⚠️ 以下所有内容总结都来自于 大语言模型的能力,如有错误,仅供参考,谨慎使用
🔴 请注意:千万不要用于严肃的学术场景,只能用于论文阅读前的初筛!
💗 如果您觉得我们的项目对您有帮助 ChatPaperFree ,还请您给我们一些鼓励!⭐️ HuggingFace免费体验
2025-01-16 更新
Generating and Detecting Various Types of Fake Image and Audio Content: A Review of Modern Deep Learning Technologies and Tools
Authors:Arash Dehghani, Hossein Saberi
This paper reviews the state-of-the-art in deepfake generation and detection, focusing on modern deep learning technologies and tools based on the latest scientific advancements. The rise of deepfakes, leveraging techniques like Variational Autoencoders (VAEs), Generative Adversarial Networks (GANs), Diffusion models and other generative models, presents significant threats to privacy, security, and democracy. This fake media can deceive individuals, discredit real people and organizations, facilitate blackmail, and even threaten the integrity of legal, political, and social systems. Therefore, finding appropriate solutions to counter the potential threats posed by this technology is essential. We explore various deepfake methods, including face swapping, voice conversion, reenactment and lip synchronization, highlighting their applications in both benign and malicious contexts. The review critically examines the ongoing “arms race” between deepfake generation and detection, analyzing the challenges in identifying manipulated contents. By examining current methods and highlighting future research directions, this paper contributes to a crucial understanding of this rapidly evolving field and the urgent need for robust detection strategies to counter the misuse of this powerful technology. While focusing primarily on audio, image, and video domains, this study allows the reader to easily grasp the latest advancements in deepfake generation and detection.
本文综述了深度伪造生成与检测的最新进展,重点关注基于最新科学进步的现代深度学习技术和工具。深度伪造技术的崛起,利用变分自编码器(VAEs)、生成对抗网络(GANs)、扩散模型和其他生成模型等技术,对隐私、安全和民主产生了重大威胁。这种虚假媒体可以欺骗个人,诋毁真实的人物和组织,助长敲诈行为,甚至威胁法律、政治和社会制度的完整性。因此,寻找适当解决方案来应对这项技术带来的潜在威胁至关重要。我们探索了各种深度伪造方法,包括面部替换、语音转换、重演和唇部同步,强调了它们在不同场景下的应用。本文深入探讨了深度伪造生成与检测之间正在进行的“军备竞赛”,分析了识别操纵内容的挑战。通过对当前方法的审视以及对未来研究方向的强调,本文对于这一快速演变领域的关键理解做出了贡献,并突显了对这一强大技术滥用进行稳健检测策略的迫切需求。虽然本文的主要焦点是音频、图像和视频领域,但研究内容使读者能够轻松掌握深度伪造生成与检测的最新进展。
论文及项目相关链接
Summary
本文回顾了最新深度伪造生成与检测技术的进展,主要探讨了现代深度学习技术和工具的运用情况。随着利用变分自编码器(VAEs)、生成对抗网络(GANs)、扩散模型以及其他生成模型等技术的深度伪造现象的兴起,对隐私、安全和民主制度产生了重大威胁。本文深入探讨了各种深度伪造方法,包括换脸、语音转换、再现和唇部同步等技术,并强调它们在不同领域的应用及潜在风险。文章还对当前的生成与检测之间的“军备竞赛”进行了深入分析,指出了识别操纵内容的挑战,并强调了未来研究方向的重要性。本文旨在让读者轻松了解深度伪造生成与检测领域的最新进展。
Key Takeaways
- 深度伪造技术已经利用最新的科学进步快速发展,包括使用变分自编码器(VAEs)和生成对抗网络(GANs)。
- 深度伪造技术存在重大威胁,可能侵犯隐私、破坏安全并影响民主制度。
- 深度伪造技术包括换脸、语音转换、再现和唇部同步等,具有在多个领域应用的潜力,包括恶意用途。
- 当前存在识别操纵内容的挑战,这体现了一个“军备竞赛”的状况,需要在未来进行更深入的研究。
- 深度伪造生成与检测领域的最新进展在音频、图像和视频领域都有涉及。
- 对深度伪造技术的滥用需要制定有效的检测策略来应对。
点此查看论文截图
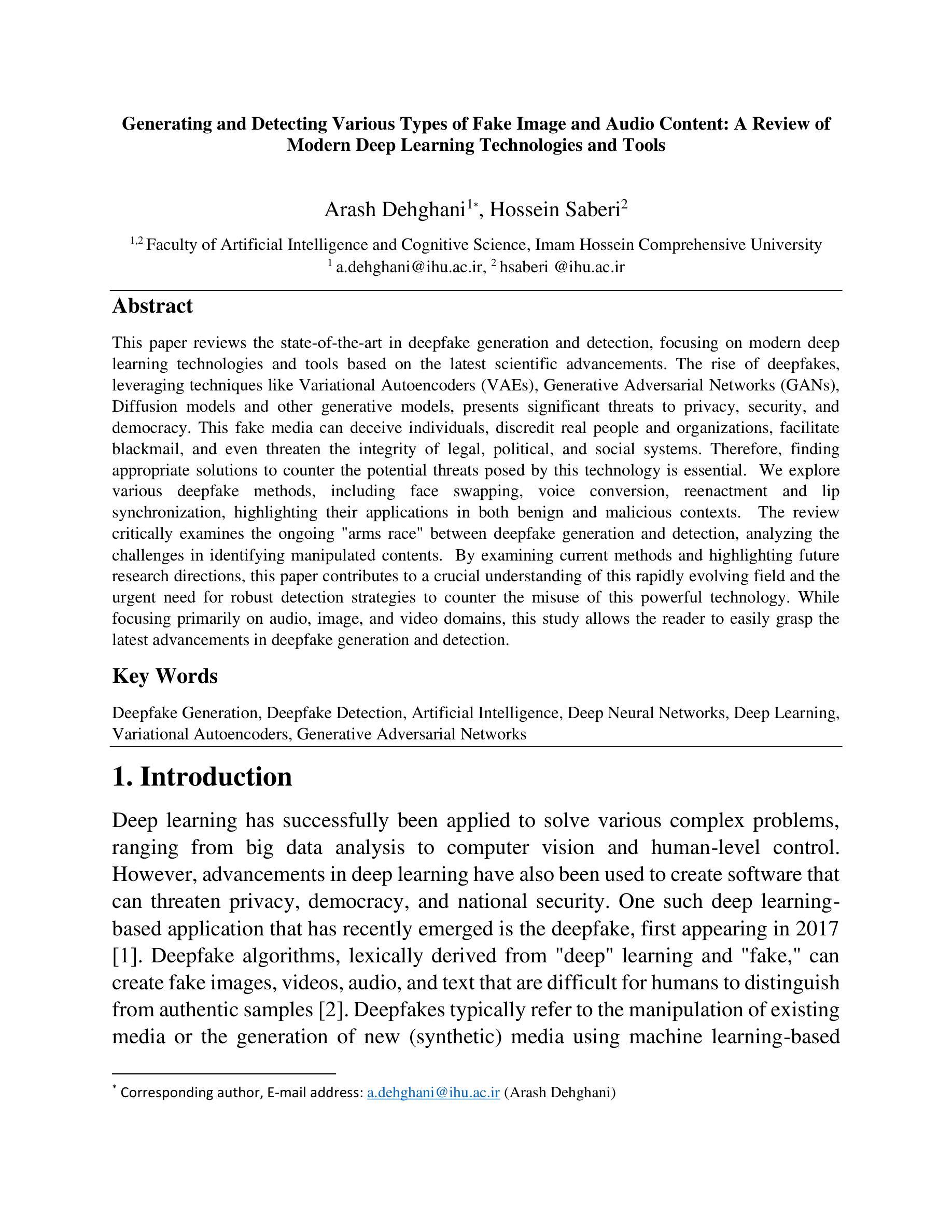