⚠️ 以下所有内容总结都来自于 大语言模型的能力,如有错误,仅供参考,谨慎使用
🔴 请注意:千万不要用于严肃的学术场景,只能用于论文阅读前的初筛!
💗 如果您觉得我们的项目对您有帮助 ChatPaperFree ,还请您给我们一些鼓励!⭐️ HuggingFace免费体验
2025-01-18 更新
WMamba: Wavelet-based Mamba for Face Forgery Detection
Authors:Siran Peng, Tianshuo Zhang, Li Gao, Xiangyu Zhu, Haoyuan Zhang, Kai Pang, Zhen Lei
With the rapid advancement of deepfake generation technologies, the demand for robust and accurate face forgery detection algorithms has become increasingly critical. Recent studies have demonstrated that wavelet analysis can uncover subtle forgery artifacts that remain imperceptible in the spatial domain. Wavelets effectively capture important facial contours, which are often slender, fine-grained, and global in nature. However, existing wavelet-based approaches fail to fully leverage these unique characteristics, resulting in sub-optimal feature extraction and limited generalizability. To address this challenge, we introduce WMamba, a novel wavelet-based feature extractor built upon the Mamba architecture. WMamba maximizes the utility of wavelet information through two key innovations. First, we propose Dynamic Contour Convolution (DCConv), which employs specially crafted deformable kernels to adaptively model slender facial contours. Second, by leveraging the Mamba architecture, our method captures long-range spatial relationships with linear computational complexity. This efficiency allows for the extraction of fine-grained, global forgery artifacts from small image patches. Extensive experimental results show that WMamba achieves state-of-the-art (SOTA) performance, highlighting its effectiveness and superiority in face forgery detection.
随着深度伪造生成技术的快速发展,对稳健、准确的面部伪造检测算法的需求变得日益关键。最近的研究表明,小波分析可以揭示在空域中无法察觉的微妙伪造痕迹。小波能够有效地捕捉重要的面部轮廓,这些轮廓通常是细长、精细且全局性的。然而,现有的基于小波的方法未能充分利用这些独特特征,导致特征提取效果不佳和通用性有限。为了解决这一挑战,我们引入了WMamba,这是一种基于Mamba架构的新型小波特征提取器。WMamba通过两个关键创新最大限度地提高了小波信息的效用。首先,我们提出了动态轮廓卷积(DCConv),它采用专门设计的可变形内核来自适应地模拟细长的面部轮廓。其次,通过利用Mamba架构,我们的方法以线性计算复杂度捕获远程空间关系。这种效率允许从小图像块中提取精细、全局的伪造痕迹。大量实验结果表明,WMamba达到了最新技术水平,凸显了其在面部伪造检测中的有效性和优越性。
论文及项目相关链接
Summary
随着深度伪造技术快速发展,对鲁棒、精准的人脸伪造检测算法的需求日益迫切。近期研究表明,小波分析能揭示在空间中无法察觉的伪造痕迹。本文提出WMamba,一种基于小波和Mamba架构的特征提取器,通过两个关键创新——动态轮廓卷积(DCConv)和利用Mamba架构实现的长程空间关系捕捉,最大化小波信息的使用效率。实验结果显示,WMamba在人脸伪造检测方面达到最新技术水平,展现出其高效性和优越性。
Key Takeaways
- 深度伪造技术的快速发展增加了对鲁棒人脸伪造检测算法的需求。
- 小波分析能揭示伪造痕迹。
- WMamba是一种基于小波和Mamba架构的特征提取器。
- WMamba通过动态轮廓卷积(DCConv)和长程空间关系捕捉两个关键创新来最大化小波信息的使用效率。
- 动态轮廓卷积(DCConv)采用可变形内核来适应面部轮廓。
- 利用Mamba架构,WMamba具有线性计算复杂度,能捕捉长程空间关系。
点此查看论文截图
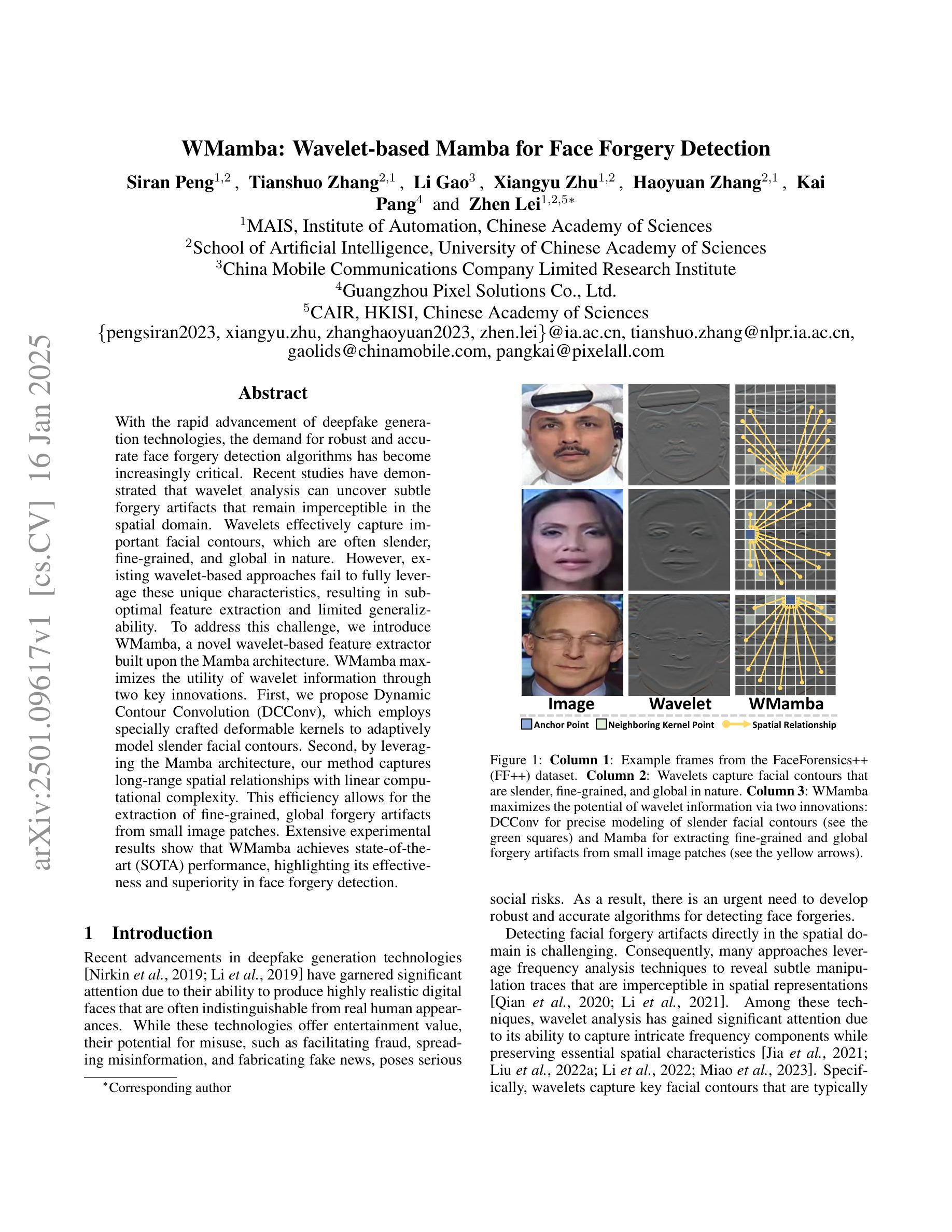
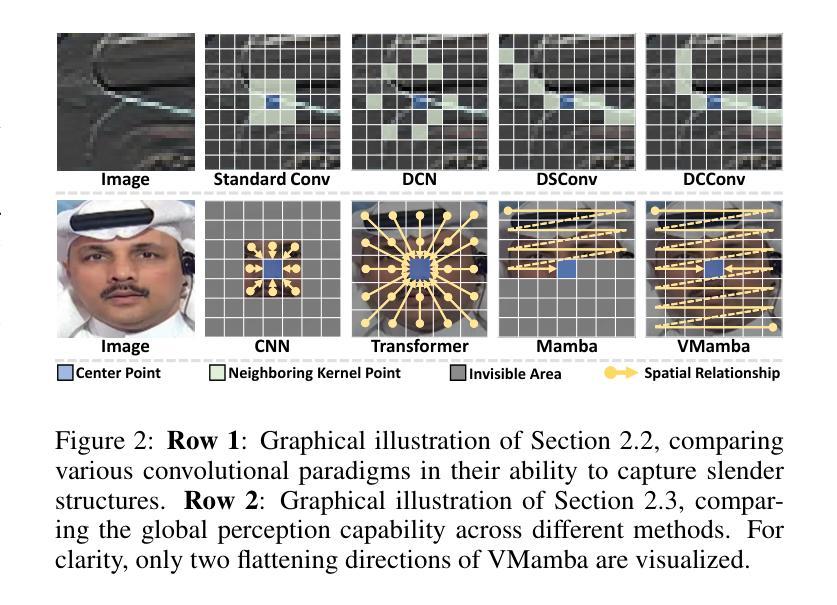
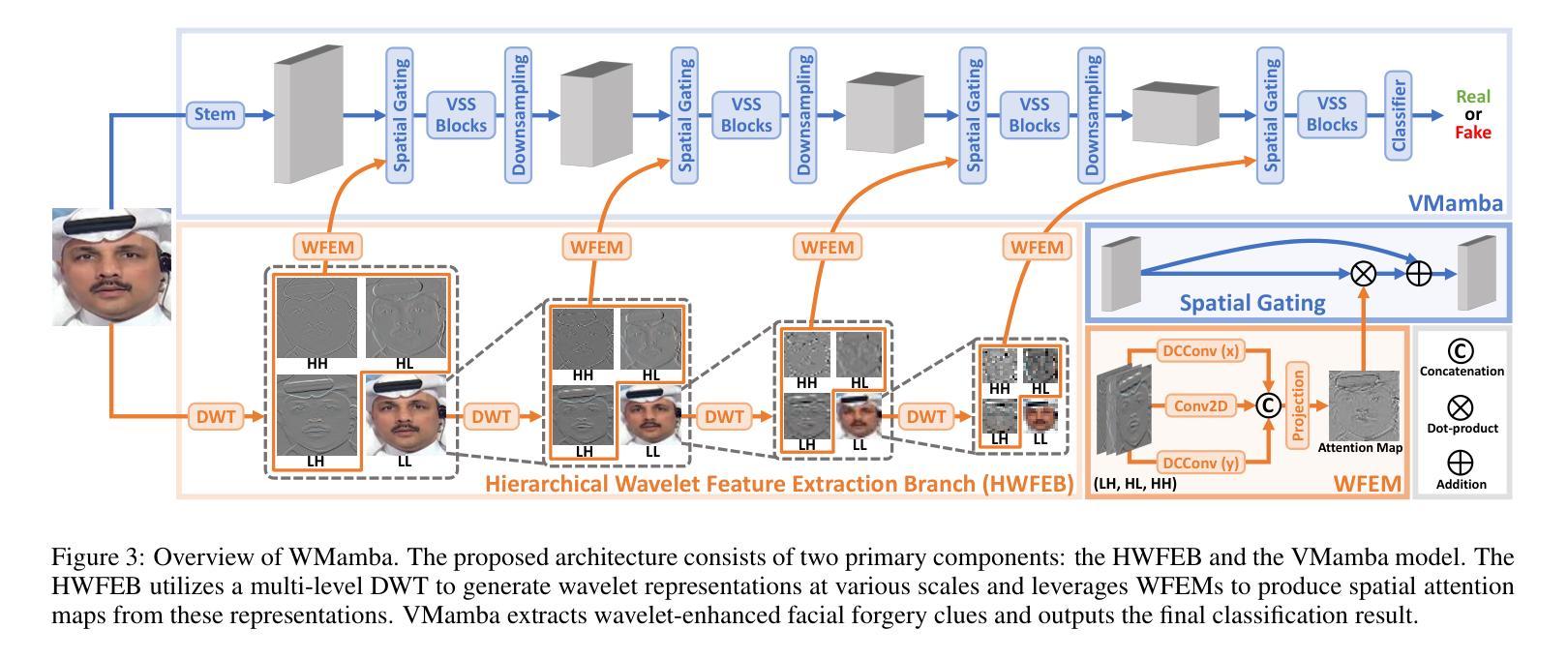
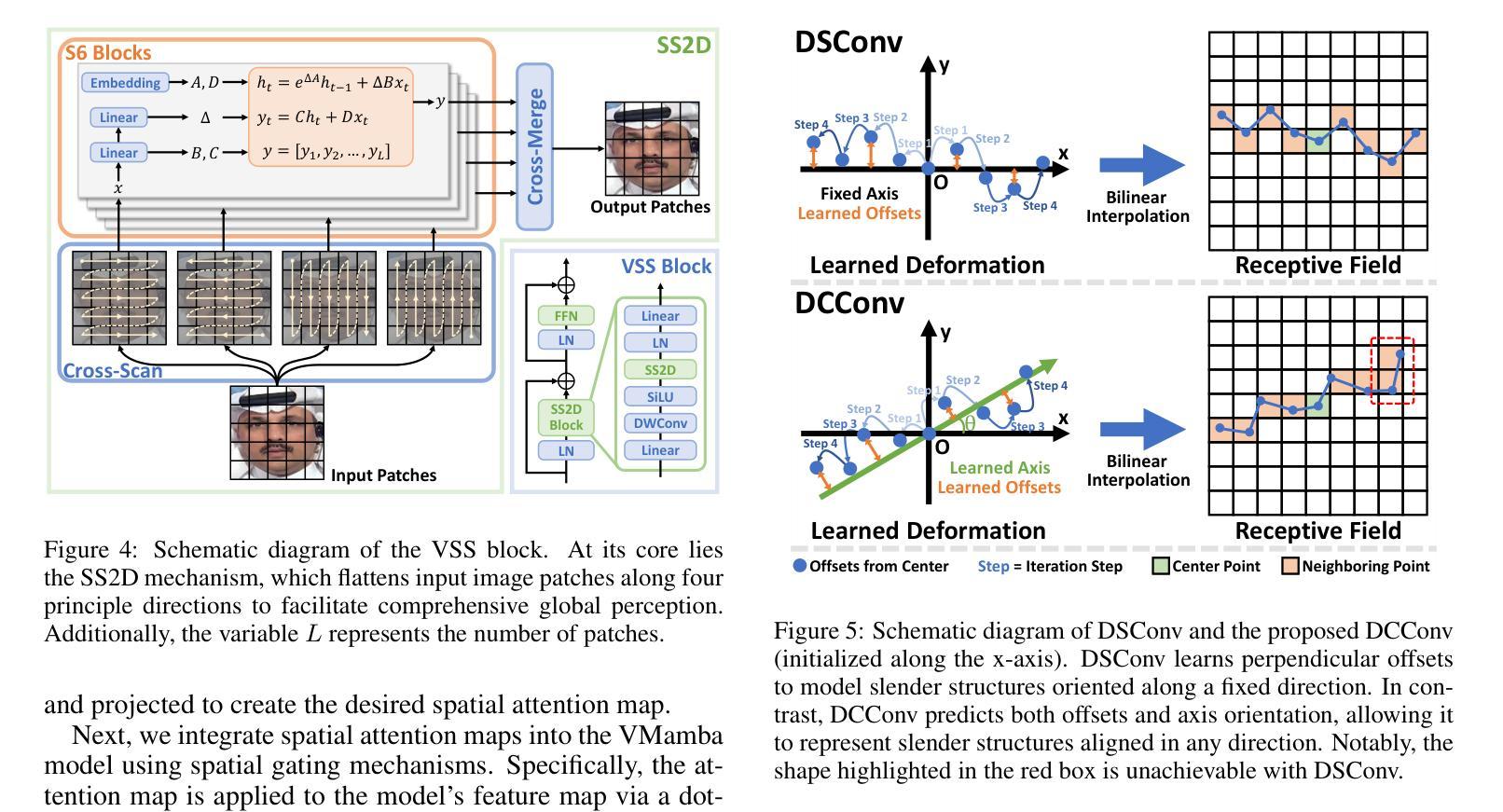
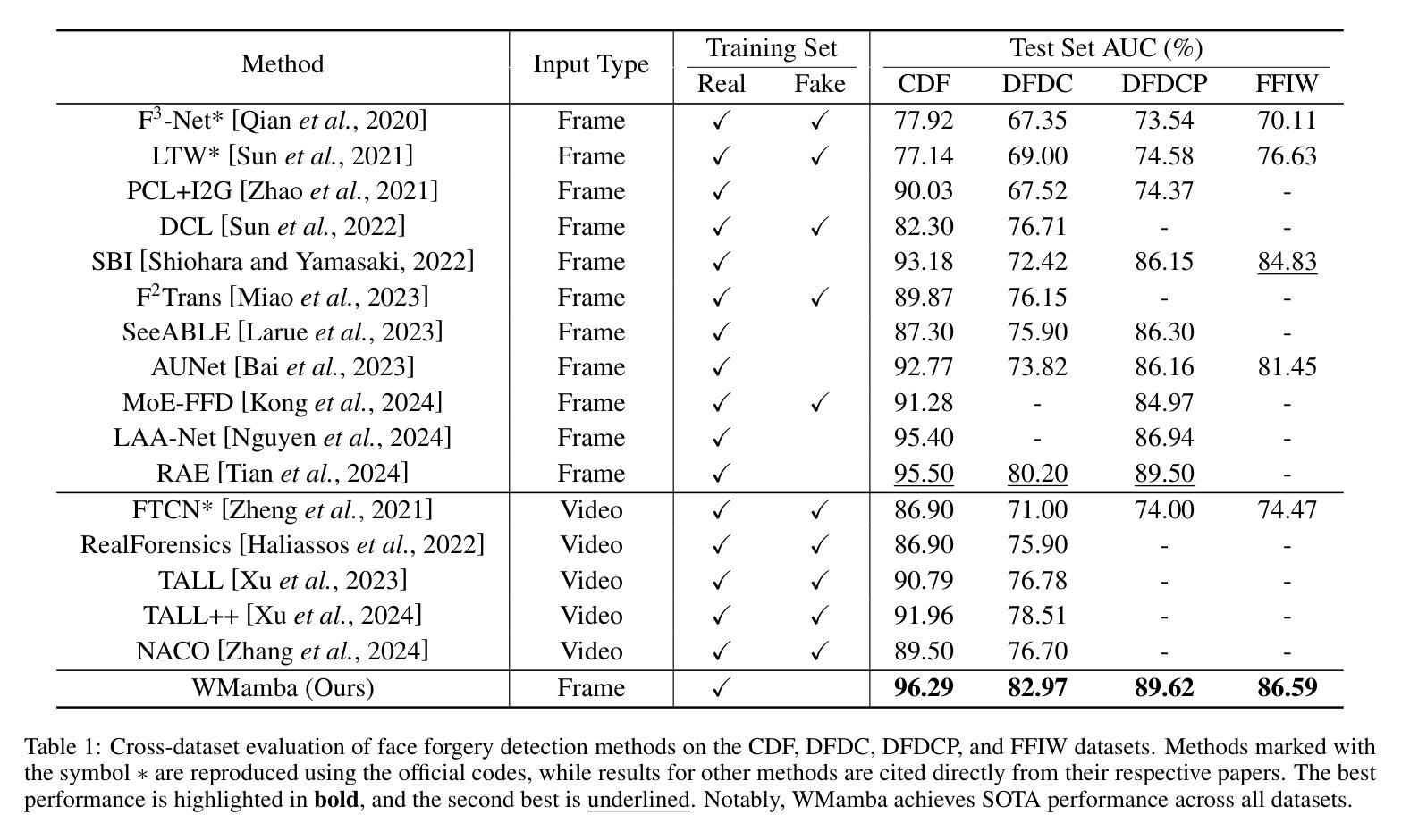
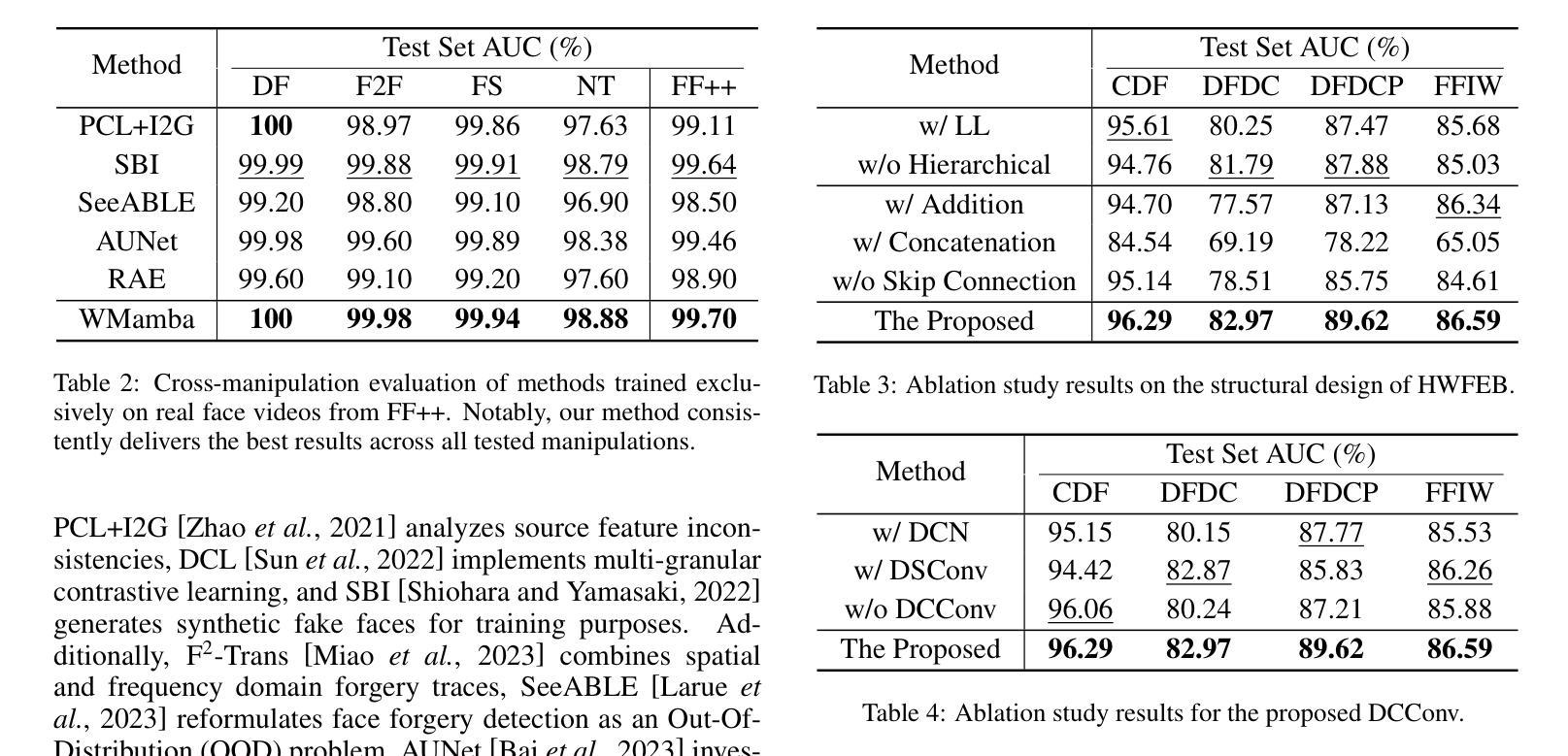