⚠️ 以下所有内容总结都来自于 大语言模型的能力,如有错误,仅供参考,谨慎使用
🔴 请注意:千万不要用于严肃的学术场景,只能用于论文阅读前的初筛!
💗 如果您觉得我们的项目对您有帮助 ChatPaperFree ,还请您给我们一些鼓励!⭐️ HuggingFace免费体验
2025-01-16 更新
RMAvatar: Photorealistic Human Avatar Reconstruction from Monocular Video Based on Rectified Mesh-embedded Gaussians
Authors:Sen Peng, Weixing Xie, Zilong Wang, Xiaohu Guo, Zhonggui Chen, Baorong Yang, Xiao Dong
We introduce RMAvatar, a novel human avatar representation with Gaussian splatting embedded on mesh to learn clothed avatar from a monocular video. We utilize the explicit mesh geometry to represent motion and shape of a virtual human and implicit appearance rendering with Gaussian Splatting. Our method consists of two main modules: Gaussian initialization module and Gaussian rectification module. We embed Gaussians into triangular faces and control their motion through the mesh, which ensures low-frequency motion and surface deformation of the avatar. Due to the limitations of LBS formula, the human skeleton is hard to control complex non-rigid transformations. We then design a pose-related Gaussian rectification module to learn fine-detailed non-rigid deformations, further improving the realism and expressiveness of the avatar. We conduct extensive experiments on public datasets, RMAvatar shows state-of-the-art performance on both rendering quality and quantitative evaluations. Please see our project page at https://rm-avatar.github.io.
我们介绍了RMAvatar,这是一种新型的人形化身表示方法,通过在网格上嵌入高斯涂抹技术来学习单目视频中的穿衣化身。我们利用明确的网格几何来表示虚拟人的运动和形状,以及使用高斯涂抹的隐式外观渲染。我们的方法主要包括两个模块:高斯初始化模块和高斯校正模块。我们将高斯嵌入三角形面部,并通过网格控制其运动,这确保了化身的低频运动和表面变形。由于LBS公式的局限性,人体骨骼难以控制复杂的非刚性变换。然后,我们设计了一个与姿势相关的高斯校正模块来学习精细的非刚性变形,进一步提高化身的真实感和表现力。我们在公共数据集上进行了大量实验,RMAvatar在渲染质量和定量评估方面都达到了最新技术水平。请访问我们的项目页面https://rm-avatar.github.io了解更多信息。
论文及项目相关链接
PDF CVM2025
Summary
RMAvatar是一种新型人类角色表示方法,采用高斯溅射技术嵌入网格学习单目视频中的角色模型。该方法利用显式网格几何表示角色的运动和形状,并使用高斯溅射技术进行隐性外观渲染。方法主要包括两个模块:高斯初始化模块和高斯校正模块。通过将高斯嵌入三角形面部并通过网格控制其运动,确保角色模型的低频运动和表面变形。由于LBS公式的局限性,人类骨骼难以控制复杂的非刚性变换。因此,设计了一个与姿势相关的高斯校正模块来学习精细的非刚性变形,进一步提高角色的真实感和表现力。在公共数据集上的实验表明,RMAvatar在渲染质量和定量评估方面均达到最佳性能。
Key Takeaways
- RMAvatar是一种基于高斯溅射技术和网格学习的人类角色表示方法。
- 该方法利用显式网格几何表示角色的运动和形状。
- RMAvatar通过嵌入高斯到三角形面部,确保角色模型的低频运动和表面变形。
- 由于LBS公式的局限性,设计了一个高斯校正模块来学习精细的非刚性变形。
- RMAvatar在公共数据集上实现了高质量的渲染和评估。
- RMAvatar项目详细信息可访问其项目页面查看。
点此查看论文截图
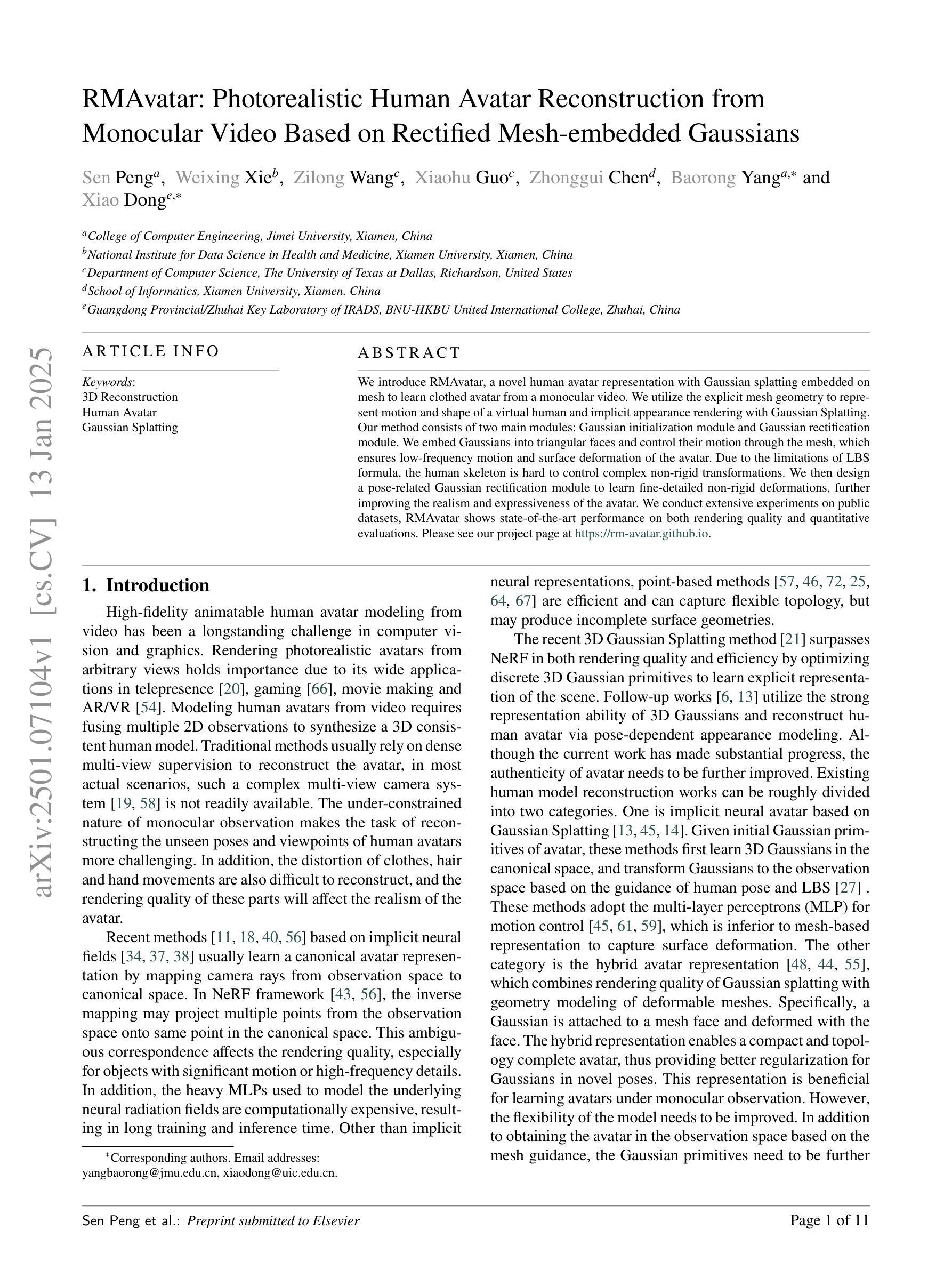
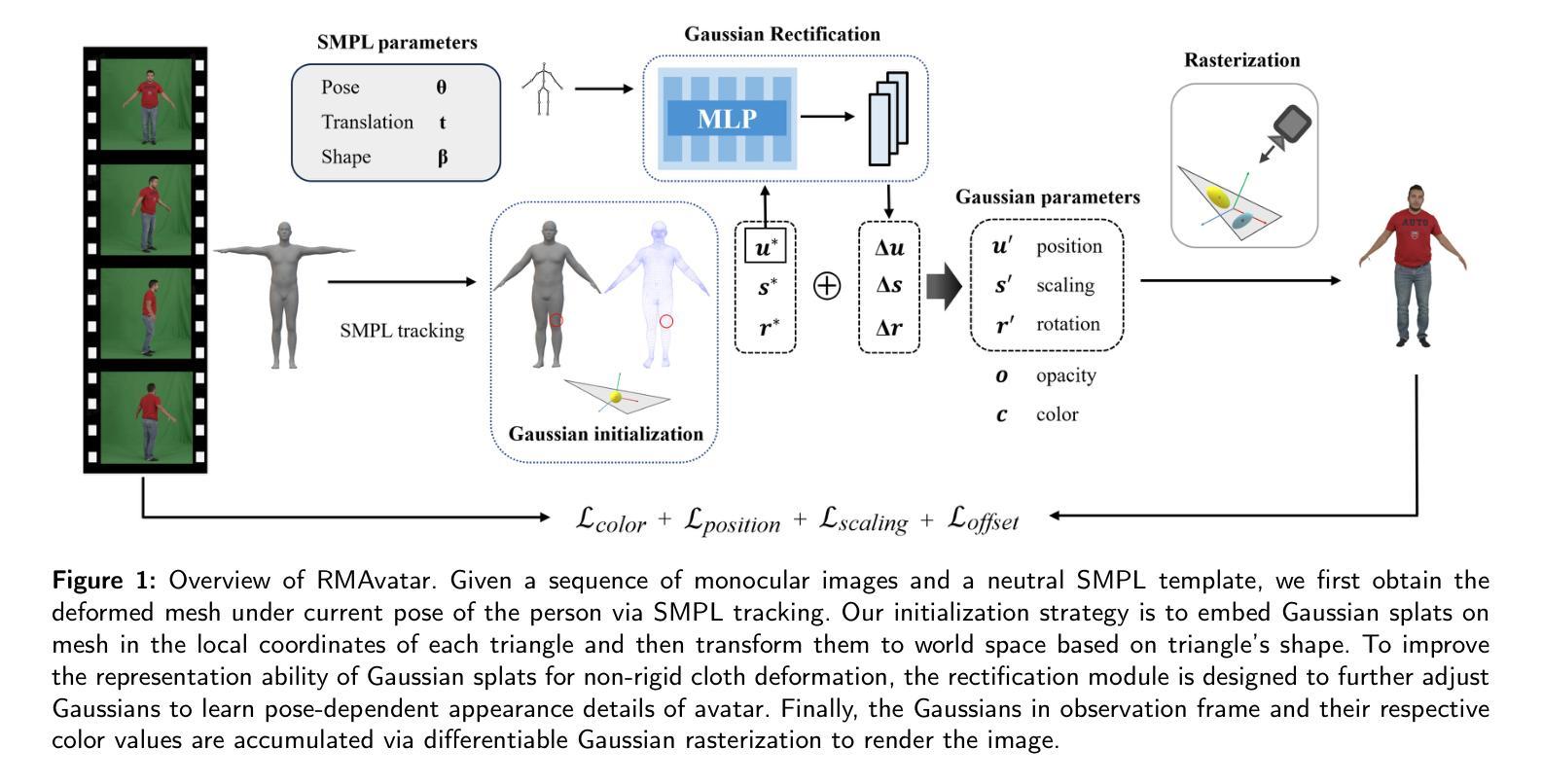
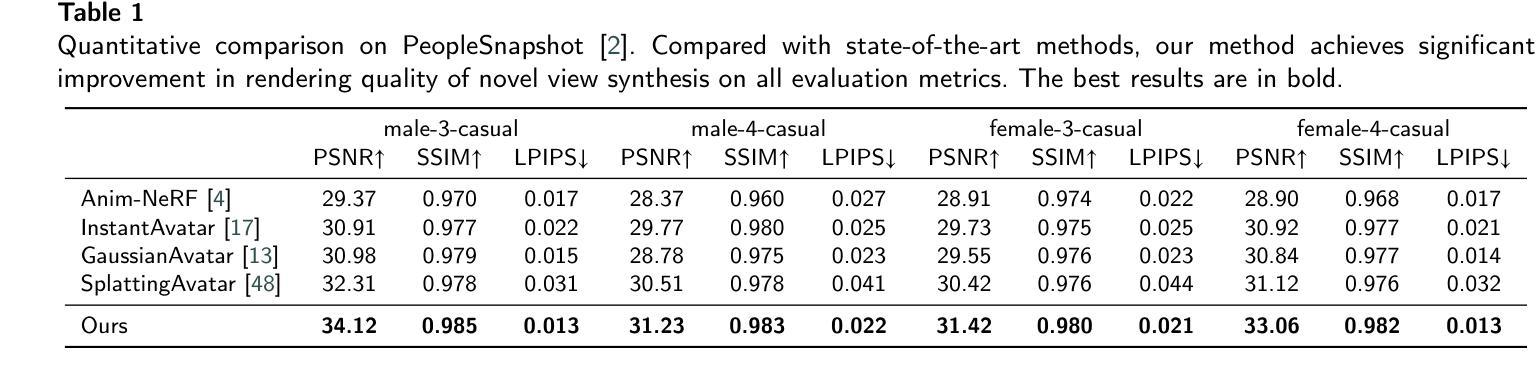

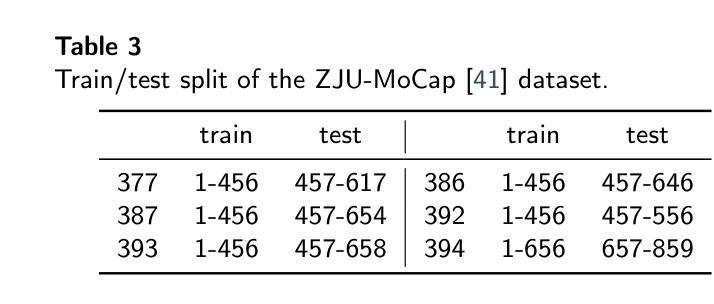
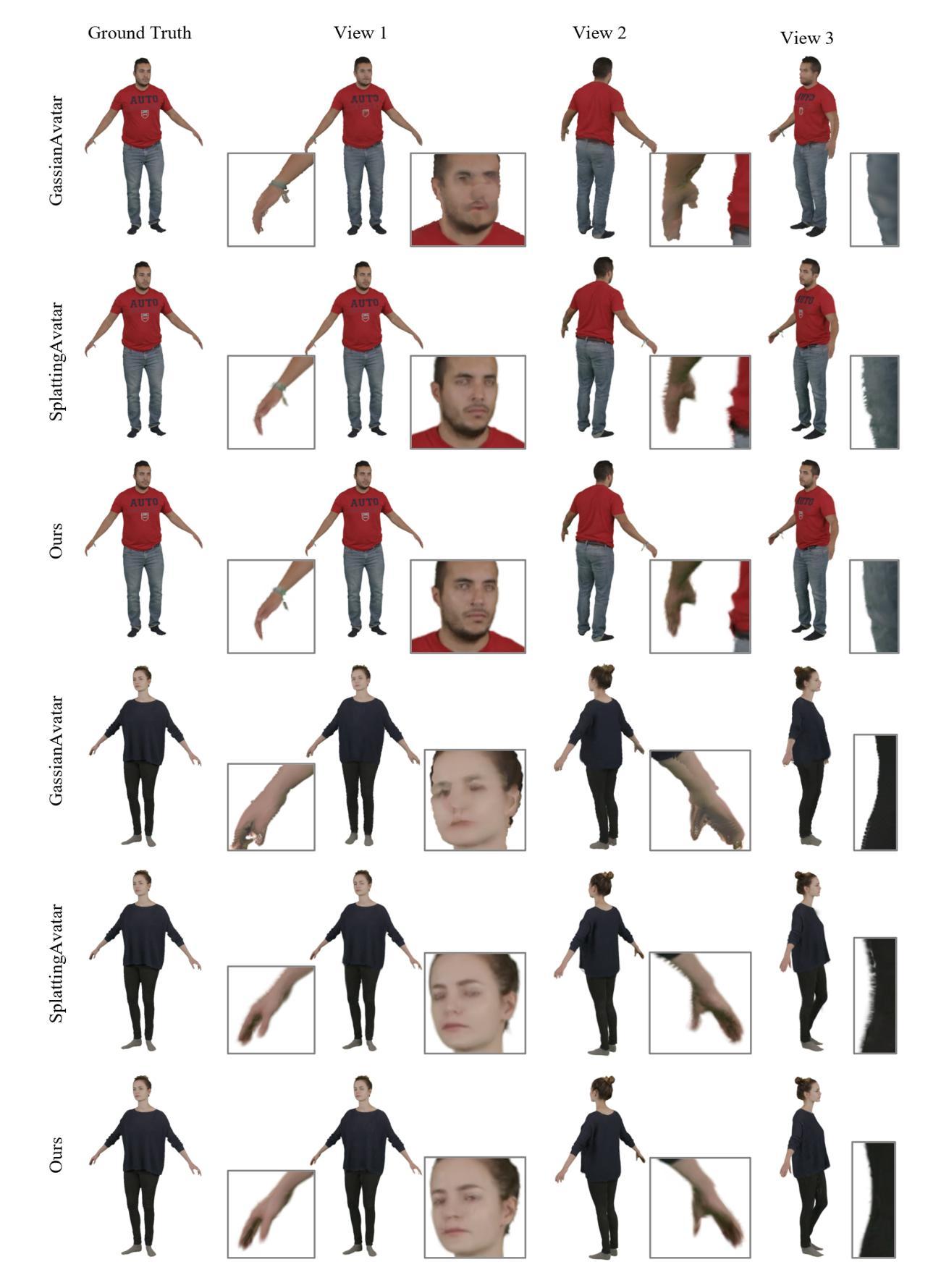
Synthetic Prior for Few-Shot Drivable Head Avatar Inversion
Authors:Wojciech Zielonka, Stephan J. Garbin, Alexandros Lattas, George Kopanas, Paulo Gotardo, Thabo Beeler, Justus Thies, Timo Bolkart
We present SynShot, a novel method for the few-shot inversion of a drivable head avatar based on a synthetic prior. We tackle two major challenges. First, training a controllable 3D generative network requires a large number of diverse sequences, for which pairs of images and high-quality tracked meshes are not always available. Second, state-of-the-art monocular avatar models struggle to generalize to new views and expressions, lacking a strong prior and often overfitting to a specific viewpoint distribution. Inspired by machine learning models trained solely on synthetic data, we propose a method that learns a prior model from a large dataset of synthetic heads with diverse identities, expressions, and viewpoints. With few input images, SynShot fine-tunes the pretrained synthetic prior to bridge the domain gap, modeling a photorealistic head avatar that generalizes to novel expressions and viewpoints. We model the head avatar using 3D Gaussian splatting and a convolutional encoder-decoder that outputs Gaussian parameters in UV texture space. To account for the different modeling complexities over parts of the head (e.g., skin vs hair), we embed the prior with explicit control for upsampling the number of per-part primitives. Compared to state-of-the-art monocular methods that require thousands of real training images, SynShot significantly improves novel view and expression synthesis.
我们提出了一种名为SynShot的新型方法,用于基于合成先验的少量驱动头部化身反演。我们解决了两个主要挑战。首先,训练可控的3D生成网络需要大量的不同序列,而这些序列的图像和高品质跟踪网格并不总是可用。其次,最先进的单眼化身模型很难推广到新的视角和表情,缺乏强大的先验知识,并且经常过度适应特定的视点分布。受仅使用合成数据训练的机器学习模型的启发,我们提出了一种从大量合成头部数据中学习先验模型的方法,这些合成数据具有不同的身份、表情和视点。凭借少量的输入图像,SynShot微调了预训练的合成先验,以弥补领域间的差距,建立一个适用于新表情和视点的逼真头部化身。我们使用3D高斯贴图和一个卷积编码器-解码器来输出UV纹理空间中的高斯参数。为了考虑头部各部分的不同建模复杂性(例如皮肤和头发),我们在先验嵌入中嵌入了明确控制,用于增加每部分基元的数量。与需要数千张真实训练图像的最先进单眼方法相比,SynShot大大改进了新颖视角和表情的合成。
论文及项目相关链接
PDF Website https://zielon.github.io/synshot/
Summary
基于合成先验数据的新型技术SynShot实现了低数据下的可驾驶头部半身像的反向渲染。该技术解决了两个主要问题:缺乏大量不同序列数据的3D生成网络训练,以及现有单眼半身像模型对新视角和表情的泛化能力受限的问题。通过合成数据集的先验模型学习,并在少量真实图像基础上微调该模型,使得该技术能模拟出真实头部半身像并能适应新视角和表情变化。该方法使用高斯网格和卷积编码器-解码器进行头部半身像建模,并考虑了头部不同部分的建模复杂性。相较于依赖数千张真实训练图像的方法,SynShot的技术更胜一筹。
Key Takeaways
- SynShot技术实现了低数据下的可驾驶头部半身像反向渲染技术。
- 它解决了训练3D生成网络缺乏大量不同序列数据的挑战。
- 基于合成数据集的先验模型学习,使得模型能够建模出逼真的头部半身像,并且能适用于新的视角和表情。
点此查看论文截图
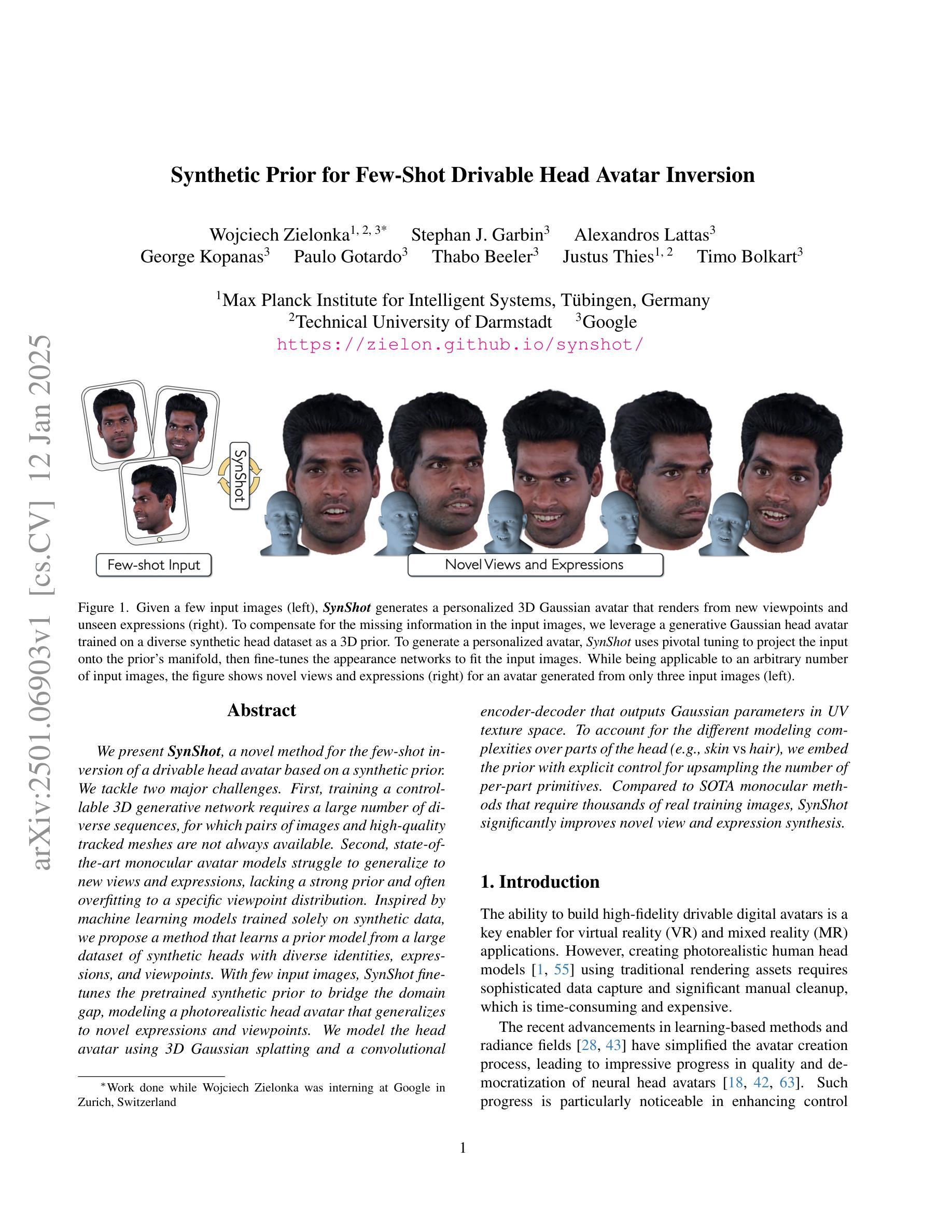
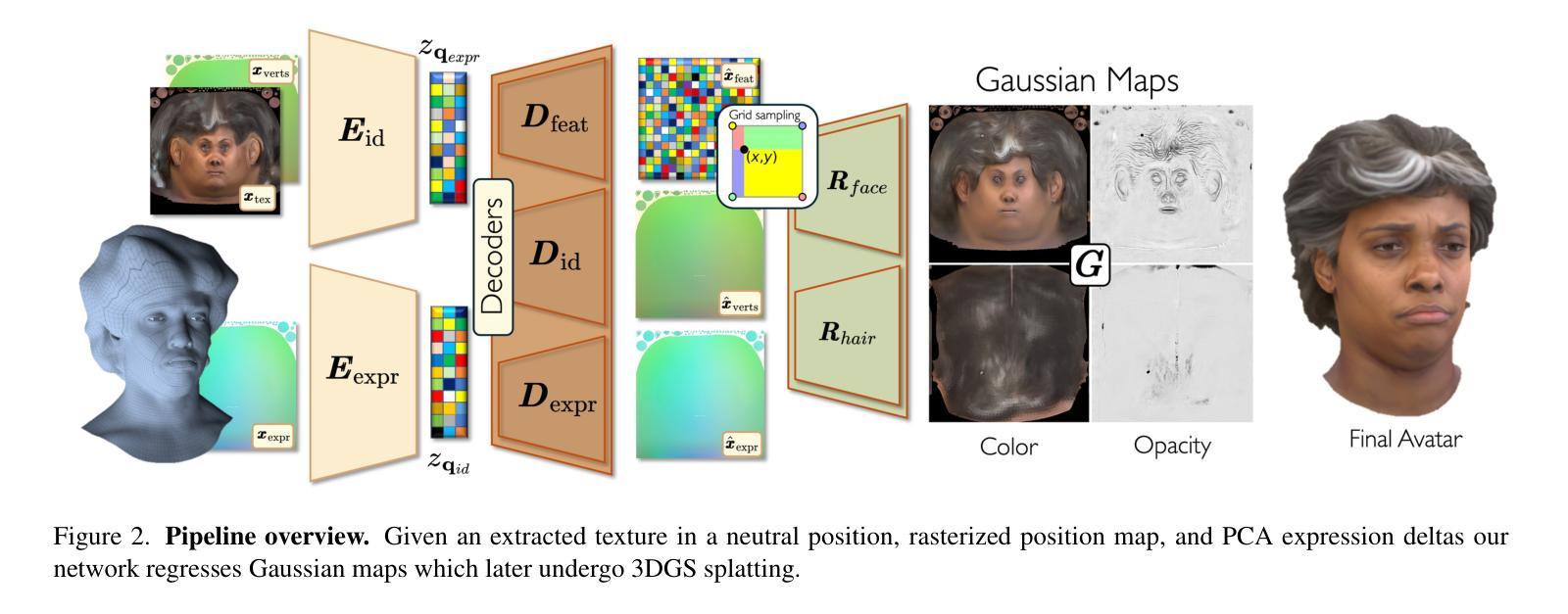
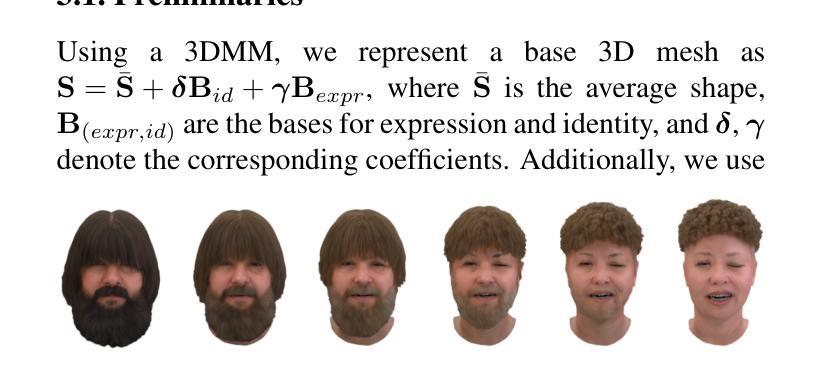
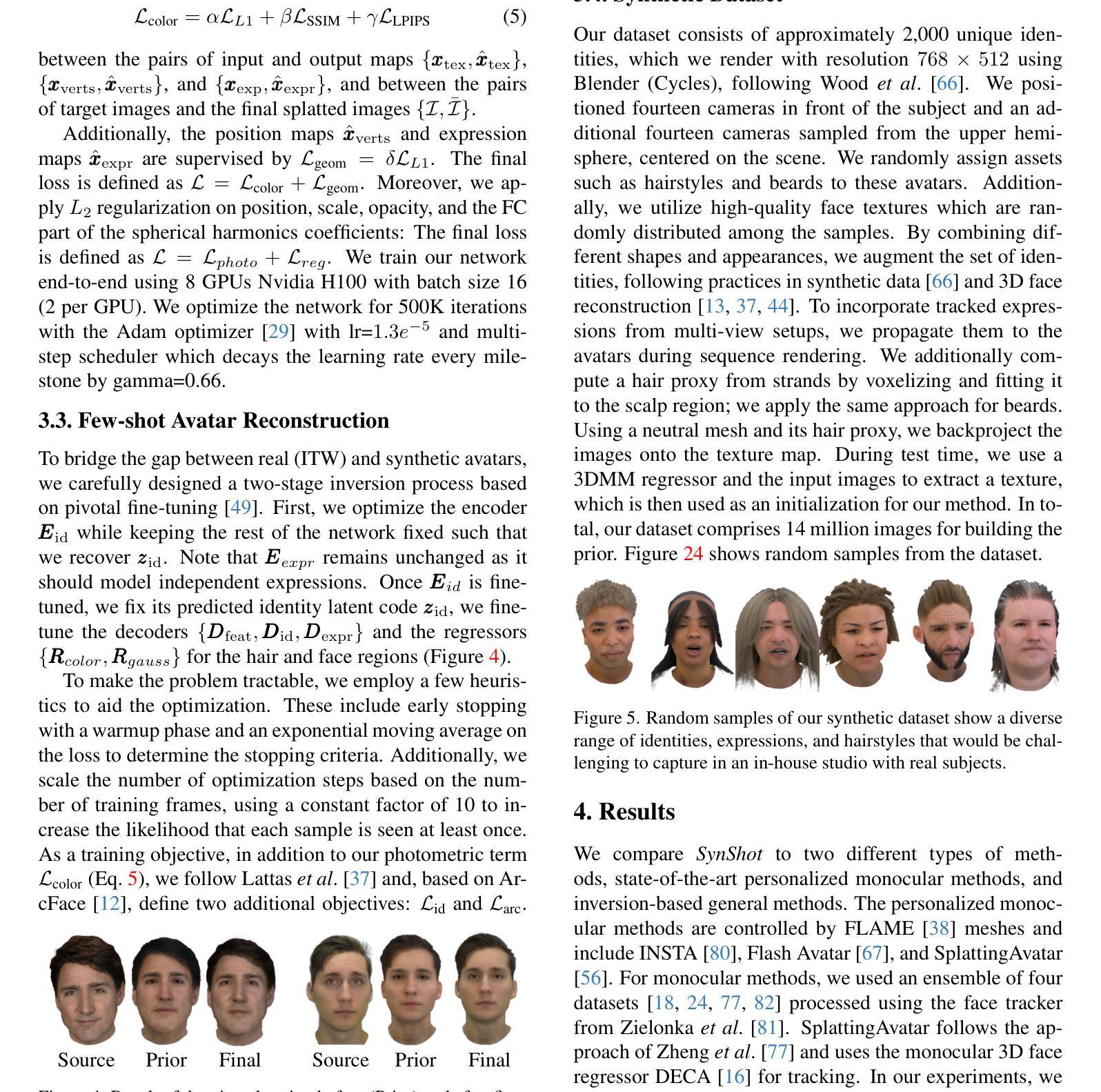
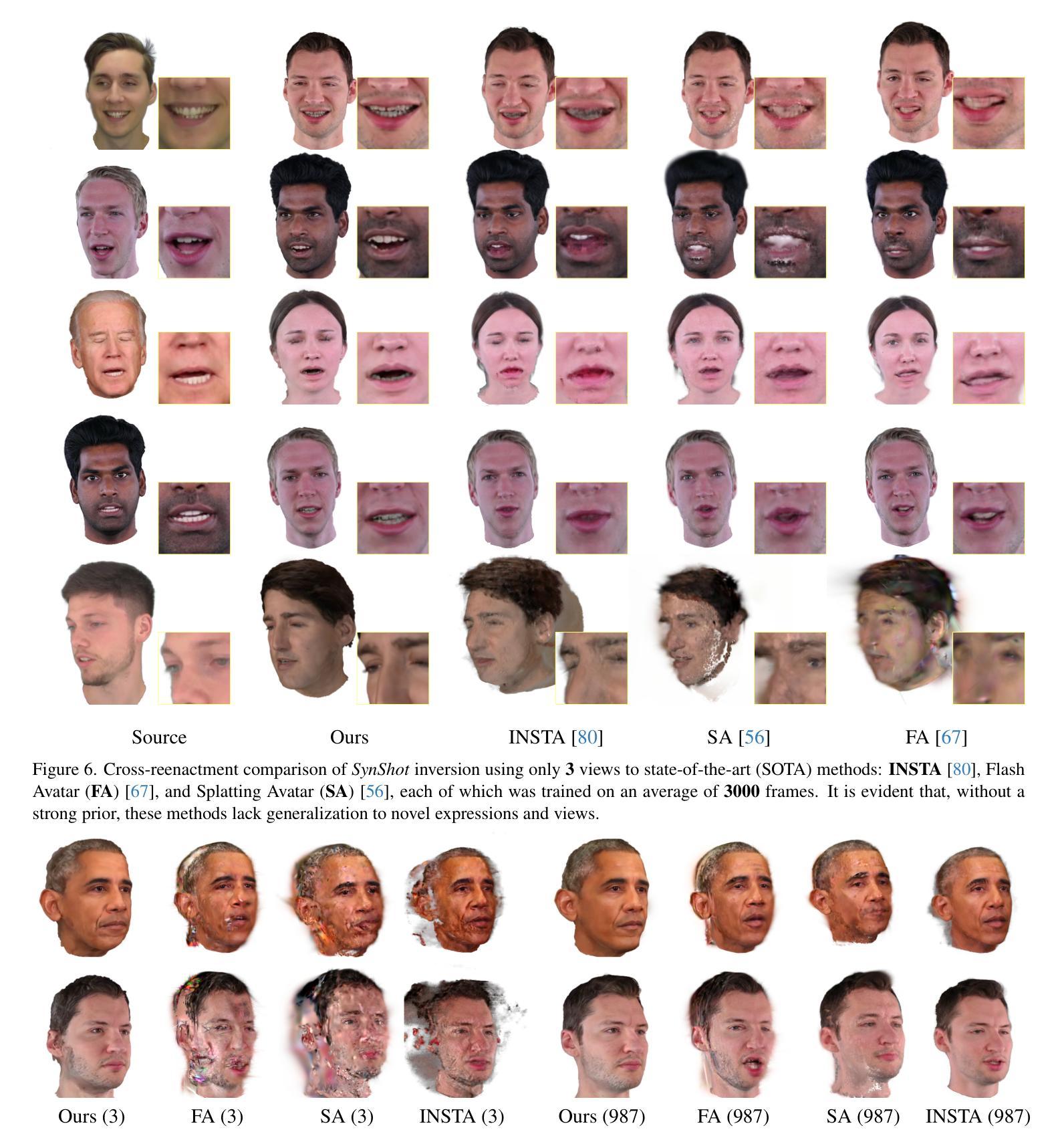
Arc2Avatar: Generating Expressive 3D Avatars from a Single Image via ID Guidance
Authors:Dimitrios Gerogiannis, Foivos Paraperas Papantoniou, Rolandos Alexandros Potamias, Alexandros Lattas, Stefanos Zafeiriou
Inspired by the effectiveness of 3D Gaussian Splatting (3DGS) in reconstructing detailed 3D scenes within multi-view setups and the emergence of large 2D human foundation models, we introduce Arc2Avatar, the first SDS-based method utilizing a human face foundation model as guidance with just a single image as input. To achieve that, we extend such a model for diverse-view human head generation by fine-tuning on synthetic data and modifying its conditioning. Our avatars maintain a dense correspondence with a human face mesh template, allowing blendshape-based expression generation. This is achieved through a modified 3DGS approach, connectivity regularizers, and a strategic initialization tailored for our task. Additionally, we propose an optional efficient SDS-based correction step to refine the blendshape expressions, enhancing realism and diversity. Experiments demonstrate that Arc2Avatar achieves state-of-the-art realism and identity preservation, effectively addressing color issues by allowing the use of very low guidance, enabled by our strong identity prior and initialization strategy, without compromising detail. Please visit https://arc2avatar.github.io for more resources.
受3D高斯喷涂技术(3DGS)在多视角设置下重建详细3D场景的有效性,以及大型二维人类基础模型的出现的启发,我们推出了Arc2Avatar。这是基于SDS的首个方法,仅使用单幅图像作为输入,并以人脸基础模型为指导。为了实现这一点,我们通过微调合成数据并修改其条件,将该模型扩展到多视角的人头生成。我们的虚拟角色与一个人脸网格模板保持密集的对应关系,允许基于blendshape的表情生成。这是通过修改后的3DGS方法、连接正则器和针对我们任务的策略初始化来实现的。此外,我们提出了一种可选的基于SDS的校正步骤,以优化blendshape表情,提高真实感和多样性。实验表明,Arc2Avatar达到了最先进的真实感和身份保留效果,通过允许使用非常低的指导有效解决了颜色问题,得益于我们的强大身份先验知识和初始化策略,且不会损失细节。更多资源请访问:https://arc2avatar.github.io。
论文及项目相关链接
PDF Project Page https://arc2avatar.github.io
Summary
新一代重建技术Arc2Avatar结合了3D高斯描画(3DGS)与人类面孔基础模型,通过单张图片即可生成详细的三维场景。Arc2Avatar使用合成数据进行微调,扩展了用于多种视角的人头生成的模型,并可通过修改条件来实现。该模型生成的虚拟角色与真实人脸模板保持紧密对应关系,并能基于blendshape生成表情。此外,Arc2Avatar还提供了一个高效的SDS校正步骤,用于优化表情的逼真度和多样性。Arc2Avatar在真实感和身份保留方面达到了业界领先水平,解决了色彩问题,并通过强大的身份先验知识和初始化策略实现了低指导下的使用。详情请访问 https://arc2avatar.github.io 。
Key Takeaways
- Arc2Avatar结合了3D高斯描画技术与人类面孔基础模型,实现了单张图片生成详细三维场景。
- Arc2Avatar扩展了用于多种视角的人头生成的模型,通过微调合成数据并修改条件实现。
- Arc2Avatar的虚拟角色与真实人脸模板保持紧密对应关系,支持基于blendshape的表情生成。
- Arc2Avatar提供了一种有效的SDS校正方法,旨在提高表情的逼真度和多样性。
- Arc2Avatar在真实感和身份保留方面表现出色,解决了色彩问题,并实现了低指导下的使用。
点此查看论文截图
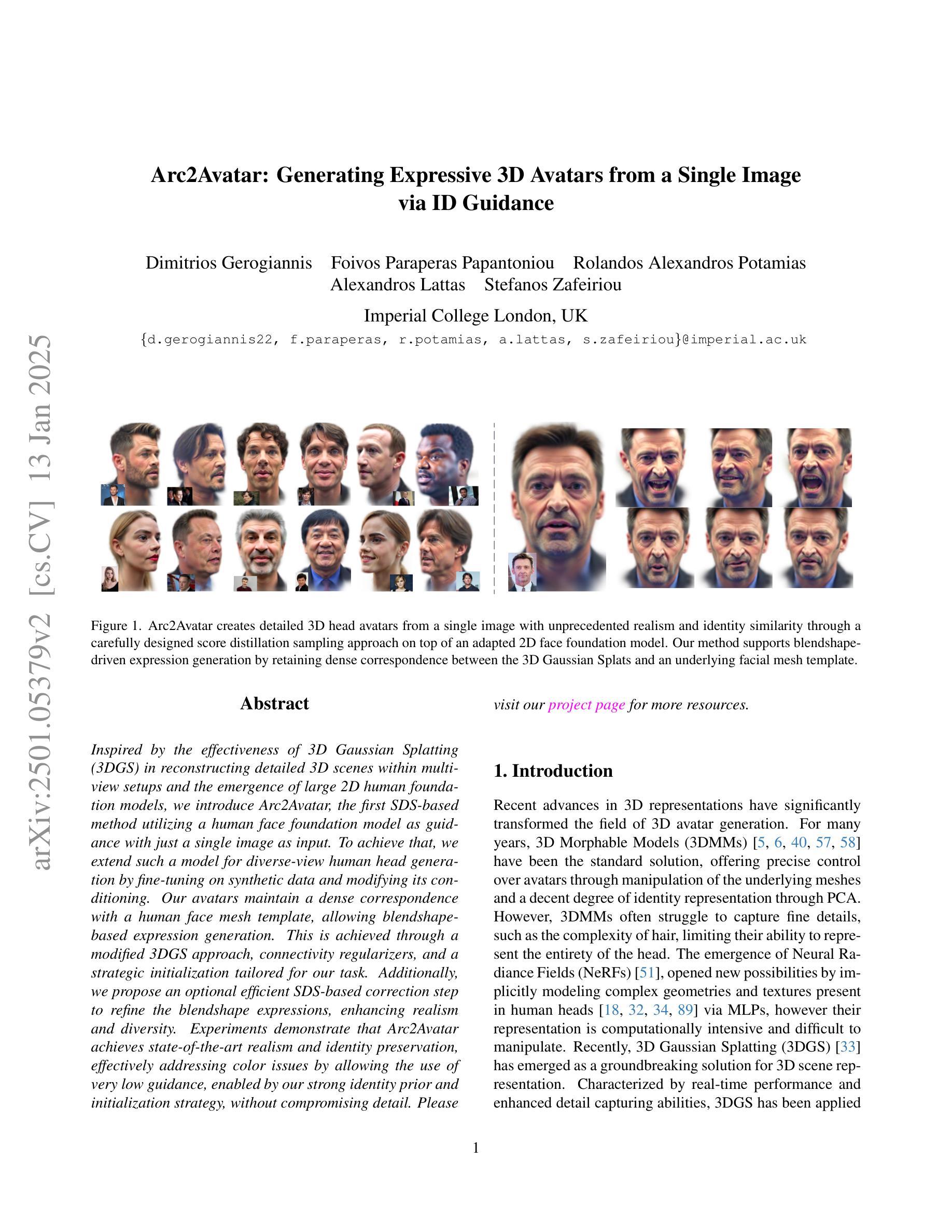
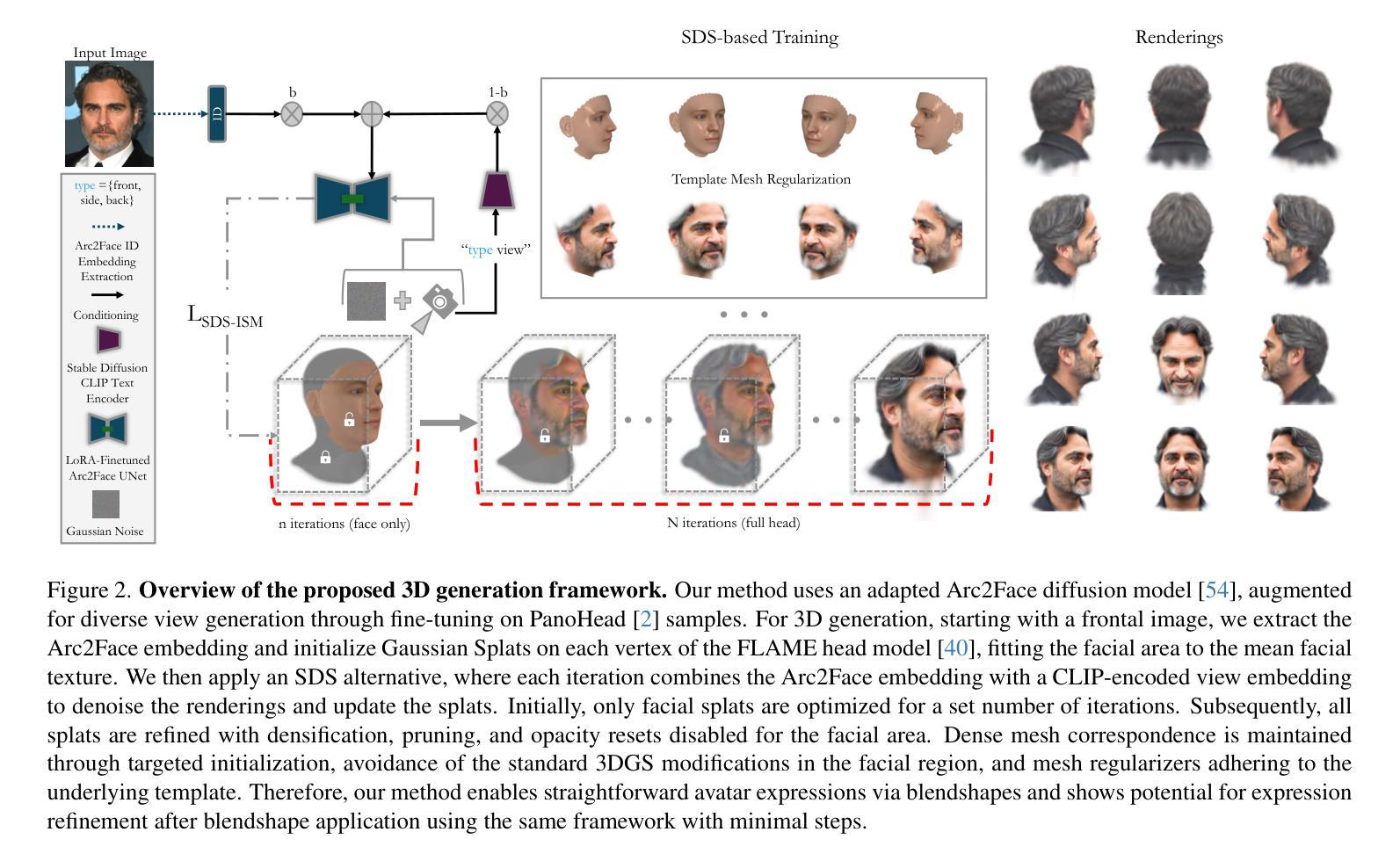
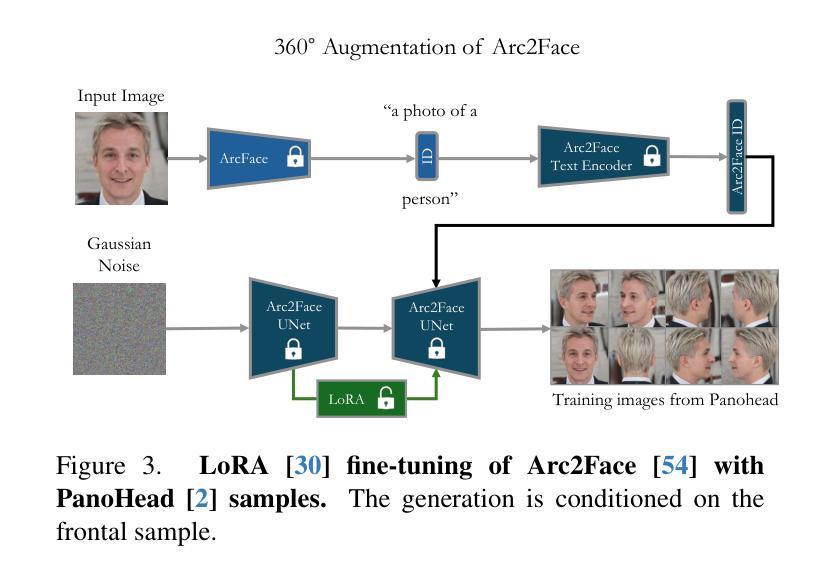
HeadGAP: Few-Shot 3D Head Avatar via Generalizable Gaussian Priors
Authors:Xiaozheng Zheng, Chao Wen, Zhaohu Li, Weiyi Zhang, Zhuo Su, Xu Chang, Yang Zhao, Zheng Lv, Xiaoyuan Zhang, Yongjie Zhang, Guidong Wang, Lan Xu
In this paper, we present a novel 3D head avatar creation approach capable of generalizing from few-shot in-the-wild data with high-fidelity and animatable robustness. Given the underconstrained nature of this problem, incorporating prior knowledge is essential. Therefore, we propose a framework comprising prior learning and avatar creation phases. The prior learning phase leverages 3D head priors derived from a large-scale multi-view dynamic dataset, and the avatar creation phase applies these priors for few-shot personalization. Our approach effectively captures these priors by utilizing a Gaussian Splatting-based auto-decoder network with part-based dynamic modeling. Our method employs identity-shared encoding with personalized latent codes for individual identities to learn the attributes of Gaussian primitives. During the avatar creation phase, we achieve fast head avatar personalization by leveraging inversion and fine-tuning strategies. Extensive experiments demonstrate that our model effectively exploits head priors and successfully generalizes them to few-shot personalization, achieving photo-realistic rendering quality, multi-view consistency, and stable animation.
本文提出了一种新型3D头部化身创建方法,能够从少量野外数据中进行高度逼真和可动画的鲁棒性概括。鉴于这个问题的未约束性质,融入先验知识至关重要。因此,我们提出了一个由先验学习阶段和化身创建阶段组成的框架。先验学习阶段利用大规模多视角动态数据集推导出的3D头部先验知识,而化身创建阶段则应用这些先验知识来进行少量个性化设置。我们的方法通过利用基于高斯拼贴技术的自动解码器网络和基于部分的动态建模来有效地捕捉这些先验知识。我们的方法采用具有个性化潜在代码的共享身份编码来学习高斯原始数据的属性。在化身创建阶段,我们通过利用反演和微调策略实现了快速的头部化身个性化。大量实验表明,我们的模型有效地利用了头部先验知识,并成功地将其推广到少量个性化设置中,实现了照片级渲染质量、多视角一致性和稳定的动画效果。
论文及项目相关链接
PDF Accepted to 3DV 2025. Project page: https://headgap.github.io/
Summary
在这个论文中,我们提出了一种全新的三维头部虚拟形象创建方法,该方法能够从少量的实际数据中实现高度保真和可动画的稳健性。为了解决这个问题,引入先验知识是必要的。因此,我们提出了一个包含先验学习阶段和虚拟形象创建阶段的框架。先验学习阶段利用大规模多视角动态数据集推导出的三维头部先验知识,而虚拟形象创建阶段则应用这些先验知识实现个性化快速创建。我们的方法利用基于高斯拼贴技术的自动解码器网络进行部分动态建模,以捕捉这些先验知识。在虚拟形象创建阶段,我们通过利用反演和微调策略实现了快速头部虚拟形象的个性化。大量实验表明,我们的模型有效地利用了头部先验知识,并成功将其推广到个性化创建中,实现了照片级渲染质量、多视角一致性和稳定的动画效果。
Key Takeaways
- 该论文介绍了一种新型三维头部虚拟形象创建方法,可从少量实际数据中实现高度保真和稳健的动画效果。
- 引入先验知识是解决此问题的关键,论文提出了一个包含先验学习阶段和虚拟形象创建阶段的框架。
- 先验学习阶段利用大规模多视角动态数据集来推导三维头部先验知识。
- 虚拟形象创建阶段应用这些先验知识实现个性化快速创建,通过反演和微调策略加速过程。
- 该方法利用高斯拼贴技术自动解码器网络和部分动态建模来捕捉先验知识。
- 模型实验证明,该方法能有效利用头部先验知识,并成功推广至个性化创建,实现照片级渲染质量。
点此查看论文截图
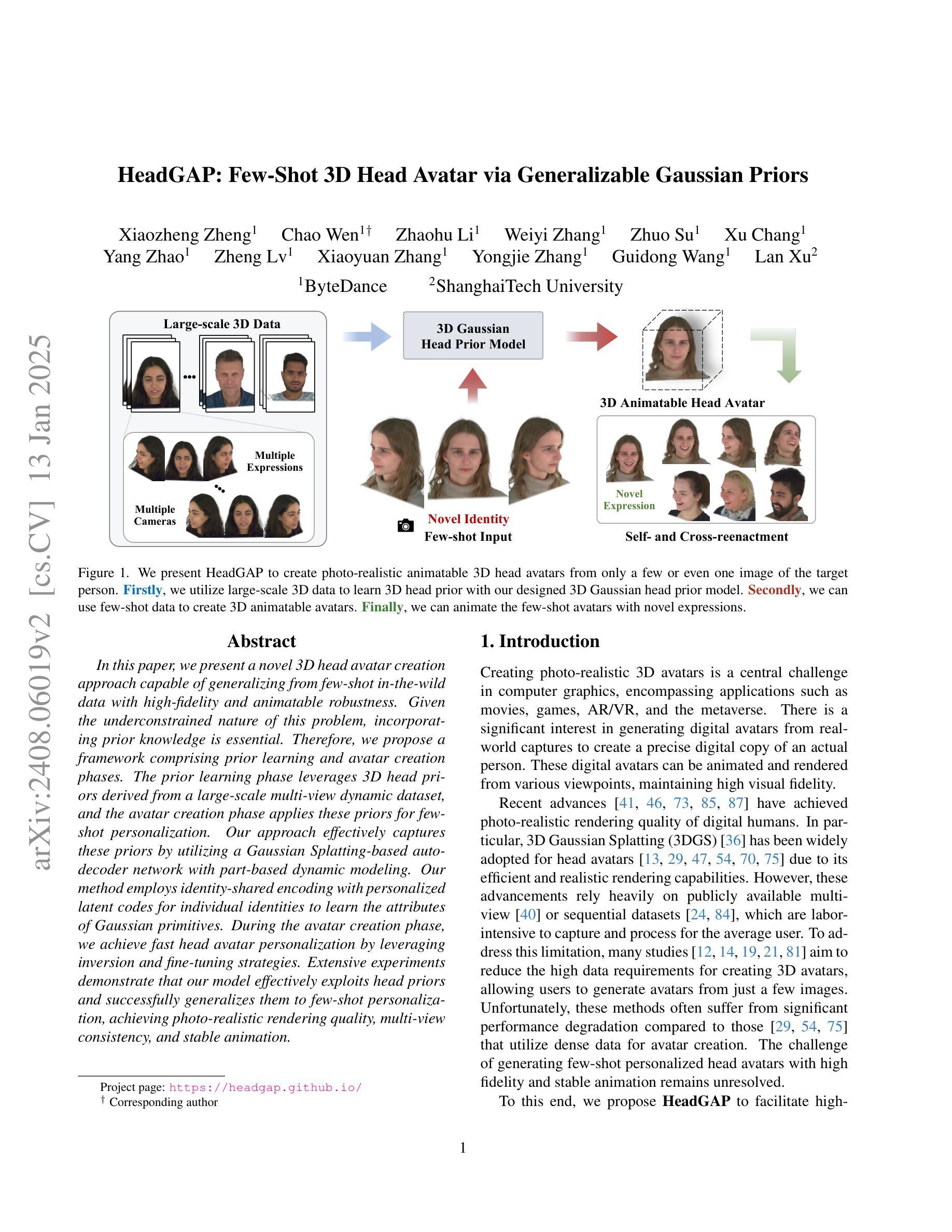
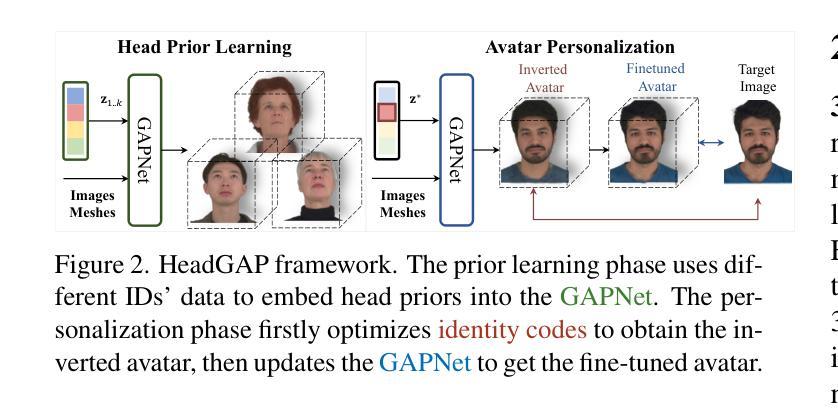
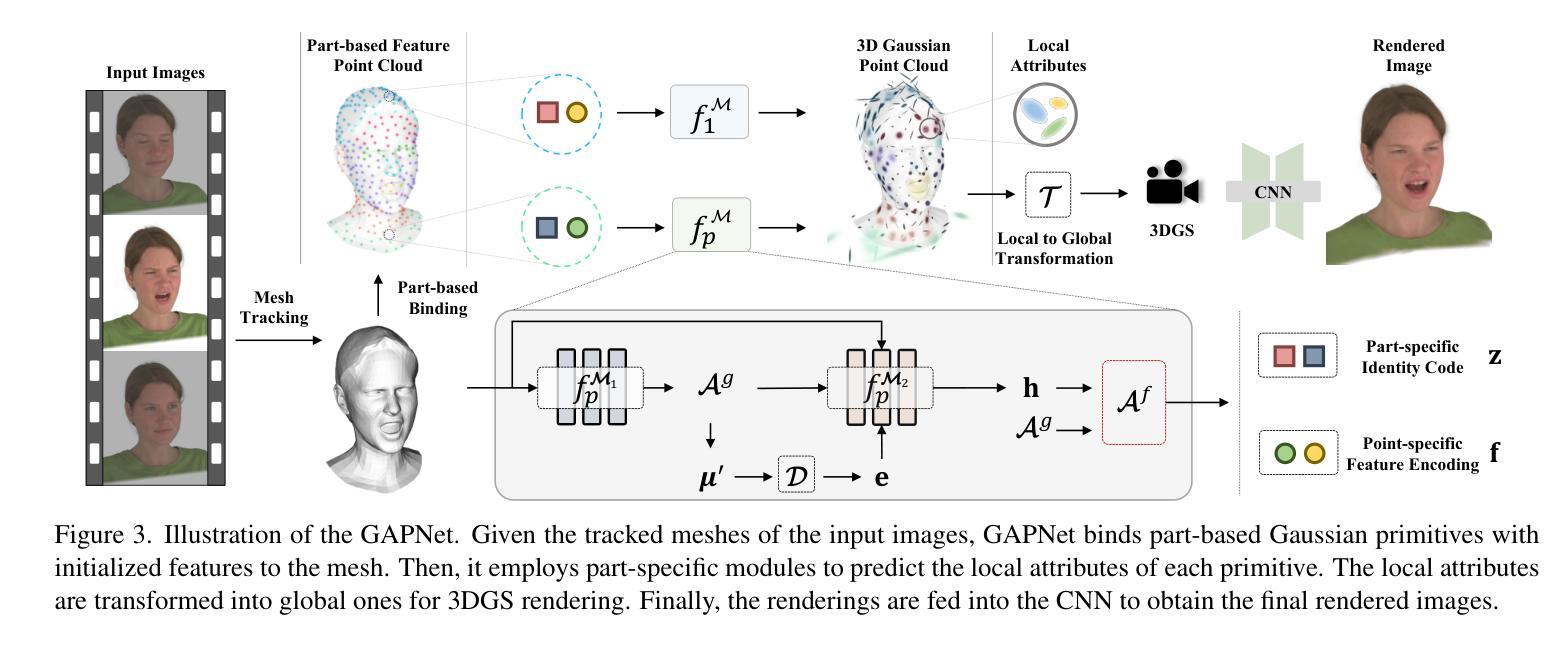
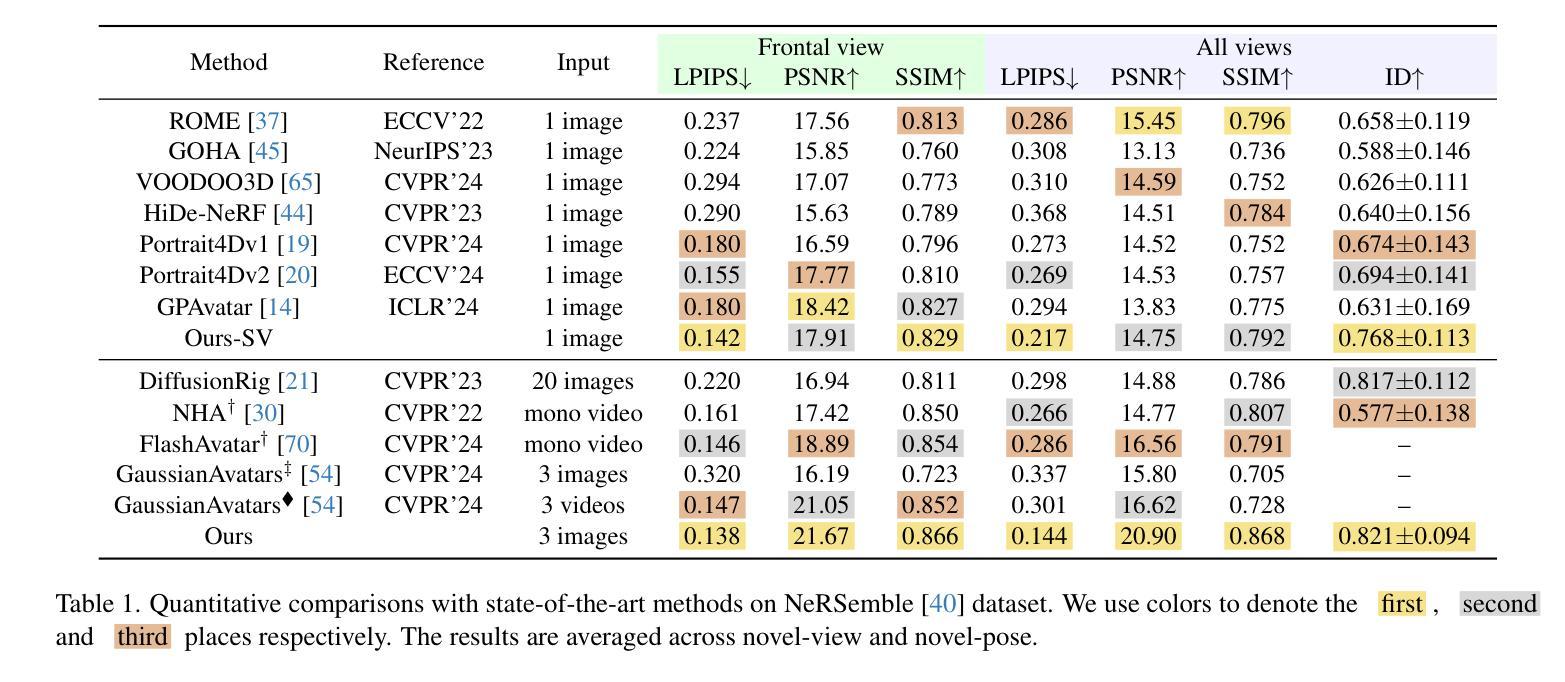
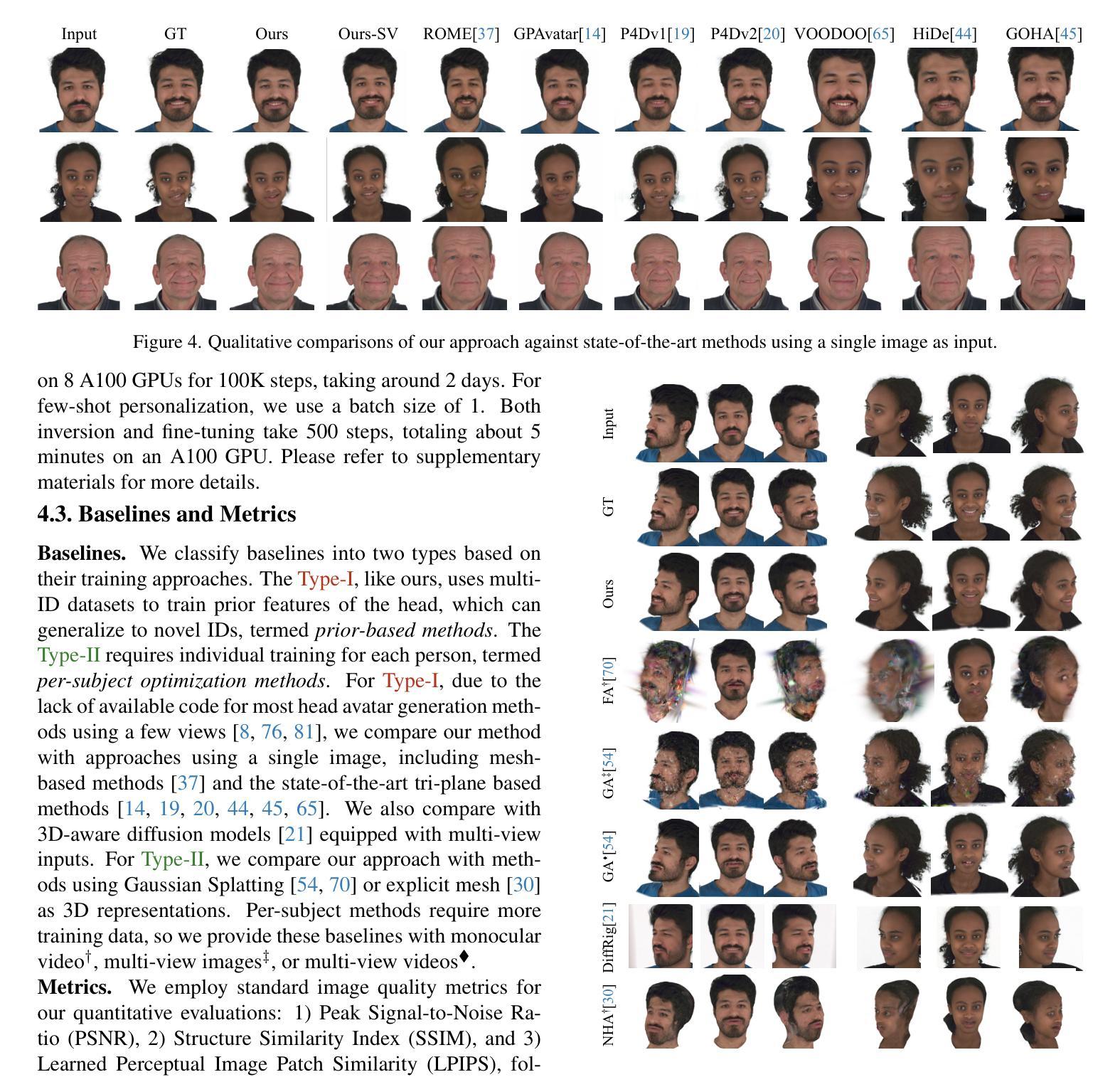