key Takeaways
- FleSpeech是一个新型多阶段语音生成框架,旨在解决现有方法的局限,如创意和灵活性的不足。
- FleSpeech通过整合多种形式的控制,实现了更灵活的语音属性操作。
- 该框架能够处理并统一不同形式的文本、音频和视觉提示,提升语音合成的适应性。
- FleSpeech支持对生成语音的精准控制,可以满足特定用户的特定需求,如调整风格同时保持选定演讲者的音色。
- 研究建立了多模态数据集的数据收集流程,为相关领域的研究和应用提供了便利。
- FleSpeech的有效性已经通过主观和客观实验得到验证。
点此查看论文截图
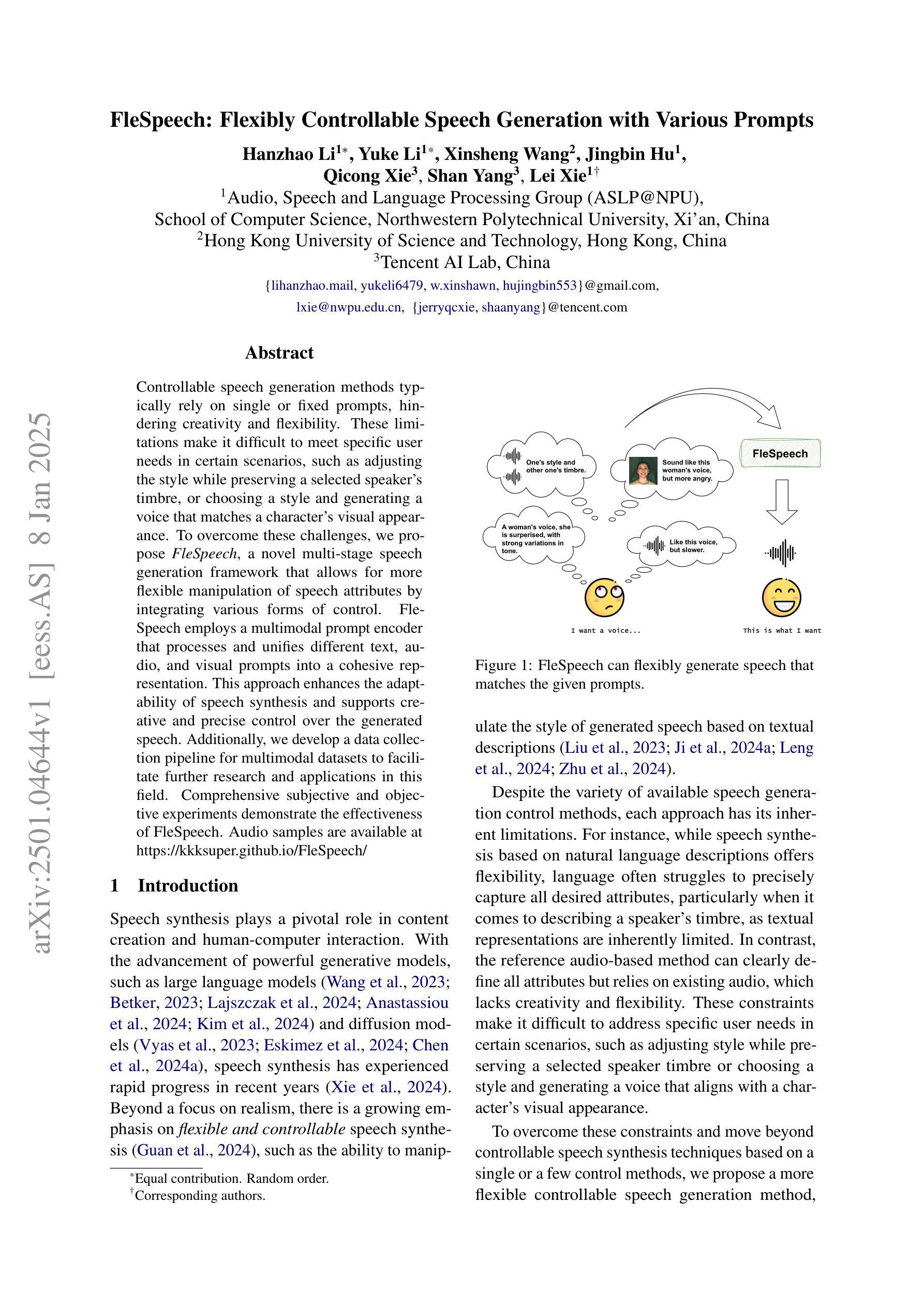
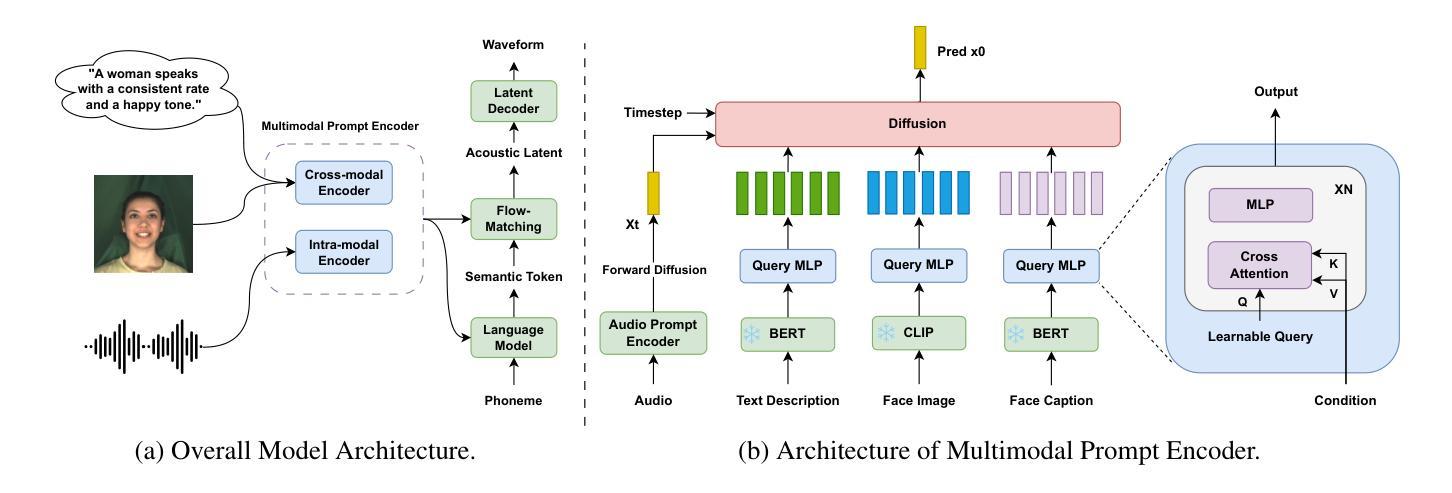
OpenOmni: Large Language Models Pivot Zero-shot Omnimodal Alignment across Language with Real-time Self-Aware Emotional Speech Synthesis
Authors:Run Luo, Ting-En Lin, Haonan Zhang, Yuchuan Wu, Xiong Liu, Min Yang, Yongbin Li, Longze Chen, Jiaming Li, Lei Zhang, Yangyi Chen, Hamid Alinejad-Rokny, Fei Huang
Recent advancements in omnimodal learning have been achieved in understanding and generation across images, text, and speech, though mainly within proprietary models. Limited omnimodal datasets and the inherent challenges associated with real-time emotional speech generation have hindered open-source progress. To address these issues, we propose openomni, a two-stage training method combining omnimodal alignment and speech generation to develop a state-of-the-art omnimodal large language model. In the alignment phase, a pre-trained speech model is further trained on text-image tasks to generalize from vision to speech in a (near) zero-shot manner, outperforming models trained on tri-modal datasets. In the speech generation phase, a lightweight decoder facilitates real-time emotional speech through training on speech tasks and preference learning. Experiments demonstrate that openomni consistently improves across omnimodal, vision-language, and speech-language evaluations, enabling natural, emotion-rich dialogues and real-time emotional speech generation.
近期多模态学习在图像、文本和语音的理解和生成方面取得了进展,但主要局限于专有模型内部。有限的多模态数据集和实时情感语音生成所固有的挑战阻碍了开源进展。为了解决这些问题,我们提出了openomni,这是一种结合多模态对齐和语音生成的两阶段训练方法,以开发先进的多模态大型语言模型。在对齐阶段,预训练的语音模型进一步在文本-图像任务上进行训练,以(接近)零样本的方式从视觉到语音进行推广,其性能优于在三元模态数据集上训练的模型。在语音生成阶段,一个轻量级的解码器通过语音任务和偏好学习进行训练,便于实时情感语音生成。实验表明,openomni在多模态、视觉语言和语音语言评估中持续提高,可实现自然、情感丰富的对话和实时情感语音生成。
论文及项目相关链接
Summary
提出了一种名为openomni的两阶段训练方法,结合多模态对齐和语音生成,以开发先进的多模态大型语言模型。首先通过对预训练的语音模型进行文本图像任务训练,实现(接近)零样本的跨视觉到语音的泛化能力。在此基础上,使用轻量级解码器进行语音生成训练,并通过偏好学习实现实时情感语音生成。此方法在跨多模态、视觉语言和语音语言评估中表现优异,可实现自然、情感丰富的对话和实时情感语音生成。
Key Takeaways
- openomni是一个两阶段的多模态学习训练方法,旨在解决多模态数据集有限和实时情感语音生成挑战的问题。
- 在对齐阶段,预训练的语音模型通过文本图像任务进行训练,实现跨视觉到语音的泛化能力。这种方法超越了基于三模态数据集训练的模型性能。
- 在语音生成阶段,轻量级解码器用于实时情感语音生成,通过语音任务和偏好学习进行训练。
- openomni在多模态、视觉语言和语音语言评估中表现优异,证明了其有效性和优越性。
- 该方法可实现自然、情感丰富的对话体验。
- 此方法利用先进的深度学习技术解决了现实世界中跨图像、文本和语音理解和生成的需求问题。尽管当前大多数研究仍限于专有模型,但openomni为开源领域提供了突破性的解决方案。
点此查看论文截图
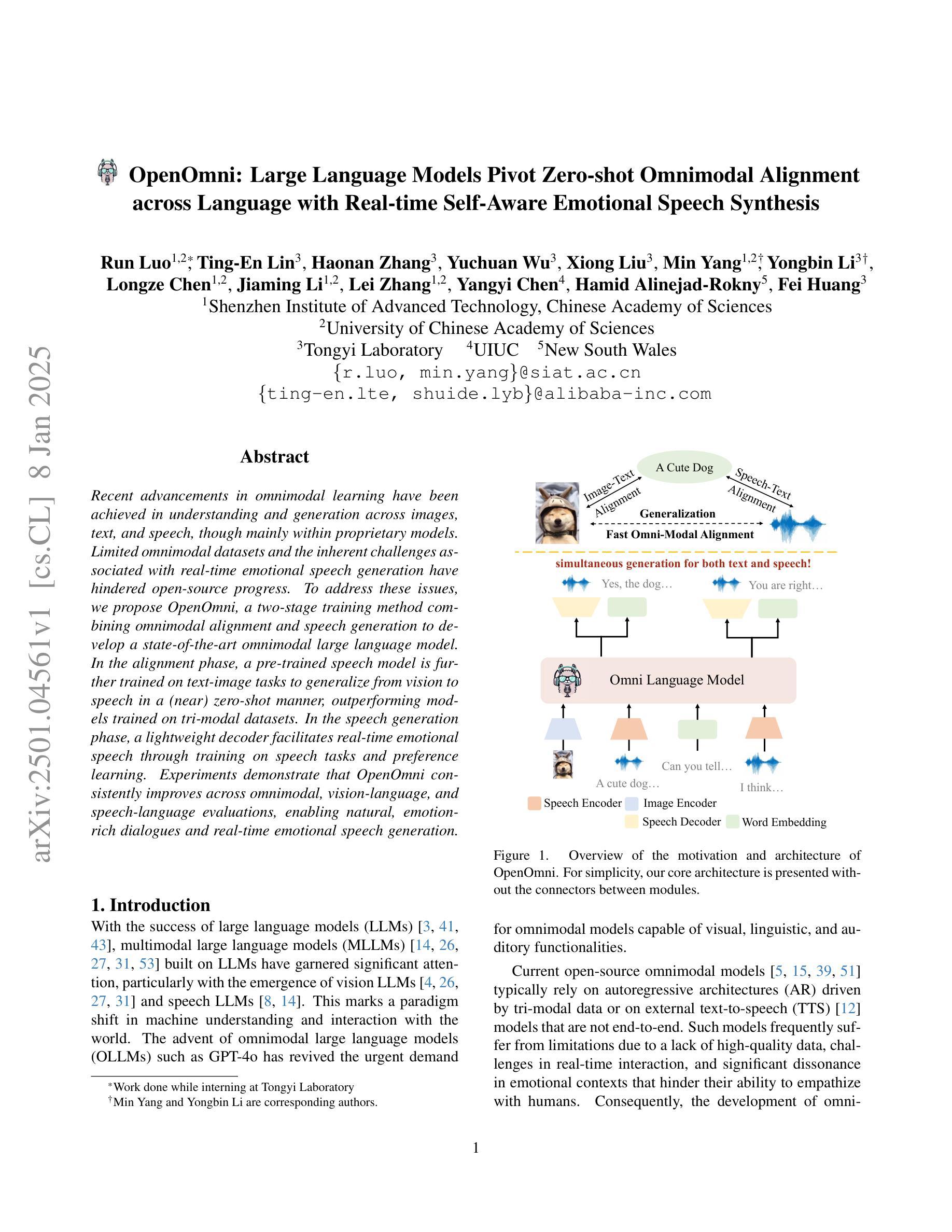
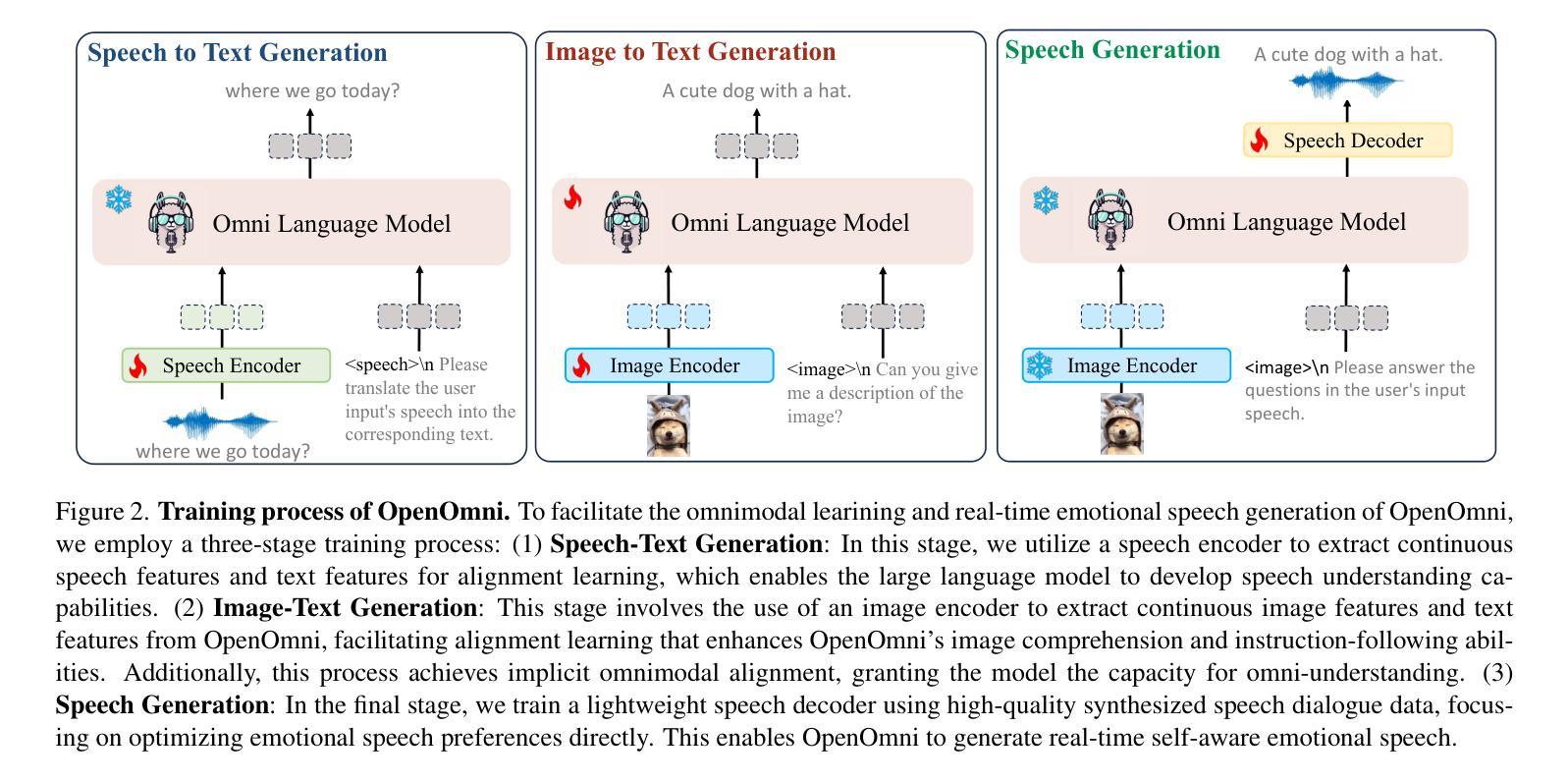
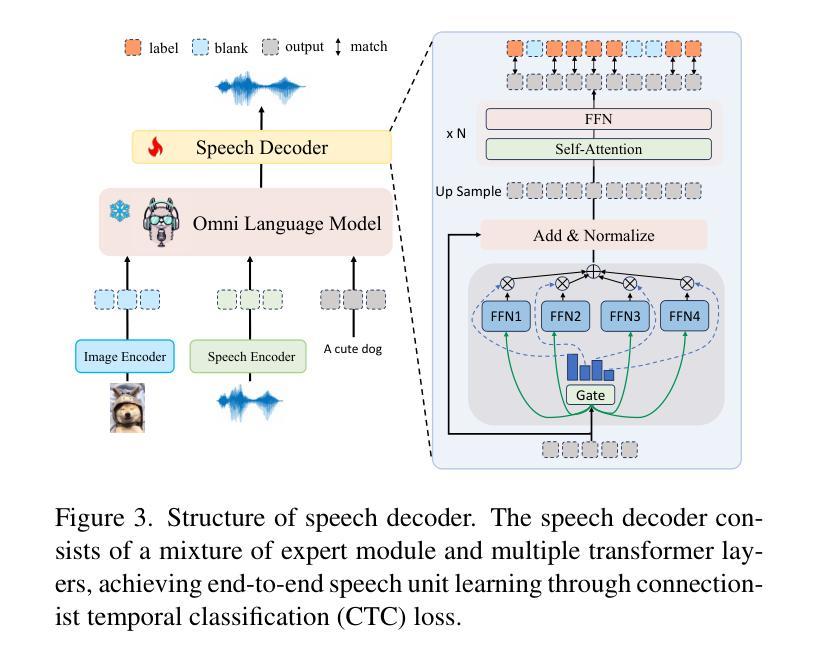
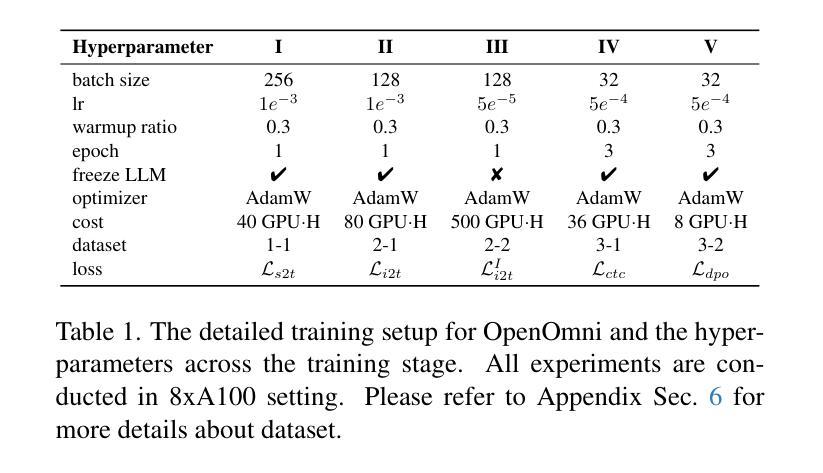
DrawSpeech: Expressive Speech Synthesis Using Prosodic Sketches as Control Conditions
Authors:Weidong Chen, Shan Yang, Guangzhi Li, Xixin Wu
Controlling text-to-speech (TTS) systems to synthesize speech with the prosodic characteristics expected by users has attracted much attention. To achieve controllability, current studies focus on two main directions: (1) using reference speech as prosody prompt to guide speech synthesis, and (2) using natural language descriptions to control the generation process. However, finding reference speech that exactly contains the prosody that users want to synthesize takes a lot of effort. Description-based guidance in TTS systems can only determine the overall prosody, which has difficulty in achieving fine-grained prosody control over the synthesized speech. In this paper, we propose DrawSpeech, a sketch-conditioned diffusion model capable of generating speech based on any prosody sketches drawn by users. Specifically, the prosody sketches are fed to DrawSpeech to provide a rough indication of the expected prosody trends. DrawSpeech then recovers the detailed pitch and energy contours based on the coarse sketches and synthesizes the desired speech. Experimental results show that DrawSpeech can generate speech with a wide variety of prosody and can precisely control the fine-grained prosody in a user-friendly manner. Our implementation and audio samples are publicly available.
控制文本到语音(TTS)系统,以合成用户期望的韵律特征的语音已引起广泛关注。为了实现控制性,当前的研究主要集中在两个主要方向:(1)使用参考语音作为韵律提示来指导语音合成;(2)使用自然语言描述来控制生成过程。然而,找到精确包含用户想要合成的韵律的参考语音需要大量的努力。TTS系统中的基于描述的指导只能确定整体的韵律,很难实现对合成语音的细粒度韵律控制。在本文中,我们提出了DrawSpeech,这是一种基于草图扩散的模型,能够基于用户绘制的任何韵律草图生成语音。具体来说,韵律草图被输入到DrawSpeech中,为用户提供预期的韵律趋势的大致指示。DrawSpeech然后根据粗略的草图恢复详细的音高和能量轮廓,并合成所需的语音。实验结果表明,DrawSpeech可以生成具有多种韵律的语音,并以用户友好的方式精确控制细粒度韵律。我们的实现和音频样本可供公众使用。
论文及项目相关链接
PDF Accepted by ICASSP 2025
Summary
文本介绍了文本转语音(TTS)系统的重要性以及目前研究中面临的挑战,即如何实现用户期望的语音韵律控制。文中提出DrawSpeech系统,该系统能够根据用户绘制的韵律草图生成语音,通过粗略的草图指示来恢复详细的音高和能量轮廓,从而合成用户期望的语音。实验结果显示,DrawSpeech系统可以生成具有各种韵律特征的语音并具有精确的细粒度韵律控制能力。此技术解决了以往寻找完全符合用户所需韵律参考语及通过自然语言描述控制语音生成过程的局限性问题。该系统具有用户友好性,其实现和音频样本已公开可用。
Key Takeaways
当前TTS系统研究主要关注如何控制语音韵律的合成过程,以符合用户的期望。主要存在两个研究方向:利用参考语音作为韵律提示和引导语音合成和利用自然语言描述来控制生成过程。但两种方法都有各自的挑战。利用参考语音难以找到完全符合用户所需韵律的参考语音;而基于自然语言描述的TTS系统只能确定整体的韵律,难以实现对合成语音的精细韵律控制。
DrawSpeech系统被提出以解决上述问题,该系统是一个基于草图扩散模型的文本转语音系统。用户可以通过绘制韵律草图来引导语音合成过程。该系统能够从用户的草图中恢复详细的音高和能量轮廓,并合成出符合用户期望的语音。实验结果显示该系统具有广泛的韵律生成能力和精确的细粒度韵律控制能力。
DrawSpeech系统提供了一种用户友好的方式来控制TTS系统的输出,使用户能够轻松地根据自己的意愿调整合成语音的韵律特征。通过绘制简单的韵律草图,用户可以直观地表达自己的意图和需求,无需复杂的自然语言描述或寻找合适的参考语音样本。
点此查看论文截图
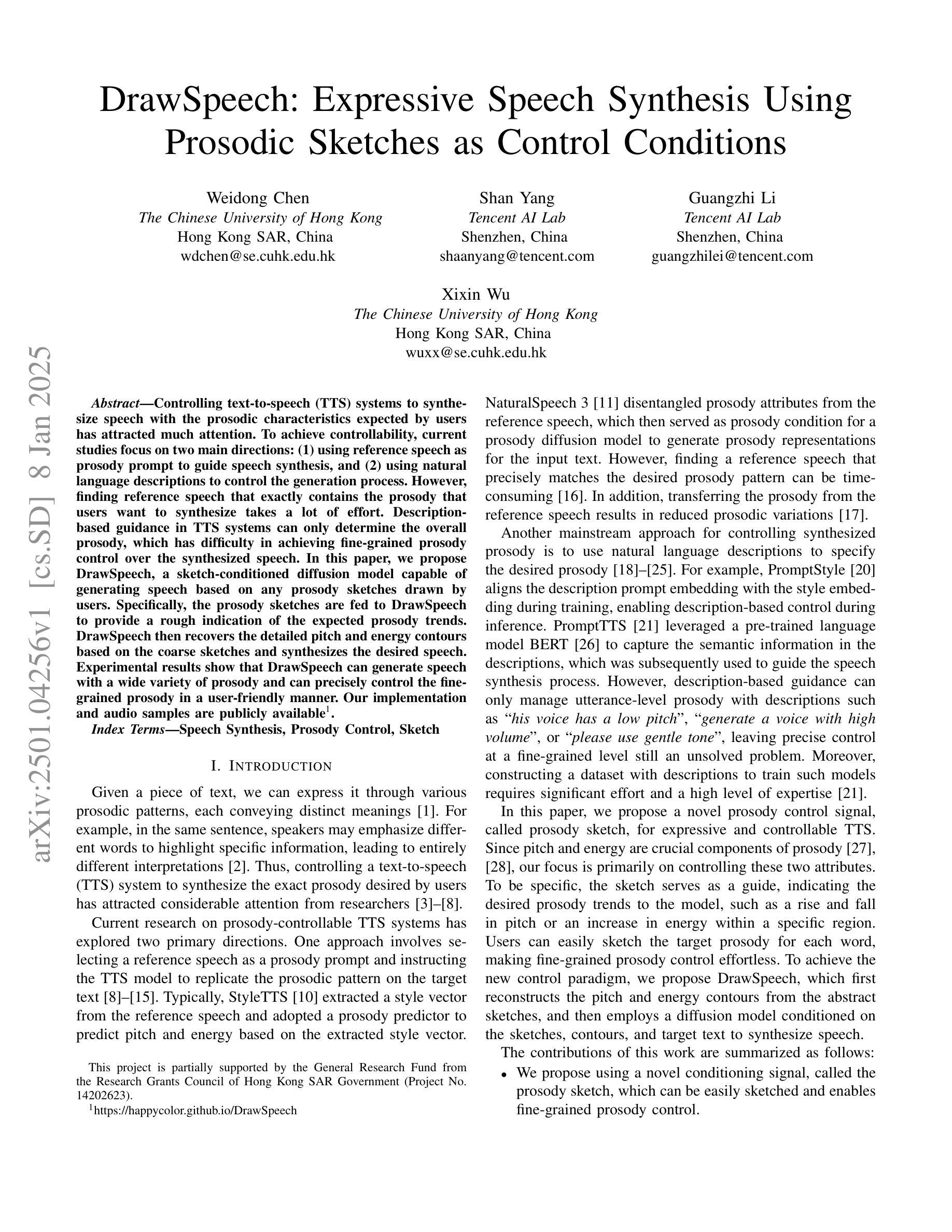
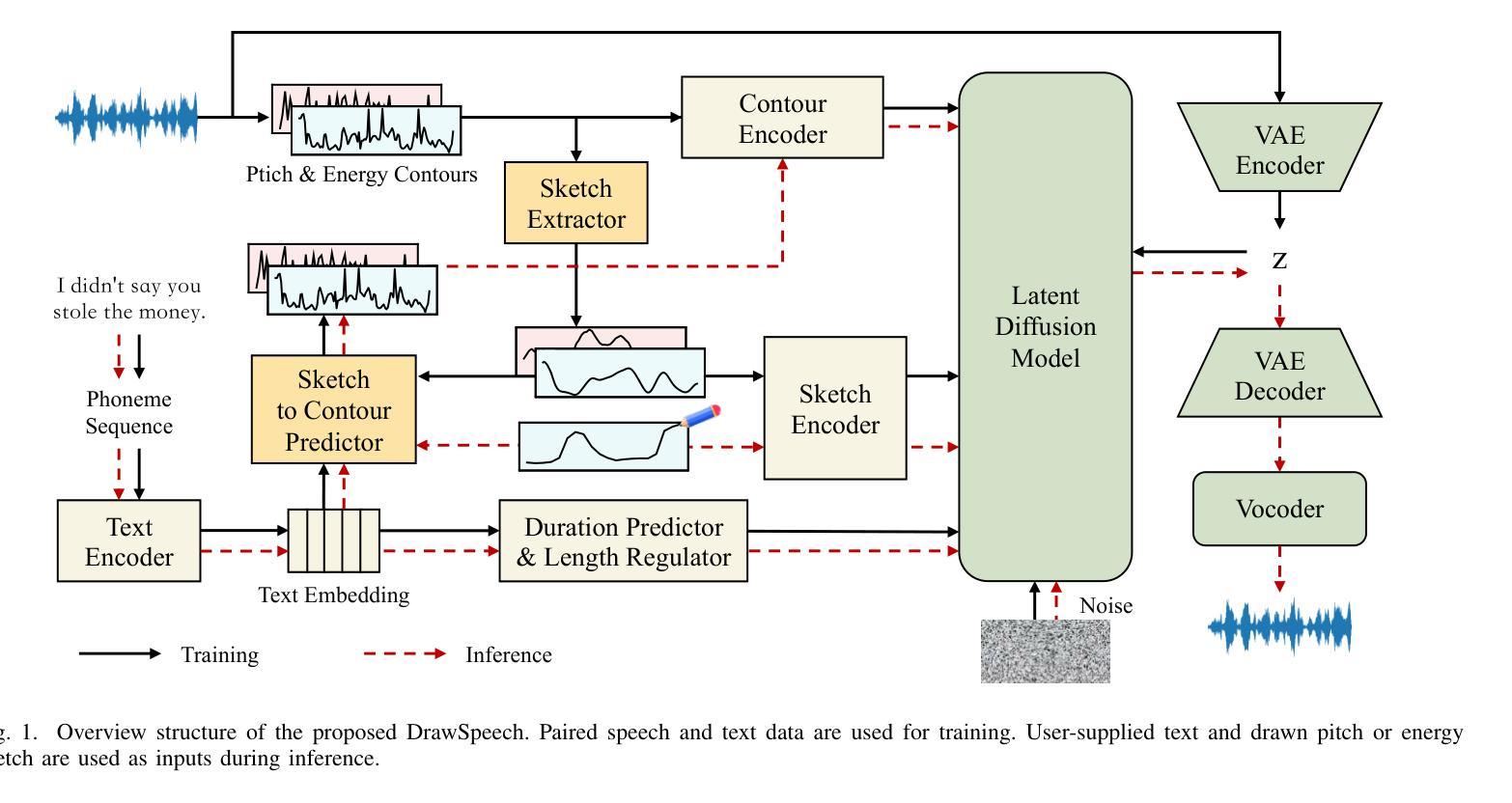
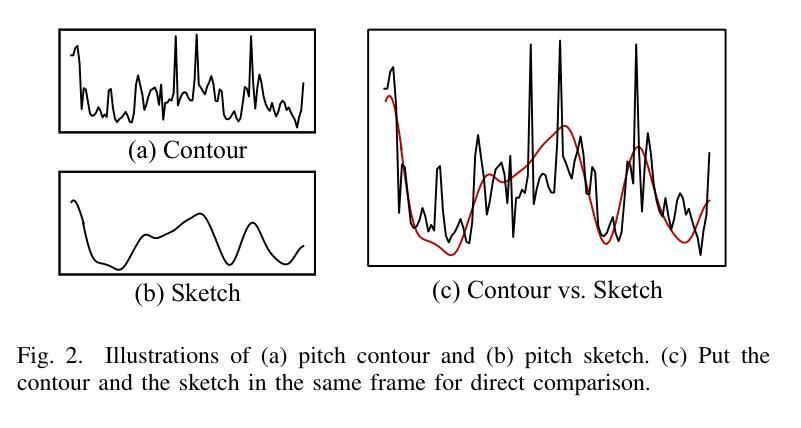
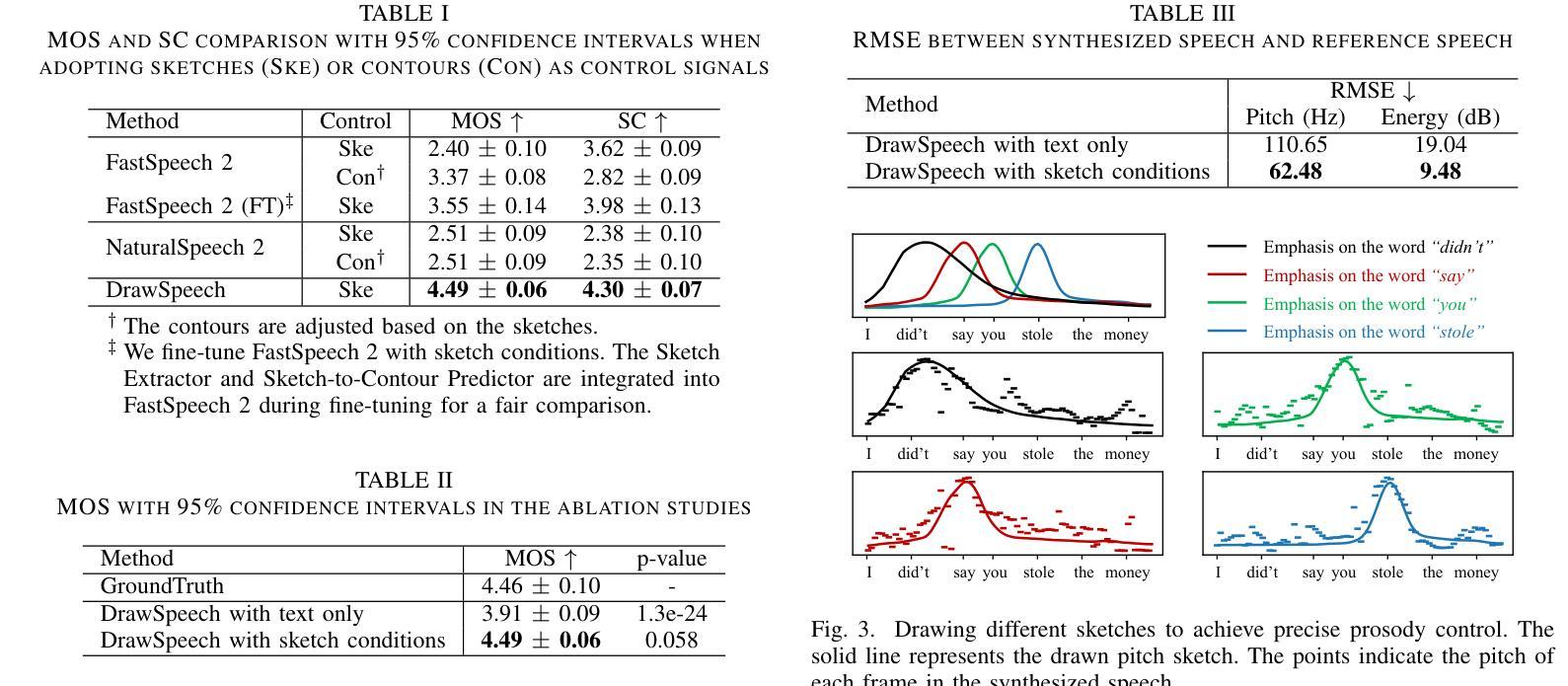
NuSTAR view of the X-ray transients Swift J174805.3-244637 and IGR J17511-3057
Authors:Aditya S. Mondal, Mahasweta Bhattacharya, Mayukh Pahari, Biplab Raychaudhuri, Rohit Ghosh, Gulab C. Dewangan
We report on the NuSTAR observations of the neutron star low-mass X-ray binary Swift J174805.3-244637 (hereafter SwiftJ17480) and the accreting millisecond X-ray pulsar IGRJ17511-3057 performed on March 4, 2023, and April 8, 2015, respectively. We describe the continuum emission of SwiftJ17480 with a combination of two soft thermal components and an additional hard X-ray emission described by a power-law. We suggest that the spectral properties of SwiftJ17480 are consistent with a soft spectral state. The source IGRJ17511-3057 exhibits a hard spectrum characterized by a Comptonized emission from the corona. The X-ray spectrum of both sources shows evidence of disc reflection. For the first time, we employ the self-consistent reflection models ({\tt relxill} and {\tt relxillNS}) to fit the reflection features in the \nustar{} spectrum. From the best-fit spectral model, we find an inner disc radius ($R_{in}$) is precisely constrained to $(1.99-2.68):R_{ISCO}$ and inclination to $30\pm 1\degree$ for SwiftJ17480. We determine an inner disc radius of $\lesssim 1.3;R_{ISCO}$ and inclination of $44\pm 3\degree$ for IGRJ17511-3057. A low inclination angle of the system is required for both sources. For the source IGRJ17511-3057, spinning at $4.1$ ms, the value of co-rotation radius ($R_{co}$) is estimated to be $\sim 42$ km ($3.6:R_{ISCO})$, consistent with the position of inner disc radius as $R_{in}\lesssim R_{co}$. We further place an upper limit on the magnetic field strength of the sources, considering the disc is truncated at the magnetospheric radius.
我们报告了使用NuSTAR对中子星低质量X射线双星Swift J174805.3-244637(以下简称Swift J17480)和增亮毫秒X射线脉冲星IGR J17511-3057于2023年3月4日和2015年4月8日的观测结果。我们用两个软热成分的组合来描述Swift J17480的连续发射,并用幂律来描述额外的硬X射线发射。我们认为Swift J17480的光谱特性与软光谱状态相符。源IGR J17511-3057表现出由日冕产生的康普顿化发射的硬光谱特性。这两个源均表现出圆盘反射的证据。我们首次采用自洽反射模型(relxill和relxillNS)来拟合NuSTAR光谱中的反射特征。根据最佳拟合光谱模型,我们精确约束了Swift J17480的内盘半径(R_{in})为(1.99-2.68)× R_{ISCO},倾角为30±1°。我们确定了IGR J17511-3057的内盘半径小于或等于约≤ R_{ISCO},倾角为44±3°。对于这两种源,系统需要一个较低的倾角。对于旋转频率为4.1毫秒的源IGR J17511-3057,协转半径(R_{co})的估计值约为~约 R_{ISCO},这与内盘半径的位置为R_{in}<R_{co}相一致。考虑到磁层半径处磁盘被截断,我们对源磁场强度设置了上限。
论文及项目相关链接
PDF 28 pages, 13 figures, 3 tables, Accepted for publication in Journal of High Energy Astrophysics (JHEAP)
Summary
本文报道了对两个天文目标NuSTAR观测结果的分析:中子星低质量X射线双星Swift J174805.3-244637和增亮的毫秒X射线脉冲星IGR J17511-3057。Swift J17480的连续发射描述为两个软热成分和一个额外的硬X射线发射的组合,表现为幂律特征。IGR J17511-3057展现出由日冕产生的硬光谱特征。两者均表现出盘反射的证据。通过自洽反射模型拟合反射特征,对Swift J17480的内盘半径和倾角进行了精确约束,而对IGR J17511-3057的这两个参数进行了估算。两者系统的倾角角均较低。对于脉冲星IGR J17511-3057,其协转半径与内盘半径相近。
Key Takeaways
- NuSTAR对两个天文目标进行了观测,分别是中子星低质量X射线双星Swift J17480和增亮的毫秒X射线脉冲星IGR J17511-3057。
- Swift J17480的连续发射包括两个软热成分和一个硬X射线发射,表现为幂律特征,与软光谱状态一致。
- IGR J17511-3057的硬光谱特征表明其源自日冕的康普顿发射。
- 两个源的X射线光谱均显示盘反射的证据。
- 通过自洽反射模型拟合反射特征,精确约束了Swift J17480的内盘半径和倾角。
- IGR J17511-3057的内盘半径小于或等于协转半径。
点此查看论文截图
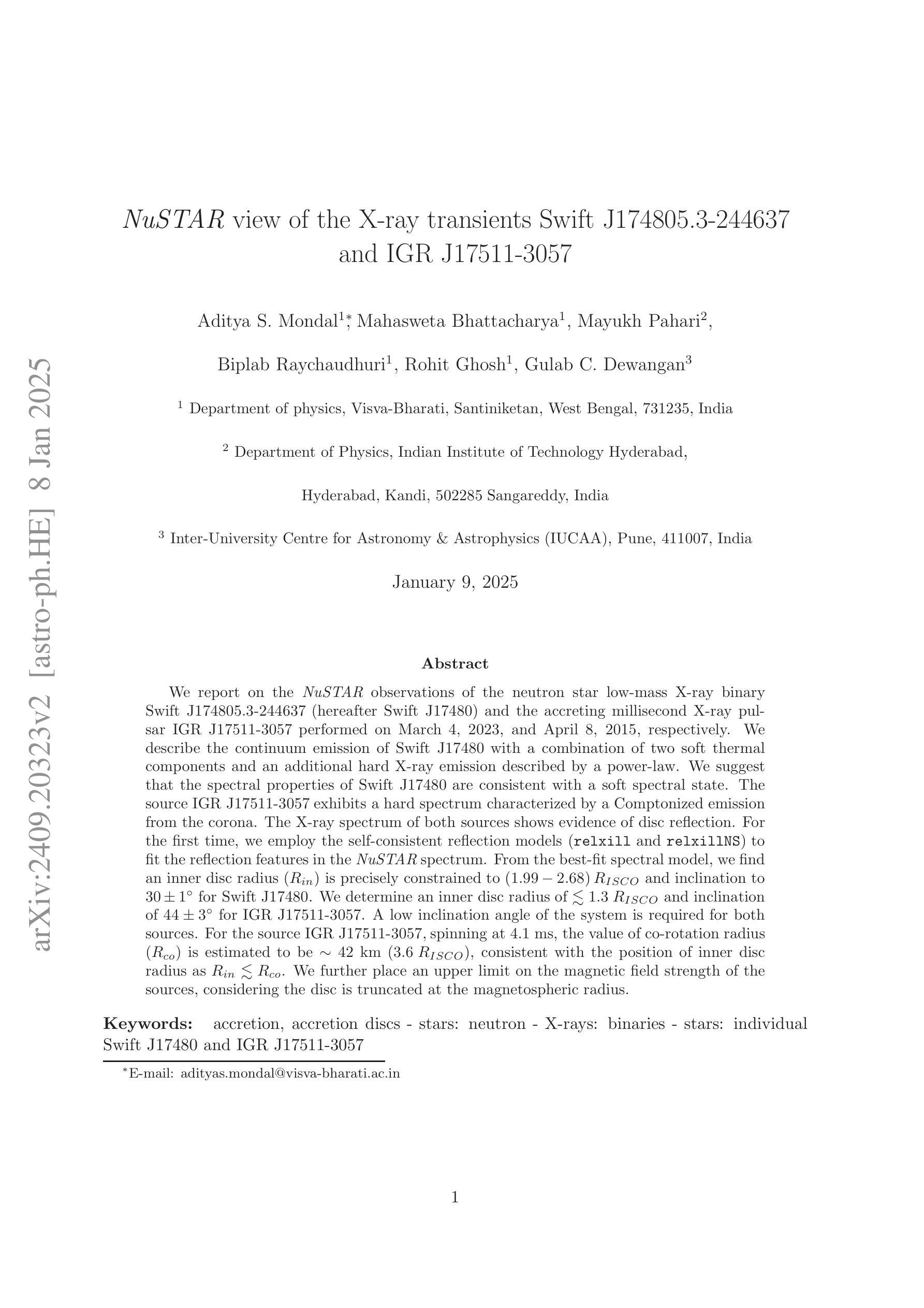
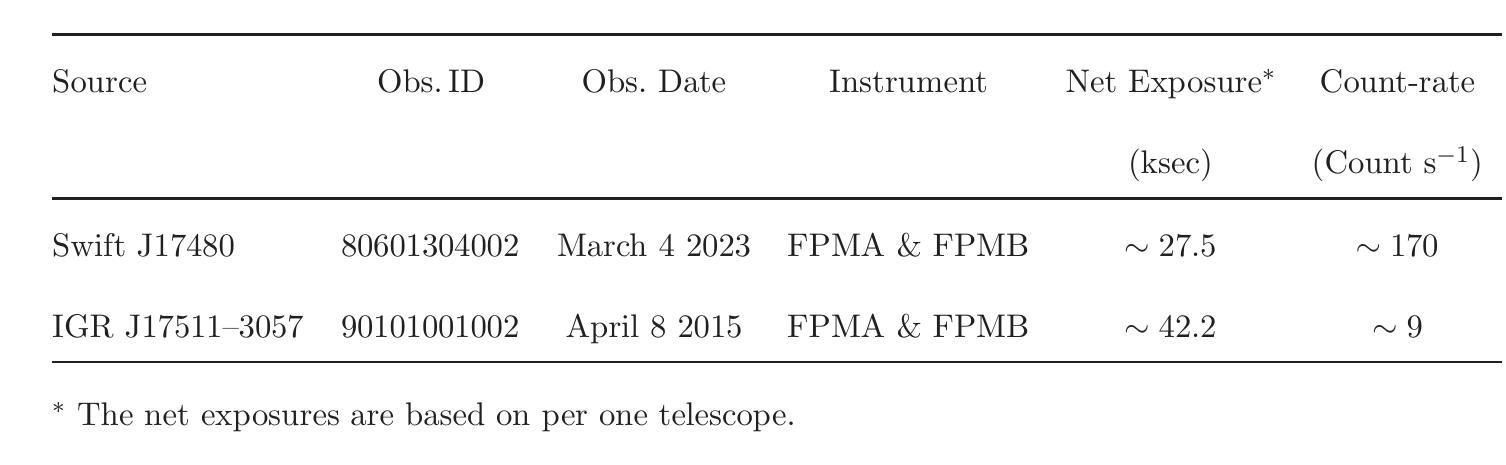
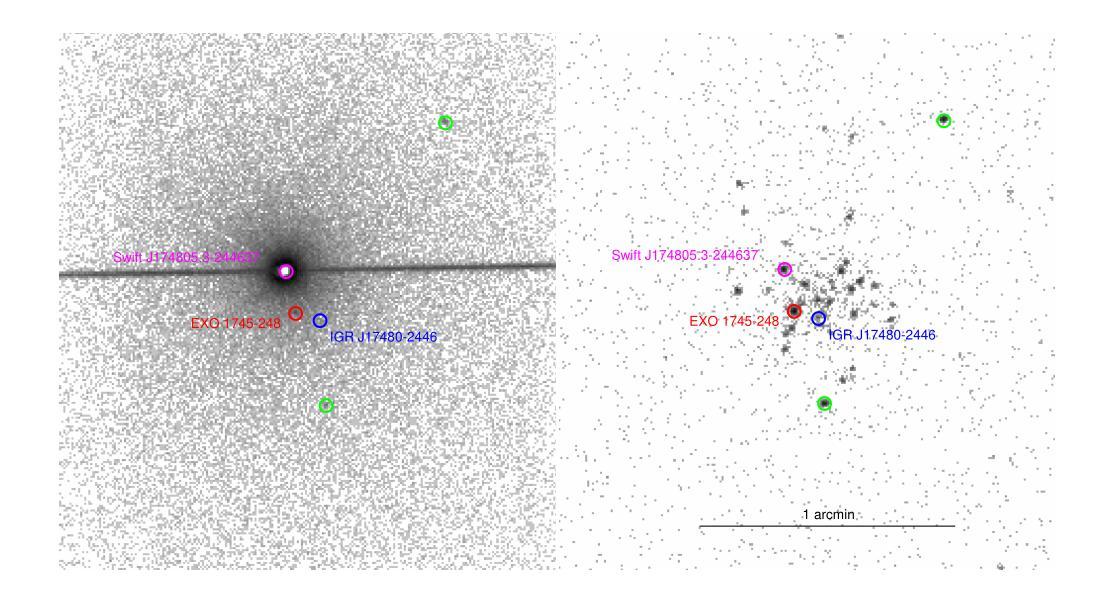
Prosody Analysis of Audiobooks
Authors:Charuta Pethe, Bach Pham, Felix D Childress, Yunting Yin, Steven Skiena
Recent advances in text-to-speech have made it possible to generate natural-sounding audio from text. However, audiobook narrations involve dramatic vocalizations and intonations by the reader, with greater reliance on emotions, dialogues, and descriptions in the narrative. Using our dataset of 93 aligned book-audiobook pairs, we present improved models for prosody prediction properties (pitch, volume, and rate of speech) from narrative text using language modeling. Our predicted prosody attributes correlate much better with human audiobook readings than results from a state-of-the-art commercial TTS system: our predicted pitch shows a higher correlation with human reading for 22 out of the 24 books, while our predicted volume attribute proves more similar to human reading for 23 out of the 24 books. Finally, we present a human evaluation study to quantify the extent that people prefer prosody-enhanced audiobook readings over commercial text-to-speech systems.
近年来,文本到语音的转换技术取得了进展,使得从文本生成自然声音的音频成为可能。然而,有声书的叙述需要读者在叙事中进行戏剧性的发音和语调变化,更依赖于情感、对话和描述。使用我们93本书与有声书对齐的数据库,我们提出了改进的语言建模模型,用于预测叙事文本中的韵律属性(音调、音量和语速)。我们的预测韵律属性与人类有声书的阅读结果相比具有更好的相关性,优于当前先进的商业文本到语音系统:在24本书中,有22本书的预测音调与人类阅读的相关性更高,有23本书的预测音量属性与人类阅读更为接近。最后,我们进行了一项人类评估研究,以量化人们喜欢韵律增强的有声书阅读程度相对于商业文本到语音系统的情况。
论文及项目相关链接
PDF Accepted to IEEE ICSC 2025
摘要
近期文本转语音技术的进展使得从文本生成自然声音的音频成为可能。然而,有声书叙述需要读者进行戏剧性的发音和语调变化,更依赖于情感、对话和叙述中的描述。我们使用93对对齐的书籍-有声书数据集,提出改进模型,利用语言建模预测叙事文本中的韵律属性(音调、音量和语速)。我们的预测韵律属性与人类有声书阅读的相关性,比目前最先进的商业TTS系统的结果要好得多:我们的预测音调与22本书中的24本的人类阅读相关性更高,而预测的音量属性与其中23本书的人类阅读更为相似。最后,我们进行了一项人类评估研究,以量化人们更喜欢韵律增强的有声书阅读而不是商业文本转语音系统。
要点
- 近期文本转语音技术使得从文本生成自然音频成为可能。
- 有声书叙述需要读者展现更多的情感和语调变化。
- 使用书籍-有声书数据集来改进模型,提高韵律属性的预测准确性。
- 改进模型包括预测音调、音量和语速等韵律属性。
- 改进模型的预测韵律属性与人类有声书阅读的相关性更高。
- 与商业TTS系统相比,改进模型在预测韵律属性方面表现更优。
点此查看论文截图
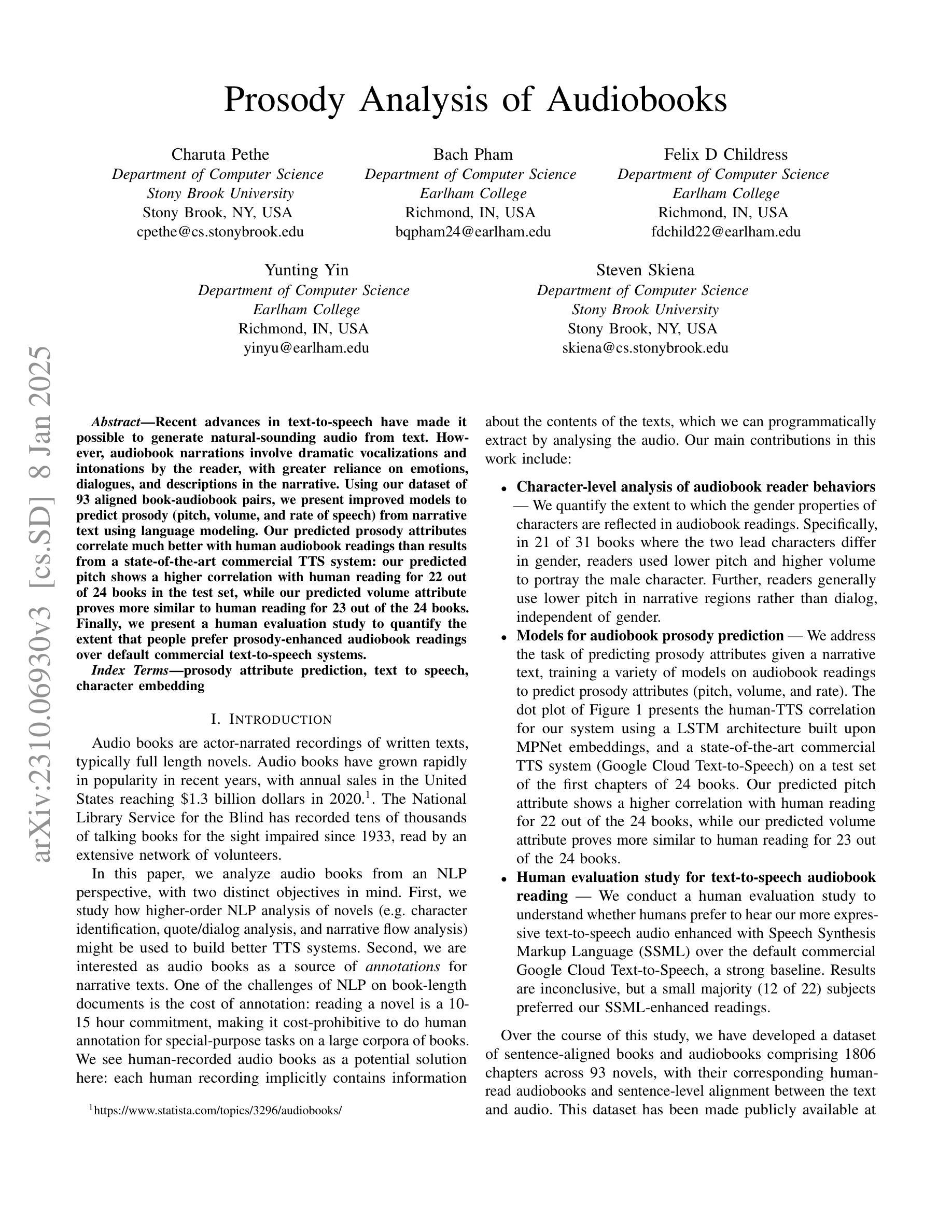
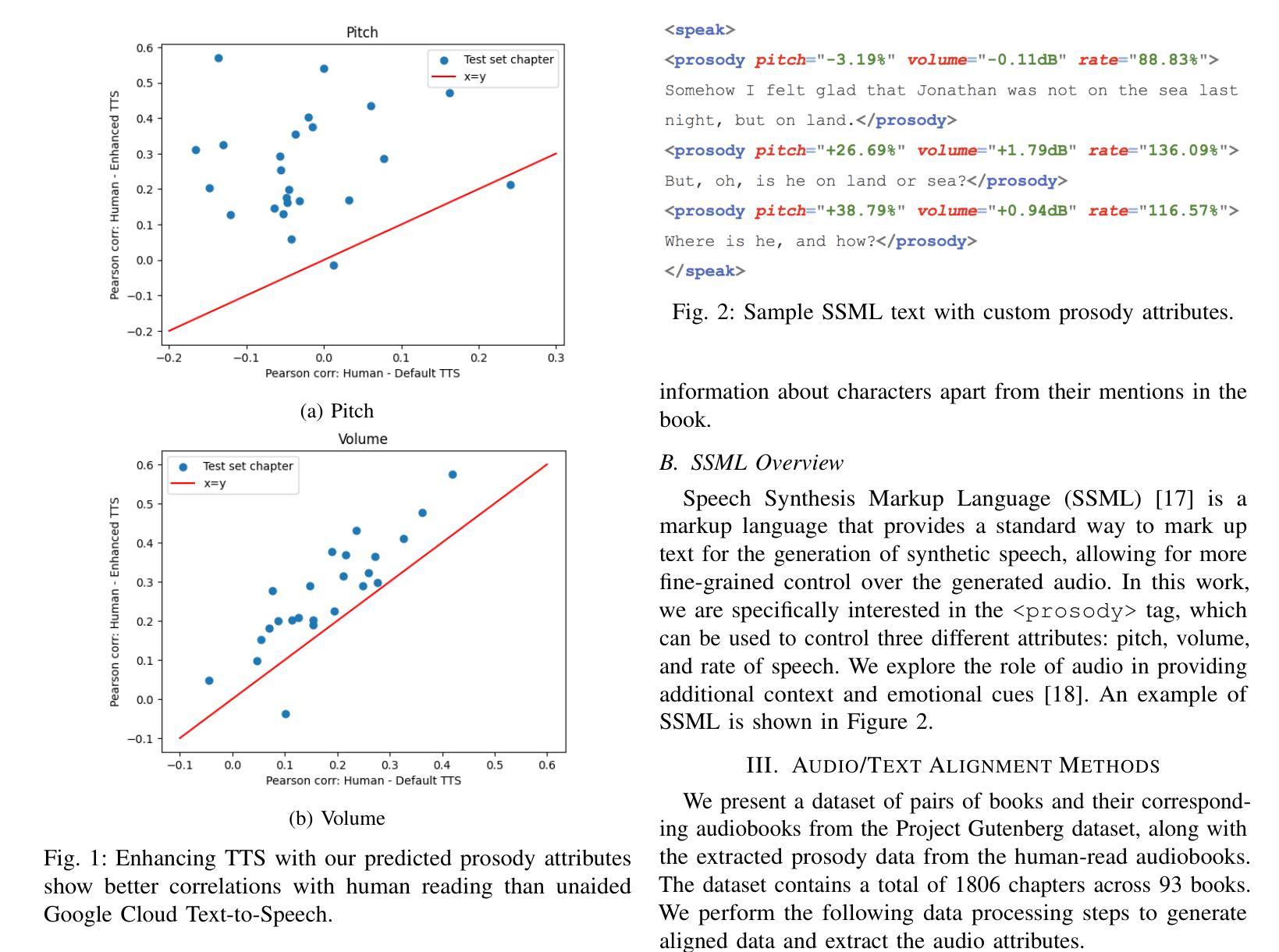
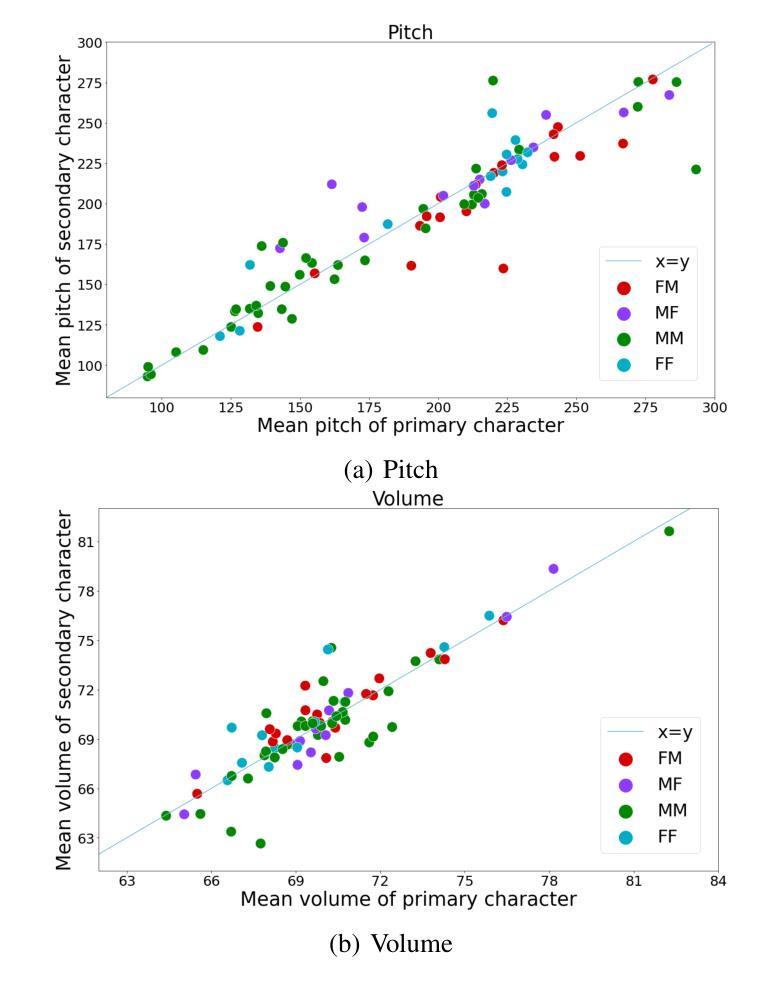
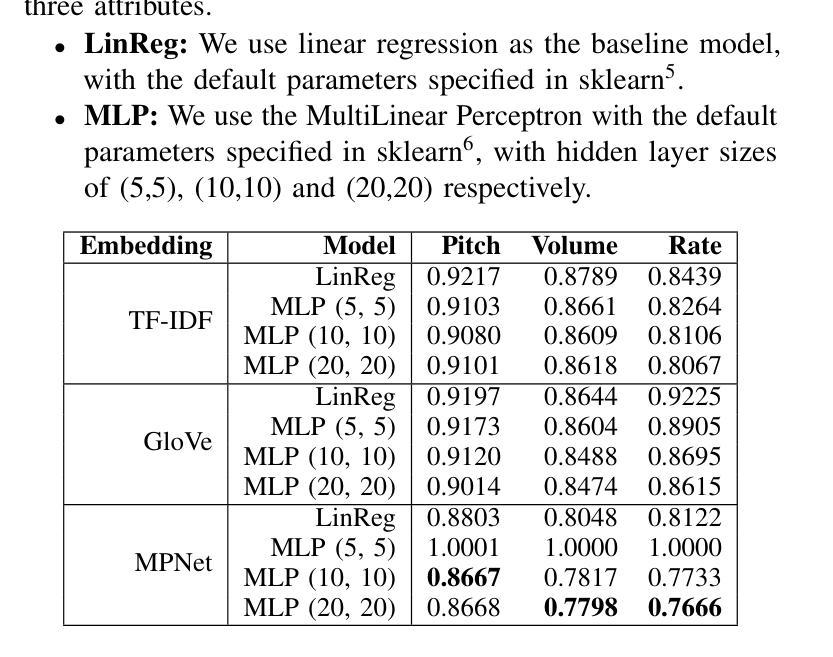
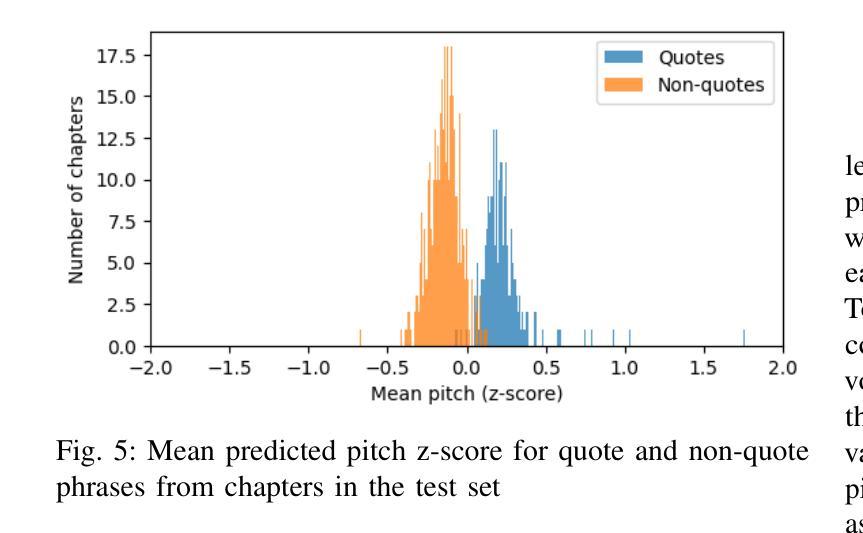
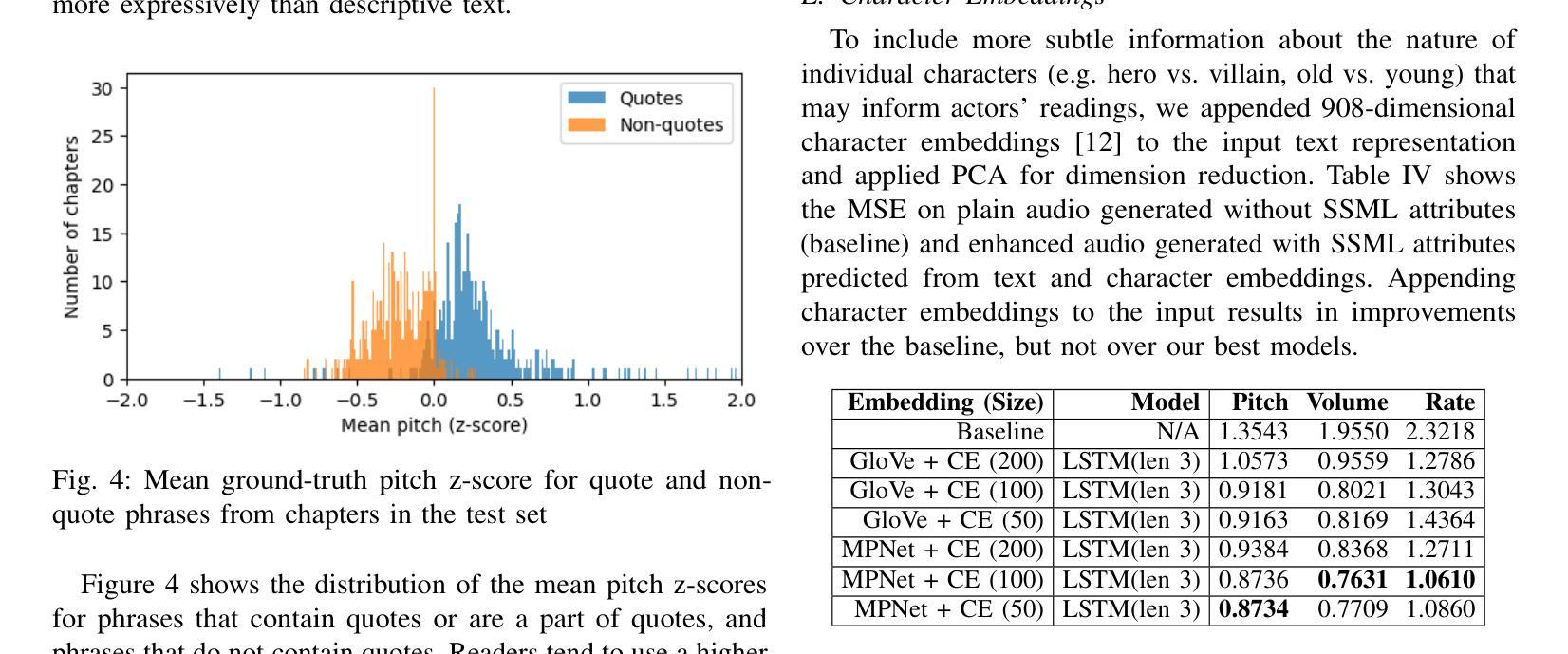
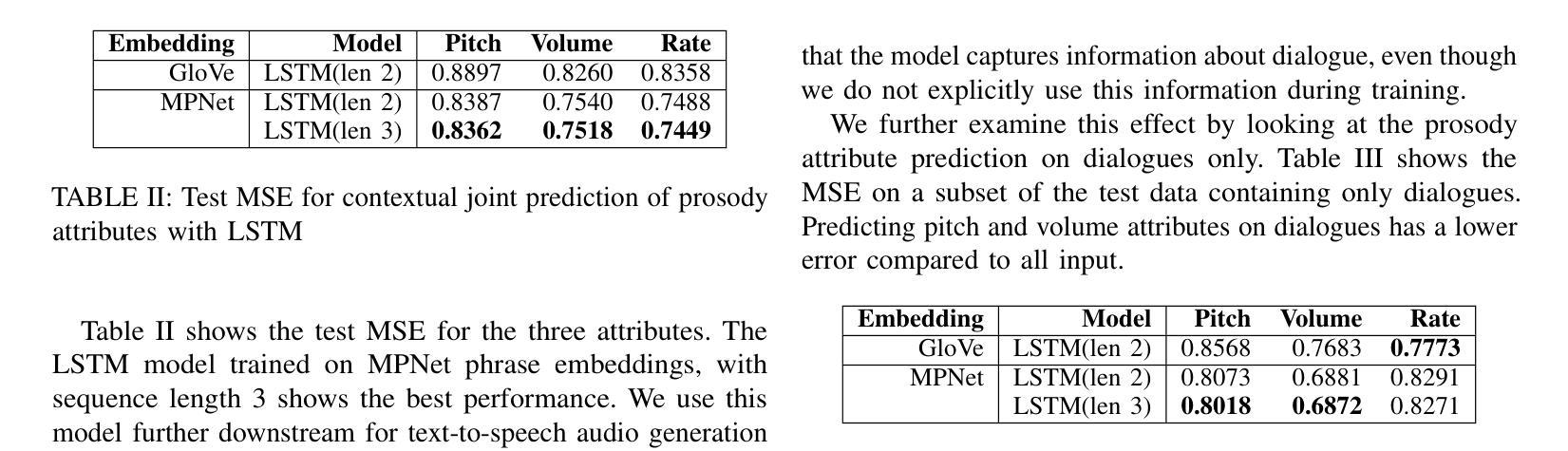
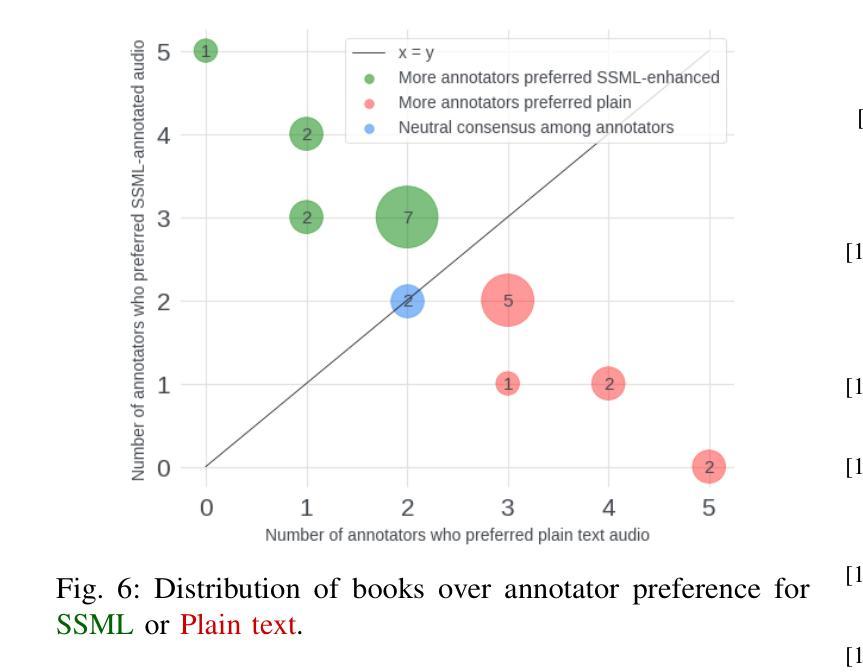