⚠️ 以下所有内容总结都来自于 大语言模型的能力,如有错误,仅供参考,谨慎使用
🔴 请注意:千万不要用于严肃的学术场景,只能用于论文阅读前的初筛!
💗 如果您觉得我们的项目对您有帮助 ChatPaperFree ,还请您给我们一些鼓励!⭐️ HuggingFace免费体验
2025-01-10 更新
Identity-Preserving Video Dubbing Using Motion Warping
Authors:Runzhen Liu, Qinjie Lin, Yunfei Liu, Lijian Lin, Ye Zhu, Yu Li, Chuhua Xian, Fa-Ting Hong
Video dubbing aims to synthesize realistic, lip-synced videos from a reference video and a driving audio signal. Although existing methods can accurately generate mouth shapes driven by audio, they often fail to preserve identity-specific features, largely because they do not effectively capture the nuanced interplay between audio cues and the visual attributes of reference identity . As a result, the generated outputs frequently lack fidelity in reproducing the unique textural and structural details of the reference identity. To address these limitations, we propose IPTalker, a novel and robust framework for video dubbing that achieves seamless alignment between driving audio and reference identity while ensuring both lip-sync accuracy and high-fidelity identity preservation. At the core of IPTalker is a transformer-based alignment mechanism designed to dynamically capture and model the correspondence between audio features and reference images, thereby enabling precise, identity-aware audio-visual integration. Building on this alignment, a motion warping strategy further refines the results by spatially deforming reference images to match the target audio-driven configuration. A dedicated refinement process then mitigates occlusion artifacts and enhances the preservation of fine-grained textures, such as mouth details and skin features. Extensive qualitative and quantitative evaluations demonstrate that IPTalker consistently outperforms existing approaches in terms of realism, lip synchronization, and identity retention, establishing a new state of the art for high-quality, identity-consistent video dubbing.
视频配音旨在从参考视频和驱动音频信号中合成逼真的、唇同步的视频。尽管现有方法能够准确地由音频驱动生成嘴巴形状,但它们往往无法保留身份特定的特征,这主要是因为它们未能有效地捕捉音频线索和参考身份视觉属性之间的微妙互动。因此,生成的结果在再现参考身份的独特纹理和结构细节方面经常缺乏保真度。为了解决这些局限性,我们提出了IPTalker,这是一种用于视频配音的新型稳健框架,它实现了驱动音频和参考身份之间的无缝对齐,同时确保唇同步精度和高保真度身份保留。IPTalker的核心是一种基于转换器的对齐机制,旨在动态捕获和建模音频特征与参考图像之间的对应关系,从而实现精确的身份感知音频视觉集成。在此基础上,通过运动扭曲策略进一步对结果进行了细化,通过空间变形参考图像以匹配目标音频驱动的配置。然后,专用的细化过程减轻了遮挡伪影,并增强了精细纹理的保留,例如嘴巴细节和皮肤特征。广泛的主观和客观评估表明,IPTalker在真实性、唇同步和身份保留方面始终优于现有方法,为高质量、身份一致的视频配音树立了新的业界标杆。
论文及项目相关链接
PDF Under Review
Summary
本文介绍了视频配音技术的新进展。针对现有方法的局限性,提出了一种新型的鲁棒框架IPTalker,实现了驱动音频与参考身份之间的无缝对齐,同时保证了唇形同步的准确性和高保真度的身份保留。其核心是基于转换器的对齐机制,能够动态捕捉和建模音频特征与参考图像之间的对应关系,从而实现精确的、具有身份意识的视听集成。在此基础上,通过运动矫正策略进一步微调结果,通过空间变形参考图像以匹配目标音频驱动的配置。同时,专门的优化过程减轻了遮挡伪影,并增强了精细纹理的保留,如口腔细节和皮肤特征。全面定性和定量评估表明,IPTalker在真实性、唇同步和身份保留方面均优于现有方法,为高质量、身份一致的视频配音树立了新的技术标杆。
Key Takeaways
- 视频配音技术旨在根据参考视频和驱动音频信号合成真实、唇形同步的视频。
- 现有方法在生成视频时难以保留身份特定特征,主要是因为它们未能有效地捕捉音频线索与视觉属性的相互作用。
- IPTalker是一种新型的鲁棒框架,旨在解决现有方法的局限性,实现驱动音频与参考身份之间的无缝对齐。
- IPTalker的核心是基于转换器的对齐机制,能够动态建模音频特征与参考图像之间的对应关系。
- IPTalker采用运动矫正策略对结果进行微调,通过空间变形参考图像以匹配目标音频配置。
- IPTalker通过专门的优化过程提高了真实感、唇同步的准确性和身份保留的效果。
点此查看论文截图
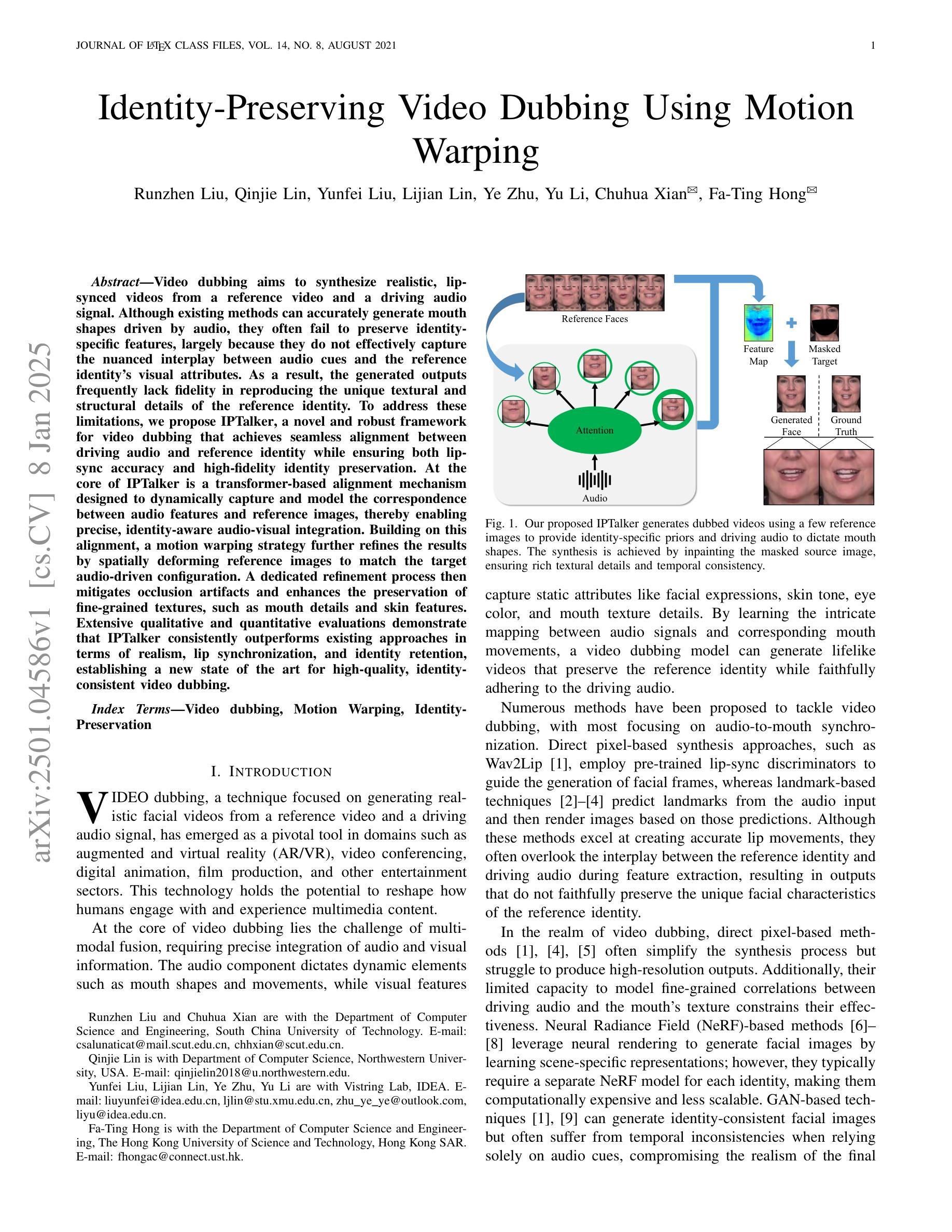
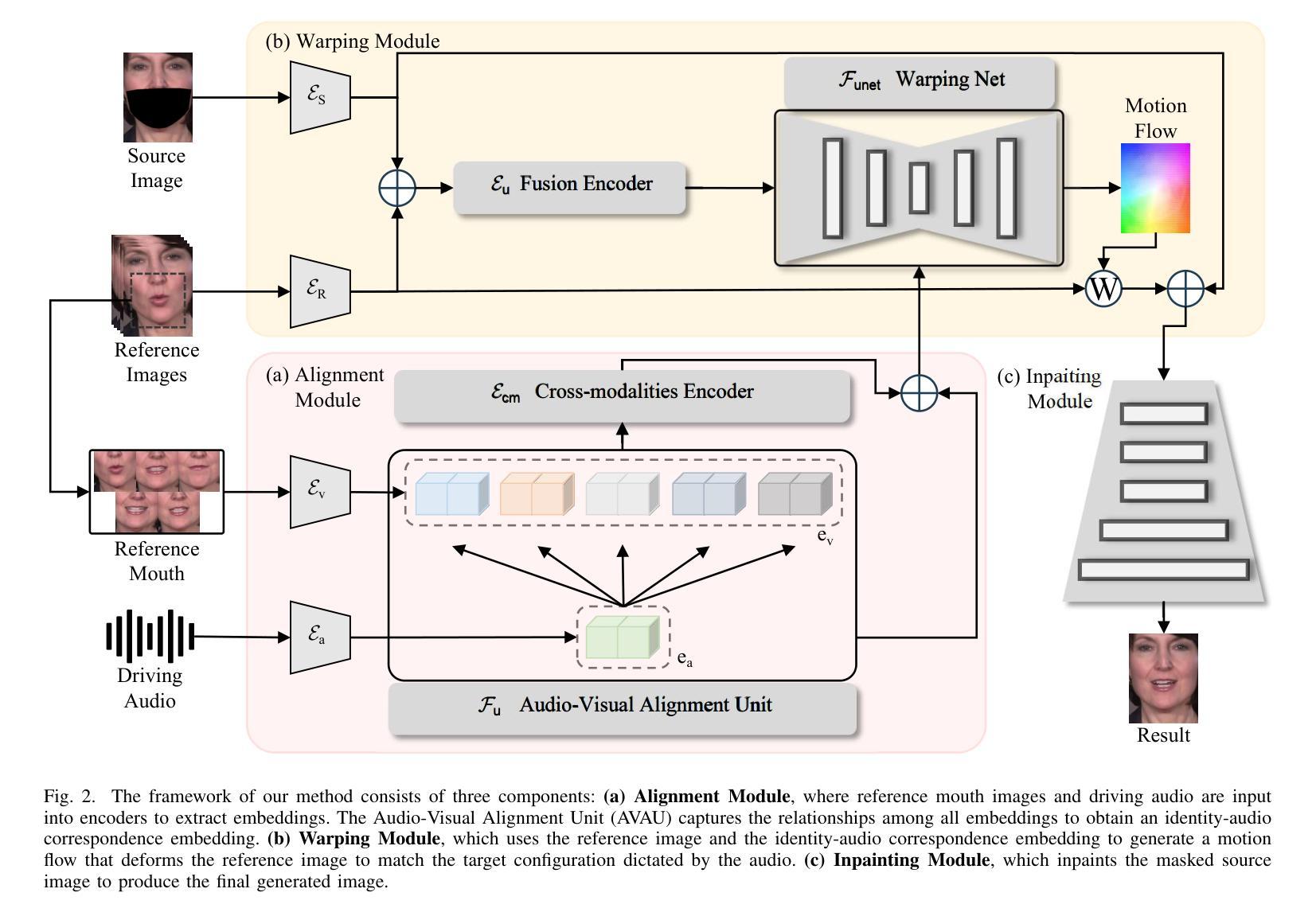
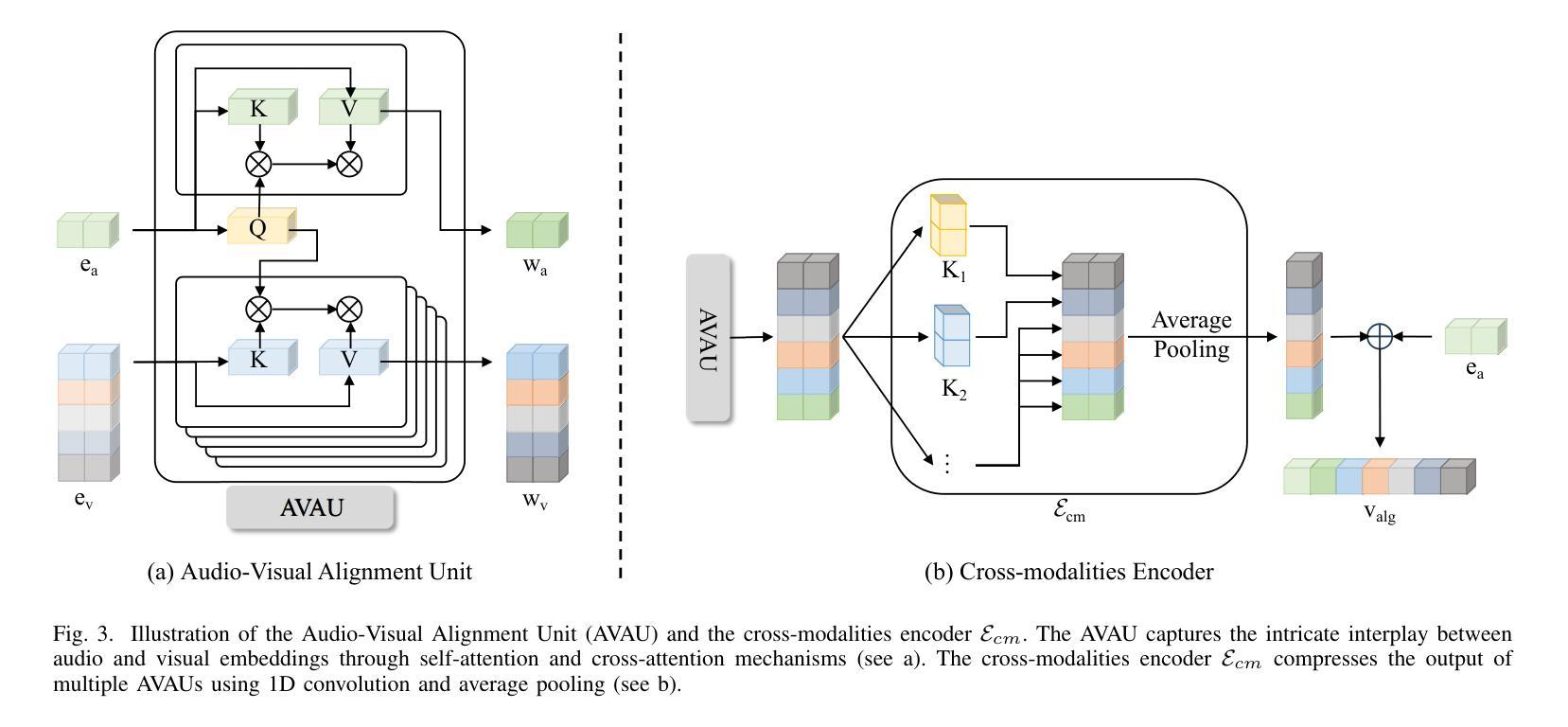
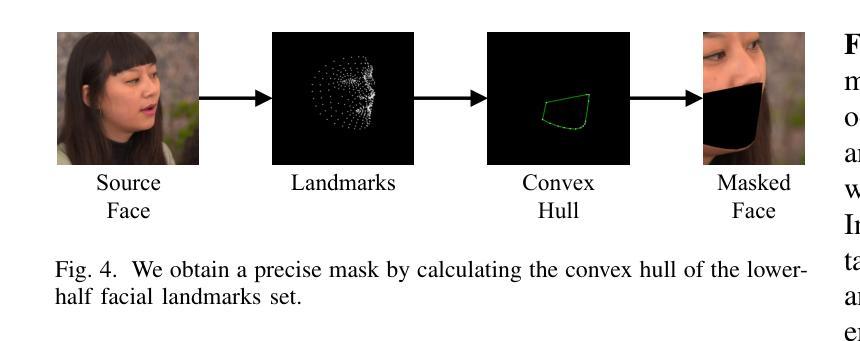
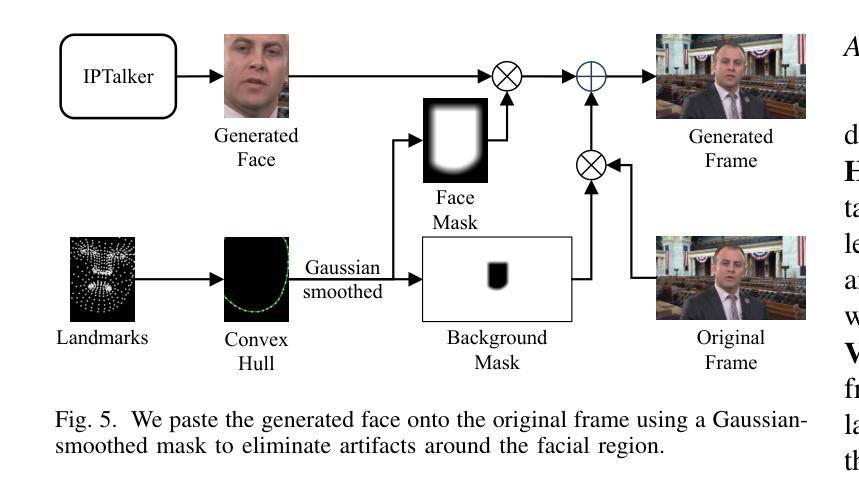
LipGen: Viseme-Guided Lip Video Generation for Enhancing Visual Speech Recognition
Authors:Bowen Hao, Dongliang Zhou, Xiaojie Li, Xingyu Zhang, Liang Xie, Jianlong Wu, Erwei Yin
Visual speech recognition (VSR), commonly known as lip reading, has garnered significant attention due to its wide-ranging practical applications. The advent of deep learning techniques and advancements in hardware capabilities have significantly enhanced the performance of lip reading models. Despite these advancements, existing datasets predominantly feature stable video recordings with limited variability in lip movements. This limitation results in models that are highly sensitive to variations encountered in real-world scenarios. To address this issue, we propose a novel framework, LipGen, which aims to improve model robustness by leveraging speech-driven synthetic visual data, thereby mitigating the constraints of current datasets. Additionally, we introduce an auxiliary task that incorporates viseme classification alongside attention mechanisms. This approach facilitates the efficient integration of temporal information, directing the model’s focus toward the relevant segments of speech, thereby enhancing discriminative capabilities. Our method demonstrates superior performance compared to the current state-of-the-art on the lip reading in the wild (LRW) dataset and exhibits even more pronounced advantages under challenging conditions.
视觉语音识别(VSR),也称为唇读,因其广泛的实际应用而备受关注。深度学习技术的出现和硬件能力的进步显著提高了唇读模型的性能。尽管有这些进展,现有数据集主要以稳定的视频录制为主,唇动变化有限。这一局限性导致模型对现实世界场景中的变化高度敏感。为了解决这一问题,我们提出了一种新型框架LipGen,旨在利用语音驱动合成视觉数据来提高模型的稳健性,从而缓解当前数据集的约束。此外,我们引入了一个辅助任务,结合语音分类和注意力机制。这种方法有助于有效地结合时间信息,引导模型关注语音的相关片段,从而提高辨别能力。我们的方法在唇读野生(LRW)数据集上展现了超越当前最前沿的性能,并在具有挑战的条件下表现出更为明显的优势。
论文及项目相关链接
PDF This paper has been accepted for presentation at ICASSP 2025
Summary
本文介绍了视觉语音识别(VSR)领域的研究进展和挑战。虽然深度学习技术和硬件能力的提高推动了该领域的发展,但现有数据集主要关注稳定视频录制,唇动变化有限,导致模型对真实场景中的变化敏感性不足。为此,提出了一种新型框架LipGen,通过利用语音驱动合成视觉数据来提高模型稳健性,并引入辅助任务和注意力机制,以提高模型的判别能力和对关键信息的捕捉能力。该方法在唇阅读领域的公开数据集上表现出卓越性能,并在具有挑战性的条件下展现出更明显的优势。
Key Takeaways
- 视觉语音识别(VSR)受到广泛关注,因其实用性强。
- 现有数据集主要关注稳定视频录制,缺乏真实场景中的变化多样性。
- LipGen框架旨在提高模型稳健性,利用语音驱动合成视觉数据来弥补现有数据集的不足。
- 引入辅助任务进行唇音分类,提高模型判别能力。
- 注意力机制有助于模型捕捉关键信息,提高效率。
- 方法在唇阅读公开数据集上表现优异。
点此查看论文截图
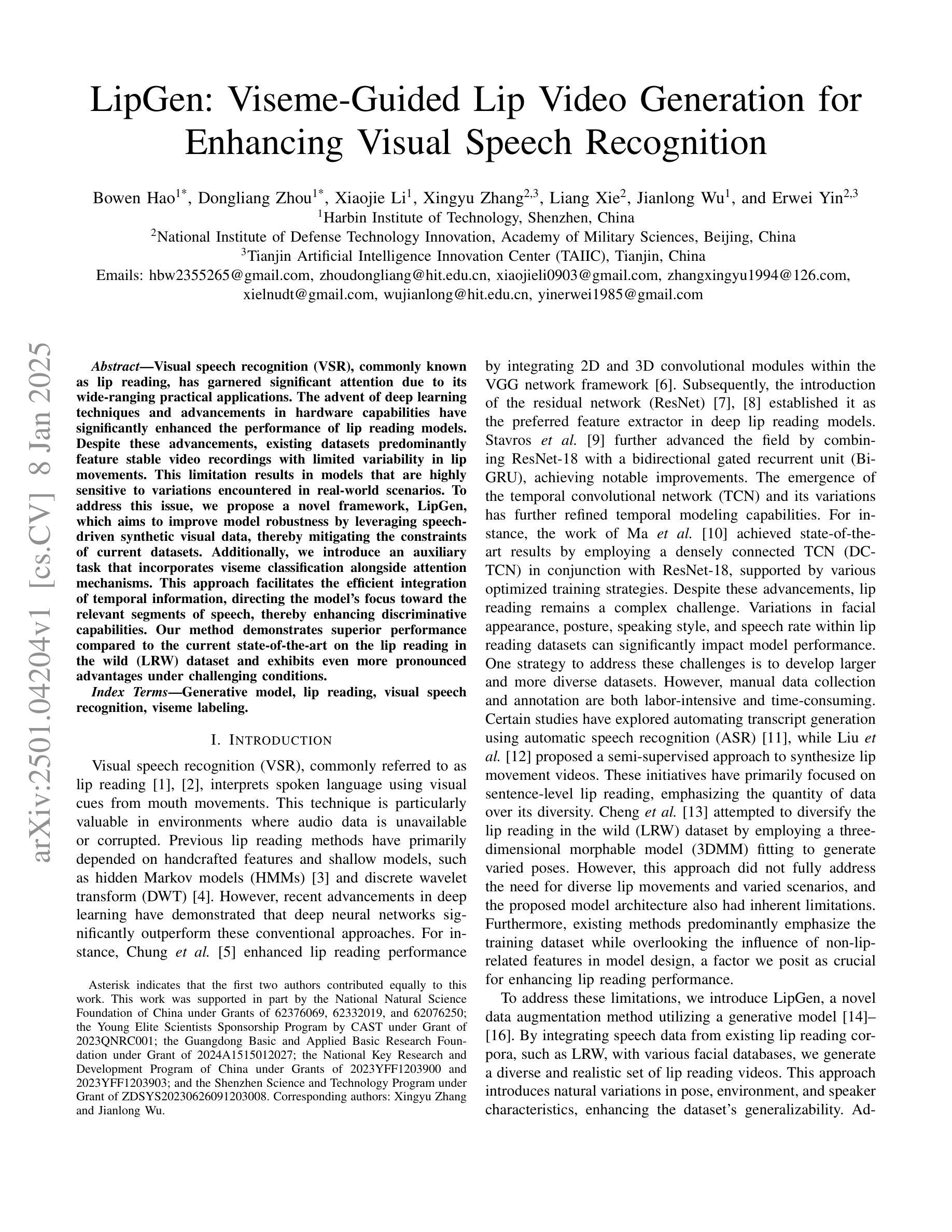
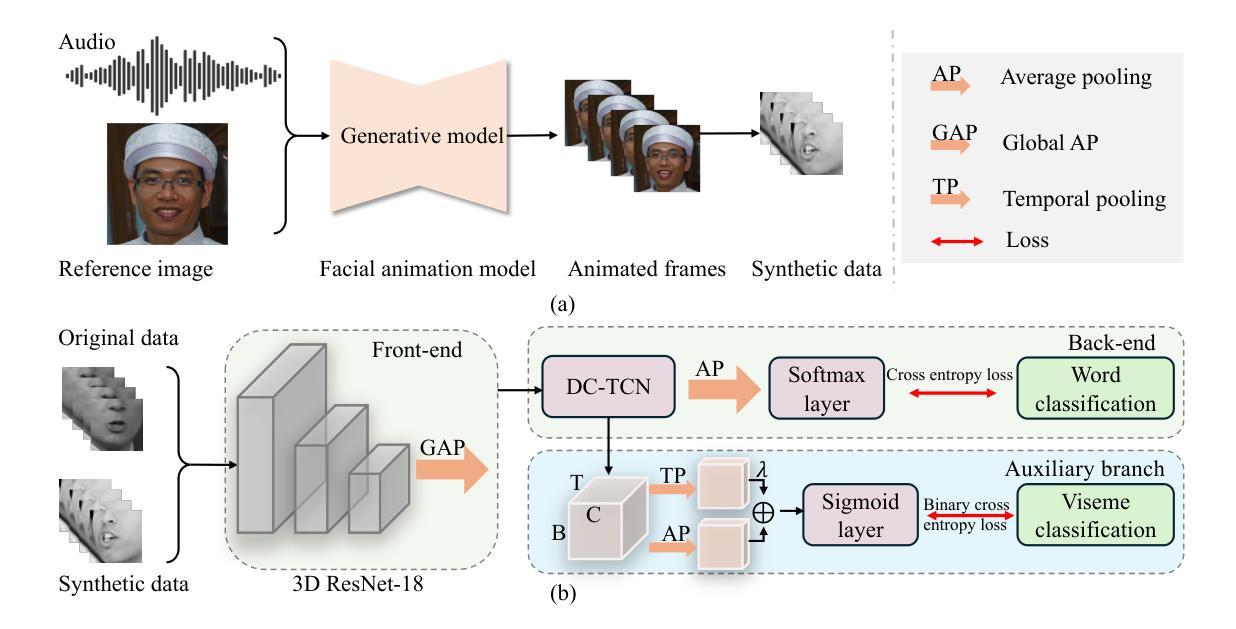
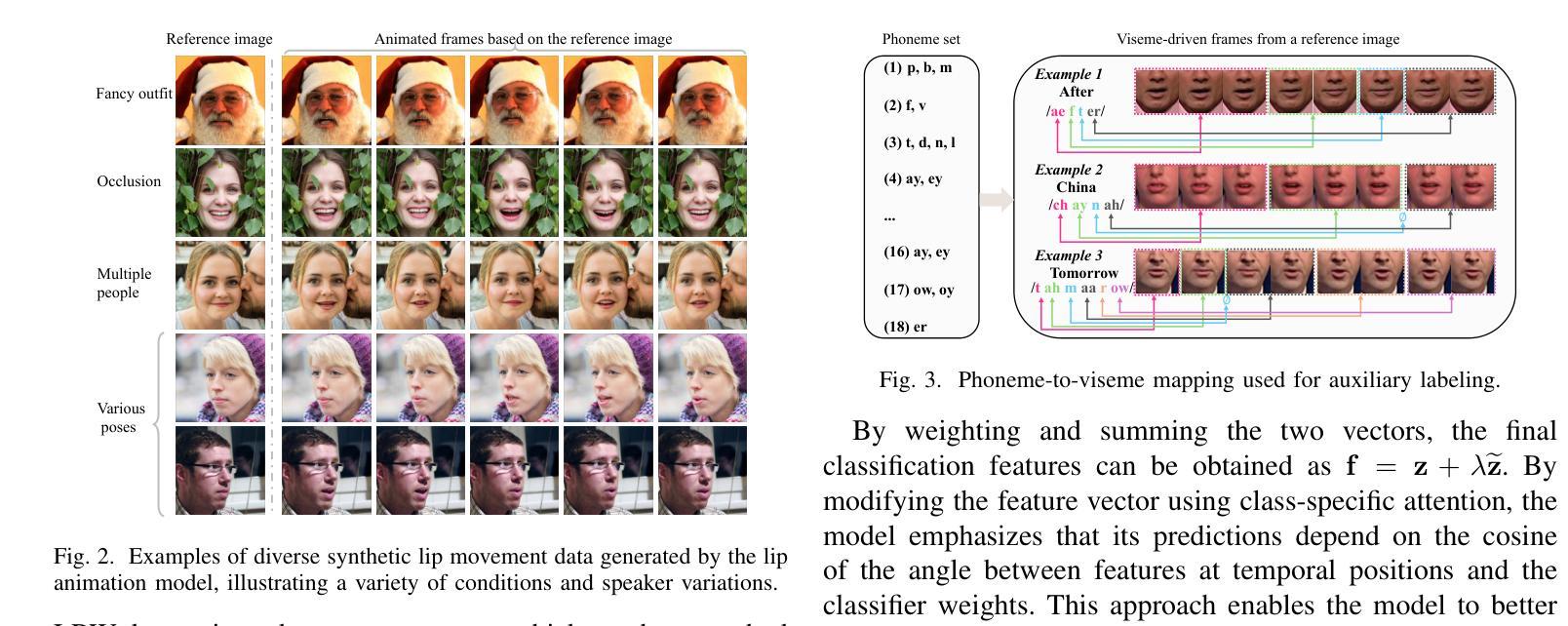
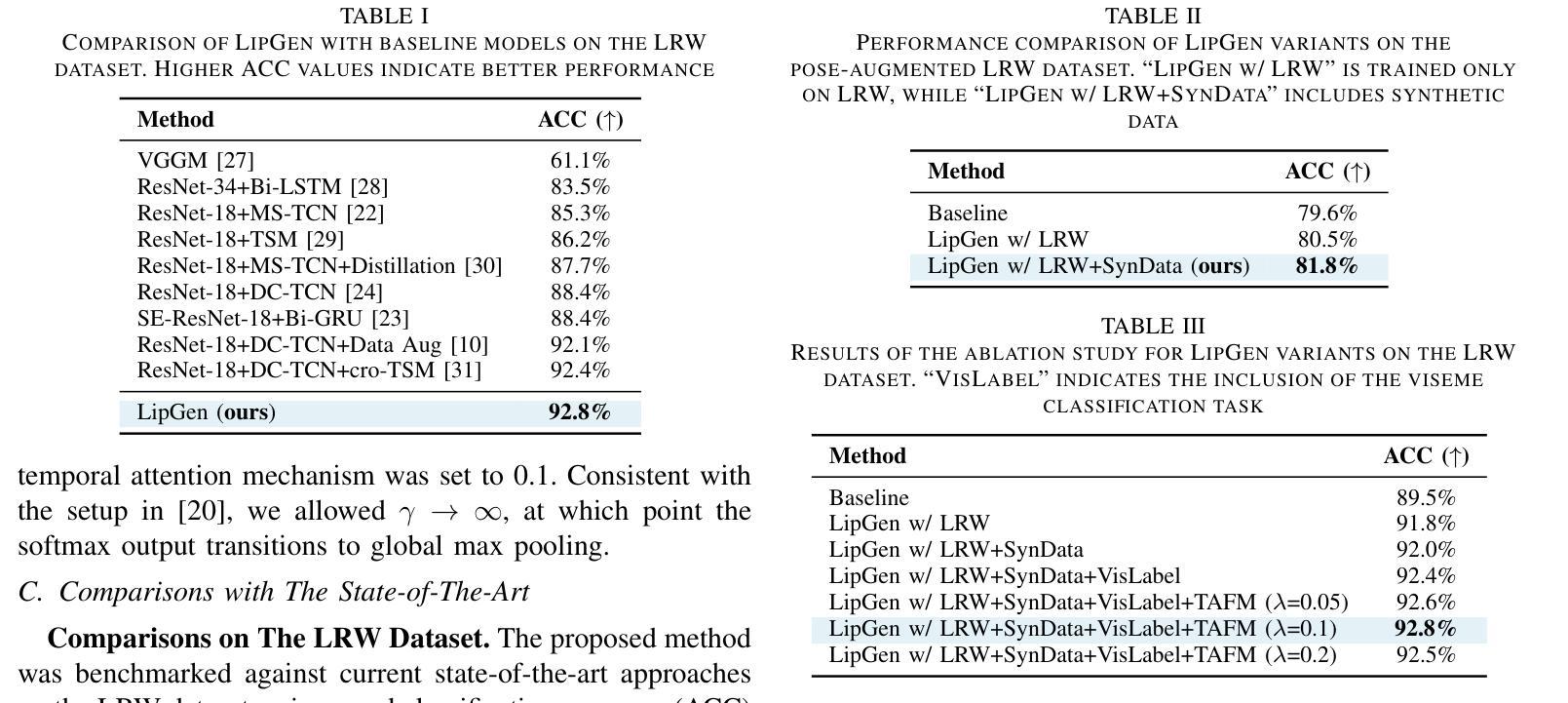