⚠️ 以下所有内容总结都来自于 大语言模型的能力,如有错误,仅供参考,谨慎使用
🔴 请注意:千万不要用于严肃的学术场景,只能用于论文阅读前的初筛!
💗 如果您觉得我们的项目对您有帮助 ChatPaperFree ,还请您给我们一些鼓励!⭐️ HuggingFace免费体验
2025-01-17 更新
Enhanced Multi-Scale Cross-Attention for Person Image Generation
Authors:Hao Tang, Ling Shao, Nicu Sebe, Luc Van Gool
In this paper, we propose a novel cross-attention-based generative adversarial network (GAN) for the challenging person image generation task. Cross-attention is a novel and intuitive multi-modal fusion method in which an attention/correlation matrix is calculated between two feature maps of different modalities. Specifically, we propose the novel XingGAN (or CrossingGAN), which consists of two generation branches that capture the person’s appearance and shape, respectively. Moreover, we propose two novel cross-attention blocks to effectively transfer and update the person’s shape and appearance embeddings for mutual improvement. This has not been considered by any other existing GAN-based image generation work. To further learn the long-range correlations between different person poses at different scales and sub-regions, we propose two novel multi-scale cross-attention blocks. To tackle the issue of independent correlation computations within the cross-attention mechanism leading to noisy and ambiguous attention weights, which hinder performance improvements, we propose a module called enhanced attention (EA). Lastly, we introduce a novel densely connected co-attention module to fuse appearance and shape features at different stages effectively. Extensive experiments on two public datasets demonstrate that the proposed method outperforms current GAN-based methods and performs on par with diffusion-based methods. However, our method is significantly faster than diffusion-based methods in both training and inference.
在这篇论文中,我们针对人物图像生成这一具有挑战性的任务,提出了一种基于交叉注意力的生成对抗网络(GAN)。交叉注意力是一种新颖且直观的多模态融合方法,该方法计算两个不同模态的特征图之间的注意力/关联矩阵。具体来说,我们提出了新型XingGAN(或CrossingGAN),它包括两个生成分支,分别捕捉人物的外观和形状。此外,我们还提出了两个新颖的交叉注意力块,以有效地转移和更新人物的形状和外观嵌入,以实现相互改进。这在其他现有的基于GAN的图像生成工作中尚未被考虑。为了进一步学习不同尺度和不同子区域中不同人物姿态之间的长期关联,我们提出了两种新型多尺度交叉注意力块。为了解决交叉注意力机制中独立关联计算导致噪声和模糊注意力权重的问题,这些问题阻碍了性能的提高,我们提出了一种名为增强注意力(EA)的模块。最后,我们引入了一种新型密集连接的协同注意力模块,以有效地融合不同阶段的外观和形状特征。在两个公共数据集上的大量实验表明,所提出的方法优于当前的基于GAN的方法,并与基于扩散的方法表现相当。然而,我们的方法在训练和推理方面都显著快于基于扩散的方法。
论文及项目相关链接
PDF Accepted to TPAMI, an extended version of a paper published in ECCV2020. arXiv admin note: substantial text overlap with arXiv:2007.09278
Summary
在本文中,我们提出了一种基于交叉注意力机制的新型生成对抗网络(GAN),用于解决人物图像生成这一挑战性问题。我们引入了交叉注意力这一新颖直观的多模态融合方法,并在其中设计了两个生成分支来分别捕捉人物的外观和形状特征。此外,我们还提出了两个新颖的交叉注意力块,以有效地转移和更新人物的形状和外观嵌入,实现相互改进。为解决独立相关计算带来的噪声和不明确注意力权重问题,我们引入了增强注意力模块。最后,我们介绍了一种新的密集连接共注意力模块,以有效融合不同阶段的外观和形状特征。在公共数据集上的广泛实验表明,该方法优于当前的GAN方法,与扩散方法表现相当,但在训练和推理速度上显著快于扩散方法。
Key Takeaways
- 提出了基于交叉注意力机制的新型GAN,针对人物图像生成具有挑战性任务进行优化。
- 设计了XingGAN(或CrossingGAN),包含两个生成分支分别捕捉人物外观和形状特征。
- 引入两个新颖的交叉注意力块,实现形状和外观嵌入的转移和更新,促进相互改进。
- 针对独立相关计算带来的问题,提出增强注意力模块以改善噪声和不明确的注意力权重。
- 为融合不同阶段的外观和形状特征,引入新的密集连接共注意力模块。
- 在公共数据集上的实验表明,该方法在性能上优于现有GAN方法,与扩散方法相当。
点此查看论文截图
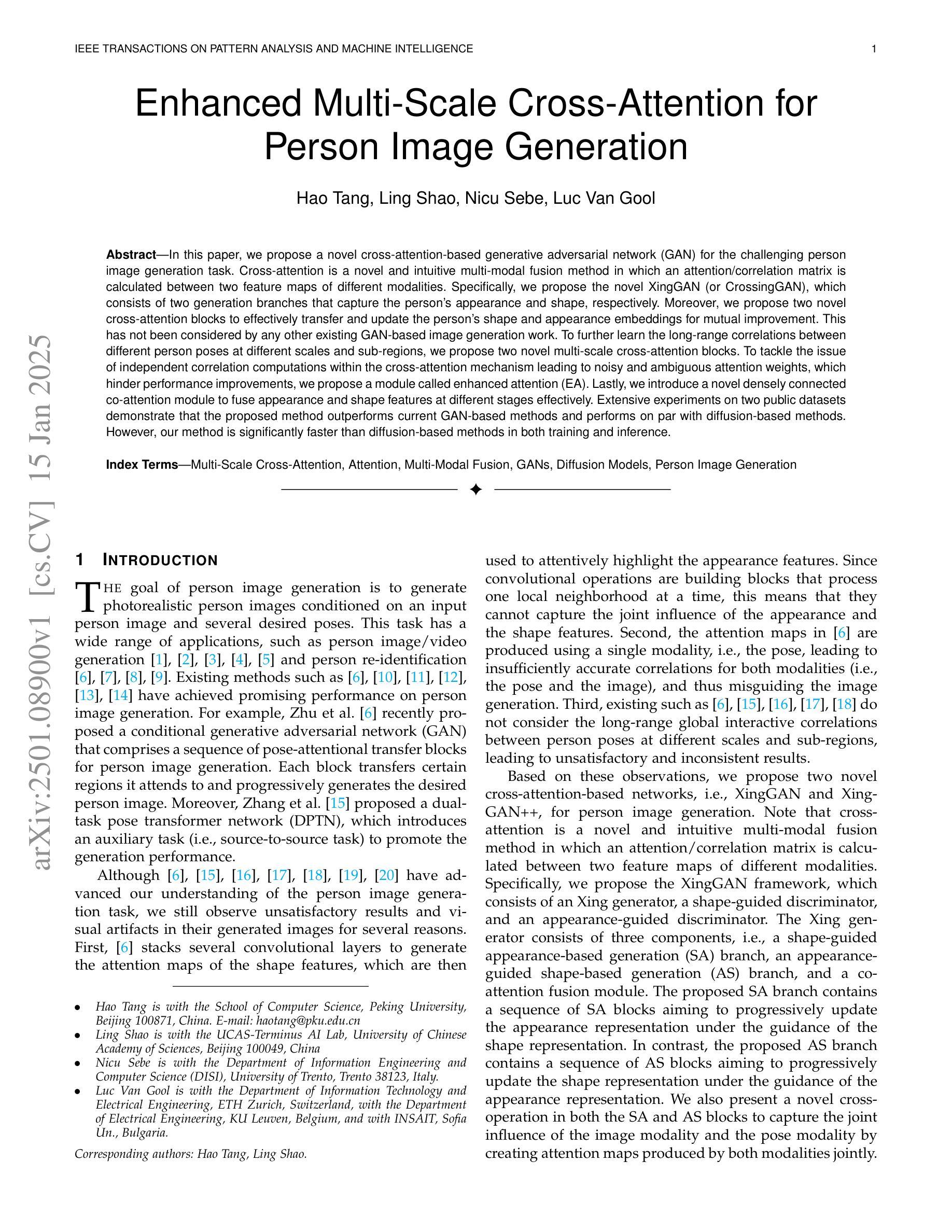
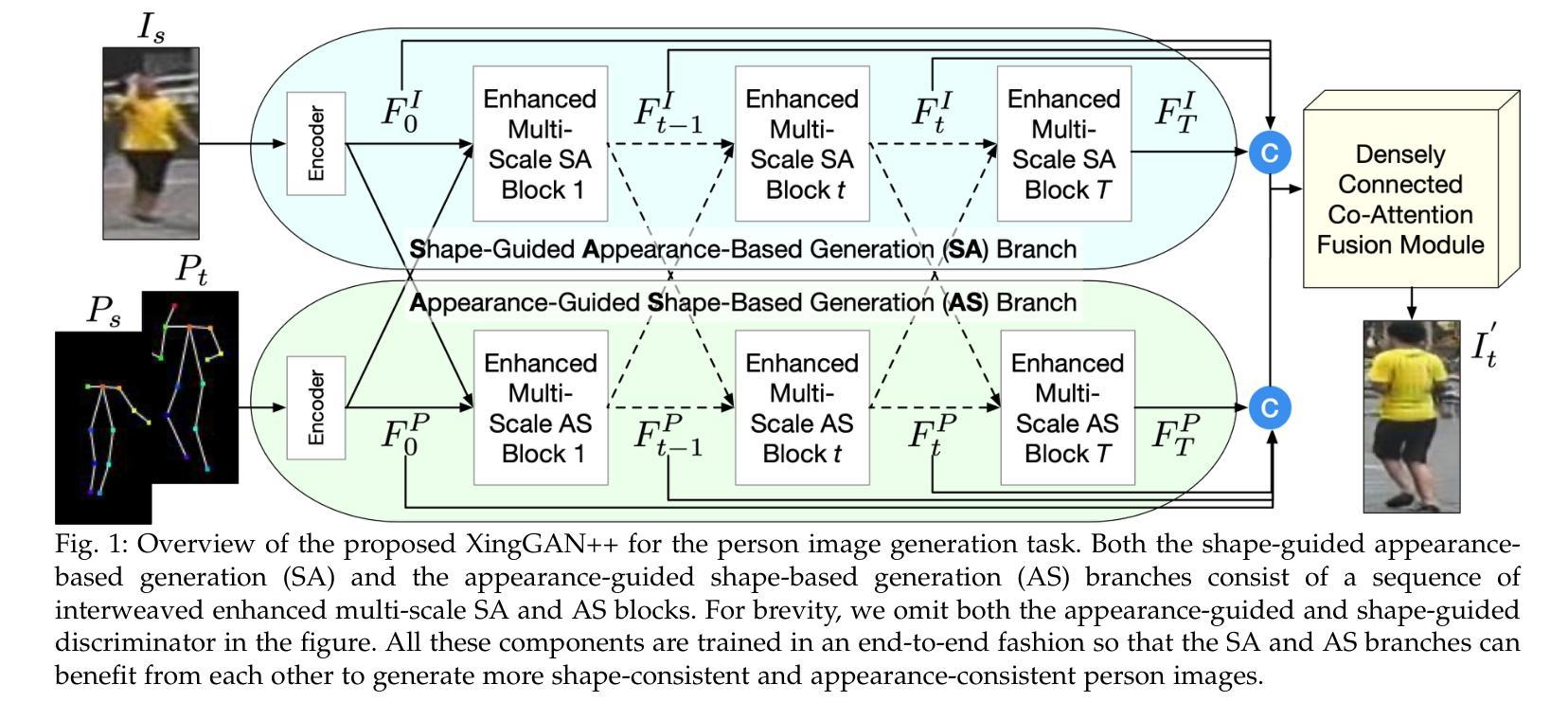
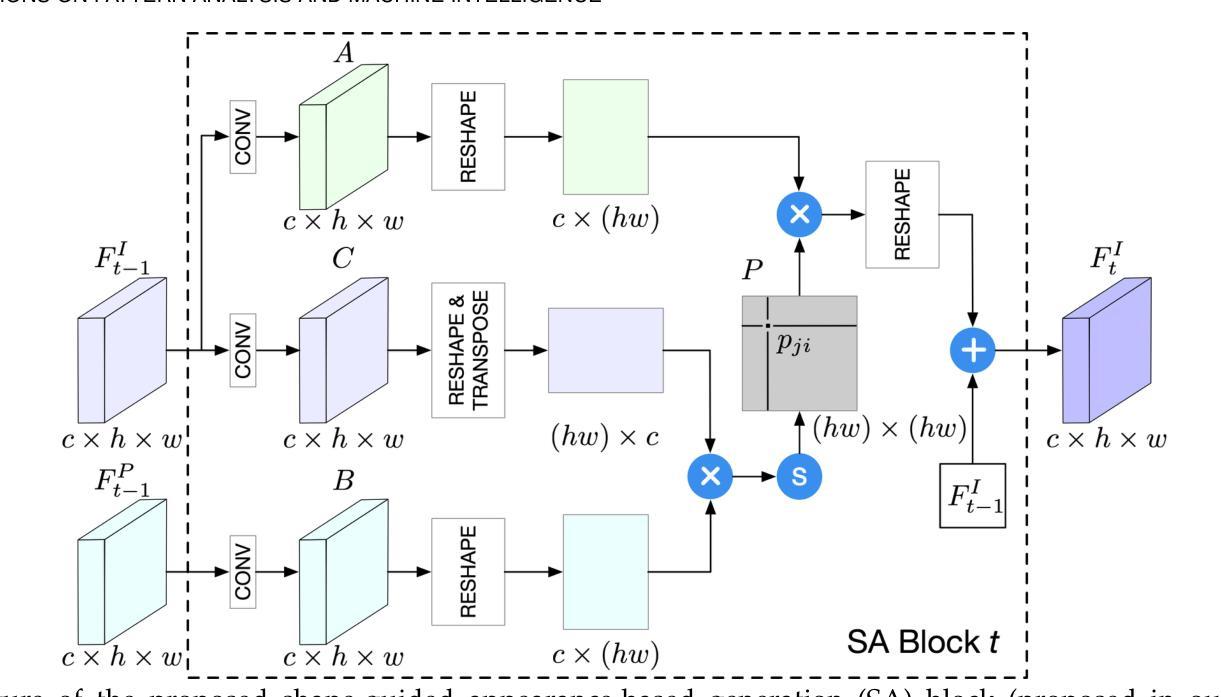
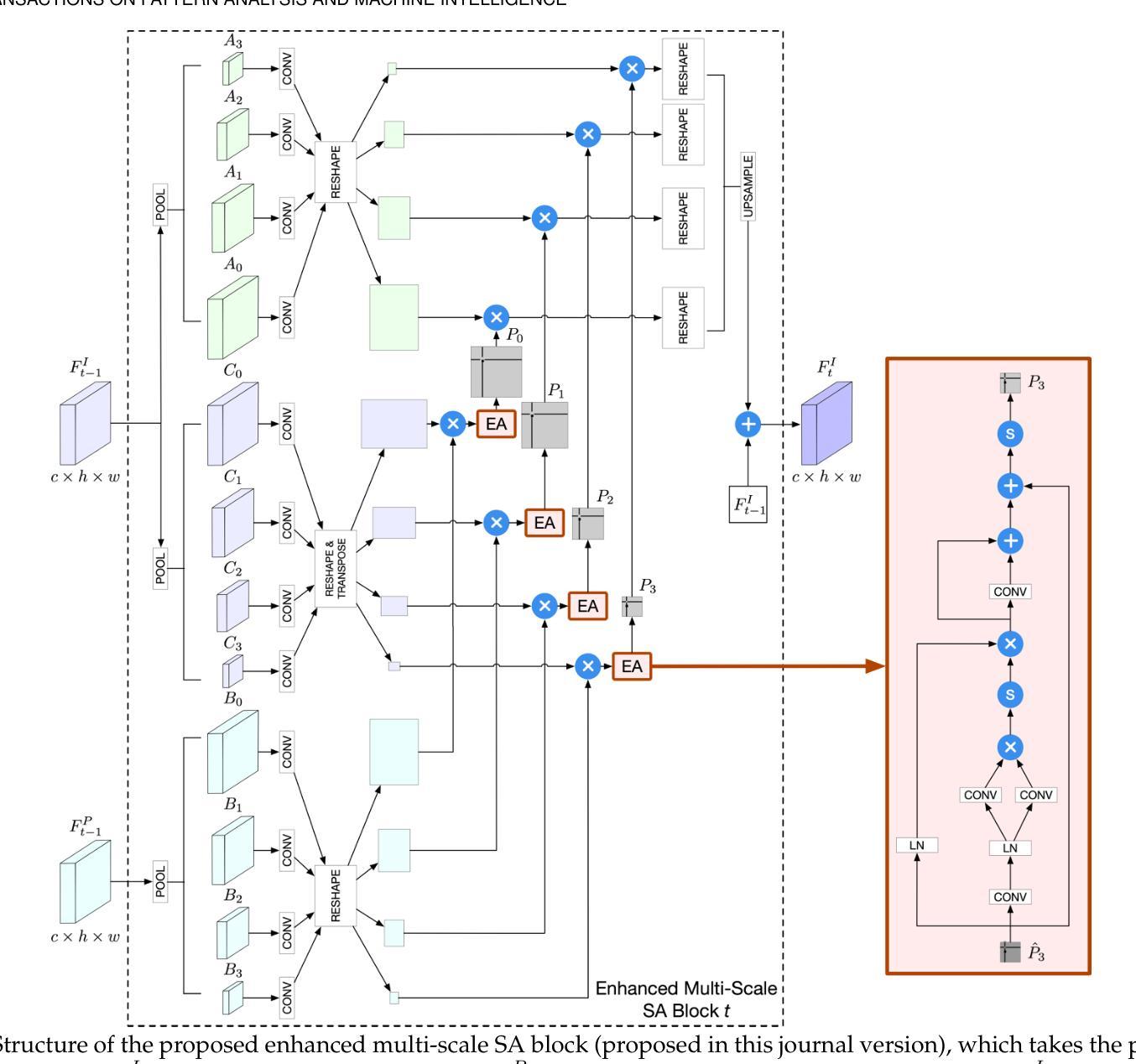