⚠️ 以下所有内容总结都来自于 大语言模型的能力,如有错误,仅供参考,谨慎使用
🔴 请注意:千万不要用于严肃的学术场景,只能用于论文阅读前的初筛!
💗 如果您觉得我们的项目对您有帮助 ChatPaperFree ,还请您给我们一些鼓励!⭐️ HuggingFace免费体验
2025-01-16 更新
A Foundational Generative Model for Breast Ultrasound Image Analysis
Authors:Haojun Yu, Youcheng Li, Nan Zhang, Zihan Niu, Xuantong Gong, Yanwen Luo, Haotian Ye, Siyu He, Quanlin Wu, Wangyan Qin, Mengyuan Zhou, Jie Han, Jia Tao, Ziwei Zhao, Di Dai, Di He, Dong Wang, Binghui Tang, Ling Huo, James Zou, Qingli Zhu, Yong Wang, Liwei Wang
Foundational models have emerged as powerful tools for addressing various tasks in clinical settings. However, their potential development to breast ultrasound analysis remains untapped. In this paper, we present BUSGen, the first foundational generative model specifically designed for breast ultrasound image analysis. Pretrained on over 3.5 million breast ultrasound images, BUSGen has acquired extensive knowledge of breast structures, pathological features, and clinical variations. With few-shot adaptation, BUSGen can generate repositories of realistic and informative task-specific data, facilitating the development of models for a wide range of downstream tasks. Extensive experiments highlight BUSGen’s exceptional adaptability, significantly exceeding real-data-trained foundational models in breast cancer screening, diagnosis, and prognosis. In breast cancer early diagnosis, our approach outperformed all board-certified radiologists (n=9), achieving an average sensitivity improvement of 16.5% (P-value<0.0001). Additionally, we characterized the scaling effect of using generated data which was as effective as the collected real-world data for training diagnostic models. Moreover, extensive experiments demonstrated that our approach improved the generalization ability of downstream models. Importantly, BUSGen protected patient privacy by enabling fully de-identified data sharing, making progress forward in secure medical data utilization. An online demo of BUSGen is available at https://aibus.bio.
基础模型已作为临床环境中处理各种任务的强大工具而出现。然而,它们在乳腺超声分析方面的潜力尚未被开发。在本文中,我们推出了BUSGen,这是专为乳腺超声图像分析设计的基础生成模型。BUSGen预训练在超过350万张乳腺超声图像上进行,已获得对乳腺结构、病理特征和临床变化的广泛知识。通过少量镜头适应,BUSGen可以生成现实且信息丰富的特定任务数据仓库,促进针对各种下游任务的模型发展。大量实验突出了BUSGen的非凡适应性,在乳腺癌筛查、诊断和预后方面显著超过了使用真实数据训练的基础模型。在乳腺癌早期诊断中,我们的方法优于所有执业医师的放射科医生(n=9),平均灵敏度提高了16.5%(P值<0.0001)。此外,我们描述了使用生成数据与收集的真实世界数据一样有效的规模效益,用于训练诊断模型。而且,大量实验表明,我们的方法提高了下游模型的泛化能力。重要的是,BUSGen通过实现完全匿名数据共享来保护患者隐私,在安全医疗数据利用方面取得了进展。BUSGen的在线演示可在https://aibus.bio上找到。
论文及项目相关链接
PDF Peking University; Stanford University; Peking University Cancer Hospital & Institute; Peking Union Medical College Hospital; Cancer Hospital, Chinese Academy of Medical Sciences
Summary
乳腺超声图像分析领域的基础模型研究取得突破。研究团队提出了针对乳腺超声图像分析的首个基础生成模型BUSGen。该模型经过超过350万张乳腺超声图像的训练,具备丰富的乳腺结构、病理特征和临床变化知识。通过少量样本适应,BUSGen能生成真实且信息丰富的任务特定数据,促进多种下游任务模型的发展。实验显示,BUSGen在乳腺癌筛查、诊断和预后等方面展现出卓越的适应性,早期乳腺癌诊断性能超过九位资深放射科医生。此外,生成的乳腺超声数据对于提升诊断模型的泛化能力具有显著效果,同时保护患者隐私。
Key Takeaways
- BUSGen是首个针对乳腺超声图像分析的基础生成模型。
- 该模型经过大量乳腺超声图像训练,具备丰富的乳腺相关知识。
- BUSGen能通过少量样本适应,生成真实且信息丰富的任务特定数据。
- 在乳腺癌筛查、诊断和预后等方面,BUSGen表现出卓越性能,超过资深放射科医生。
- 生成的乳腺超声数据能有效提升诊断模型的泛化能力。
- BUSGen重视保护患者隐私,实现完全匿名数据共享。
点此查看论文截图
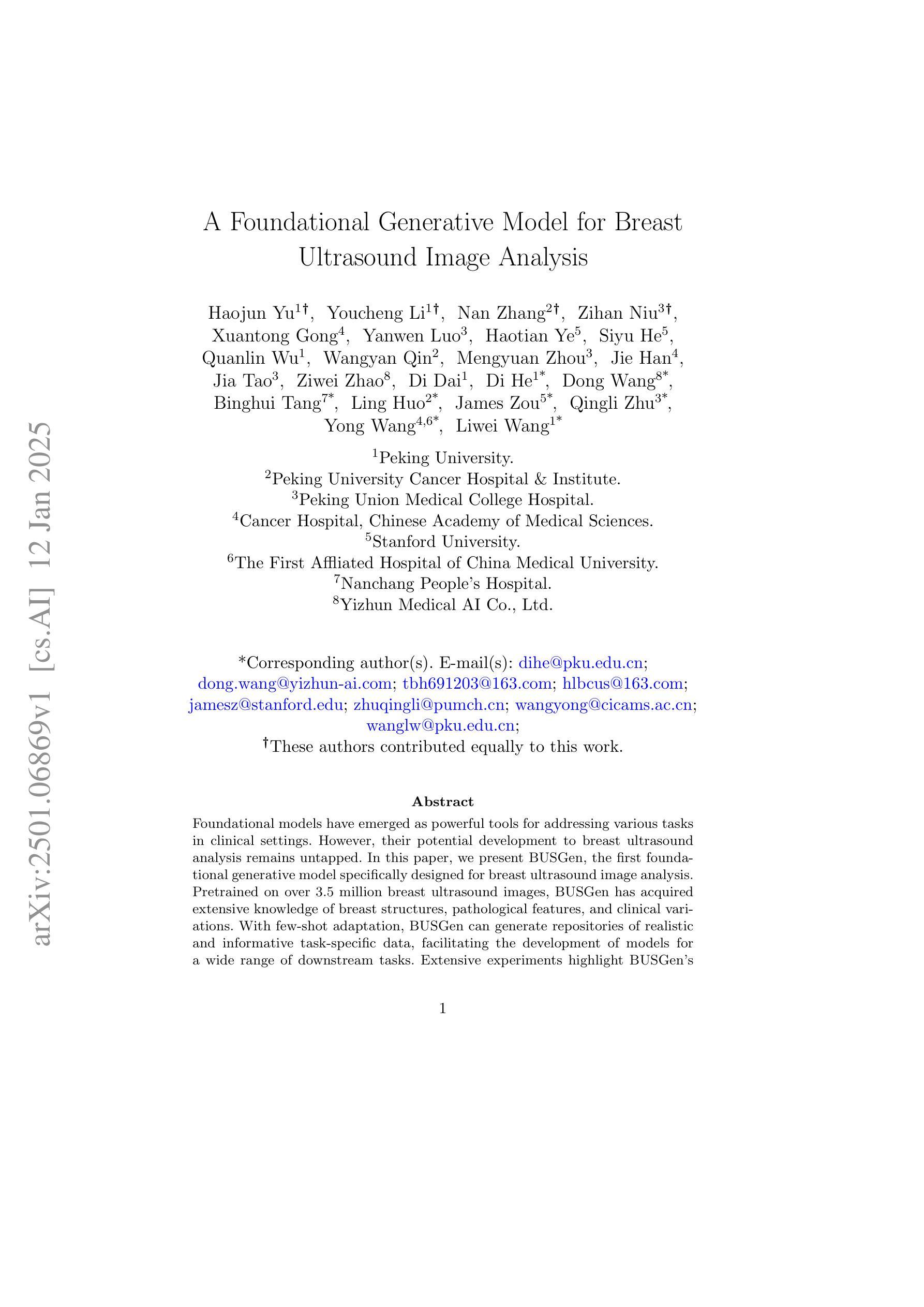
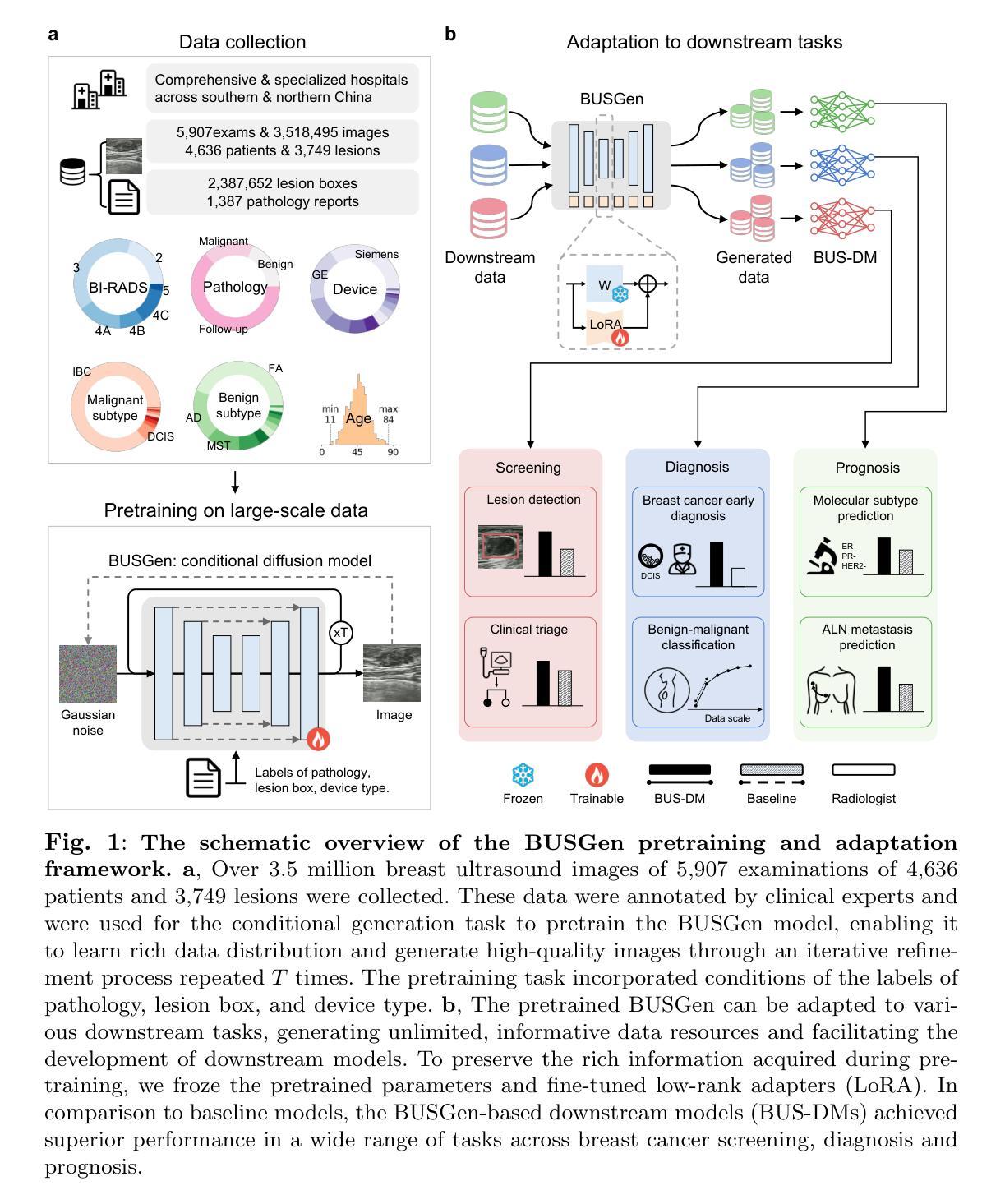
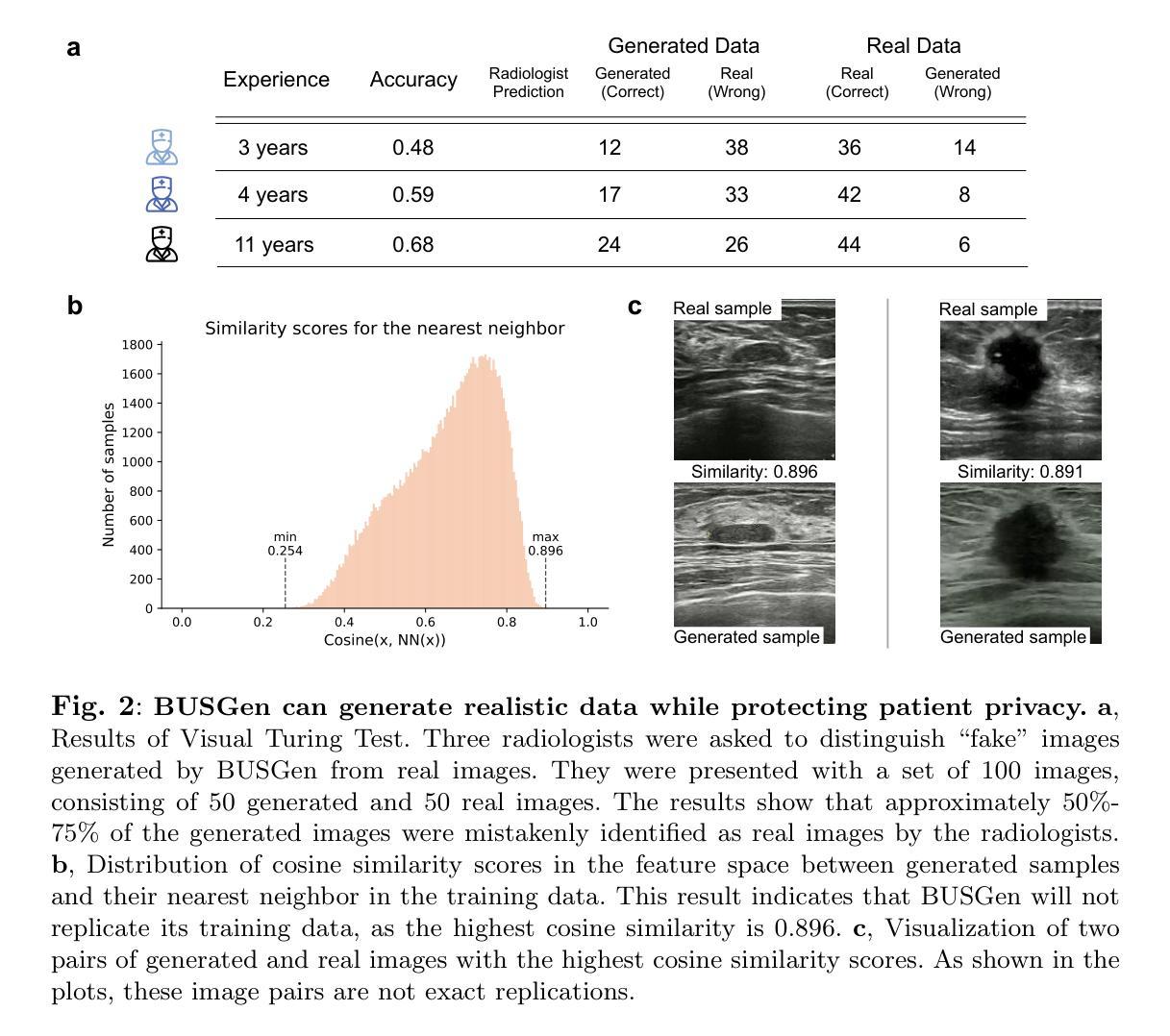