⚠️ 以下所有内容总结都来自于 大语言模型的能力,如有错误,仅供参考,谨慎使用
🔴 请注意:千万不要用于严肃的学术场景,只能用于论文阅读前的初筛!
💗 如果您觉得我们的项目对您有帮助 ChatPaperFree ,还请您给我们一些鼓励!⭐️ HuggingFace免费体验
2025-01-17 更新
DynamicFace: High-Quality and Consistent Video Face Swapping using Composable 3D Facial Priors
Authors:Runqi Wang, Sijie Xu, Tianyao He, Yang Chen, Wei Zhu, Dejia Song, Nemo Chen, Xu Tang, Yao Hu
Face swapping transfers the identity of a source face to a target face while retaining the attributes like expression, pose, hair, and background of the target face. Advanced face swapping methods have achieved attractive results. However, these methods often inadvertently transfer identity information from the target face, compromising expression-related details and accurate identity. We propose a novel method DynamicFace that leverages the power of diffusion model and plug-and-play temporal layers for video face swapping. First, we introduce four fine-grained face conditions using 3D facial priors. All conditions are designed to be disentangled from each other for precise and unique control. Then, we adopt Face Former and ReferenceNet for high-level and detailed identity injection. Through experiments on the FF++ dataset, we demonstrate that our method achieves state-of-the-art results in face swapping, showcasing superior image quality, identity preservation, and expression accuracy. Besides, our method could be easily transferred to video domain with temporal attention layer. Our code and results will be available on the project page: https://dynamic-face.github.io/
面部替换技术将源面部的身份转移到目标面部,同时保留目标面部的表情、姿态、发型和背景等属性。先进的面部替换方法已经取得了吸引人的效果。然而,这些方法常常会在不经意间从目标面部转移身份信息,损失表情相关的细节和准确的身份。我们提出了一种新型方法DynamicFace,它利用扩散模型和即插即用的时间层进行视频面部替换。首先,我们利用3D面部先验知识,引入了四种精细的面部条件。所有条件都被设计成相互独立,以实现精确和独特的控制。然后,我们采用Face Former和ReferenceNet进行高级和详细的身份注入。在FF++数据集上的实验表明,我们的方法在面部替换方面达到了最新水平,显示出卓越的图片质量、身份保留和表情准确性。此外,我们的方法可以通过时间注意力层轻松转移到视频领域。我们的代码和结果将在项目页面公布:https://dynamic-face.github.io/
论文及项目相关链接
Summary
本文介绍了一种基于扩散模型和插拨式时间层技术的新型动态面部替换方法。该方法利用精细化的三维面部先验,引入了四种精细面部条件,通过Face Former和ReferenceNet进行高级和详细的身份注入。实验证明,该方法在面部替换方面达到最新技术水平,具有出色的图像质量、身份保留和表情准确性。此外,该方法可轻松应用于视频领域,具有时间注意力层。
Key Takeaways
- 介绍了基于扩散模型和插拨式时间层技术的动态面部替换新方法。
- 使用三维面部先验引入四种精细面部条件,实现精准独特的控制。
- 采用Face Former和ReferenceNet进行高级和详细的身份注入。
- 在FF++数据集上的实验证明该方法在面部替换方面达到最新技术水平。
- 该方法具有出色的图像质量、身份保留和表情准确性。
- 方法可轻松应用于视频领域,具有时间注意力层。
点此查看论文截图
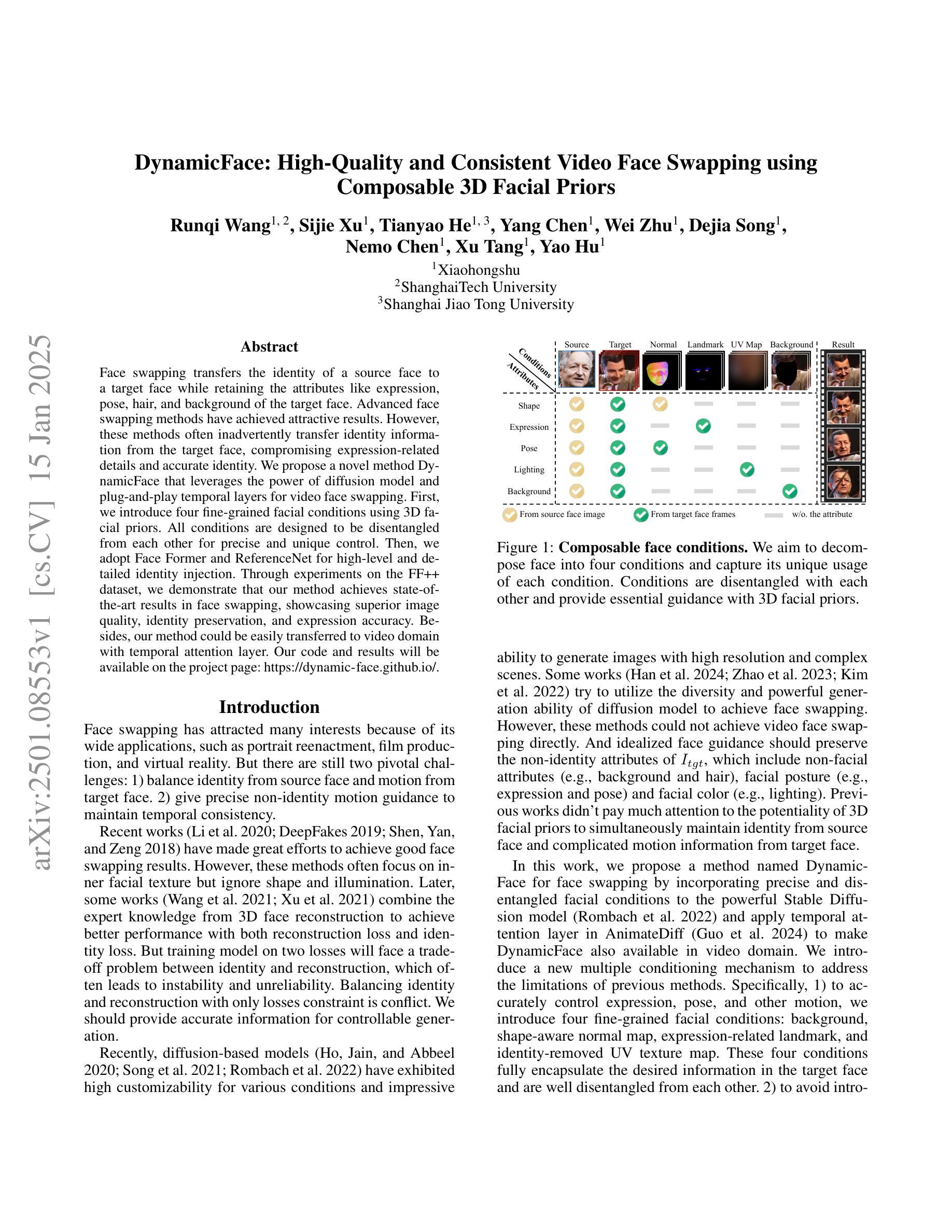
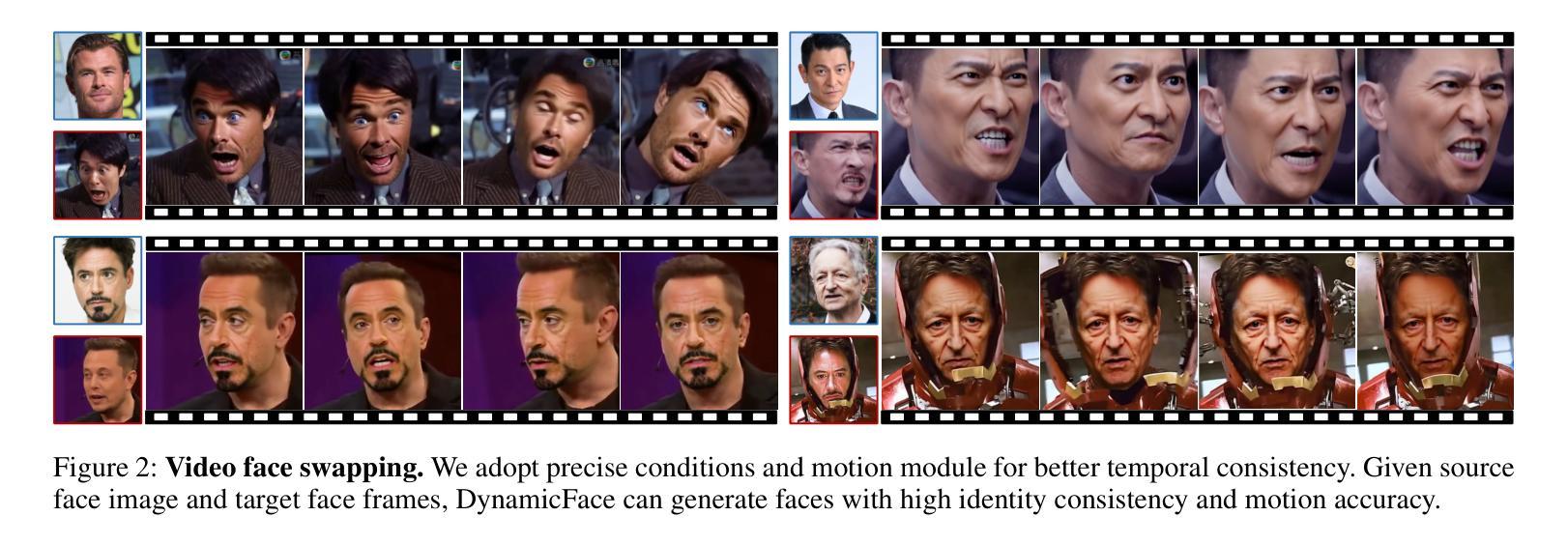
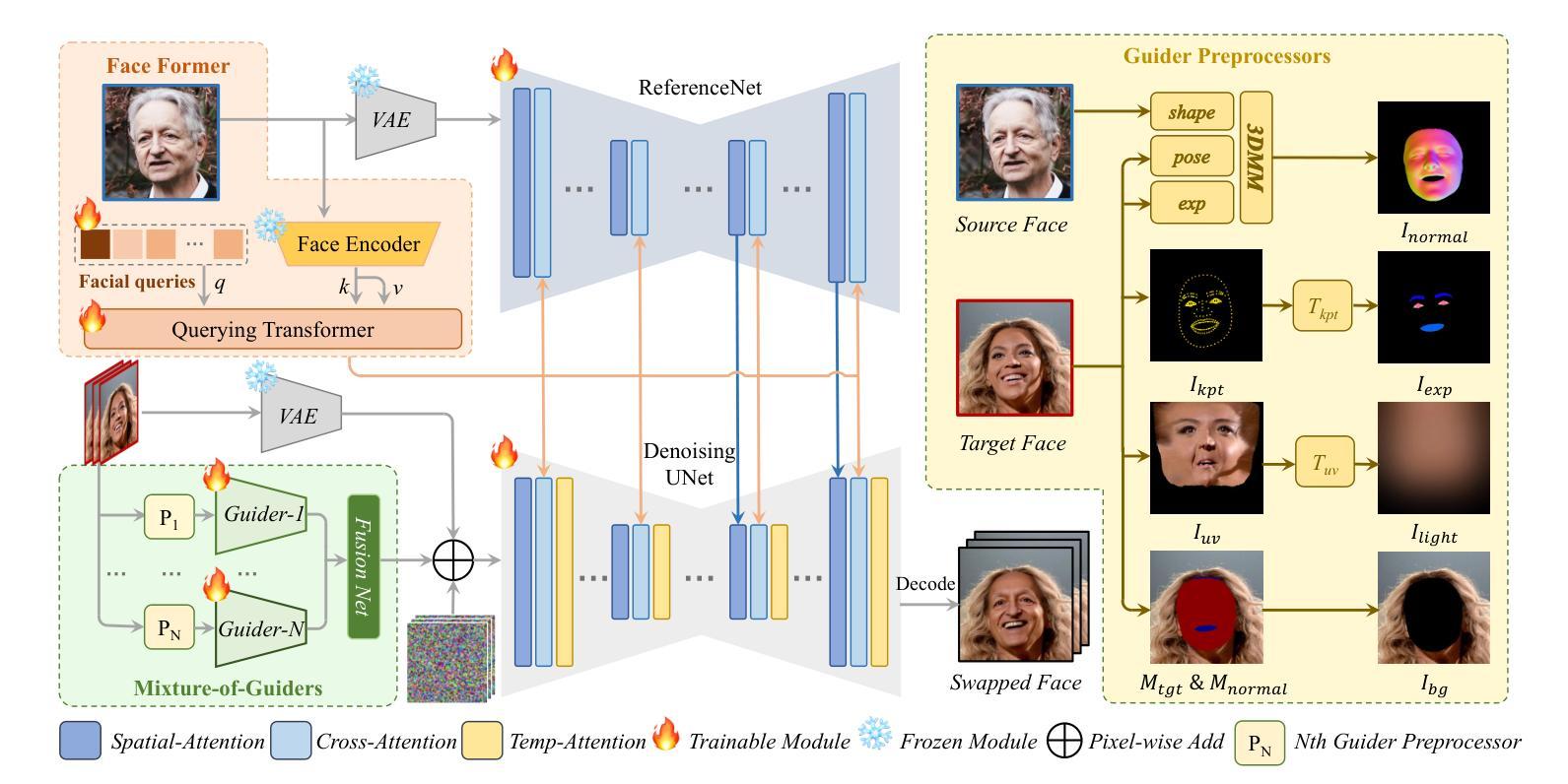
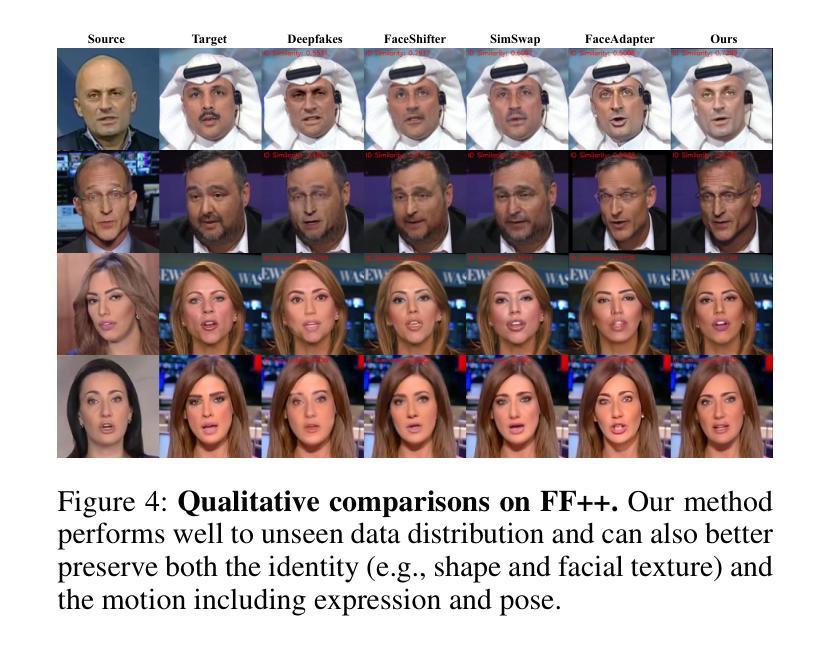
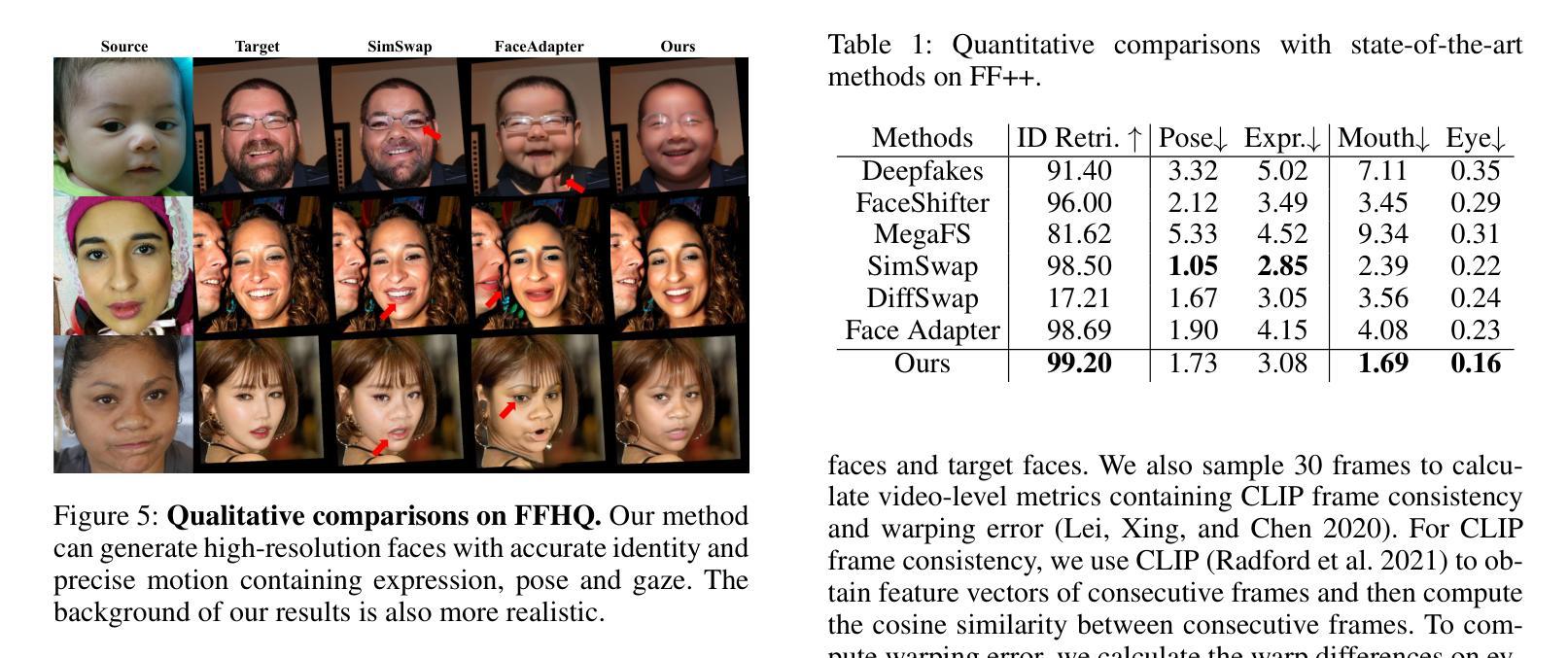