⚠️ 以下所有内容总结都来自于 大语言模型的能力,如有错误,仅供参考,谨慎使用
🔴 请注意:千万不要用于严肃的学术场景,只能用于论文阅读前的初筛!
💗 如果您觉得我们的项目对您有帮助 ChatPaperFree ,还请您给我们一些鼓励!⭐️ HuggingFace免费体验
2025-01-16 更新
Defect Detection Network In PCB Circuit Devices Based on GAN Enhanced YOLOv11
Authors:Jiayi Huang, Feiyun Zhao, Lieyang Chen
This study proposes an advanced method for surface defect detection in printed circuit boards (PCBs) using an improved YOLOv11 model enhanced with a generative adversarial network (GAN). The approach focuses on identifying six common defect types: missing hole, rat bite, open circuit, short circuit, burr, and virtual welding. By employing GAN to generate synthetic defect images, the dataset is augmented with diverse and realistic patterns, improving the model’s ability to generalize, particularly for complex and infrequent defects like burrs. The enhanced YOLOv11 model is evaluated on a PCB defect dataset, demonstrating significant improvements in accuracy, recall, and robustness, especially when dealing with defects in complex environments or small targets. This research contributes to the broader field of electronic design automation (EDA), where efficient defect detection is a crucial step in ensuring high-quality PCB manufacturing. By integrating advanced deep learning techniques, this approach enhances the automation and precision of defect detection, reducing reliance on manual inspection and accelerating design-to-production workflows. The findings underscore the importance of incorporating GAN-based data augmentation and optimized detection architectures in EDA processes, providing valuable insights for improving reliability and efficiency in PCB defect detection within industrial applications.
本研究提出了一种利用改进型YOLOv11模型结合生成对抗网络(GAN)对印刷电路板(PCBs)表面缺陷进行检测的先进方法。该方法专注于识别六种常见缺陷类型:缺孔、鼠咬、开路、短路、毛刺和虚焊。通过采用GAN生成合成缺陷图像,数据集增加了多样化和逼真的模式,提高了模型泛化的能力,特别是对于毛刺等复杂和不常见的缺陷。在PCB缺陷数据集上评估了增强型YOLOv11模型,在准确性、召回率和稳健性方面显示出显著改进,特别是在处理复杂环境或小目标缺陷时。该研究为电子设计自动化(EDA)这一更广泛的领域做出了贡献,其中高效的缺陷检测是确保高质量PCB制造的关键步骤。通过集成先进的深度学习技术,此方法提高了缺陷检测的自动化和精度,减少了对手动检查的依赖,并加速了设计到生产的流程。研究结果强调了将基于GAN的数据增强和优化检测架构纳入EDA过程的重要性,为工业应用中提高PCB缺陷检测的可靠性和效率提供了宝贵的见解。
论文及项目相关链接
Summary
本研究提出了一种利用改进YOLOv11模型与生成对抗网络(GAN)结合的先进方法,用于印刷电路板(PCB)表面缺陷检测。该方法专注于识别六种常见缺陷类型,并通过GAN生成合成缺陷图像来扩充数据集,提高了模型对复杂和罕见缺陷的泛化能力。研究评估了增强型YOLOv11模型在PCB缺陷数据集上的表现,结果显示其在准确率、召回率和稳健性方面有明显提升。本研究为电子设计自动化(EDA)领域做出贡献,该研究中深度学习的先进技术应用提高了缺陷检测的自动化和精度,减少了人工检测依赖,并加速了从设计到生产的工作流程。研究结果表明,在EDA过程中结合GAN数据增强和优化检测架构至关重要,为工业应用中提高PCB缺陷检测的可靠性和效率提供了宝贵见解。
Key Takeaways
- 研究提出了一种利用改进YOLOv11模型和GAN进行PCB表面缺陷检测的新方法。
- 该方法可以识别六种常见缺陷类型,并通过GAN生成合成缺陷图像来扩充数据集。
- GAN的应用提高了模型对复杂和罕见缺陷的泛化能力。
- 增强型YOLOv11模型在PCB缺陷数据集上的准确率、召回率和稳健性有所提升。
- 研究成果对电子设计自动化(EDA)领域有贡献,提高了缺陷检测的自动化和精度。
- 集成深度学习的先进技术有助于减少人工检测依赖,并加速设计到生产的过程。
点此查看论文截图
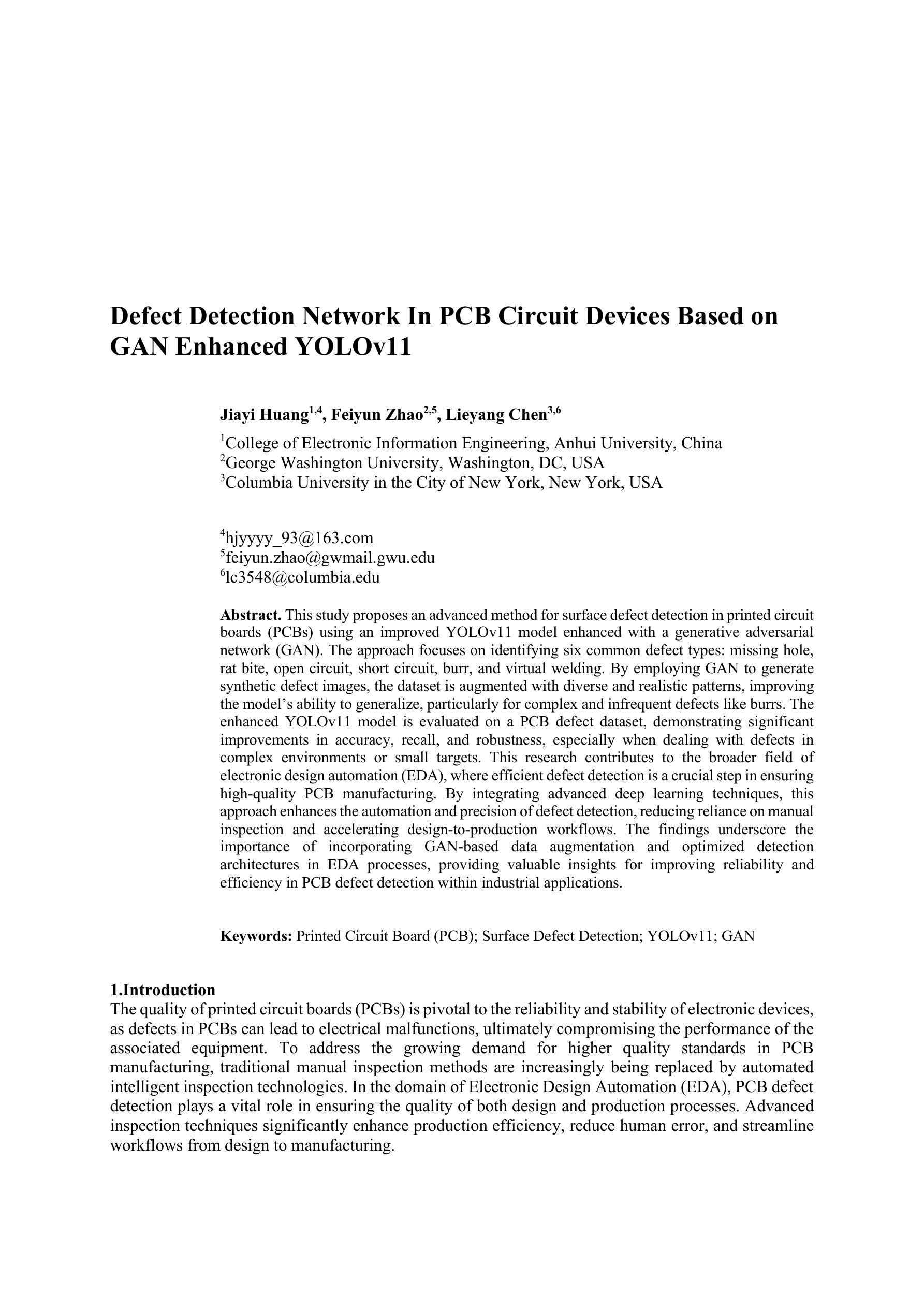
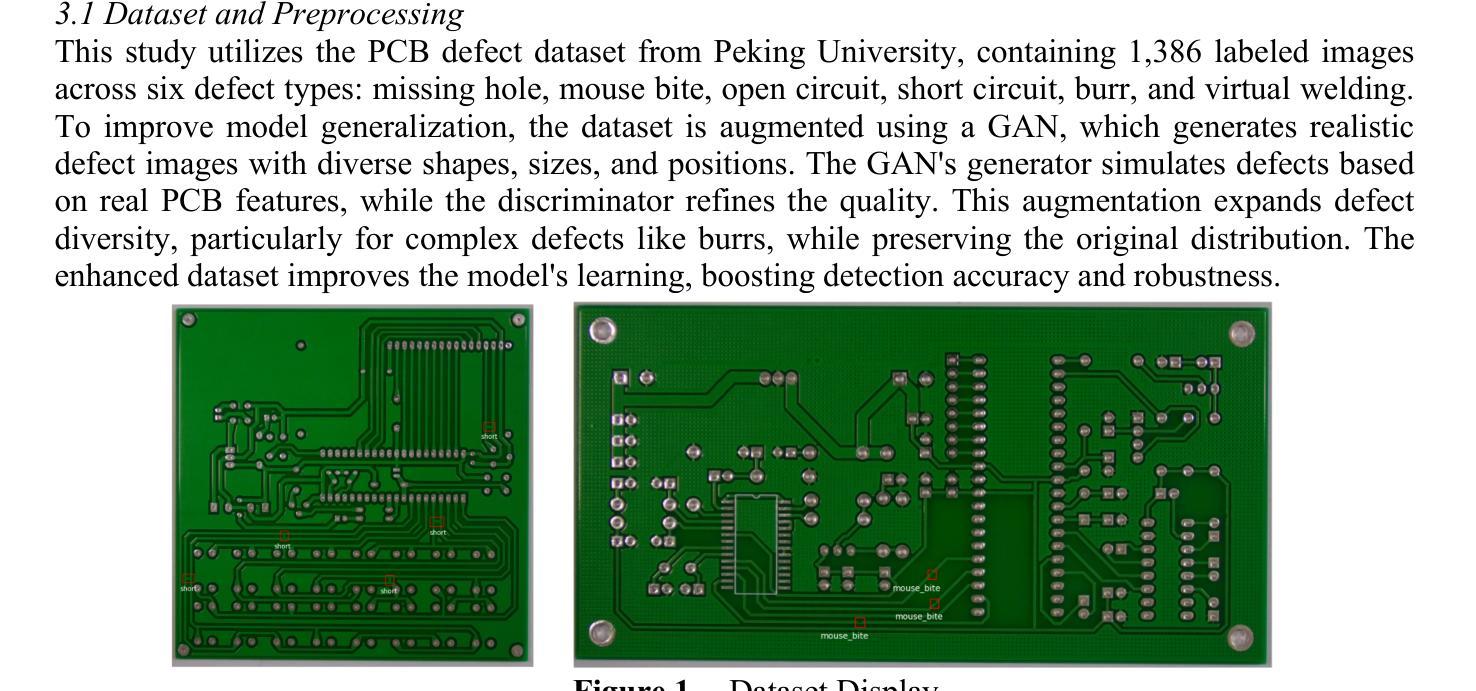
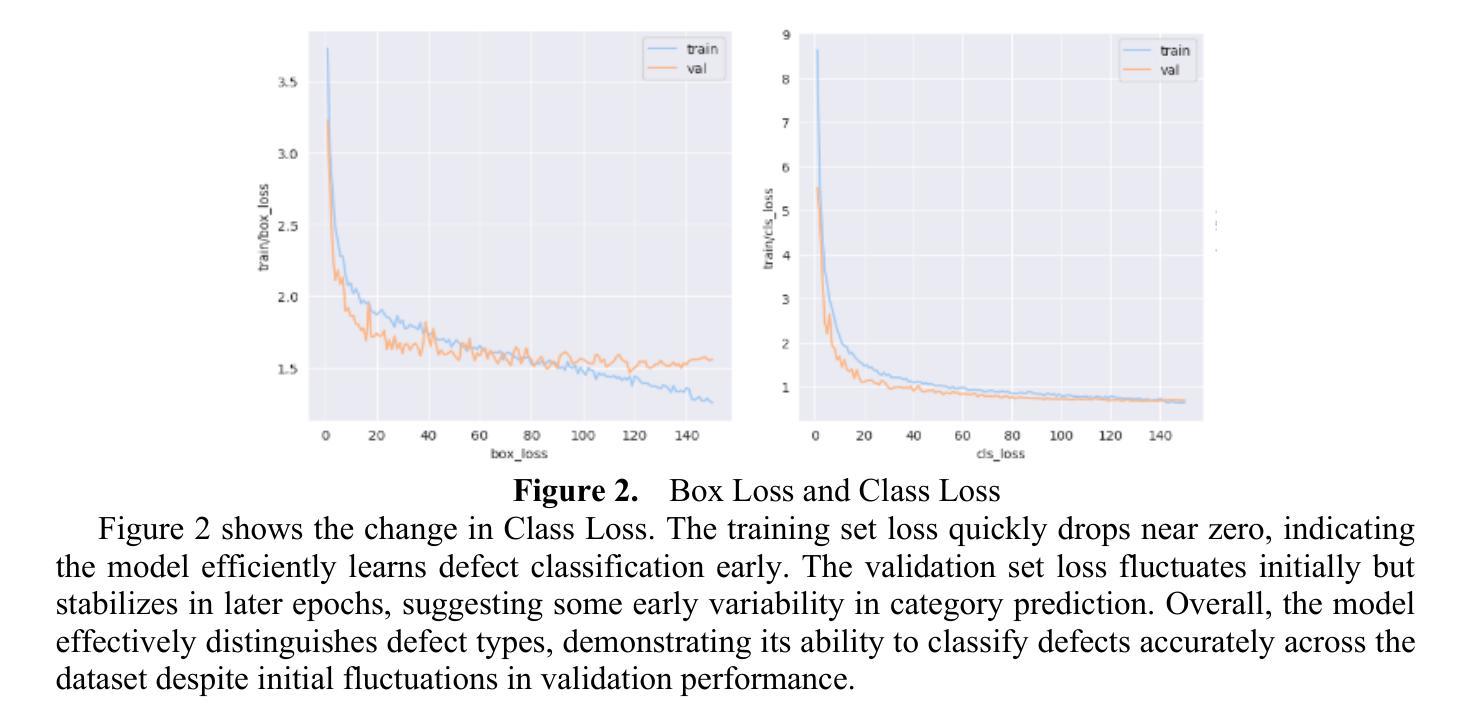
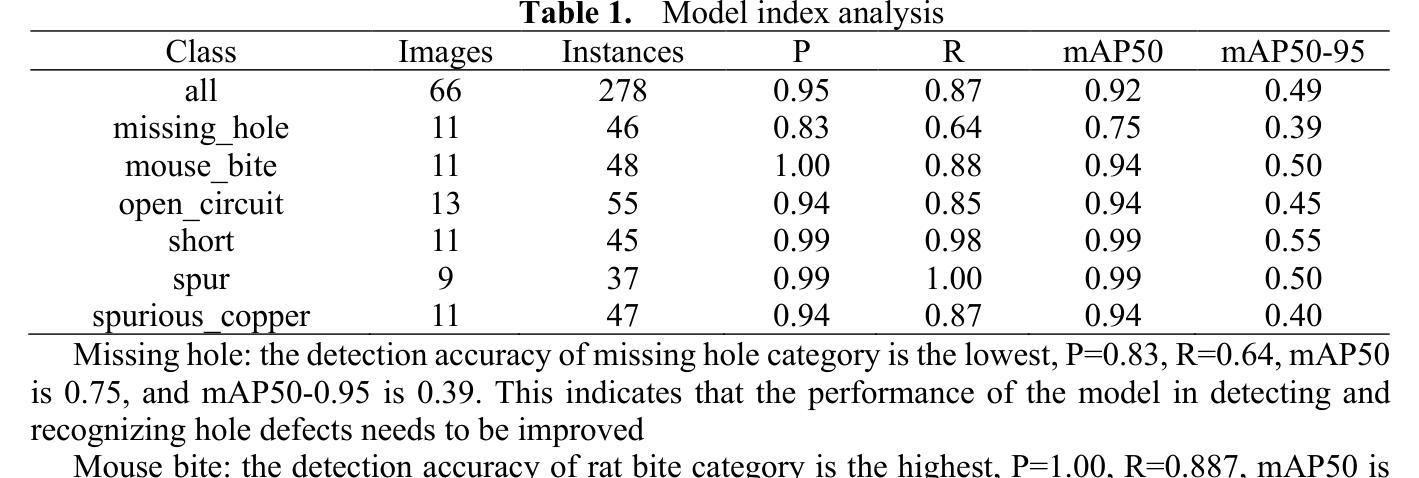
Underwater Image Enhancement using Generative Adversarial Networks: A Survey
Authors:Kancharagunta Kishan Babu, Ashreen Tabassum, Bommakanti Navaneeth, Tenneti Jahnavi, Yenka Akshaya
In recent years, there has been a surge of research focused on underwater image enhancement using Generative Adversarial Networks (GANs), driven by the need to overcome the challenges posed by underwater environments. Issues such as light attenuation, scattering, and color distortion severely degrade the quality of underwater images, limiting their use in critical applications. Generative Adversarial Networks (GANs) have emerged as a powerful tool for enhancing underwater photos due to their ability to learn complex transformations and generate realistic outputs. These advancements have been applied to real-world applications, including marine biology and ecosystem monitoring, coral reef health assessment, underwater archaeology, and autonomous underwater vehicle (AUV) navigation. This paper explores all major approaches to underwater image enhancement, from physical and physics-free models to Convolutional Neural Network (CNN)-based models and state-of-the-art GAN-based methods. It provides a comprehensive analysis of these methods, evaluation metrics, datasets, and loss functions, offering a holistic view of the field. Furthermore, the paper delves into the limitations and challenges faced by current methods, such as generalization issues, high computational demands, and dataset biases, while suggesting potential directions for future research.
近年来,随着水下环境所带来的挑战需要克服,利用生成对抗网络(GANs)进行水下图像增强的研究涌现。水下图像面临光衰减、散射和颜色失真等问题,这些问题严重降低了水下图像的质量,限制了其在关键应用中的使用。生成对抗网络(GANs)因能学习复杂转换并生成逼真的输出,已成为增强水下照片的强大工具。这些进步已应用于现实世界的应用,包括海洋生物学和生态系统监测、珊瑚礁健康评估、水下考古和自主水下车辆(AUV)导航。本文探讨了水下图像增强的所有主要方法,包括物理模型、非物理模型、基于卷积神经网络(CNN)的模型和最新基于GAN的方法。本文全面分析了这些方法、评估指标、数据集和损失函数,为这一领域提供了整体视角。此外,本文还深入探讨了当前方法所面临的局限性和挑战,如泛化问题、高计算需求和数据集偏见,同时提出了未来研究的潜在方向。
论文及项目相关链接
PDF 15 pages, 7 figures, 2 tables
Summary
近年来,针对水下图像增强的研究涌现,利用生成对抗网络(GANs)应对水下环境挑战。光线衰减、散射和颜色失真等问题严重降低水下图像质量,影响其在实际应用中的使用。GANs因能学习复杂转换并产生真实输出,成为增强水下照片的有力工具。该论文探讨了水下图像增强的主要方法,包括物理模型、无物理模型、基于卷积神经网络(CNN)的方法和最先进的基于GAN的方法。论文全面分析了这些方法、评估指标、数据集和损失函数,并深入探讨了当前方法面临的挑战和局限性,如通用化问题、高计算需求和数据集偏见,同时提出了未来研究的可能方向。
Key Takeaways
- 水下图像增强研究受到广泛关注,主要应对水下环境带来的挑战。
- 水下图像面临光线衰减、散射和颜色失真等问题。
- GANs成为增强水下照片的有力工具,能学习复杂转换并产生真实输出。
- 论文全面探讨了水下图像增强的各种方法,包括物理模型、无物理模型、CNN和GAN等方法。
- 当前方法面临通用化问题、高计算需求和数据集偏见等挑战。
- 论文提供了对水下图像增强领域的全面分析,包括评估指标、数据集和损失函数。
点此查看论文截图
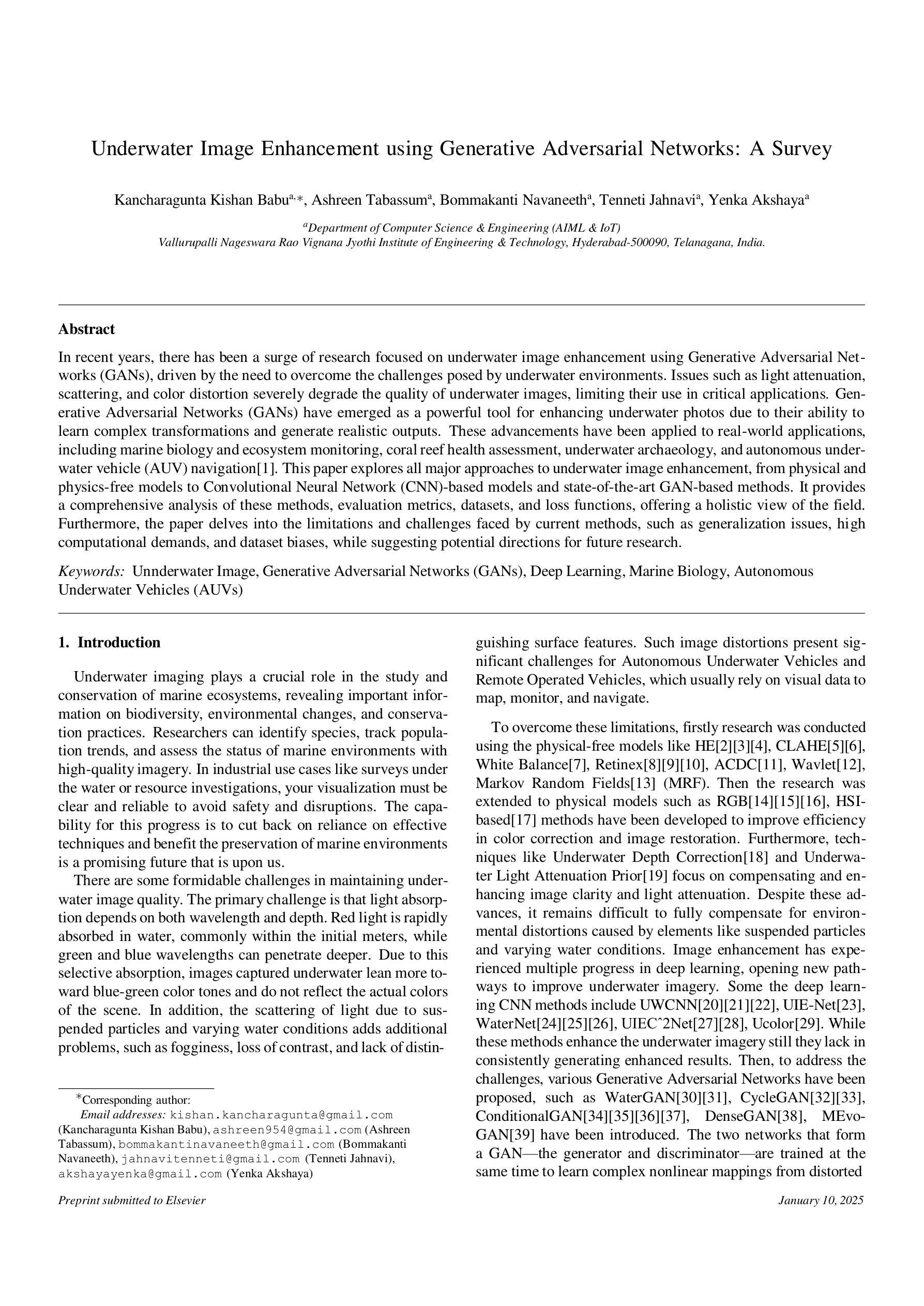
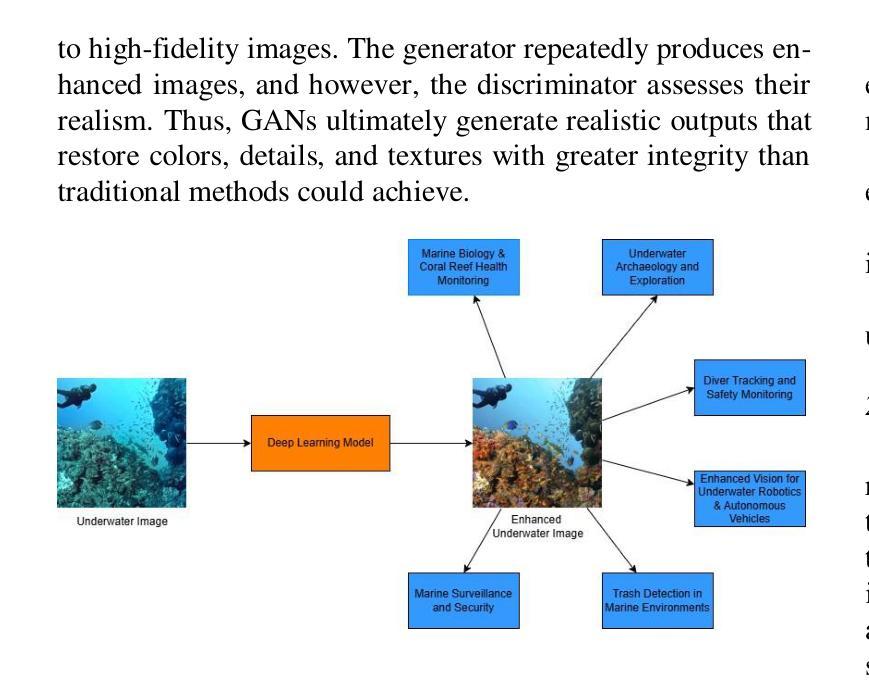
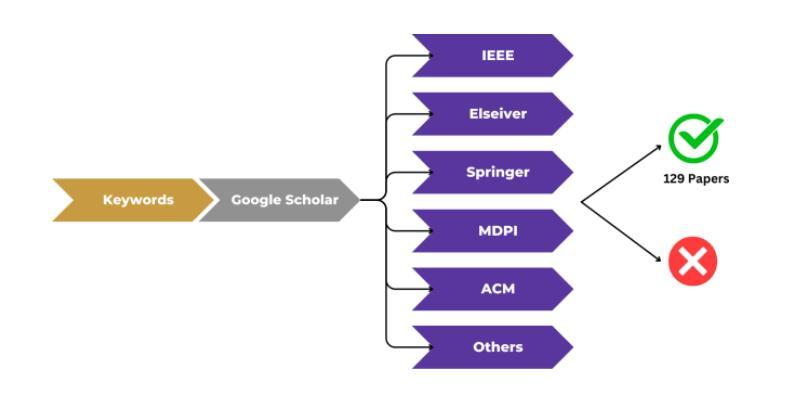
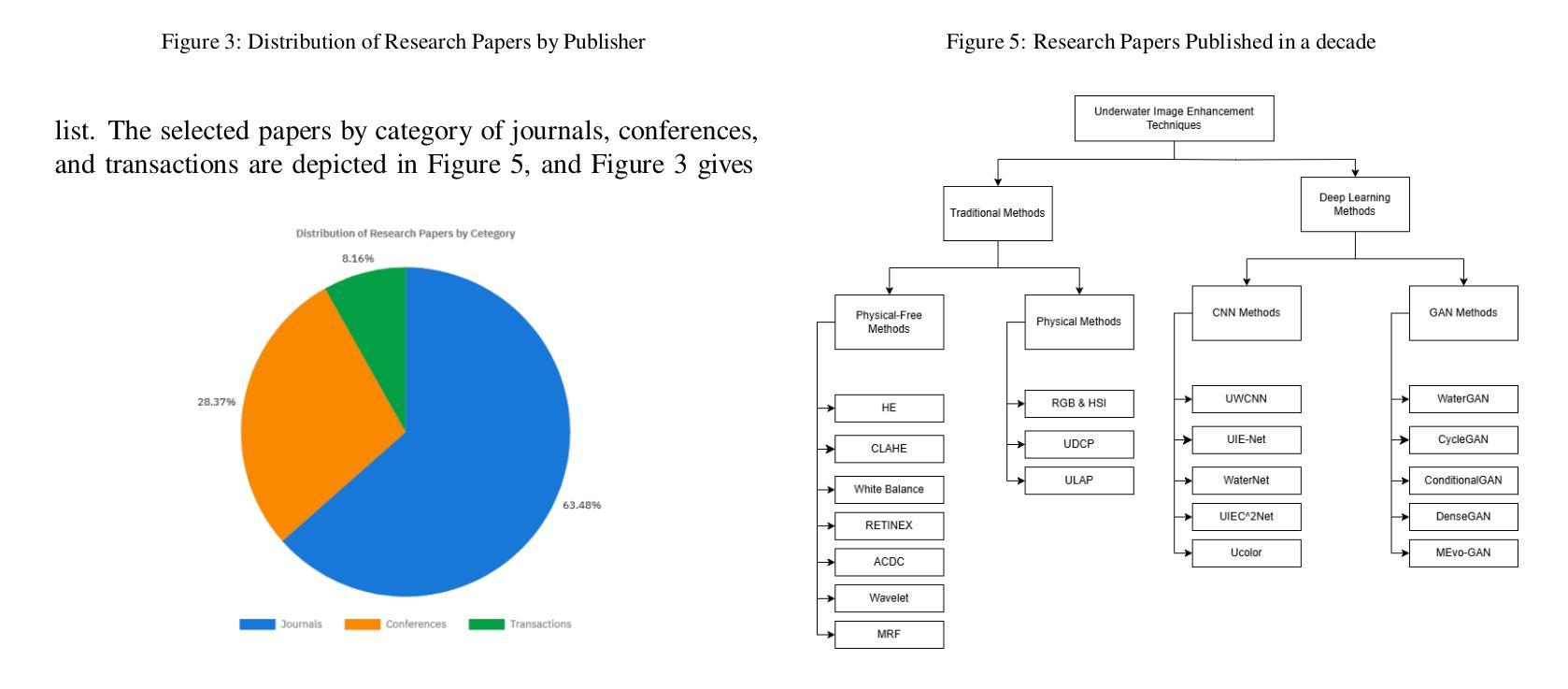
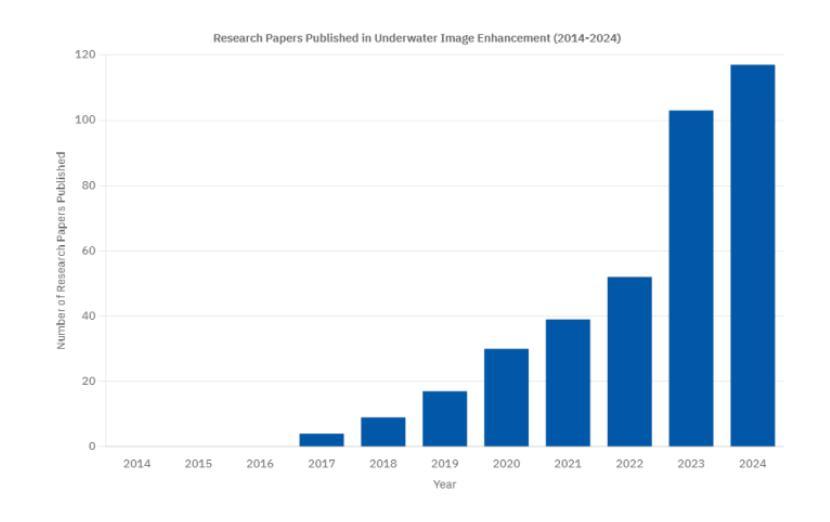
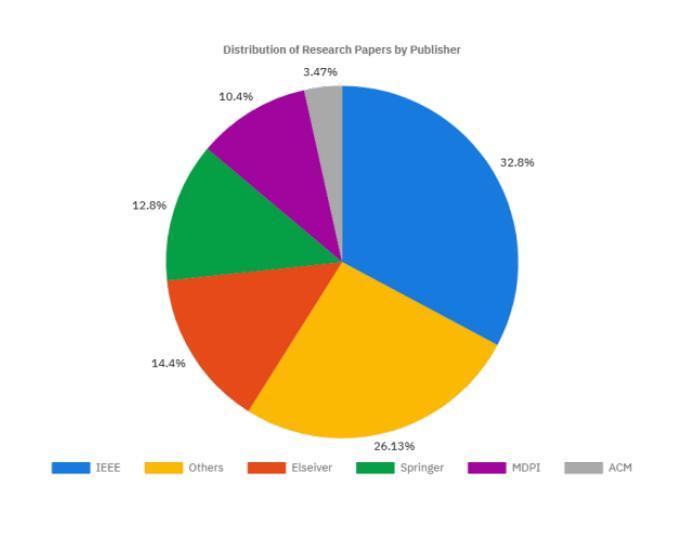
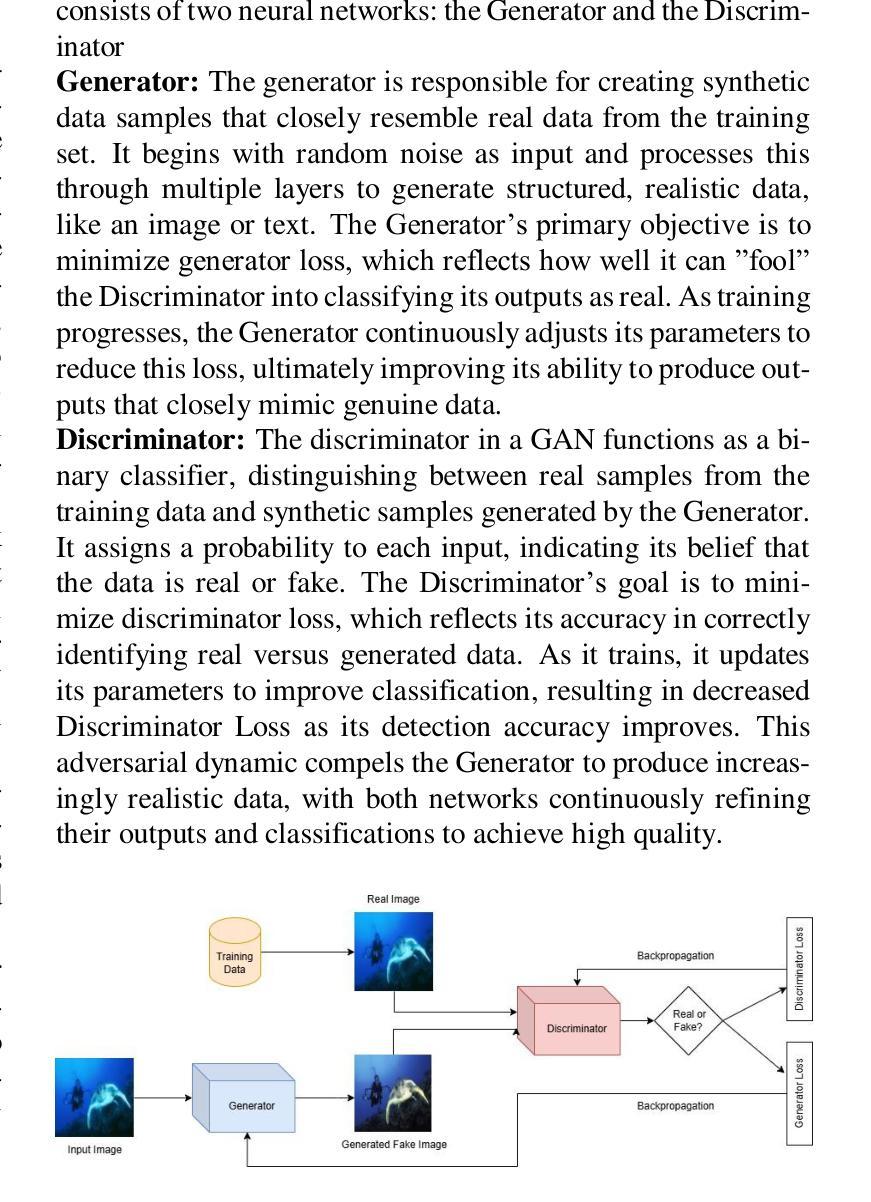
Generating and Detecting Various Types of Fake Image and Audio Content: A Review of Modern Deep Learning Technologies and Tools
Authors:Arash Dehghani, Hossein Saberi
This paper reviews the state-of-the-art in deepfake generation and detection, focusing on modern deep learning technologies and tools based on the latest scientific advancements. The rise of deepfakes, leveraging techniques like Variational Autoencoders (VAEs), Generative Adversarial Networks (GANs), Diffusion models and other generative models, presents significant threats to privacy, security, and democracy. This fake media can deceive individuals, discredit real people and organizations, facilitate blackmail, and even threaten the integrity of legal, political, and social systems. Therefore, finding appropriate solutions to counter the potential threats posed by this technology is essential. We explore various deepfake methods, including face swapping, voice conversion, reenactment and lip synchronization, highlighting their applications in both benign and malicious contexts. The review critically examines the ongoing “arms race” between deepfake generation and detection, analyzing the challenges in identifying manipulated contents. By examining current methods and highlighting future research directions, this paper contributes to a crucial understanding of this rapidly evolving field and the urgent need for robust detection strategies to counter the misuse of this powerful technology. While focusing primarily on audio, image, and video domains, this study allows the reader to easily grasp the latest advancements in deepfake generation and detection.
本文综述了深度伪造生成与检测的最新进展,重点关注基于最新科学进展的现代深度学习技术和工具。利用变分自编码器(VAEs)、生成对抗网络(GANs)、扩散模型和其他生成模型的深度伪造技术崛起,对隐私、安全和民主构成了重大威胁。这种虚假媒体可以欺骗个人,诋毁真实的人物和组织,助长敲诈行为,甚至威胁法律、政治和社会制度的完整性。因此,寻找适当的方法来解决这项技术的潜在威胁至关重要。本文探讨了各种深度伪造方法,包括面部替换、声音转换、重制和唇同步,重点介绍了它们在良性环境和恶意环境下的应用。本文深入分析了深度伪造生成与检测之间不断升级的“军备竞赛”,并分析了识别操纵内容的挑战。通过考察当前的方法并强调未来的研究方向,本文为这一快速演变的领域提供了关键理解,并凸显了迫切需要强大的检测策略来应对这种强大技术的误用。虽然主要关注音频、图像和视频领域,但本研究使读者能够轻松了解深度伪造生成与检测的最新进展。
论文及项目相关链接
Summary
深度伪造技术利用变分自编码器(VAEs)、生成对抗网络(GANs)、扩散模型和其他生成模型等技术给隐私、安全和民主带来严重威胁。本文回顾了深度伪造生成与检测的最新进展,深入探讨了识别操纵内容所面临的挑战,并强调了对抗误用这一强大技术的稳健检测策略的重要性。
Key Takeaways
- 深度伪造技术运用现代深度学习和科学进步为基础的工具进行生成,如Variational Autoencoders (VAEs) 和 Generative Adversarial Networks (GANs)。
- 深度伪造技术存在显著威胁个人隐私、安全和民主。
- 深度伪造技术可用于良性及恶意场合,包括身份冒充、语音转换、重演和唇同步等应用。
- 当前面临挑战在于识别操纵内容,存在一场深度伪造生成与检测之间的“军备竞赛”。
- 急需稳健的检测策略来对抗深度伪造技术的误用。
- 本文不仅聚焦于音频、图像和视频领域,还使读者易于掌握深度伪造生成与检测的最新进展。
点此查看论文截图
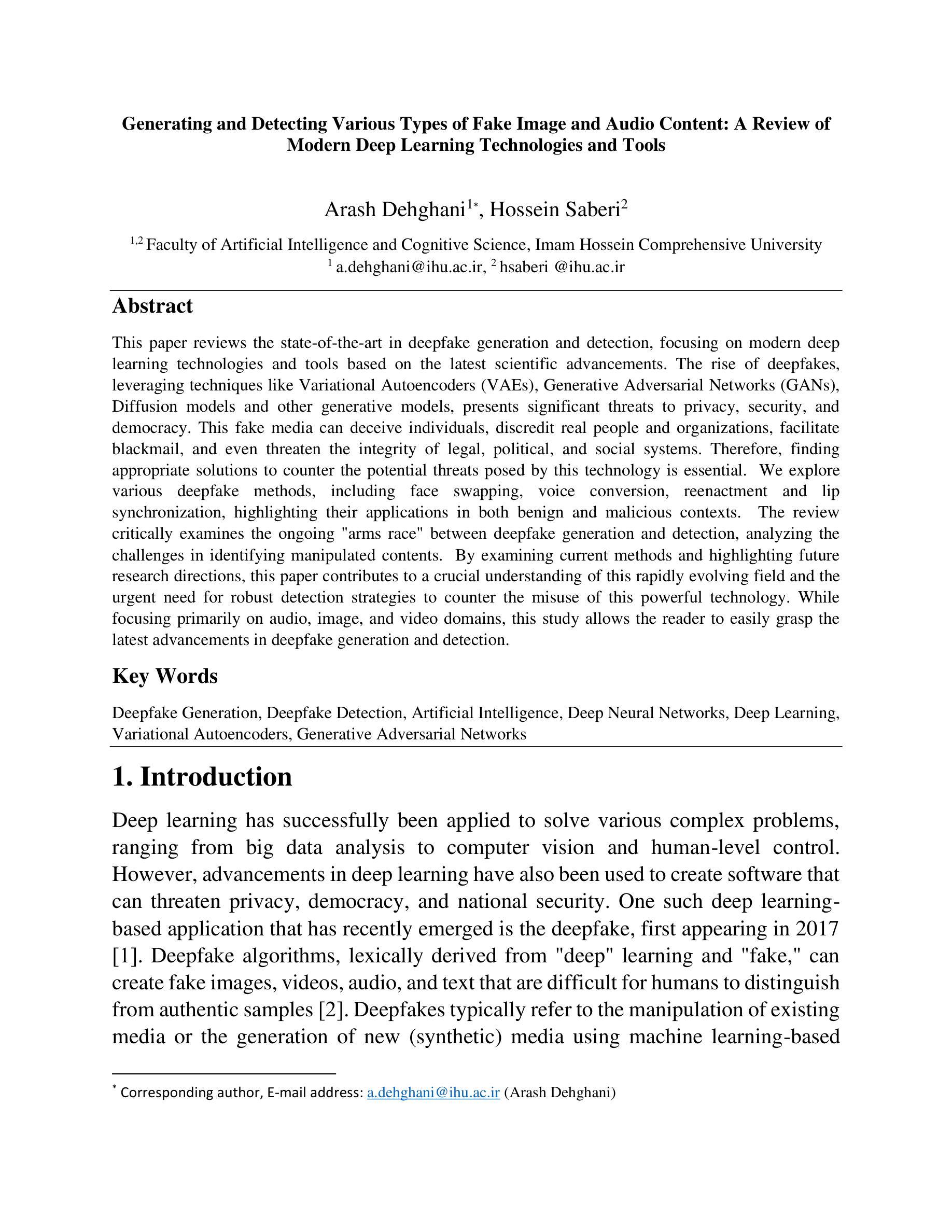